Posts
Comments
I've been tempted to do this sometime, but I fear the prior is performing one very important role you are not making explicit: defining the universe of possible hypothesis you consider.
In turn, defining that universe of probabilities defines how bayesian updates look like. Here is a problem that arises when you ignore this: https://www.lesswrong.com/posts/R28ppqby8zftndDAM/a-bayesian-aggregation-paradox
shrug
I think this is true to an extent, but a more systematic analysis needs to back this up.
For instance, I recall quantization techniques working much better after a certain scale (though I can't seem to find the reference...). It also seems important to validate that techniques to increase performance apply at large scales. Finally, note that the frontier of scale is growing very fast, so even if these discoveries were done with relatively modest compute compared to the frontier, this is still a tremendous amount of compute!
even a pause which completely stops all new training runs beyond current size indefinitely would only ~double timelines at best, and probably less
I'd emphasize that we currently don't have a very clear sense of how algorithmic improvement happens, and it is likely mediated to some extent by large experiments, so I think is more likely to slow timelines more than this implies.
I agree! I'd be quite interested in looking at TAS data, for the reason you mentioned.
I think Tetlock and cia might have already done some related work?
Question decomposition is part of the superforecasting commandments, though I can't recall off the top of my head if they were RCT'd individually or just as a whole.
ETA: This is the relevant paper (h/t Misha Yagudin). It was not about the 10 commandments. Apparently those haven't been RCT'd at all?
We actually wrote a more up to date paper here
I cowrote a detailed response here
https://www.cser.ac.uk/news/response-superintelligence-contained/
Essentially, this type of reasoning proves too much, since it implies we cannot show any properties whatsoever of any program, which is clearly false.
Here is some data through Matthew Barnett and Jess Riedl
Number of cumulative miles driven by Cruise's autonomous cars is growing as an exponential at roughly 1 OOM per year.
That is to very basic approximation correct.
Davidson's takeoff model illustrates this point, where a "software singularity" happens for some parameter settings due to software not being restrained to the same degree by capital inputs.
I would point out however that our current understanding of how software progress happens is somewhat poor. Experimentation is definitely a big component of software progress, and it is often understated in LW.
More research on this soon!
algorithmic progress is currently outpacing compute growth by quite a bit
This is not right, at least in computer vision. They seem to be the same order of magnitude.
Physical compute has growth at 0.6 OOM/year and physical compute requirements have decreased at 0.1 to 1.0 OOM/year, see a summary here or a in depth investigation here
Another relevant quote
Algorithmic progress explains roughly 45% of performance improvements in image classification, and most of this occurs through improving compute-efficiency.
is not a transpose! It is the timestep . We are raising to the -th power.
Thanks!
Our current best guess is that this includes costs other than the amortized compute of the final training run.
If no extra information surfaces we will add a note clarifying this and/or adjust our estimate.
Thanks Neel!
The difference between tf16 and FP32 comes to a x15 factor IIRC. Though also ML developers seem to prioritise other characteristics than cost effectiveness when choosing GPUs like raw performance and interconnect, so you can't just multiply the top price performance we showcase by this factor and expect that to match the cost performance of the largest ML runs today.
More soon-ish.
Because there is more data available for FP32, so it's easier to study trends there.
We should release a piece soon about how the picture changes when you account for different number formats, plus considering that most runs happen with hardware that is not the most cost-efficient.
Note that Richard is not treating knightian uncertainty as special and unquantifiable, but instead is giving examples of how to treat it like any other uncertainty, that he is explicitly quantifying and incorporating in his predictions.
I'd prefer calling Richard's "model error" to separate the two, but I'm also okay appropriating the term as Richard did to point to something coherent.
To my knowledge, we currently don’t have a way of translating statements about “loss” into statements about “real-world capabilities”.
My intuition is that it's not a great approximation in those cases, similar to how in regular Laplace the empirical approximation is not great when you have eg N<5
Id need to run some calculations to confirm that intuition though.
Here is a 2012 meme about SolidGoldMagikarp
This site claims that the strong SolidGoldMagikarp was the username of a moderator involved somehow with Twitch Plays Pokémon
I still don't understand - did you mean "when T/t is close to zero"?
