Why aren’t we testing general intelligence distribution?
post by B Jacobs (Bob Jacobs) · 2020-05-26T16:07:30.833Z · LW · GW · 1 commentThis is a question post.
Contents
Answers 36 Vaniver 14 Kaj_Sotala 4 James_Miller 2 George None 1 comment
IQ supposedly measures general intelligence. Assuming the g-factor exists, why are we using IQ to measure it? IQ tests get tweaked so the end result will always form a normal distribution. I checked wikipedia to figure out why, but while it said some people claim that LCT is a good reason to believe the g-factor will have a normal distribution, it didn’t seem terribly confident (and it also didn’t give a source). Even “The Bell Curve” used an intelligence test that didn’t return a bell curve at all. They said they had to turn it into a bell curve to prevent 'skew':
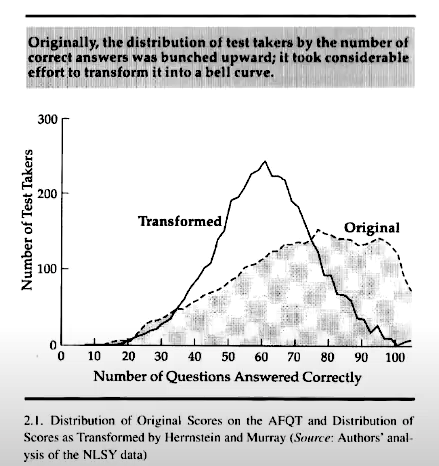
But isn’t this putting the cart before the horse? Surely it would be interesting to see what distributions we would get if we did intelligence testing without a result in mind? I get that it is really difficult to measure, but if a test consistently gave us a different distribution, we could learn valuable insights into how human minds work. So is there a reason why we aren’t testing the hypothesis that general intelligence will follow a normal distribution?
Answers
First, you might be interested in tests like the Wonderlic, which are not transformed to a normal variable, and instead use raw scores. [As a side note, the original IQ test was not normalized--it was a quotient!--and so the name continues to be a bit wrong to this day.]
Second, when we have variables like height, there are obvious units to use (centimenters). Looking at raw height distributions makes sense. When we discover that the raw height distribution (split by sex) is a bell curve, that tells us something about how height works.
When we look at intelligence, or results on intelligence tests, there aren't obvious units to use. You can report raw scores (i.e. number of questions correctly answered), but in order for the results to be comparable the questions have to stay the same (the Wonderlic has multiple forms, and differences between the forms do lead to differences in measured test scores). For a normalized test, you normalize each version separately, allowing you to have more variable questions and be more robust to the variation in questions (which is useful as an anti-cheating measure).
But 'raw score' just pushes the problem back a step. Why the 50 questions of the Wonderlic? Why not different questions? Replace the ten hardest questions with easier ones, and the distribution looks different. Replace the ten easiest questions with harder ones, and the distribution looks different. And for any pair of tests, we need to construct a translation table between them, so we can know what a 32 on the Wonderlic corresponds to on the ASVAB.
Using a normal distribution sidesteps a lot of this. If your test is bad in some way (like, say, 5% of the population maxing out the score on a subtest), then your resulting normal distribution will be a little wonky, but all sufficiently expressive tests can be directly compared. Because we think there's this general factor of intelligence, this also means tests are more robust to inclusion or removal of subtests than one might naively expect. (If you remove 'classics' from your curriculum, the people who would have scored well on classics tests will still be noticeable on average, because they're the people who score well on the other tests. This is an empirical claim; the world didn't have to be this way.)
"Sure," you reply, "but this is true of any translation." We could have said intelligence is uniformly distributed between 0 and 100 and used percentile rank (easier to compute and understand than a normal distribution!) instead. We could have thought the polygenic model was multiplicative instead of additive, and used a lognormal distribution instead. (For example, the impact of normally distributed intelligence scores on income seems multiplicative, but if we had lognormally distributed intelligence scores it would be linear instead.) It also matters whether you get the splitting right--doing a normal distribution on height without splitting by sex first gives you a worse fit.
So in conclusion, for basically as long as we've had intelligence testing there have been normalized and non-normalized tests, and today the normalized tests are more popular. From my read, this is mostly for reasons of convenience, and partly because we expect the underlying distribution to be normal. We don't do everything we could with normalization, and people aren't looking for mixture Gaussian models in a way that might make sense.
