SIA and the Two Dimensional Doomsday Argument
post by KatjaGrace · 2010-11-11T22:00:17.000Z · LW · GW · 0 commentsThis post might be technical. Try reading this if I haven’t explained everything well enough.
When the Self Sampling Assumption (SSA) is applied to the Great Filter it gives something pretty similar to the Doomsday Argument, which is what it gives without any filter. SIA gets around the original Doomsday Argument. So why can’t it get around the Doomsday Argument in the Great Filter?
The Self Sampling Assumption (SSA) says you are more likely to be in possible worlds which contain larger ratios of people you might be vs. people know you are not*.
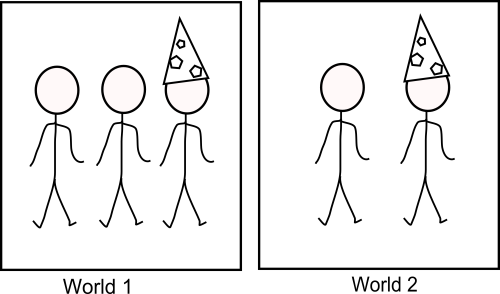
If you have a silly hat, SSA says you are more likely to be in world 2 - assuming Worlds 1 and 2 are equally likely to exist (i.e. you haven't looked aside at your companions), and your reference class is people.
The Doomsday Argument uses the Self Sampling Assumption. Briefly, it argues that if there are many generations more humans, the ratio of people who might be you (are born at the same time as you) to people you can’t be (everyone else) will be smaller than it would be if there are few future generations of humans. Thus few generations is more likely than previously estimated.
An unusually large ratio of people in your situation can be achieved by a possible world having unusually few people unlike you in it or unusually many people like you, or any combination of these.
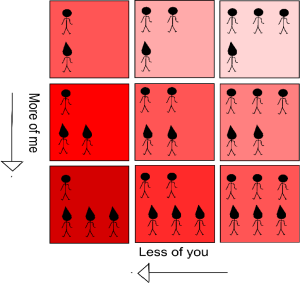
Fewer people who can't be me or more people who may be me make a possible world more likely according to SSA.
For instance on the horizontal dimension, you can compare a set of worlds which all have the same number of people like you, and different numbers of people you are not. The world with few people unlike you has the largest increase in probability.
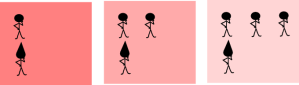
The top row from the previous diagram. The Doomsday Argument uses possible worlds varying in this dimension only.
The Doomsday Argument is an instance of variation in the horizontal dimension only. In every world there is one person with your birth rank, but the numbers of people with future birth ranks differ.
At the other end of the spectrum you could be comparing worlds with the same number of future people and vary the number of current people, as long as you are ignorant of how many current people there are.
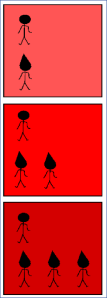
The vertical axis. The number of people in your situation changes, while the number of others stays the same. The world with a lot of people like you gets the largest increase in probability.
This gives a sort of Doomsday Argument: the population will fall, most groups won’t survive.
The Self Indication Assumption (SIA) is equivalent to using SSA and then multiplying the results by the total population of people both like you and not.
In the horizontal dimension, SIA undoes the Doomsday Argument. SSA favours smaller total populations in this dimension, which are disfavoured to the same extent by SIA, perfectly cancelling.
[1/total] * total = 1
(in bold is SSA shift alone)
In vertical cases however, SIA actually makes the Doomsday Argument analogue stronger. The worlds favoured by SSA in this case are the larger ones, because they have more current people. These larger worlds are further favoured by SIA.
[(total – 1)/total]*total = total – 1
The second type of situation is relatively uncommon, because you will tend to know more about the current population than the future population. However cases in between the two extremes are not so rare. We are uncertain about creatures at about our level of technology on other planets for instance, and also uncertain about creatures at some future levels.
This means the Great Filter scenario I have written about is an in between scenario. Which is why the SIA shift doesn’t cancel the SSA Doomsday Argument there, but rather makes it stronger.
Expanded from p32 of my thesis.
——————————————-
*or observers you might be vs. those you are not for instance – the reference class may be anything, but that is unnecessarily complicated for the the point here.

0 comments
Comments sorted by top scores.