What's r?
That's exactly right, and I think the approximation holds as long as T/t>>1.
This is quite intuitive - as the amount of data goes to infinity, the rate of events should equal the number of events so far divided by the time passed.
If you want to join the Spanish-speaking EA community, you can do so through this link!
I agree with the sentiment that indiscriminate regulation is unlikely to have good effects.
I think the step that is missing is analysing the specific policies No-AI Art Activist are likely to advocate for, and whether it is a good idea to support it.
My current sense is that data helpful for alignment is unlikely to be public right now, and so harder copyright would not impede alignment efforts. The kind of data that I could see being useful are things like scores and direct feedback. Maybe at most things like Amazon reviews could end up being useful for toy settings.
Another aspect that the article does not touch on is that copyright enforcement could have an adverse effect. Currently there is basically no one trying to commercialize training dataset curation because enforcing copyright use is a nightmare. It is in fact a common good. I'd expect there would be more incentives to create large curated datasets if this was not the case.
Lastly, here are some examples of "no AI art" legislation I expect the movement is likely to support:
- Removing copyright protection of AI generated images
- Enforcing AI training data to be strictly opt-in
- Forcing AI content to be labelled as such
Besides regulation, I also expect activists to 4) pressure companies to deboost AI made content in social medial sites.
My general impression is that 3) is slightly good for AI safety. People in the AI Safety community have advocated for it in the past, convincingly.
I'm more agnostic on 1), 2) and 4).
1 and 4 will make AI generation less profitable, but also it's somewhat confused - it's a weird double standard to apply to AI content over human made content.
2 makes training more annoying, but could lead to commercialization of datasets and more collective effort being put into building them. I also think there is a possibly a coherent moral case for it, which I'm still trying to make my mind about, regardless of the AI safety consequences.
All in all, I am confused, though I wholeheartedly agree that we should be analysing and deciding to support specific policies rather than eg the anti AI art movement as a whole.
Great work!
Stuart Armstrong gave one more example of a heuristic argument based in the presumption of independence here.
Here are my quick takes from skimming the post.
In short, the arguments I think are best are A1, B4, C3, C4, C5, C8, C9 and D. I don't find any of them devastating.
A1. Different calls to ‘goal-directedness’ don’t necessarily mean the same concept
I am not sure I parse this one.I am reading it as "AI systems might be more like imitators than optimizers" from the example, which I find moderately persuasive
A2. Ambiguously strong forces for goal-directedness need to meet an ambiguously high bar to cause a risk
I am not sure I understand this one either.I am reading it as "there might be no incentive for generality" which I dont find persuasive - I think there is a strong incentive
B1. Small differences in utility functions may not be catastrophic
I dont find this persuasive. I think the evidence from optimization theory setting variables to extreme values is suggestive enough to suggest this is not the default
B2. Differences between AI and human values may be small
B3. Maybe value isn’t fragile
The only example we have of general intelligence (humans) seems to have strayed pretty far from evolutionary incentives, so I find this unpersuasive
B4. [AI might only care about]Short-term goals
I find that somewhat persuasive, or at least not obviously wrong, similar to A1. There is a huge incentive for instilling long term thinking though.
C1. Human success isn’t from individual intelligence
I dont find this persuasive. Im not convinced there is a meaningful difference between "a single AGI" and "a society of AGIs". A single AGI could be running a billion independent threads of thought and outspeed humans.
C2. AI agents may not be radically superior to combinations of humans and non-agentic machines
I dont find this persuasive. Seems unlikely that human-in-the-loop is going to have any advantages over pure machines.
C3. Trust
I find this plausible but not convincing
C4. Headroom
Plausible but not convincing. I dont find any of the particular examples of lack of headroom convincing, and I think the prior should be that there is a lot of headroom
C5. Intelligence may not be an overwhelming advantage
I find this moderately persuasive though not entirely convincing
C6. Unclear that many goals realistically incentivise taking over the universe
I find this unconvincing. I think there are many reasons to expect that taking over the universe is a convergent goal.
