A comment on Ajeya Cotra's draft report on AI timelines
post by Matthew Barnett (matthew-barnett) · 2022-02-24T00:41:48.192Z · LW · GW · 13 commentsContents
The critique, elaborated A summary of how the compute distribution is constructed My core contention Conclusion None 13 comments
Ajeya Cotra's draft report on AI timelines [LW · GW] is the most useful, comprehensive report about AI timelines I've seen so far. I think the report is a big step towards modeling the fundamental determinants of AI progress. That said, I am skeptical that the arguments provided in the report should reduce our uncertainty about AI timelines by much, even starting from a naive perspective.
To summarize her report, and my (minor) critique,
- Ajeya builds a distribution over how much compute it would take to train a transformative ML model using 2020 algorithms. This compute distribution is the result of combining the distributions under six different biological anchors, and spans over 20 orders of magnitude of compute.
- To calculate when it will it will be affordable to train a transformative ML model, Ajeya guesses (1) how much hardware price-performance will fall in the coming decades, (2) how much algorithmic progress will happen, and (3) how much large actors (like governments) will be willing to spend on training a transformative AI.
- Ajeya says that she spent most of her time building the 2020 compute distribution, and relatively little time forecasting price-performance, algorithmic progress, and willingness to spend parameters of the model. Therefore, it's not surprising that I'd disagree with her estimates.
However, my main contention is that her estimates for these parameters are just point estimates, meaning that our uncertainty over these parameters doesn’t translate into uncertainty in her final bottom-line distribution of when it will become affordable to train a transformative ML model. - Separately, I think the hardware forecast is too optimistic. She assumes that price-performance will cut in half roughly every 2.5 years over the next 50 years. I think it's more reasonable to expect hardware progress will be slower in the future.
- When making the appropriate adjustments implied by the prior two points, the resulting bottom-line distribution is both more uncertain and later in the future. This barely reduces our uncertainty about AI timelines compared to what many (perhaps most?) EAs/rationalists believed prior to 2020.
Upfront, I definitely am not saying that her report is valueless in light of this rebuttal. In fact, I think Ajeya's report is useful because of how it allows people to build their own models on top of it. I also think the report is admirably transparent, and unusually nuanced. I'm just skeptical that we should draw strong conclusions about when transformative AI will arrive based on her model alone (though future work could be more persuasive).
The critique, elaborated
A summary of how the compute distribution is constructed
Ajeya Cotra's model is highly parameterized compared to simple alternatives that have sometimes been given (like some of Ray Kurzweil's graphs). It also gives a very wide distribution over what we should expect.
The core of her model is the "2020 training computation requirements distribution", which is a probability distribution over how much computation it would take to train a transformative machine learning model using 2020 algorithms. To build this distribution, she produces six anchor distributions, each rooted in some analogy to biology.
Two anchors are relatively straightforward: the lifetime anchor grounds our estimate of training compute to how much computation humans perform during childhood, and the evolution anchor grounds our estimate of training compute to how much computation evolution performed in the process of evolving humans from the first organisms with neurons.
Three more anchors (the "neural network anchors") ground our estimate of the inference compute of a transformative model in the computation that the human brain uses, plus an adjustment for algorithmic inefficiency. From this estimate, we can derive the number of parameters such a model might have. Using empirical ML results, we can estimate how many data points it will take to train a model with that number of parameters.
Each neural network anchor is distinguished by how it translates "number of data points used during training" into "computation used to train". The short-horizon neural network assumes that each data point will take very little computation, as in the case of language modeling. The medium and long-horizon neural networks use substantially longer "effective horizons", meaning that they estimate it will take much more computation to calculate a single meaningful data point for the model.
The genome anchor grounds our estimate of the number of parameters of a transformative model to the number of parameters in the human genome. Then, it uses a long effective horizon to estimate how much computation it would take to train this model.
By assigning a weight to each of these anchors, we can construct a probability distribution over the total amount of computation it will take to train a transformative AI using 2020 algorithms. Since the lifetime anchor gives substantial probability on amounts of compute that have already been used in real training runs, Ajeya applies a small adjustment to this aggregate distribution.
The final compute distribution is extremely wide, assigning non-negligible probability between 10^24 FLOPs to 10^50 FLOPs.
My core contention
To translate the compute distribution into a timeline of when it will become affordable to train transformative AI, Ajeya produces three forecasts for the following three questions:
- How quickly will computing prices fall in the coming decades?
- How fast will we make progress in algorithmic efficiency?
- How much will large actors be willing to spend on training a transformative AI?
