Posts
Comments
The Bay Area, where MATS is based, is not the governance hub of the US;
The Bay is an AI hub, home to OpenAI, Google, Meta, etc., and therefore an AI governance hub. Governance is not governments. Important decisions are being made there - maybe more important decisions than in DC. To quote Allan Dafoe:
AI governance concerns how humanity can best navigate the transition to a world with advanced AI systems[1]. It relates to how decisions are made about AI[2], and what institutions and arrangements would help those decisions to be made well.
Also, many, many AI governance projects go hand-in-hand with technical expertise.
Maybe more broadly, AI strategy is part of AI governance.
Agree that this discussion is surprisingly often confusing and people use the terms interchangeably. Unfortunately, readers often referred to our training compute measurement as a measure of performance, rather than a quantity of executed operations. However, I don't think that this is necessarily due to the abbreviations but also due to the lack of understanding of what one measures. Next to making the distinction more clear with the terms, one should probably also explain it more and use terms such as quantity and performance.
For my research, I've been trying to be consistent with FLOPs (smaller case s) referring to the quantity. While FLOPS or FLOP/s refer to the performance: operations per second. (FWIW, during my time in computer engineering, it has been the norm to use FLOPs for quantity and FLOPS for performance.)
The term Petaflop/s-days also helps - outlining how long the performance (Petaflop/s) runs for how many days, therefore measuring a quantity of operations.
Note it gets even more complicated once we take the number representation (floating point 32bit, 16bit, or even bfloat16) into consideration. Therefore, I'm also in favor of maybe switching at one point towards OPs and OP/s and also document the used number representation for actual technical documentation (such as reporting the compute of ML models).
They trained it on TPUv3s, however, the robot inference was run on a Geforce RTX 3090 (see section G).
TPUs are mostly designed for data centers and are not really usable for on-device inference.
I'd be curious to hear more thoughts on how much we could already scale it right now. Looks like that data might be a bottleneck?
Some thoughts on compute:

Gato estimate: 256 TPUv3 chips for 4 days a 24hours = 24'574 TPUv3-hours (on-demand costs are $2 per hour for a TPUv3) =$49'152
In comparison, PaLM used 8'404'992 TPUv4 hours and I estimated that it'd cost $11M+. If we'd assume that someone would be willing to spend the same compute budget on it, we could make the model 106x bigger (assuming Chinchilla scaling laws). Also tweeted about this here.
The size of the model was only(?) limited due to latency requirements for the robotics part.
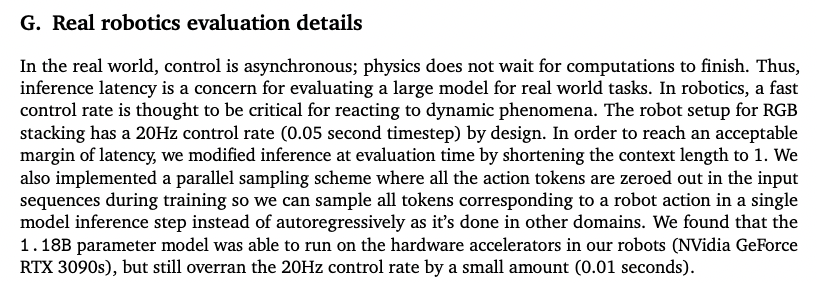
It took Google 64 days to train PaLM using more than 6'000 TPU chips. Using the same setup (which is probably one of the most interconnected and capable ML training systems out there), it'd take 912 years.
I recently estimated the training cost of PaLM to be around $9M to $17M.
Please note all the caveats and this is only estimating the final training run costs using commercial cloud computing (Google's TPUv3).
As already, said a 10T parameter model using Chinchilla scaling laws would be around FLOPs. That's 5200x more compute than PaLM ( FLOPs).
Therefore, .
So a conservative estimate is around $47 to $88 billion.
Thanks for the thoughtful response, Connor.
I'm glad to hear that you will develop a policy and won't be publishing models by default.
Glad to see a new Alignment research lab in Europe. Good luck with the start and the hiring!
I'm wondering, you're saying:
That being said, our publication model is non-disclosure-by-default, and every shared work will go through an internal review process out of concern for infohazards.
That's different from Eleuther's position[1]. Is this a change of mind or a different practice due to the different research direction? Will you continue open-sourcing your ML models?
- ^
"A grassroots collective of researchers working to open source AI research."
From their paper:
We trained PaLM-540B on 6144 TPU v4 chips for 1200 hours and 3072 TPU v4 chips for 336 hours including some downtime and repeated steps.
That's 64 days.
It's roughly an order of magnitude more compute than GPT-3.