C7. Quantity of new cognitive labor is an empirical question, not addressed
I dont find this superpersuasive. In particular I think there is a good chance that once we have AGI we will be in a hardware overhang and be able to execute tons of AGI-equivalents
C8. Speed of intelligence growth is ambiguous
I find this plausible
C9. Key concepts are vague
Granted but not a refutation in itself
D1. The argument overall proves too much about corporations
I find this somewhat persuasive
Eight examples, no cherry-picking:
Nit: Having a wall of images makes this post unnecessarily harder to read.
I'd recommend making a 4x2 collage with the photos so they don't take that much space.
As it is often the case, I just found out that Jaynes was already discussing a similar issue to the paradox here in his seminal book.
I also found this thread of math topics on AI safety helpful.
Ah sorry for the lack of clarity - let's stick to my original submission for PVE
That would be:
[0,1,0,1,0,0,9,0,0,1,0,0]
Yes, I am looking at decks that appear in the dataset, and more particularly at decks that have faced a deck similar to the rival's.
Good to know that one gets similar results using the different scoring functions.
I guess that maybe the approach does not work that well ¯\_(ツ)_/¯
Thank you for bringing this up!
I think you might be right, since the deck is quite undiverse and according to the rest diversity is important. That being said, I could not find the mistake in the code at a glance :/
Do you have any opinions on [1, 1, 0, 1, 0, 1, 2, 1, 1, 3, 0, 1]? This would be the worst deck amongst the decks that played against a deck similar to the rival's in my code, according to my code.
Marius Hobbhahn has estimated the number of parameters here. His final estimate is 3.5e6 parameters.
Anson Ho has estimated the training compute (his reasoning at the end of this answer). His final estimate is 7.8e22 FLOPs.
Below I made a visualization of the parameters vs training compute of n=108 important ML system, so you can see how DeepMind's syste (labelled GOAT in the graph) compares to other systems.
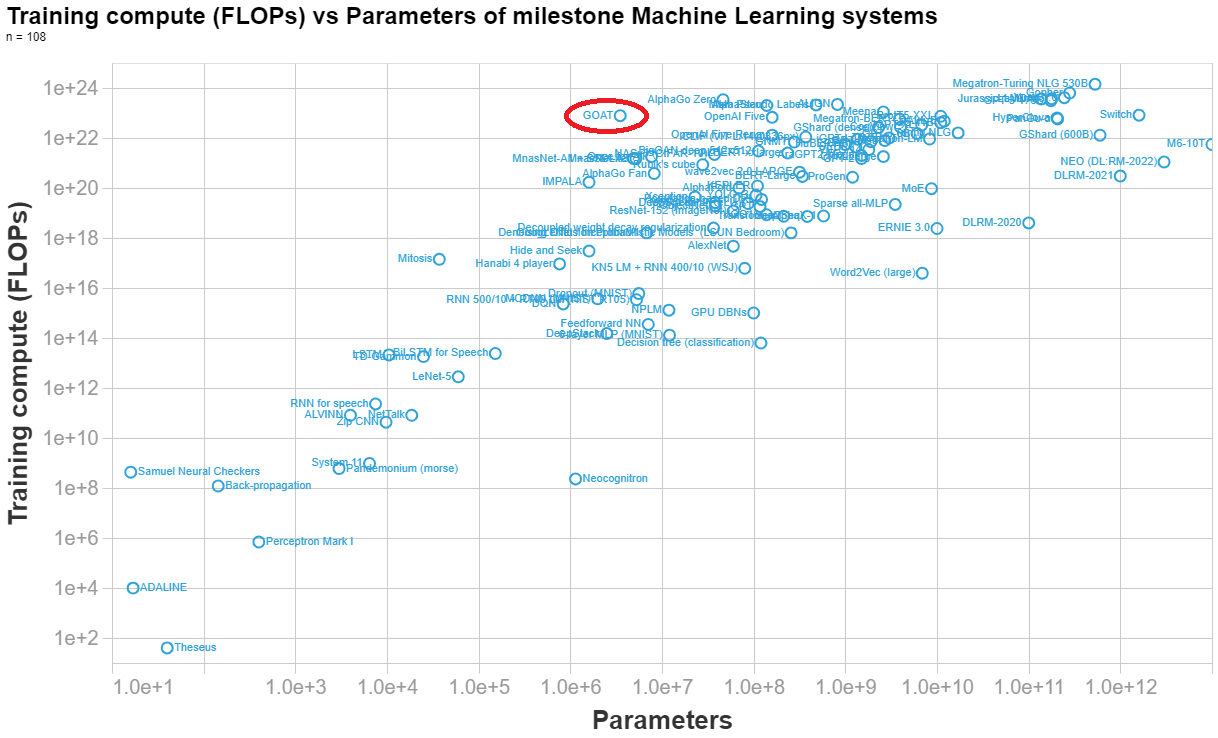
[Final calculation]
(8 TPUs)(4.20e14 FLOP/s)(0.1 utilisation rate)(32 agents)(7.3e6 s/agent) = 7.8e22 FLOPs==========================
NOTES BELOW[Hardware]
- "Each agent is trained using 8 TPUv3s and consumes approximately 50,000 agent steps (observations) per second."