It has been some years since I looked at the literature, but if I recall correctly, the problem is that g is defined on a population level rather than on the individual level. You can't directly measure someone's raw g because the raw g is meaningless by itself.
Suppose that you have an intelligence test composed of ten subtests, each of which may earn a person up to 10 points, for a total of 100 points. You give that test to a number of people, and then notice that on average, a person doing well on one of the subtests means that they are likely to do better on the other subtests as well. You hypothesize that this is explained by a "general intelligence factor". You find that if you assign each of your test-takers an "intelligence score", then the average subtest score of all the test-takers who share that level of intelligence is some subtest-dependent constant times their "intelligence score".
Let's say that I was one of the people taking your test. Your equations say that I have a g of 2. Subtest A has a factor loading of 1, so I should get 1 * 2 = 2 points on it. Subtest B has a factor loading of 4.5, so I should get 4.5 * 2 = 9 points on it. It turns out that I actually got 9 points on subtest A and 2 points on subtest B, exactly the opposite pattern than the one you predicted! Does this mean that you have made an error? No, because the factor loadings were only defined as indicating the score averaged over all the test-takers who shared my estimated g, rather than predicting anything definite about any particular person.
This means that I can get a very different score profile from my estimated g would predict, for as long as enough others with my estimated g are sufficiently close to the estimate. So my estimated g is not very informative on an individual level. Compare this to measuring someone's height, where if they are 170 cm on one test, they are going to be 170 cm regardless of how you measure it.
Now suppose that you are unhappy with the subtests that you have chosen, so you throw out some and replace them with new ones. It turns out that the new ones are substantially harder: on the old test, people got an average g of 4, but now they only get an average of 2. How do you compare people's results between the old and the new test? Especially since some people are going to be outliers and perform better on the new test - maybe I get lucky and increase my g to a 3. It's as if we were measuring people's heights in both meters and feet, but rather than one straightforwardly converting to another, two people with the same height in meters might have a different height in feet.
Worse, there's also no reason why the intelligence test would have to have 10 subtests that award 10 points each. Maybe I devise my own intelligence test: it has 6 subtests that give 0-6 points each, 3 subtests that give 2-8 points each, and 4 subtests that give 0-20 points each. The resulting raw score distributions and factor loadings are going to be completely different. How do you compare people's results on your old test, your new test, and my test?
Well, one way to compare them would be to just say that you are not even trying to measure raw g (which is not well-defined for individuals anyway), you are measuring IQ. It seems theoretically reasonable that whatever-it-is-that-intelligence-tests-measure would be normally distributed, because many biological and psychometric quantities are, so you just define IQ as following a normal distribution and fit all the scores to that. Now we can at least say that "Kaj got an IQ of 115 on his own test and an IQ of 85 on Bob's test", letting us know that I'm one standard deviation above the median on my test and one standard deviation below it on your test. That gives us at least some way of telling what the raw scores mean.
Suppose that you did stick to just one fixed test, and measured how the raw scores change over time. This is something that is done - it's how the Flynn effect was detected, as there were increasing raw scores. But there are also problems with that, as seen from all the debates over what the Flynn effect changes actually mean.
Let's say that an intelligence test contains a subtest measuring the size of your vocabulary. The theoretical reason for why vocabulary size is thought to correlate with intelligence is that people learn the meaning of new words by hearing them used in a context. With a higher intelligence, you need to hear a word used fewer times to figure out its meaning, so smarter people will on average have larger vocabularies. Now, words are culturally dependent and people will be exposed to different words just by random chance... but if all of the people who the test was normed on are from the same cultural group, then on average, getting a higher score on the vocabulary subtest is still going to correlate with intelligence.
But suppose that you use exactly the same test for 20 years. The meanings of words change: a word that was common two decades ago might be practically nonexistent today ("phone booth"). Or another might become more common. Or a subtest might measure some kind of abstract reasoning, and then people might start playing puzzle games that feature more abstract logic reasoning. Assuming that people's scores on an IQ test can be decomposed into something like (talent + practice), changes in culture can invalidate intertemporal comparisons by changing the amount of practice people get.