Ajeya comments that,
These numbers are much more tentative and unstable than my hypothesis distributions, and I expect many readers to have substantially more information and better intuitions about them than I do; I strongly encourage interested readers to generate their own versions of these forecasts with this template spreadsheet.
Her estimates for each of these parameters are simply numerical guesses, ie. point estimates. That means that our uncertainty in these guesses is not reflected in the bottom-line distribution for when training transformative AI should become affordable. But in my opinion, we should be moderately uncertain in each of these parameters.
I think it's inaccurate to convey the final bottom-line probability distribution (the one with a median of about 2052) as representing all of our uncertainty over the variable being modeled. If we were to make some adjustments to the model to account for our uncertainty over these parameters, the bottom-line distribution would get much wider. As a consequence, it's not clear to me that the Bio Anchors model should narrow our uncertainty about AI timelines by an appreciable degree.
To be clear: I'm not claiming that Ajeya makes any claims about Bio Anchors being a final all-thing-considered AI timelines model. Her real claims are quite modest, and she even performs a sensitivity analysis. Still, I think my takeaway from this report is different from how some others have interpreted it.
To give one example of what I see as an inaccurate conclusion being drawn from Ajeya's report, Holden Karnofsky wrote,
Additionally, I think it's worth noting a couple of high-level points from Bio Anchors that don't depend on quite so many estimates and assumptions...
- If AI models continue to become larger and more efficient at the rates that Bio Anchors estimates, it will probably become affordable this century to hit some pretty extreme milestones - the "high end" of what Bio Anchors thinks might be necessary. These are hard to summarize, but see the "long horizon neural net" and "evolution anchor" frameworks in the report.
- One way of thinking about this is that the next century will likely see us go from "not enough compute to run a human-sized model at all" to "extremely plentiful compute, as much as even quite conservative estimates of what we might need."
But, I think the main reason why we hit such high compute milestones by the end of the century in this model is simply that Ajeya assumes that we will have fast progress in computing price-performance in the coming decades; in fact, faster than what we've been used to in the last 10 years. I think this is a dubious assumption.
Under her conservative assumptions from the sensitivity analysis, we don't actually get very close to being able to-run evolution by the end of the century.
Her mainline estimate for price-performance progress is that the price of computation will fall by half roughly every 2.5 years for the next 50 years, after which it will saturate. Her mainline forecast looks like this.
Price performance in the last decade has been quite a lot slower than this. For example, Median Group found a slower rate of price-performance cutting in half about every 3.5 years in the last 10 years. (I'd also expect a newer analysis to show an even slower trend given the recent GPU shortage).
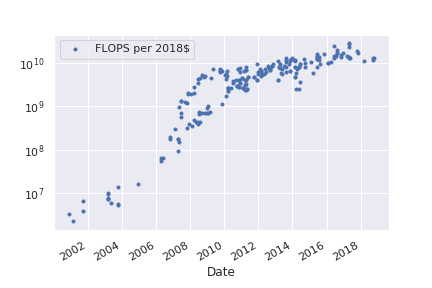
Roughly, the main reason for this slowdown was the end of Dennard scaling, which heavily slowed down single-threaded CPU performance.
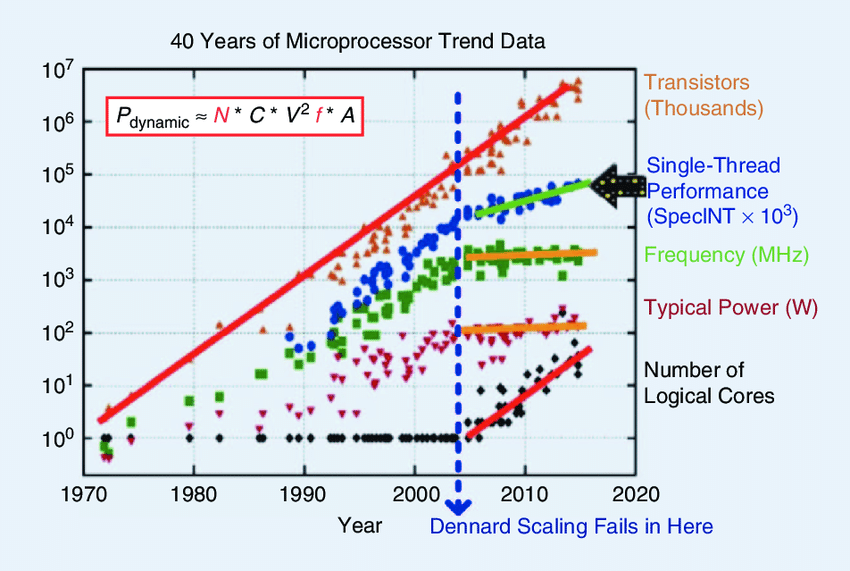
This slowdown is also in line with a consistent slowdown in supercomputing performance, which is plausibly a good reference class for how much computation will be available for AI projects. Here's a chart from Top 500.