ML Model | Compute [FLOPs] | x GPT-3 |
---|---|---|
GPT-3 (2020) | 3.1e23 | 1 |
Gopher (2021-12) | 6.3e23 | ≈2x |
Chinchilla (2022-04) | 5.8e23 | ≈2x |
PaLM (2022-04) | 2.5e24 | ≈10x |
It's to our knowledge now the most compute intensive model ever trained.
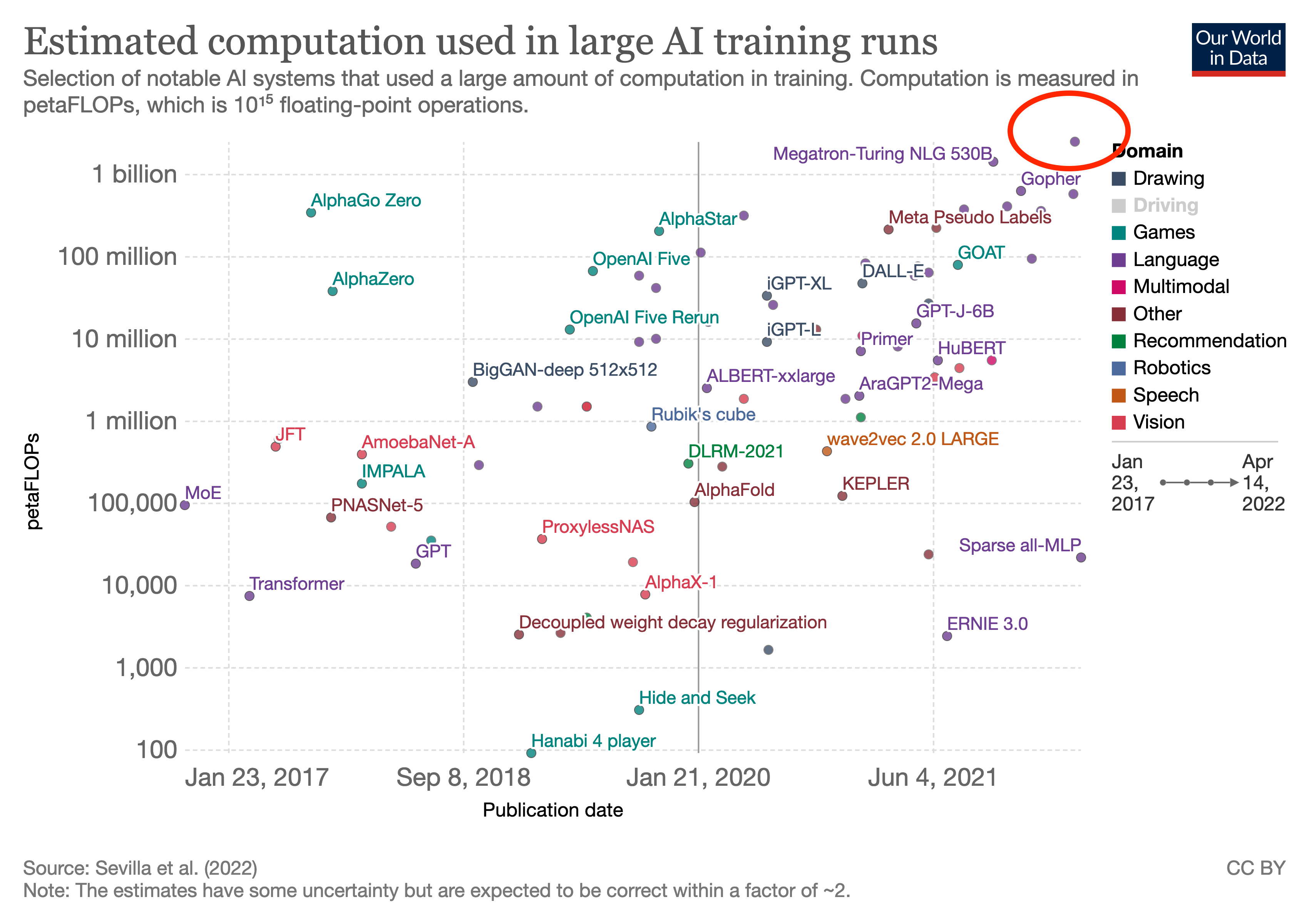
Minor correction. You're saying:
> So training a 1-million parameter model on 10 books takes about as many FLOPS as training a 10-million parameter model on one book.
You link to FLOP per second aka FLOPS, whereas you're talking about the plural of FLOP, a quantity (often used is FLOPs).
I'm wondering: could one just continue training Gopher (the previous bigger model) on the newly added data?
Thanks for the comment! That sounds like a good and fair analysis/explanation to me.
We basically lumped the reduced cost of FLOP per $ and increased spending together.
