Literature review of TAI timelines
post by Jsevillamol, keith_wynroe, David Atkinson · 2023-01-27T20:07:38.186Z · LW · GW · 7 commentsThis is a link post for https://epochai.org/blog/literature-review-of-transformative-artificial-intelligence-timelines
Contents
Highlights Introduction Caveats Results Read the rest of the review here None 7 comments
We summarize and compare several models and forecasts predicting when transformative AI will be developed.
Highlights
- The review includes quantitative models, including both outside and inside view, and judgment-based forecasts by (teams of) experts.
- While we do not necessarily endorse their conclusions, the inside-view model the Epoch team found most compelling is Ajeya Cotra’s “Forecasting TAI with biological anchors”, the best-rated outside-view model was Tom Davidson’s “Semi-informative priors over AI timelines”, and the best-rated judgment-based forecast was Samotsvety’s AGI Timelines Forecast [EA · GW].
- The inside-view models we reviewed predicted shorter timelines (e.g. bioanchors has a median of 2052) while the outside-view models predicted longer timelines (e.g. semi-informative priors has a median over 2100). The judgment-based forecasts are skewed towards agreement with the inside-view models, and are often more aggressive (e.g. Samotsvety assigned a median of 2043).
Introduction
Over the last few years, we have seen many attempts to quantitatively forecast the arrival of transformative and/or general Artificial Intelligence (TAI/AGI) using very different methodologies and assumptions. Keeping track of and assessing these models’ relative strengths can be daunting for a reader unfamiliar with the field. As such, the purpose of this review is to:
- Provide a relatively comprehensive source of influential timeline estimates, as well as brief overviews of the methodologies of various models, so readers can make an informed decision over which seem most compelling to them.
- Provide a concise summarization of each model/forecast distribution over arrival dates.
- Provide an aggregation of internal Epoch subjective weights over these models/forecasts. These weightings do not necessarily reflect team members’ “all-things-considered” timelines, rather they are aimed at providing a sense of our views on the relative trustworthiness of the models.
For aggregating internal weights, we split the timelines into “model-based” and “judgment-based” timelines. Model-based timelines are given by the output of an explicit model. In contrast, judgment-based timelines are either aggregates of group predictions on, e.g., prediction markets, or the timelines of some notable individuals. We decompose in this way as these two categories roughly correspond to “prior-forming” and “posterior-forming” predictions respectively.
In both cases, we elicit subjective probabilities from each Epoch team member reflective of:
- how likely they believe a model’s assumptions and methodology to be essentially accurate, and
- how likely it is that a given forecaster/aggregate of forecasters is well-calibrated on this problem,
respectively. Weights are normalized and linearly aggregated across the team to arrive at a summary probability. These numbers should not be interpreted too literally as exact credences, but rather a rough approximation of how the team views the “relative trustworthiness” of each model/forecast.