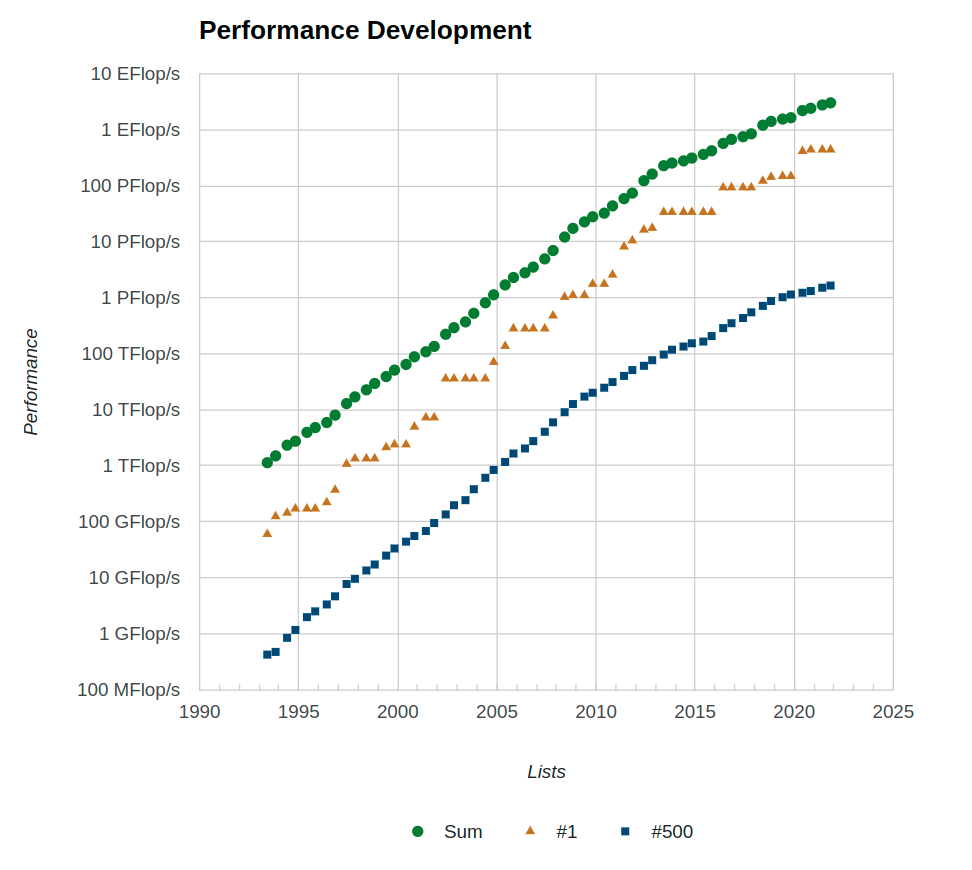
So why does she forecast that prices will cut in half every 2.5 years, instead of a slower rate, matching recent history? In the report, she explains,
This is slower than Moore’s law (which posits a ~1-2 year doubling time and described growth reasonably well until the mid-2000s) but faster than growth in effective FLOP per dollar from ~2008 to 2018 (a doubling time of ~3-4 years). In estimating this rate, I was attempting to weigh the following considerations:
- Other things being equal, the recent slower trend is probably more informative than older data, and is fairly likely to reflect diminishing returns in the silicon chip manufacturing industry.
- However, the older trend of faster growth has held for a much longer period of time and through more than one change in “hardware paradigms.” I don’t think it makes sense to extrapolate the relatively slower growth from 2008 to 2018 over a period of time several times longer than that
- Additionally, a technical advisor informs me that the NVIDIA A100 GPU (released in 2020) is substantially more powerful than the V100 that it replaced, which could be more consistent with a ~2-2.5 year doubling time than a ~3.5 year doubling time.
Of these points, I find point 2 to be a weak argument in favor of fast future growth, and I find point 3 particularly weak. All things considered, I expect the price-performance trend to stay at its current pace (halving every 3-4 years) or to get even slower, as we get closer to saturating with fundamental physical limits. It just seems hard to imagine that, after experiencing the slowdown we saw in the last decade, hardware engineers will suddenly find a new paradigm that enables much quicker progress in the medium-term.
