King David's %: Establishing a new symbol for Bayesian probability.
post by Paul Logan (paul-logan) · 2022-06-26T19:47:57.047Z · LW · GW · 1 commentsThis is a link post for https://laulpogan.substack.com/p/king-davids-establishing-a-new-symbol
Contents
“The old Frequentist vs. Bayesian debate” Bayes to the back now y’all ‰ ‰ 👀 How do I type that thing? Shift ⇧, Option ⌥, R. Calibration, Luria, and Linda “Percentage points better define belief”- Why stop there? Conclusion Bayesian: ‰ Frequentist: % None 1 comment
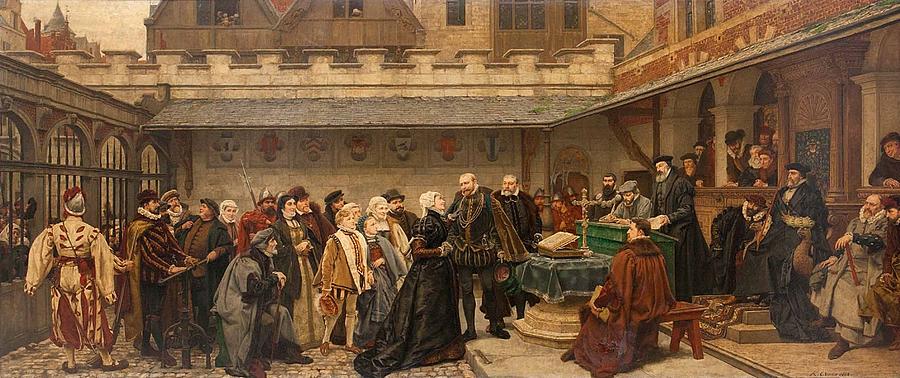
“The old Frequentist vs. Bayesian debate”
Disclaimer #1 (Bias): I’m not a huge fan of using expert testimony to establish predictions about what will happen in the future. That said, I respect the Bayesian hustle- it’s more pragmatic and dabbles less in empirical fetishism. Though I associate frequentist methods with objectivity here, I acknowledge experiment design is a subjective process and that it’s impossible for something to be well and truly “objective.”
Disclaimer #2 (Context): Establishing a dichotomy of Bayesian vs. Frequentist probability is convenient but misleading. Bayesian probability is a singular method, Frequentist probability represents an entire family of methods. There exist other approaches besides these two, such as classical probability and propensity probability. All of these probabilities pass % around like green apple vodka in a highschooler’s basement.
This take confuses me a bit because it seems to conflate two different types of probability: level of conviction (Bayesian) and likelihood from projection (Frequentist). Using the same sign to model opinion and statistical inference has always felt weird to me. On one side of the coin, you have a completely subjective opinion, not at all tied to empirical facts and figures (bare with me here Bayesians, we’re circling back), on the other you have a rigid probability based on empirical observation.
If you flip a coin 10,000 times and 5050 are heads and 4950 are tails, a frequentist might declare “there will always be a 49.5% of the coin landing on tails. If we flipped 100,000 coins, roughly 49,500 would land tails.” A Bayesian who knows a thing or two about coin flips might look the first statistician in the eye and say “Wanna bet?”
Disclaimer #3 (Correctness): I’m not a statistician, and I did my best to frame the difference between these two approaches in the way that was most helpful for me to initially understand. I know this doesn’t discuss P values, but I don’t think that information is especially helpful in helping the uninitiated understand. If you have a better paragraph-long explanation please comment/reach out so I can update this post.
Why the hell would you ever use the same symbol to denote these two ideas? Well, they crawled out of the same academic primordial sludge: statistics. It makes sense that within the confines of the domain of origin everyone can be reasonable, rational adults that understand the difference. When we step out of stats-land though, things get rough.
Bayes to the back now y’all
Disclaimer #4 (Evidence): Tonight’s post isn’t going to include investigation into the societal burden that comes from confusing these two types of probability, I’m planning another post for that.
Scott’s old posts offer solid defense for co-mingling the two concepts: all decisions are predictions. But that’s in a perfect, frictionless, rational world. That’s unfortunately not the world we inhabit.
A friend of mine recently took a new role at a new company, where he is now a lead. As the head of a department he is having to adjust to the problem that sometimes people take his wild speculation or brainstorming more seriously than he does. Anyone speaking publicly using probability has this same issue. Though their intention may be pure, their context comes out in the wash, and others will take action on their words.
Laymen like myself don’t have the context to quickly differentiate between a probability representing a belief and a probabilistic experimental result. I talk a bit further down about how the willfully ignorant get a lot of implicit benefit from these two meanings sheltering under one innocuous symbol. It’s not Bayes’ fault that there isn’t good public education around statistics.
I think we need to split % into two symbols, Bayesian and non-Bayesian. One representing qualitative reasoning, reasoning from subjective observation, and one representing quantitative reasoning, reasoning from objective results.
“Gut check, how sure do you feel?” vs. “When considering past data, what is the forecasted likelihood of this event?”
