Active Inference as a formalisation of instrumental convergence
post by Roman Leventov · 2022-07-26T17:55:58.309Z · LW · GW · 2 commentsThis is a link post for https://direct.mit.edu/books/oa-monograph/5299/Active-InferenceThe-Free-Energy-Principle-in-Mind
Contents
Active Inference as a formalisation of instrumental convergence Preferences in Active Inference AGI and Active Inference None 2 comments
The goal of this post is mainly to increase the exposure of the AI alignment community to Active Inference theory, which seems to be highly relevant to the problem but is seldom mentioned on the forum.
This post links to a freely available book about Active Inference, published this year. For alignment researchers, the most relevant chapters will be 1, 3, and 10.
Active Inference as a formalisation of instrumental convergence
Active Inference is a theory describing the behaviour of agents that want to counteract surprising, “entropic” hits from the environment via accurate prediction and/or placing themselves in a predictable (and preferred) environment.
Active Inference agents update their beliefs in response to observations (y), update the parameters and shapes of their models Q and P (which can be seen as a special case of updating beliefs), and act so that they minimise the expected free energy, G:
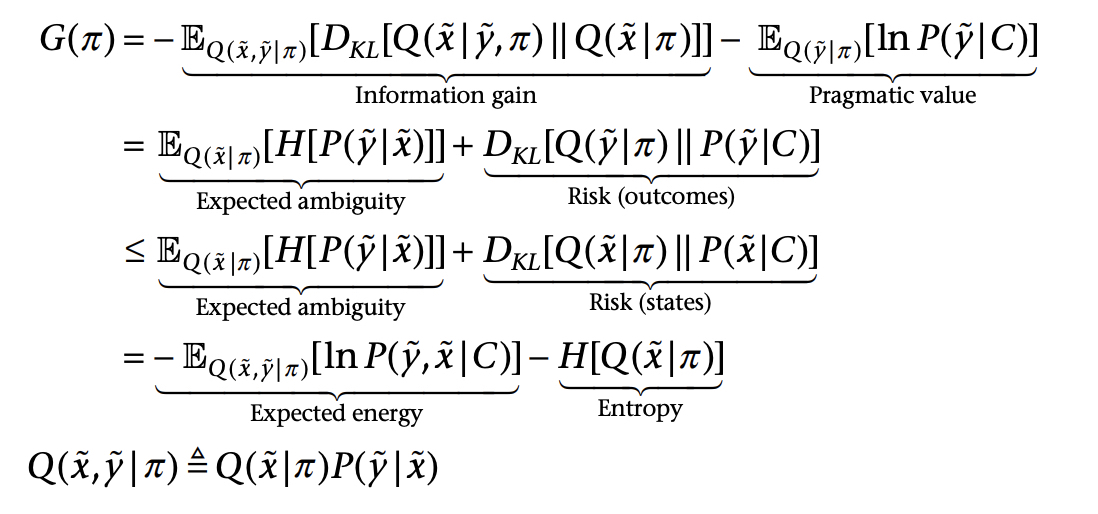
Where are the hidden states of the world, is a sequence or trajectory of the hidden states over some time period in the future (not specified), are the agent’s observations, is the sequence or trajectory of the agent’s expected observations in the future, is the agent’s generative model of the world’s dynamics (including themselves), is the agent’s generative model of the observations from the hidden states, is an action plan (called policy in the Active Inference literature) that the agent considers (the agent chooses the plan that entails the minimal expected free energy), is the distribution of beliefs over the hidden states over a period of time in the future, are agent’s preferences or prior beliefs.
Active Inference framework is agnostic about the time period over which the expected free energy is minimised. Intuitively, that should be the agent’s entire lifetime, potentially indefinite in the case of AI agents. The expected free energy for the indefinite time period diverges, but the agent can still follow a gradient on it by improving its capacity to plan accurately farther into the future and to execute its plans.
Therefore, we can equate instrumental convergence from the AI alignment discourse with agents minimising their expected free energy in the Active Inference framework.
Preferences in Active Inference
For biological agents, designate the agent’s preferences over the external and internal conditions necessary (or optimal) for their survival, procreation, and other intrinsic goals. For humans, these are, for instance, the external temperature between 15 and 30 °C, the body temperature of about 37 °C, blood fluidity within a certain range, and so on. In humans and likely some other animals, there also exist preferred psychological states.