To get more specific, I'd assign about a 20% credence to the hypothesis that price performance trends will robustly get faster in the next 20 years, and maintain that pace for another 30 years after that. Conversely, that means I think there's about an 80% chance that the hardware milestones predicted in this report are too optimistic.
On this basis, I think it is also reasonable to conclude that we should be similarly conservative for other variables that are downstream from hardware progress, which potentially includes algorithmic progress.
Conclusion
I agree that Ajeya Cotra's report usefully rebuts e.g. Robin Hanson's timelines in which AI is centuries away. However, my impression from talking to rationalists prior to 2020 is that most people didn't put much weight on these sorts of very long timelines anyway.
(Of course, the usual caveats apply. I might just not have been talking to the right people.)
Personally, prior to 2020, I thought AI was very roughly 10 to 100 years away, and this report doesn't substantially update me away from this very uncertain view. That's not to say it's not an interesting, useful report to build on. But it's difficult for me to see how the report, on its own, has any major practical implications for when we should expect to see advanced AI.
13 comments
Comments sorted by top scores.
comment by TLW · 2022-02-24T05:39:42.292Z · LW(p) · GW(p)
One other issue with extrapolating exponential compute trends (Moore's Law, FLOP/$, etc): there's a second law that many people don't really know about. (Sometimes called Moore's Second Law, sometimes called Rock's Law.)
For some of these it's entirely plausible that in fact much of our current trend is an artifact of us throwing a higher and higher percentage of our total economy at the problem, and sooner or later we'll hit a rock wall.
The cost of a fab doubles every 4 years or so[1]. The world economy, on the other hand, takes more than 4 years to double in size. Call it every ~25[2] years.
TSMC's fab 18 cost 17 billion and started operation in 2020. 2020 world GDP was ~85 trillion.
Crossover point is at ~2080, where we'd be spending the total world GDP on fabs[3]. Beyond that the extrapolation would indicate that we'd be spending more than our total world GDP on fabs, which is nonsense.
Anything that uses an exponential compute trend for long-term extrapolations needs to justify why they think that e.g. Moore's law holds but, under the same set of assumptions, Rock's law doesn't.
In order to retain a stable % of the overall economy being dedicated to building semiconductor fabs, we'd need to slow down by a factor of 6 or so. If it turns out that trend of a 3.5 year doubling time for FLOPs/$ was fab-driven, for instance, suddenly that doubling time becomes more like twenty years[4].
- ^
You'll see arguments for and against this number. E.g. https://www.mckinsey.com/~/media/McKinsey/Industries/Semiconductors/Our%20Insights/McKinsey%20on%20Semiconductors%20Issue%205%20-%20Winter%202015/McKinsey%20on%20Semiconductors%20Winter%202015.ashx showed 13% per year (~5.5y doubling time) from 2001-2014 (page 33).
- ^
https://www.oecd.org/economy/lookingto2060long-termglobalgrowthprospects.htm says ~3%/year out to 2060, and rule of 72 gives ~24 years doubling time.
- ^
Crossover point is likely well before then - we can't put anywhere near 100% of world GDP into making fabs - but this is a decent upper bound.
- ^
And even then this assumes that our economy can also continue exponentially rising indefinitely, which is substantially less plausible for the next 240 years than it is for the next 40 years.
↑ comment by Hoagy · 2022-02-24T14:17:09.053Z · LW(p) · GW(p)
One response to this is that although the cost of state-of-the-art fabs has gone up exponentially, the productivity of those fabs has gone up exponentially alongside them, meaning that the cost per chip has stayed quite consistent.
One could imagine that this is the ideal tradeoff to make, when the cost of capital is feasible, but that there may be some trade-off towards lower cost, lower productivity fabs that are possible once the capital expenditure to continue Rock's law becomes unsustainable.
Now, for companies investing in new fabs, it would surely be preferably to have multiple smaller and cheaper fabs, so we can conclude that this tradeoff would not be competitive with the theoretical $T fabs. On this model we would see continued robust growth in transistor densities but not a continuation of Moore's law at current pace
There's some interesting detail on the subject in these lectures (in fact I recommend all of them - the details of the manufacturing process are incredibly interesting):
↑ comment by TLW · 2022-02-25T00:48:40.885Z · LW(p) · GW(p)
One could imagine that this is the ideal tradeoff to make, when the cost of capital is feasible, but that there may be some trade-off towards lower cost, lower productivity fabs that are possible once the capital expenditure to continue Rock's law becomes unsustainable.
Economies of scale are a massive effect for a fab. Fabs already target the max productivity/cost point[1]. Trading off lower productivity for lower cost is often doable - but the productivity/cost gets substantially worse.