A report from CSET on AI and Compute projects the costs by using two strongly simplified assumptions: (I) doubling every 3.4 months (based on OpenAI's previous report) and (II) computing cost stays constant. This could give you some ideas on rather upper bounds of projected costs.
Carey's previous analysis uses this dataset from AI Impacts and therefore assumes:
[..] while the cost per unit of computation is decreasing by an order of magnitude every 4-12 years (the long-run trend has improved costs by 10x every 4 years, whereas recent trends have improved costs by 10x every 12 years).
Thanks for sharing your thoughts. As you already outlined, the report mentions at different occasions that the hardware forecasts are the least informed:
“Because they have not been the primary focus of my research, I consider these estimates unusually unstable, and expect that talking to a hardware expert could easily change my mind.”
This is partially the reason why I started looking into this a couple of months ago and still now on the side. A couple of points come to mind:
-
I discuss the compute estimate side of the report a bit in my TAI and Compute series. Baseline is that I agree with your caveats and list some of the same plots. However, I also go into some reasons why those plots might not be that informative for the metric we care about.
-
Many compute trends plots assume peak performance based on the specs sheet or a specific benchmark (Graph500). This does not translate 1:1 to "AI computing capabilities" (let's refer to them as effective FLOPs). See a discussion on utilization in our estimate training compute piece and me ranting a bit on it in my appendix of TAI and compute.
-
I think the same caveat applies to the TOP500. I'd be interested in a Graph500 trend over time (Graph 500 is more about communication than pure processing capabilities).
-
Note that all of the reports and graphs usually refer to performance. Eventually, we're interested in FLOPs/$.
-
Anecdotally, EleutherAI explicitly said that the interconnect was their bottleneck for training GPT-NeoX-20B.
-
-
What do you think about hardware getting cheaper? I summarize Cotra's point here.
- I don't have a strong view here only "yeah seems plausible to me".
Overall, there will either be room for improvement in chip design, or chip design will stabilize which enables the above outlined improvements in the economy of scale (learning curves). Consequently, if you believe that technological progress (more performance for the same price) might halt, the compute costs will continue decreasing, as we then get cheaper (same performance for a decreased price).
Overall, I think that you're saying something "this can't go on and the trend has already slowed down". Whereas I think you're pointing towards important trends, I'm somewhat optimistic that other hardware trends might be able to continue driving the progress in effective FLOP. E.g., most recently the interconnect (networking multiple GPUs and creating clusters). I think a more rigorous analysis of the last 10 years could already give some insights into which parts have been a driver of more effective FLOPs.
For this reason, I'm pretty excited about MLCommons benchmarks or something LambdaLabs -- measuring the performance we might care about for AI.
Lastly, I'm working on better compute cost estimates and hoping to have something out in the next couple of months.
Thanks, appreciate the pointers!
co-author here
I like your idea. Nonetheless, it's pretty hard to make estimates on "total available compute capacity". If you have any points, I'd love to see them.
Somewhat connected is the idea of: What ratio of this progress/trend is due to computational power improvements versus increased spending? To get more insights on this, we're currently looking into computing power trends and get some insights into the development of FLOPS/$ over time.
Comparing custom ML hardware (e.g. Google's TPUs or Baidu's Kunlun, etc) is tricky to put on these sorts of comparisons. For those I think the MLPerf Benchmarks are super useful. I'd be curious to hear the authors' expectations of how this research changes in the face of more custom ML hardware.
I'd be pretty excited to see more work on this. Jaime already shared our hardware sheet where we collect information on GPUs but as you outline that's the peak performance and sometimes misleading.
Indeed, the MLPerf benchmarks are useful. I've already gathered their data in this sheet and would love to see someone playing around with it. Next to MLPerf, Lambda Labs also shares some standardized benchmarks.
Great post! I especially liked that you outlined potential emerging technologies and the economic considerations.
Having looked a bit into this when writing my TAI and Compute sequence, I agree with your main takeaways. In particular, I'd like to see more work on DRAM and the interconnect trends and potential emerging paradigms.
I'd be interested in you compute forecasts to inform TAI timelines. For example Cotra's draft report assumes a doubling time of 2.5 years for the FLOPs/$ but acknowledges that this forecast could be easily improved by someone with more domain knowledge -- that could be you.
Thanks!
- I'm working with a colleague on the trends of the three components (compute, memory, and interconnect) over time of compute systems and then comparing it to our best estimates for the human brain (or other biological anchors). However, this will still take some time but I hope we will be able to share it in the future (≈ till the end of the year).
Thanks for the correction and references. I just followed my "common sense" from lectures and other pieces.
What do you think made AlexNet stand out? Is it the depth and use of GPUs?
Thanks for the feedback, Gunnar. You're right - it's more of a recap and introduction. I think the "newest" insight is probably the updates in Section 2.3.
I also would be curious to know in which aspects and questions you're most interested in.