Caveats
- Not every model/report operationalizes AGI/TAI in the same way, and so aggregated timelines should be taken with an extra pinch of salt, given that they forecast slightly different things.
- Not every model and forecast included below yields explicit predictions for the snapshots (in terms of CDF by year and quantiles) which we summarize below. In these cases, we have done our best to interpolate based on explicit data-points given.
- We have included models and forecasts that were explained in more detail and lent themselves easily to a probabilistic summary. This means we do not cover less explained forecasts like Daniel Kokotajlo [LW · GW]’s and influential pieces of work without explicit forecasts such as David Roodman’s Modelling the Human Trajectory.
Results
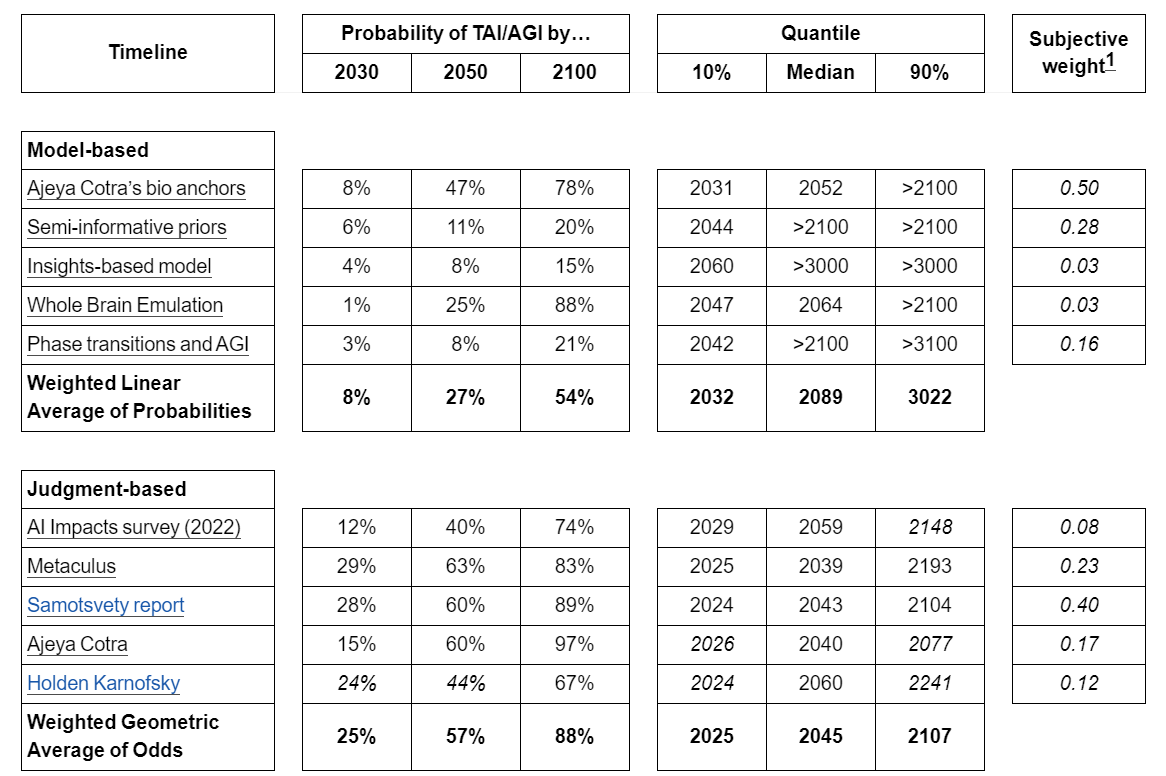
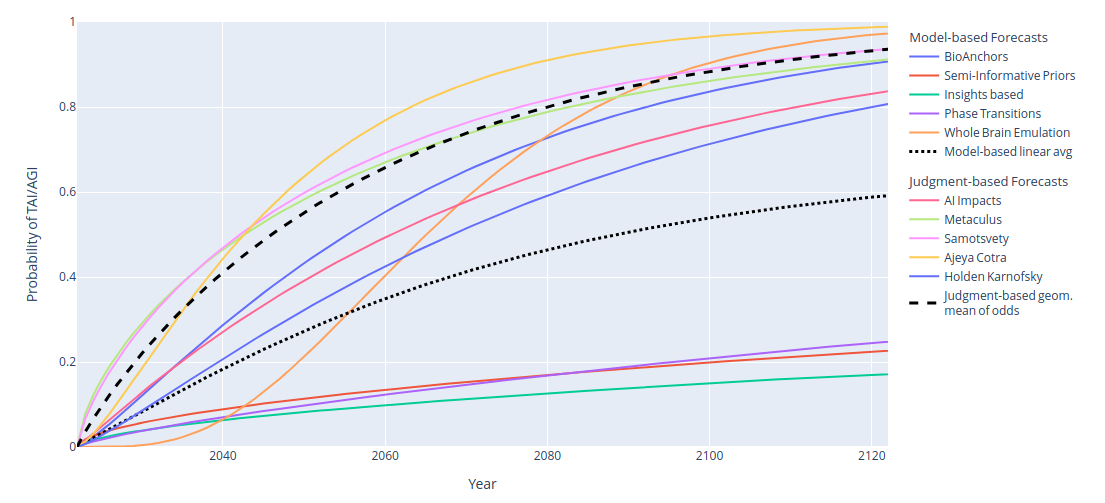
Read the rest of the review here
7 comments
Comments sorted by top scores.
comment by Lukas Finnveden (Lanrian) · 2023-01-29T06:39:15.975Z · LW(p) · GW(p)
The numbers you use from Holden says that he thinks AGI by 2036 is more than 10%. But when fitting the curves you put that at exactly 10%, which will predictably be an underestimate. It seems better to fit the curves without that number and just check that the result is higher than 10%.
Replies from: David Atkinson↑ comment by David Atkinson · 2023-01-31T02:35:12.480Z · LW(p) · GW(p)
Thanks very much for catching this. We've updated the extrapolation to only consider the two datapoints that are precisely specified. With so few points, the extrapolation isn't all that trustworthy, so we've also added some language to (hopefully) make that clear.
comment by Charbel-Raphaël (charbel-raphael-segerie) · 2023-12-29T21:50:55.799Z · LW(p) · GW(p)
Metaculus is now at 2031. I wonder how the folks at OpenPhil have updated since then.
comment by konstantin (konstantin@wolfgangpilz.de) · 2023-02-01T14:56:25.849Z · LW(p) · GW(p)
Great work, helped me to get clarity on which models I find useful and which ones I don't.
The tool on the page doesn't seem to work for me though, tried Chrome and Safari.
↑ comment by David Atkinson · 2023-02-01T15:43:29.232Z · LW(p) · GW(p)
Should be fixed now! Thanks for noticing this.
Replies from: konstantin@wolfgangpilz.de↑ comment by konstantin (konstantin@wolfgangpilz.de) · 2023-02-01T16:29:29.188Z · LW(p) · GW(p)
Cheers! Works for me
comment by konstantin (konstantin@wolfgangpilz.de) · 2023-02-01T16:36:41.852Z · LW(p) · GW(p)
To improve the review, an important addition would be to account for the degree to which different methods influence one another.
E.g. Holden and Ajeya influence one another heavily through conversations. And as Metaculus and Samotsvety, they already incorporate the other models, most notably the bioanchors framework. Maybe you are already correcting for this in the weighted average?
Also, note that e.g., Ajeya uses her own judgment to set the weights for the different models within the bioanchors framework.
Overall, I think right now there is a severe echo chamber effect within most of the forecasts that lets me weigh full outside views, such as the semi-informative priors much higher