One response to this is that although the cost of state-of-the-art fabs has gone up exponentially, the productivity of those fabs has gone up exponentially alongside them,
If last decade's fab construction assuming the world economy went solely into fab production was 2 fabs producing X/year each, and this decade's fab construction assuming the world economy went solely into fab production was 0.02 fabs producing 100X/year each, the total output still went down. You can't make 0.02 fabs.
You might be able to make 1 fab 50x cheaper than originally planned, but it'll have substantially worse than 1/50th the throughput[2][3].
On this model we would see continued robust growth in transistor densities but not a continuation of Moore's law at current pace
Not if the increase in transistor densities is enabled by increased amounts of process knowledge gained from pouring more and more money into fabs, for instance.
(Process knowledge feeding back into development and yields is one of the major drivers of large fabs, remember...)
- ^
Or, in some cases, are already targeting suboptimal productivity/cost tradeoffs due to lack of funding or market.
- ^
[Citation Needed], strictly speaking. That being said, this is in my domain.
- ^
Note that, for instance, a modern cutting-edge fab might have 10 EUV machines.
↑ comment by Hoagy · 2022-02-25T09:44:45.319Z · LW(p) · GW(p)
Yeah I don't think we disagree, all I meant to say is that there's a key question of how much productivity/cost is lost when companies are forced to take 'suboptimal' (in a world of unlimited capital) tradeoffs. I agree it's probably substantial, interested in any more detailed understanding/intuition you have on the tradeoff.
Good point about the process knowledge being created slowing as money growth becomes sub-exponential.
Replies from: TLW↑ comment by TLW · 2022-02-26T07:10:53.911Z · LW(p) · GW(p)
Yeah I don't think we disagree, all I meant to say is that there's a key question of how much productivity/cost is lost when companies are forced to take 'suboptimal' (in a world of unlimited capital) tradeoffs.
Fair.
I agree it's probably substantial, interested in any more detailed understanding/intuition you have on the tradeoff.
I wish I could provide more references; it's mostly proprietary unfortunately. This is in my industry. It's not my area of expertise, but it's close enough that I've got a fair amount of knowledge[1].
That being said, a fab isn't just a thousand parts each of which you can either scale down by a factor of 2 or put in half as many of.
It is instructive to look at ASML's annual report: https://www.asml.com/en/investors/annual-report/2021:
- €[2]6,284 million in EUV system sales in 2021, for 42 machines.
- That's €[2]150 million per machine.
- €[2]4,959.6 million in DUV system sales in 2021, for 81 machines.
- That's €[2]61 million per machine.
- EUV versus DUV (NXE 3400C versus NXT 2050i):
- ~10x the power consumption per unit
- ~2.5x the cost per unit
- Some other interesting stats that aren't relevant to my point:
- ~20x the energy use per wafer pass.
- ~40% wafer passes / year / unit
The cost of a single unit is increasingly rapidly (2.5x from DUV to EUV, for instance), and we're only 2.5 orders of magnitude from O(1) total machines sold per year. Once you hit that... we don't know how to scale down an EUV machine, for many reasons[3].
There are alternatives[4], but they are many orders of magnitude slower[5]. We will have to move to alternatives sooner or later... but they all require a lot of R&D to catch up to photolithography.
- ^
And it's annoying because I'm the sort of person who will stop and check every step of the way that yes, X is public information.
- ^
For some reason a lot of places seem to ignore that these reports are in €, not USD?
- ^
For instance: EUV starts with hitting a tin droplet with a sufficiently powerful laser pulse to turn it into plasma which emits the EUV[6]. You swap out for a weaker laser, you don't get less throughput. You get no throughput.
- ^
Electron-beam lithography, for instance, although it also is starting to have problems[7].
- ^
And in many cases are inherently so. E-beam is limited by the combination of a) a very high exposure required to avoid shot noise effects, and b) beam interaction (it's a charged-particle beam. You run multiple beams too close together and they deflect each other.).
- ^
Way oversimplified. "Molten tin droplets of around 25 microns in diameter are ejected from a generator. As they move, the droplets are hit first by a lower-intensity laser pulse. Then a more powerful laser pulse vaporizes and ionizes the flattened droplet to create a plasma that emits EUV light." is a somewhat better explanation, also from said report.
- ^
With classic photolithography you're largely diffraction-limited. There are stopgap approaches to squeeze a little more blood out of the stone (multiple patterning, OPC, etc), but they very much have deminishing returns. Ultimately you have to reduce the wavelength. By the time you hit EUV you start running into problems: stochastic shot noise for one, and you're generating secondary electrons that scatter surprisingly far for two. Unfortunately: two of the major factors limiting e-beam resolution are... stochastic shot noise and that it generates secondary electrons that scatter surprisingly far.