So if someone who was 20 took your test in 2000 and got a raw score of 58, and someone who is 20 takes your test in 2020 and also gets a raw score of 58, this might not indicate that they have the same intelligence either... even if they get exactly the same scores on all the subtests. Periodically re-norming the raw scores helps make them more comparable in this case as well; that way we can at least know that what their ranking relative to other 20-year-olds in the same year was.
If general intelligence is a polygenic trait it will be normally distributed.
↑ comment by Richard_Kennaway · 2020-05-26T17:34:52.629Z · LW(p) · GW(p)
To what extent has that been empirically tested?
The page you linked only gives a weak argument (3 genes to give a normal distribution of colour in maize?) and no references to empirical observations of the distribution. The video on the page, talking about skin colour, does not claim anything about the distribution, beyond the fact that there is a continuous range. Even with all of the mixing that has taken place in the last few centuries, the world does not look to me like skin colour is normally distributed.
Even Fisher's original paper on the subject says only "The great body of available statistics show us that the deviations of a human measurement from its mean follow very closely the Normal Law of Errors" near the beginning, then proceeds with pure mathematics.
I can think of several ways in which a polygenic trait might not be normally distributed. I do not know whether these ever, rarely, or frequently happen. Only a small number of genes involved. Large differences in the effects of these genes. Multiplicative rather than additive affect. The central limit theorem doesn't work so well in those situations.
And the graph of raw scores in the OP is clearly not a normal distribution. Would you justify transforming it into a normal distribution because that is how the "real" thing "must" be distributed? That would render the belief in normal distributions untestable.
↑ comment by B Jacobs (Bob Jacobs) · 2020-05-26T16:59:50.063Z · LW(p) · GW(p)
Wikipedia says:
Research on heritability of IQ implies, from the similarity of IQ in closely related persons, the proportion of variance of IQ among individuals in a population that is associated with genetic variation within that population. This provides an estimate of genetic versus environmental influence for phenotypic variation in IQ in that population as environmental factors may be correlated with genetic factors. "Heritability", in this sense, "refers to the genetic contribution to variance within a population and in a specific environment".[1] In other words, heritability is a mathematical estimate that indicates an upper bound on how much of a trait's variation within that population can be attributed to genes. There has been significant controversy in the academic community about the heritability of IQ since research on the issue began in the late nineteenth century.[2] Intelligence in the normal range is a polygenic trait, meaning that it is influenced by more than one gene,[3][4] specifically over 500 genes.[5]
So while what you say is true, we cannot know that general intelligence is polygenic because this is once again talking about IQ. Of course IQ will imply intelligence is polygenic, because IQ itself has a normal distribution. We are once again putting the cart before the horse.
Basically, the way I would explain it, you are right, using a bell curve and using various techniques to make your data fit it is stupid.
This derives from two reasons, one is am artifact, the fact that distributions were computation-simplyfing mechanisms in the past, even though this is no longer true. More on this here: https://www.lesswrong.com/posts/gea4TBueYq7ZqXyAk/named-distributions-as-artifacts [LW · GW]
This is the same mistake, broadly speaking, as using something like pearsonr instead of an arbitrary estimator (or even better, 20 of them) and a k-fold-crossvalidation in order to determine "correlation" as a factor of the predictive power of the best models.
Second, and see an SSC post on this that does the subject better justice (completely missing the point), we love drawing metaphorical straight line, we believe and give social status to people that do this.
If you were to study intelligence with an endpoint/goal in mind, or with the goal of explaining the world, the standard dist would be useless. Except for one goal, that of making your "findings" seem appealing, of giving them extra generalizability/authorizativeness that they lack, normalizing tests and results to fit the bell curve does exactly that.
1 comment
Comments sorted by top scores.
comment by gwern · 2020-05-26T18:25:01.616Z · LW(p) · GW(p)
Even “The Bell Curve” used an intelligence test that didn’t return a bell curve at all. They said they had to turn it into a bell curve to prevent 'skew'
Isn't that just a ceiling effect? They have roughly 100 items, and the AFQT is designed to screen out the dumb (to avoid recruiting them into the military), not measure the normal or the brilliant. So the right tail gets chopped off and heaped (everyone answering 90-100 questions right; remember that there's always random error in answering individual questions), even though the rest looks just like a normal distribution.