- TPUv3 (half precision): 4.2e14 FLOP/s
- Number of TPUs: 8
- Utilisation rate: 0.1[Timesteps]
- Figure 16 shows steps per generation and agent. In total there are 1.5e10 + 4.0e10 + 2.5e10 + 1.1e11 + 2e11 = 3.9e11 steps per agent.
- 3.9e11 / 5e4 = 8e6 s → ~93 days
- 100 million steps is equivalent to 30 minutes of wall-clock time in our setup. (pg 29, fig 27)
- 1e8 steps → 0.5h
- 3.9e11 steps → 1950h → 7.0e6 s → ~82 days
- Both of these seem like overestimates, because:
“Finally, on the largest timescale (days), generational training iteratively improves population performance by bootstrapping off previous generations, whilst also iteratively updating the validation normalised percentile metric itself.” (pg 16)
- Suggests that the above is an overestimate of the number of days needed, else they would have said (months) or (weeks)?
- Final choice (guesstimate): 85 days = 7.3e6 s[Population size]
- 8 agents? (pg 21) → this is describing the case where they’re not using PBT, so ignore this number
- The original PBT paper uses 32 agents for one task https://arxiv.org/pdf/1711.09846.pdf (in general it uses between 10 and 80)
- (Guesstimate) Average population size: 32
Fixed, thanks!
Here is my very bad approach after spending ~one hour playing around with the data
- Filter decks that fought against a similar to the rivals deck, using a simple measure of distance (sum of absolute differences between the deck components)
- Compute a 'score' of the decks. The score is defined as the sum of 1/deck_distance(deck) * (1 or -1 depending on whether the deck won or lost against the challenger)
- Report the deck with the maximum score
So my submission would be: [0,1,0,1,0,0,9,0,0,1,0,0]
Seems like you want to include A, L, P, V, E in your decks, and avoid B, S, K. Here is the correlation between the quantity of each card and whether the deck won. The ordering is ~similar when computing the inclusion winrate for each card.
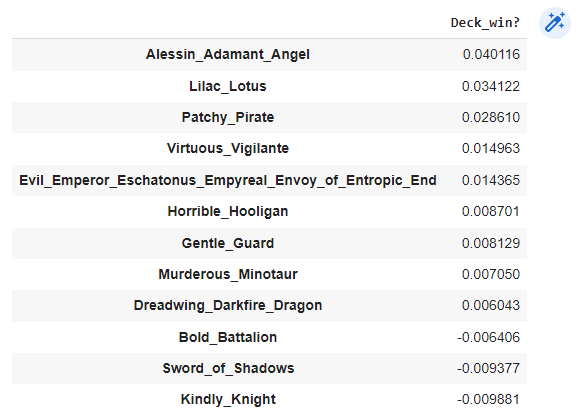
Thanks for the comment!
I am personally sympathetic to the view that AlphaGo Master and AlphaGo Zero are off-trend.
In the regression with all models the inclusion does not change the median slope, but drastically increases noise, as you can see for yourself in the visualization selecting the option 'big_alphago_action = remove' (see table below for a comparison of regressing the large model trend without vs with the big AlphaGo models).