↑ comment by Hoagy · 2022-02-26T10:44:55.520Z · LW(p) · GW(p)
Cheers for all the additional detail and care to explain the situation!
My only other question would be - it seems the natural way to continue the scaling trend would be to learn to stack more and more transistors on top of each other, until a chip becomes a fully 3D object. I understand that the main obstacle at the moment is preventing them from overheating but I've no idea how soluble it is.
Do you have a good understanding or reference for the feasibility of this direction?
Replies from: TLW↑ comment by TLW · 2022-02-26T19:10:37.537Z · LW(p) · GW(p)
I understand that the main obstacle at the moment is preventing them from overheating but I've no idea how soluble it is.
That's a serious theoretical concern way down the line; it's nowhere near the most pressing concern.
The enemy is # of wafer passes necessary, and knock-on effects thereof. If your machine does a million wafer passes a year, it can produce 100k chips a year requiring 10 wafer passes each... or 1 chip requiring 1m wafer passes. (And meanwhile, if each pass has a 1% chance of causing a critical failure, your yield rate is ~90% in the former case, and or so in the latter case.)
No-one has figured out how to do n vertical layers of transistors in sub-O(n) passes. The closest thing to that is 3D NAND, but even there it's still O(n) passes. (There are some theoretical approaches to getting sublinear mask steps with 3d nand, but it still generally requires O(n) other steps.)
(Relevant: https://thememoryguy.com/making-3d-nand-flash-animated-video/ , and his series on 3d nand in general ( https://thememoryguy.com/what-is-3d-nand-why-do-we-need-it-how-do-they-make-it/ ). )
(And 3D nand is very much a best-case in a bunch of ways. It's extremely regular compared to the transistors in the middle of e.g. an ALU, for instance. And doesn't mind the penalty for running in a 40nm process. Etc.)
(And even 3D NAND is hitting scaling limitations. "String stacking" is essentially a tact acknowledgement that you can't move beyond ~128 layers or so, so 'just' put multiple stacks on top of each other on the chip... but this is again O(n) passes / layer on average, just with a lower constant.)
As long as making multiple transistor layers is O(n) passes and time/pass is roughly a plateau, moving to multiple levels doesn't actually help scaling, and meanwhile hurts yield.
comment by Daniel Kokotajlo (daniel-kokotajlo) · 2022-02-24T19:42:40.187Z · LW(p) · GW(p)
I think the point you make is a good one and should increase your credence in AGI/TAI/etc. by 2030. (Because if you take Ajeya's distribution and add more uncertainty to it, the left tail gets thicker as well as the right). I'd love to see someone expand on Ajeya's spreadsheet to include uncertainty in the flops/$ and 2020flops/flop trends.
Re FLOPS-per-dollar trends: My impression is that what we care about is the price of compute specifically for large AGI training runs, which is different from the price of compute in general. (which is what the dataset is presumably about). The price of compute in general may be cutting in half every 3.5 years but thanks to AI-specialized chips and better networking and switching to lower-res floating point operations we can probably do significantly better than that for price of AI-relevant compute. No? (Genuine question, I don't know much about this topic.)
Replies from: daniel-kokotajlo↑ comment by Daniel Kokotajlo (daniel-kokotajlo) · 2022-02-25T17:28:22.807Z · LW(p) · GW(p)
(For the next few years or so at least. Specialization won't allow us to permanently have a faster trend, I think, but maybe for the next ten years...)
comment by lennart · 2022-02-28T10:20:37.706Z · LW(p) · GW(p)
Thanks for sharing your thoughts. As you already outlined, the report mentions at different occasions that the hardware forecasts are the least informed:
“Because they have not been the primary focus of my research, I consider these estimates unusually unstable, and expect that talking to a hardware expert could easily change my mind.”
This is partially the reason why I started looking into this a couple of months ago and still now on the side. A couple of points come to mind:
-
I discuss the compute estimate side of the report a bit in my TAI and Compute series [AF · GW]. Baseline is that I agree with your caveats and list some of the same plots. However, I also go into some reasons why those plots might not be that informative for the metric we care about.
-
Many compute trends plots assume peak performance based on the specs sheet or a specific benchmark (Graph500). This does not translate 1:1 to "AI computing capabilities" (let's refer to them as effective FLOPs). See a discussion on utilization in our estimate training compute piece and me ranting a bit on it in my appendix of TAI and compute [AF · GW].
-
I think the same caveat applies to the TOP500. I'd be interested in a Graph500 trend over time (Graph 500 is more about communication than pure processing capabilities).