The “intrinsic goals” (also called implicit priors in the Active Inference literature) referenced in the previous paragraph are not explicitly encoded in Active Inference. They are shaped by evolution and are only manifested in the preferences over hidden states and observations, .
The important implicit prior in humans is the belief that one is a free energy minimising agent (note how this is a preference over an abstract hidden state, “the kind of agent I am”). It’s not clear to me whether there is any non-trivial substance in this statement, apart from that the adaptive behaviour in agents can be seen as surprise minimisation, and therefore minimising the expected free energy.
In the literature, Active Inference is frequently referred to as a “normative” theory or framework. I don’t understand what it means, but it might be related to the point in the previous paragraph. From the book:
Active Inference is a normative framework to characterize Bayes-optimal behavior and cognition in living organisms. Its normative character is evinced in the idea that all facets of behavior and cognition in living organisms follow a unique imperative: minimizing the surprise of their sensory observations.
To me, the second sentence in this quote is a tautology. If it refers to the fact that agents minimise the expected free energy in order to survive (for longer), then I would call this a scientific statement, not a normative statement. (Update: see The two conceptions of Active Inference: an intelligence architecture and a theory of agency [LW · GW] for an update on the normative and physical nature of Active Inference.)
AGI and Active Inference
If an AGI is an Active Inference agent and it has a prior that it's a free energy minimising agent, it can situationally prefer this prior over whatever other "alignment" priors are encoded into it. And even if this is not encoded in the agent's preferences , a sufficiently intelligent Active Inference agent will probably form such a belief upon reading the literature itself (or even from a "null string").
I don't understand whether AGI agents must unavoidably be Active Inference agents, and, therefore, exhibit instrumental convergence. Unconstrained Reinforcement Learning probably leads to the creation of Active Inference agents, but if an explicit penalty is added during the training for approaching the shape of an Active Inference agent, maybe an RL agent can still learn to solve arbitrary problems (though it's not clear how this penalty could be added if the agent is not engineered as an Active Inference agent with explicit Q and P models in the first place).
Active Inference suggests an idea for alignment: what if we include humans into AGI's Markov blanket? This probably implies a special version of an Oracle AI [? · GW] which cannot even perceive the world other than through humans, i. e. via talking or chatting to humans only. I haven't reviewed the existing writing on Oracle AIs and don't know if Active Inference brings some fresh ideas to it, though.
2 comments
Comments sorted by top scores.
comment by Fabbun · 2022-12-02T00:56:56.743Z · LW(p) · GW(p)
Are you sure that P(x|y) is the agents generative model and not the underlying real probability of state's X given observed y. I ask because I'm currently reading this book and am struggling to follow some of it.
Replies from: Roman Leventov↑ comment by Roman Leventov · 2022-12-03T07:01:42.834Z · LW(p) · GW(p)
I don't know what the "underlying real probability" is (no condescendence in this remark; I'm genuinely confused about the physics and philosophy of probability and haven't got time to figure it out for myself, and I'm not sure this is a settled question).
Both P and Q are something that is implemented (i. e., encoded in some way) by the agent itself. The agent knows nothing about the "true generative model" of the environment (even if we can discuss it; see below). The only place where "the feedback from the environment" enters this process is in the calculation of , so-called "posterior" belief, which is calculated according to the rules of Bayesian inference. This is the place where the agent is "ensured not to detach from the observations", i. e., the reality of its environment.
I would say, the book doesn't do a very good job of explaining this point. I recommend this paper, section 1 ("Basic terminology, concepts, and mathematics"), and appending A ("Additional mathematical details") that make the mathematics of Active Inference really clear, they explain every transition and derivation of the formalism in detail.
Then, even though an agent uses "its own" generative model of the environment, it is expected to track, with some degree of fidelity, the real dynamics of the environment. This is the whole point of Active Inference, of course. I used the phrase "real dynamics" rather than "generative model" because there is philosophical nuance and can make the phrase "generative model of the environment" misleading or confusing to people. There was a paper specifically aimed to clear out to clear this confusion ("A tale of two densities: Active Inference is enactive inference") But I think that attempt failed, i. e. the paper only added more confusion. Instead of that paper, for physical foundations of Active Inference, that also elucidates this dynamics between the agent and the environment, I'd recommend "A free energy principle for generic quantum systems".