In appendix B we study the effects of removing AlphaGo Zero and AlphaGo Master when studying record-setting models. The upper bound of the slope is affected dramatically, and the R2 fit is much better when we exclude them, see table 6 reproduced below.
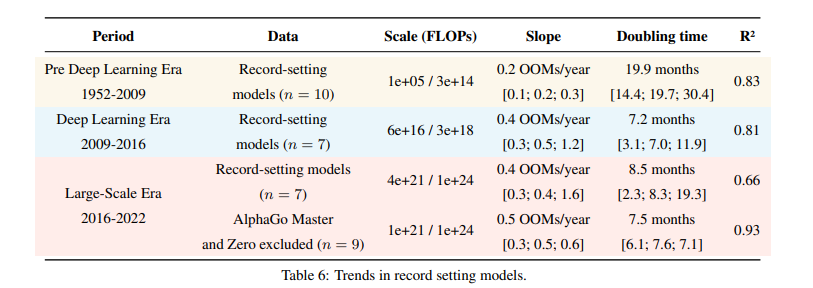
Following up on this: we have updated appendix F of our paper with an analysis of different choices of the threshold that separates large-scale and regular-scale systems. Results are similar independently of the threshold choice.
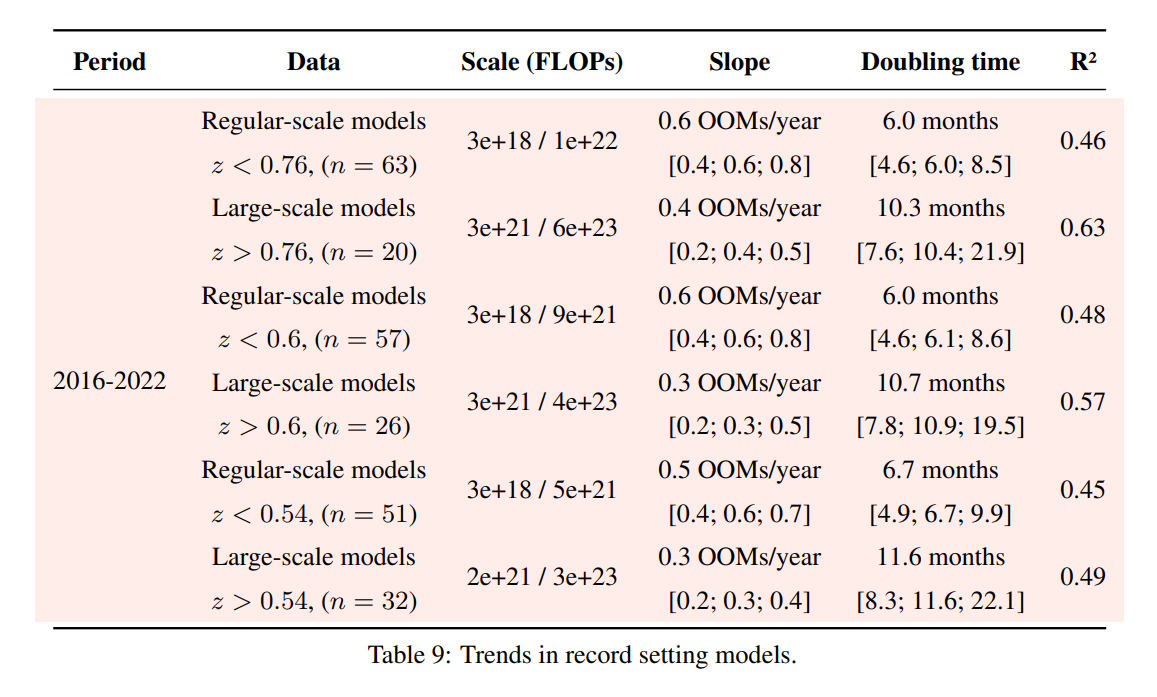
Thanks for engaging!
To use this theorem, you need both an (your data / evidence), and a (your parameter).
Parameters are abstractions we use to simplify modelling. What we actually care about is the probability of unkown events given past observations.