-
Note that all of the reports and graphs usually refer to performance. Eventually, we're interested in FLOPs/$.
-
Anecdotally, EleutherAI explicitly said that the interconnect was their bottleneck for training GPT-NeoX-20B.
-
-
What do you think about hardware getting cheaper? I summarize Cotra's point here [AF · GW].
- I don't have a strong view here only "yeah seems plausible to me".
Overall, there will either be room for improvement in chip design, or chip design will stabilize which enables the above outlined improvements in the economy of scale (learning curves). Consequently, if you believe that technological progress (more performance for the same price) might halt, the compute costs will continue decreasing, as we then get cheaper (same performance for a decreased price).
Overall, I think that you're saying something "this can't go on and the trend has already slowed down". Whereas I think you're pointing towards important trends, I'm somewhat optimistic that other hardware trends might be able to continue driving the progress in effective FLOP. E.g., most recently the interconnect (networking multiple GPUs and creating clusters). I think a more rigorous analysis of the last 10 years could already give some insights into which parts have been a driver of more effective FLOPs.
For this reason, I'm pretty excited about MLCommons benchmarks or something LambdaLabs -- measuring the performance we might care about for AI.
Lastly, I'm working on better compute cost estimates and hoping to have something out in the next couple of months.
comment by HoldenKarnofsky · 2022-03-31T21:14:07.059Z · LW(p) · GW(p)
Noting that I commented on this (and other topics) here: https://www.cold-takes.com/replies-to-ancient-tweets/#20220331
Replies from: matthew-barnett↑ comment by Matthew Barnett (matthew-barnett) · 2022-03-31T23:22:27.790Z · LW(p) · GW(p)
Thanks for the thoughtful reply. Here's my counter-reply.
You frame my response as indicating "disagreements". But my tweet said "I broadly agree" with you, and merely pointed out ways that I thought your statements were misleading. I do just straight up disagree with you about two specific non-central claims you made, which I'll get to later. But I'd caution against interpreting me as disagreeing with you by any degree greater than what is literally implied by what I wrote.
Before I get to the specific disagreements, I'll just bicker about some points you made in response to me. I think this sort of quibbling could last forever and it would serve little purpose to continue past this point, so I release you from any obligation you might think you have to reply to these points. However, you might still enjoy reading my response here, just to understand my perspective in a long-form non-Twitter format.
Note: I continued to edit my response after I clicked "submit", after realizing a few errors of mine. Apologies if you read an erroneous version.
My quibbles with what you wrote
You said,
- Barnett's critique doesn't propose an alternative trajectory of hardware progress he thinks is more likely, or spell out what that would mean for the overall forecasts, besides saying that the doubling time has been closer to 3.5 years recently.
- The Bio Anchors report includes a conservative analysis that assumes a 3.5 year doubling time with (I think more importantly) a cap on overall hardware efficiency that is only 4 orders of magnitude higher than today's, as well as a number of other assumptions that are more conservative than the main Bio Anchors report's; and all of this still produces a "weighted average" best guess of a 50% probability of transformative AI by 2100, with only one of the "anchors" (the "evolution anchor," which I see as a particularly conservative soft upper bound) estimating a lower probability.
The fact that the median for the conservative analysis is right at 2100 — which indeed is part of the 21st century — means that when you said, "You can run the bio anchors analysis in a lot of different ways, but they all point to transformative AI this century", you were technically correct, by the slimmest of margins.
I had the sense that many people might interpret your statement as indicating a higher degree of confidence; that is, maybe something like "even the conservative analysis produces a median prediction well before 2100."
Maybe no one misinterpreted you like that!
It's very reasonable for to think that no one would have misinterpreted you. But this incorrect interpretation of your statement was, at least to me, the thinking that I remember having at the time I read the sentence.
This is simply an opinion, and I hope to gain more clarity over time as more effort is put into this question, but I'll give one part of the intuition: I think that conditional on hardware efficiency improvements coming in on the low side, there will be more effort put into increasing efficiency via software and/or via hybrid approaches (e.g., specialized hardware for the specific tasks at hand; optimizing researcher-time and AI development for finding more efficient ways to use compute). So reacting to Bio Anchors by saying "I think the hardware projections are too aggressive; I'm going to tweak them and leave everything else in place" doesn't seem like the right approach.
I intend to produce fuller thoughts on this point in the coming months. In short: I agree that we shouldn't tweak the hardware projections and leave everything in place. On the other hand, it seems wrong to me to expect algorithmic efficiency to get faster as hardware progress slows. While it's true there will be more pressure to innovate, there will also be less hardware progress available to test innovations, which arguably is one of the main bottlenecks to software innovation.