Problem is, we want to retain some meaning, and we need to be able to type it. Lucky us, there’s already an answer on the Ascii table, the Per Mille symbol:
‰
For those of you upset because this already has meaning in statistics, please remember we’re trying to establish meaning for laymen as well, who suffer without it. Also, I really like this one because it looks like the :eyes: emoji, which we often use to convey suspicion.
‰ 👀
So here’s the proposition: from now on, when you’re talking about a Bayesian, belief-based probability, type ‰. When you’re talking about a frequentist, empirical probability, type %.
Disclaimer #4 (Efficacy): Keeping one type of probability under the old symbol is probably a bad idea because adoption will never be universal and we’ll just create more confusion. So I’m open to other symbol suggestions!
How do I type that thing?
On Windows:
Hold alt and type 0137.
Alternatively, hold the windows key and the period key to bring up the emoji keyboard, find ‰ under symbols, which is a tab labeled with an omega (Ω).
On Mac:
Shift ⇧, Option ⌥, R.
Hit Ctrl ⌃, Command ⌘, Space to bring up the emoji keyboard, then find ‰ under “Punctuation.”
Calibration, Luria, and Linda
“Now wait a minute,” you might say, “ What about Brier score? Scott Alexander calibrates his predictions annually. Prediction isn’t just subjective, it’s a skill that we can measure!”
We’re getting into the entire map-territory distinction here in that we have to strike a balance between pragmatism and accuracy. I’m not saying we should completely throw up our hands and declare helplessness like Uzbek peasants[1] when asked about what colors bears should be in the north.1 I’m just saying we can only help our arguments by being more clear about the process and reasoning we used to arrive at them.
If you’re worried that your arguments will be less effective when they are more difficult to misrepresented as empirically derived, then you probably have some more significant things to worry about. The Linda Problem (or conjunction fallacy)is the perfect example of how clarifying probability types can change experimental and social results. When posited in the form of frequencies rather than just saying “which is more probable” the fallacy demonstrated in previous trials at 85% completely disappeared.
“Percentage points better define belief”- Why stop there?
I agree with this argument. To take it further, I think there’s an argument to be made for conveying maximal context in sharing information. I would rather have information translated to me with a gut feeling of how confident the source is in it. Maybe the internet would be a much better place if we all had chips in our brains that put a surety level next to every statement that could be read as fact (30‰).
Of course, that system would be unfairly weighted towards determined idiots and the willfully ignorant (100‰). We want to know if someone both believes and has sufficiently considered the information they pass on (65‰, 45s). So, maybe we also want the chips to give us a “time in seconds spent considering this concept before communicating it”. [2]
I think what we are really seeking here is context (66‰, 10s). As information conglomerates, it very quickly becomes decontextualized (30‰, 22s). This is my biggest complaint about using probability for confidence in belief as opposed to a representation of likelihood. Once a statistic has been decontextualized, it becomes difficult to tell which is which without chasing down sources and peeling back their methodology (25‰, 6s).
Scott tends to do this well and I think that’s why a lot of people like him (30‰, 3s). He’s in rare form (80‰, 2s) in his most recent book review, in which he leaves some of the author’s statistics seeming anemic, and supports others while taking the conclusions drawn to task. Would Scott’s review have been easier to write with differentiation between Bayesian and frequentist probability? Now that I’m thinking about it, maybe not (13‰, 1m30s).
This last section of the article spun out into fantasy about brain chips, but I think the idea that we want to provide as much context as possible to our audience holds true. We probably also want to include the 24hr google search history of the speaker leading up to when a given statement is made, along with their historic success at prediction. 😛
Conclusion
Let’s be more specific with our probability symbol:
Bayesian: ‰
Frequentist: %
- ^
I hate the Uzbek peasant dialogue. Like, really, viscerally hate it. /u/fubo puts it best. Why are we still talking about villagers’ response to questioning by an official-ish stranger from the imperial core responsible for the tyranny that had so thoroughly raped their way of life? That is well known for using logic traps as evaluations for party loyalty, which can result in a one way trip to Siberia? Don’t forget that Luria became a doctor in the first place because they were murdering all the geneticists.
- ^
Let’s assume the magic chip gives us a time that’s weighted relative to the subject’s average thinking speed, adjusted against a population norm (don’t worry if you’re confused, I am too, this sentence is absolute gobbledygook). This way slow thinkers don’t get to seem more insightful.
1 comments
Comments sorted by top scores.
comment by ChristianKl · 2022-07-02T07:50:27.877Z · LW(p) · GW(p)
“Gut check, how sure do you feel?” vs. “When considering past data, what is the forecasted likelihood of this event?”
This sounds like a strawman. Bayesians do consider past data as well. If you read about Superforcasting, finding the right reference class and reasoning based on it is a key feature.
I think we need to split % into two symbols, Bayesian and non-Bayesian. One representing qualitative reasoning, reasoning from subjective observation, and one representing quantitative reasoning, reasoning from objective results.
The bayesian rule allows for engaging in quantitative reasoning just fine. While you can argue that choosing priors is a subjective act, so is choosing the distribution in frequentist statistics. Usually, frequentists model things that are not normally distributed with the normal distribution and just hope the modeling error is tolerable so they are not matching any objective truth either. Even the archetypical example of the normal distribution, human height is not normally distributed as there are a lot more dwarfs than the distribution would suggest.