You start out discussing what appears to be a combination of two forecasts
To clarify: this is not what I wanted to discuss. The expert is reporting how you should update your priors given the evidence, and remaining agnostic on what the priors should be.
A likelihood isn't just something you multiply with your prior, it is a conditional pmf or pdf with a different outcome than your prior.
The whole point of Bayesianism is that it offer a precise, quantitative answer to how you should update your priors given some evidence - and that is multiplying by the likelihoods.
This is why it is often recommend in social sciences and elsewhere to report your likelihoods.
I'm not sure we ever observe [the evidence vector] directly
I agree this is not common in judgemental forecasting, where the whole updating process is very illegible. I think it holds for most Bayesian-leaning scientific reporting.
it is pretty clear from your post that you're talking about in the sense used above, not .
I am not, I am talking about evidence = likelihood vectors.
One way to think about this is that the expert is just informing us about how we should update our beliefs. "Given that the pandemic broke out in Wuhan, your subjective probability of a lab break should increase and it should increase by this amount". But the final probability depends on your prior beliefs, that the expert cannot possibly know.
I don't think there is a unique way to go from to, let's say, , where is the expert's probability vector over and your probability vector over .
Yes! If I am understanding this right, I think this gets to the crux of the post. The compression is lossy, and neccessarily loses some information.
Great sequence - it is a nice compendium of the theories and important thought experiments.
I will probably use this as a reference in the future, and refer other people here for an introduction.
Looking forward to future entries!
I am glad Yair! Thanks for giving it a go :)
Those I know who train large models seem to be very confident we will get 100 Trillion parameter models before the end of the decade, but do not seem to think it will happen, say, in the next 2 years.
FWIW if the current trend continues we will first see 1e14 parameter models in 2 to 4 years from now.
I am pretty pumped about this. Google docs + latex support is huge game for me.
There's also a lot of research that didn't make your analysis, including work explicitly geared towards smaller models. What exclusion criteria did you use? I feel like if I was to perform the same analysis with a slightly different sample of papers I could come to wildly divergent conclusions.
It is not feasible to do an exhaustive analysis of all milestone models. We necessarily are missing some important ones, either because we are not aware of them, because they did not provide enough information to deduce the training compute or because we haven't gotten to annotate them yet.