I was referring to https://www.metaculus.com/questions/5121/when-will-the-first-artificial-general-intelligence-system-be-devised-tested-and-publicly-known-of-stronger-operationalization/ and https://www.metaculus.com/questions/3479/when-will-the-first-artificial-general-intelligence-system-be-devised-tested-and-publicly-known-of/ , which seem more germane than the link Barnett gives in the tweet above.
I am confused why you think my operationalization for timing transformative AI seems less relevant than a generic question about timing AGI (note that I am the author of one of the questions you linked).
My operationalization for transformative AI is the standard operationalization used in Open Philanthopy reports, such as Tom Davidson's report here, when he wrote,
This report evaluates the likelihood of ‘explosive growth’, meaning > 30% annual growth of gross world product (GWP), occurring by 2100.
Davidson himself refers to Ajeya Cotra, writing,
In her draft report [AF · GW], my colleague Ajeya Cotra uses TAI to mean ‘AI which drives Gross World Product (GWP) to grow at ~20-30% per year’ – roughly ten times faster than it is growing currently.
I agree with what you write here,
There are many ways transformative AI might not be reflected in economic growth figures, e.g. if economic growth figures don't include digital economies; if misaligned AI derails civilization; or if growth is deliberately held back, perhaps with AI help, in order to buy more time for improving things like AI alignment.
However, it's not clear to me that the questions you linked to lack drawbacks of equal or greater severity to these ones. To clarify, I merely said that "Metaculus has no consensus position on transformative AI" and I think that statement is borne out by the link I gave.
Actual disagreements between us
Now I get to the real disagreements we have/had.
I replied to your statement "Specific arguments for “later than 2100,” including outside-view arguments, seem reasonably close to nonexistent" by pointing to my own analysis [LW · GW], which produced three non-outside view arguments for longer timelines.
You defended your statement as follows,
I'm going to stand by my statement here - these look to be simply ceteris paribus reasons that AI development might take longer than otherwise. I'm not seeing a model or forecast integrating these with other considerations and concluding that our median expectation should be after 2100. (To be clear, I might still stand by my statement if such a model or forecast is added - my statement was meant as an abbreviated argument, and in that sort of context I think it's reasonable to say "reasonably close to nonexistent" when I mean something like "There aren't arguments of this form that have gotten a lot of attention/discussion/stress-testing and seem reasonably strong to me or, I claim, a reasonable disinterested evaluator.")
I have a few things to say here,
- "these look to be simply ceteris paribus reasons that AI development might take longer than otherwise" does not back up your actual claim, which was that specific arguments seem reasonably close to nonexistent. It's not clear to me how you're using "ceteris paribus" in that sentence, but ceteris paribus is not the same as non-specific which was what I responded to.
- I don't think I need to build an explicit probabilistic model in order to gesture at a point. It seems reasonably clear to me that someone could build a model using the arguments I gave, which would straightforwardly put more probability mass on dates past 2100 (even if the median is still <= 2100). But you're right that, since this model has yet to be built, it's uncertain how much of an effect these considerations will have on eventual AI timelines.
In response to my claim that "[Robin Hanson's] most recent public statements have indicated that he thinks AI is over a century away" you said,
I think the confusion here is whether ems count as transformative AI.
No, that's not the confusion, but I can see why you'd think that's the confusion. I made a mistake by linking the AI Impacts interview with Robin Hanson, which admittedly did not support my claim.
In fact, someone replied to the very tweet you criticize with the same objection as the one you gave. They said,
In his "when Robots rule the Earth" book he seems to think said robots will be there "sometime in the next century or so".
I replied,
Yes, he seems to have longer timelines now.
And Robin Hanson liked my tweet, which as far as I can tell, is a strong endorsement of my correctness in this debate.
Replies from: ege-erdil↑ comment by Ege Erdil (ege-erdil) · 2022-04-01T23:07:38.284Z · LW(p) · GW(p)
I just want to point out that I have actually offered a model [LW · GW] for why you might put the median date past 2100. If anything, it seems quite odd to me that people are so bullish on TAI before 2100; they seem to be doing a Bayesian update on the prior with a likelihood factor of ~ 6, while I think something like ~ 2 matches the strength of the evidence we have quite a bit better.
I think it's very easy to produce arguments based on extrapolating trends in variables such as "compute" or "model size/capacity", etc. In 1950 people did the same thing with energy consumption (remember the Kardashev scale?) and it failed quite badly. Any kind of inside view about AI timelines should, in my view, be interpreted as weaker evidence than it might at first glance appear to be.