Our criteria for inclusion is outlined in appendix A. Essentially it boils down to ML models that have been cited >1000 times, models that have some historical significance and models that have been deployed in an important context (eg something that was deployed as part of Bing search engine would count). For models in the last two years we were more subjective, since there hasn't been enough time for the more relevant work to stand out the test of time.
We also excluded 5 models that have abnormally low compute, see figure 4.
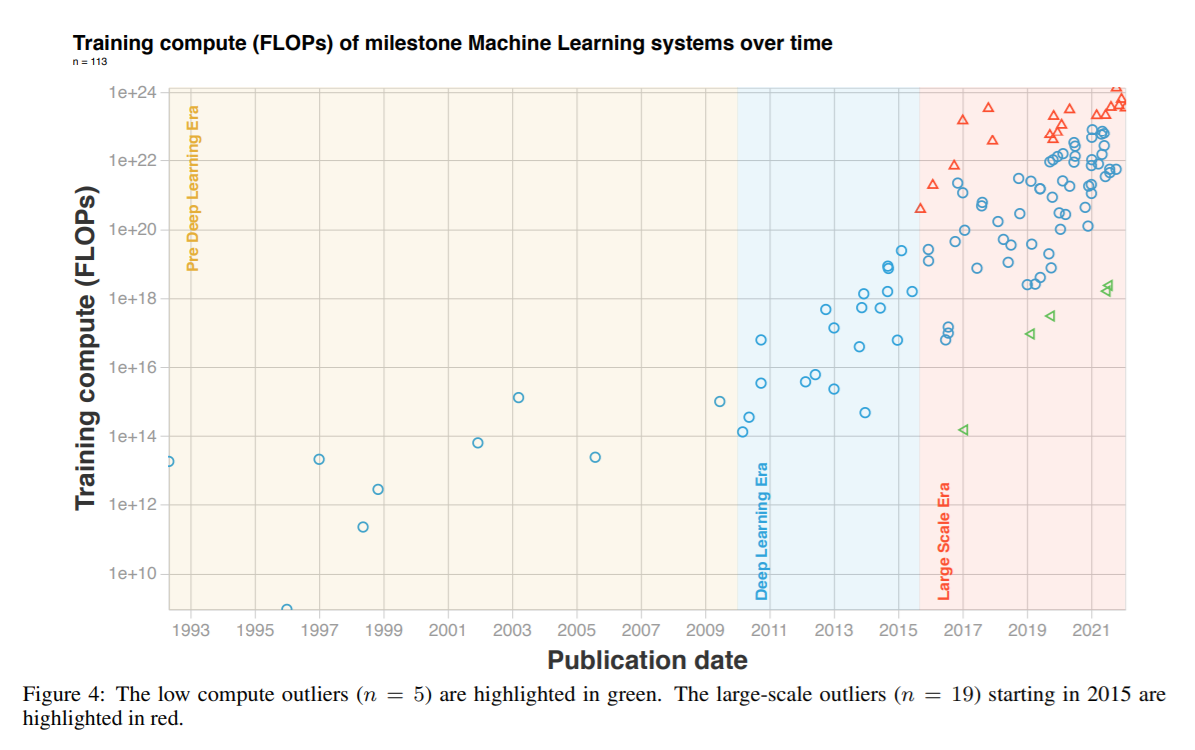
We tried playing around with the selection of papers that was excluded and it didn't significantly change our conclusions, though obviously the dataset is biased in many ways. Appendix G discusses the possible biases that may have crept in.
Great questions! I think it is reasonable to be suspicious of the large-scale distinction.
I do stand by it - I think the companies discontinuously increased their training budgets around 2016 for some flagship models.[1] If you mix these models with the regular trend, you might believe that the trend was doubling very fast up until 2017 and then slowed down. It is not an entirely unreasonable interpretation, but it explains worse the discontinuous jumps around 2016. Appendix E discusses this in-depth.
The way we selected the large-scale models is half intuition and half convenience. We compare the compute of each model to the log compute of nearby papers (within 2 years), and we call it large scale if its log compute exceeds 0.72 standard deviations of the mean of that sample.
I think there is a reasonable case for including NASv3, Libratus, Megatron-LM, T5-3B, OpenAI Five, Turing NLG, iGPT-XL, GShard (dense), Switch, DALL-E, Pangu-α, ProtT5-XXL and HyperClova on either side of this division.
Arguably we should have been more transparent about the effects of choosing a different threshold - we will try to look more into this in the next update of the paper.
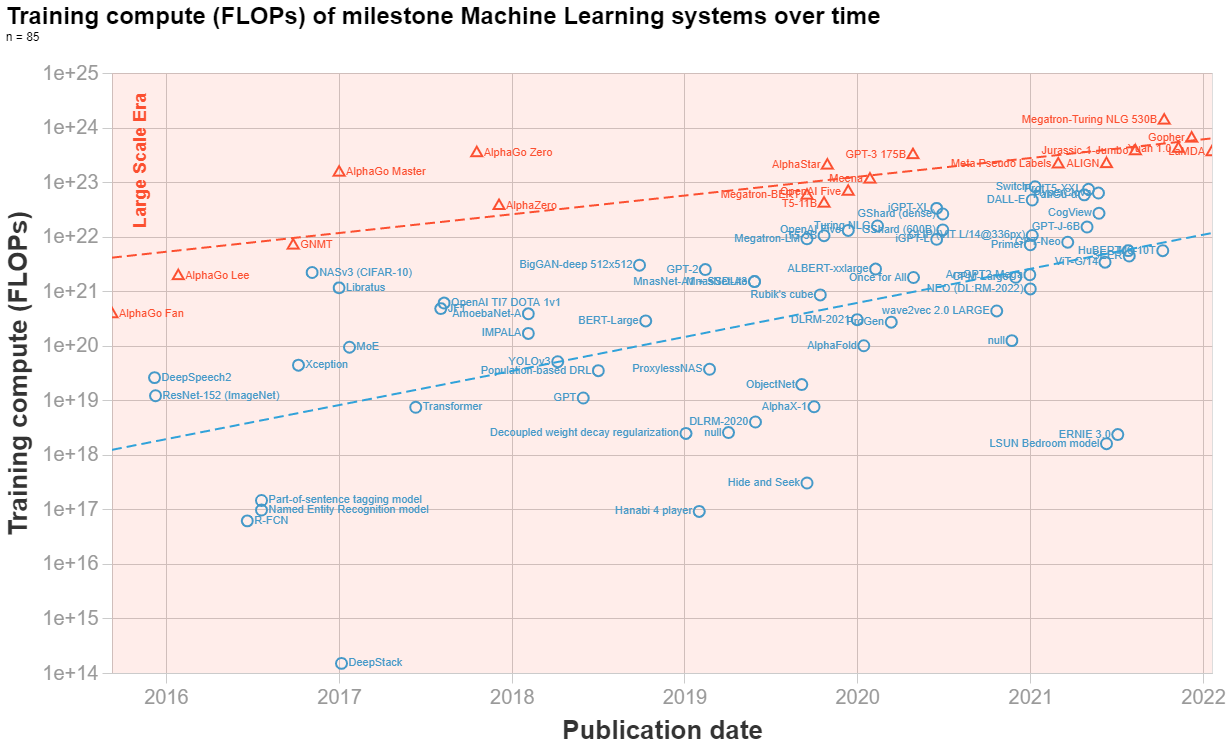
- ^
See appendix F for a surface discussion
Great idea!
You can modify your utility function as part of a bargaining or pre commitment strategy.
Thanks, fixed!
I'd also recommend putting everything after "Should you read this book? Possibly!" at the top, instead of at the bottom, since it's still relevant to the majority of people who don't read the whole post.
That's a good point, I'll try later to reorder it and write a new outro.
I feel like the approach of "investigate past trends" has passed the 80/20 point of informing our AI timelines, and I'd probably prefer those researchers to orient to new questions that illuminate different facets of the AI strategic landscape.
I specialise in researching this topic. My impression is that barely anyone has looked at past technological trends, neither in academia nor in the LW/EA community. I am generally quite excited about more people looking into this space, because it seems neglected and the kind of topic where EA/LW type of people have a significant edge.
A negative rapid test should be necessary before ending isolation. The CDC’s new guidelines don’t say this but this seems overdetermined and obvious to me. If you care about not being infectious, you should check on that before exposing others.
How common are false positives after infection?
Recovered patients: Patients who have recovered from COVID-19 can continue to have detectable SARS-CoV-2 RNA in upper respiratory specimens for up to 3 months after illness onset. However, replication-competent virus has not been reliably recovered from such patients, and they are not likely infectious.
Which suggests they might be not that uncommon.
UTSouthwesterner Medical Center says that (the CDC says something similar):
How can a patient have a positive PCR test but not be infectious?
Reverse transcriptase-quantitative polymerase chain reaction (RT-qPCR) is the primary method used to diagnose SARS-CoV-2 infection and many other viruses. The test works by creating copies of viral genetic material in respiratory samples over many cycles. A single copy of viral RNA can be amplified to more than 2 trillion copies during a typical 40-cycle testing protocol. Amplification of genomic sequence is measured in cycle thresholds (Ct) and the Ct values correlate with whether a sample contains infectious virus particles. If the viral RNA is detected after just a few amplification cycles (i.e. at a low Ct value), that means high RNA levels are present and thus more viable virus; however, because this test is designed to be a sensitive diagnostic tool (not for monitoring disease progression), a very high Ct value (low RNA level) is set as a positive range cutoff. This means that very low levels of the RNA or viral genomic fragments can test “positive” for months after the acute infection but are not associated with live virus. This detection issue is common for other RNA viruses.
Which suggests to me that PCR testing might be prone to false positive after an infection, but does not clarify to me if Rapid Antigen Tests are also prone to false positives.
My guess is that false positives in the Rapid Antigen Test are uncommon, since the proposed causal mechanism for why PCRs give false positives is because of the viral load amplification, which does not happen with Rapid Antigen Tests.
The key question: should I end isolation after 10 days regardless of rapid antigen test result?