Posts
Comments
I like pointing out this confusion. Here's a grab-bag of some of the things I use it for, to try to pull them apart:
- actors/institutions far away from the compute frontier produce breakthroughs in AI/AGI tech (juxtaposing "only the top 100 labs" vs "a couple hackers in a garage")
- once a sufficient AI/AGI capability is reached, that it will be quickly optimized to use much less compute
- amount of "optimization pressure" (in terms of research effort) pursuing AI/AGI tech, and the likelihood that they missed low-hanging fruit
- how far AI/AGI research/products are away from the highest-value-marginal-use of compute, and how changes making AI/AGI the biggest marginal profit of compute would change things
- the legibility of AI/AGI research progress (e.g. in a high-overhang world, small/illegible labs can make lots of progress)
- the likelihood of compute-control interventions to change the trajectory of AI/AGI research
- the asymmetry between compute-to-build (~=training) and compute-to-run (~=inference) of advanced AI/AGI technology
probably also others im forgetting
Comparing AI Safety-Capabilities Dilemmas to Jervis' Cooperation Under the Security Dilemma
I've been skimming some things about the Security Dilemma (specifically Offense-Defense Theory) while looking for analogies for strategic dilemmas in the AI landscape.
I want to describe a simple comparison here, lightly held (and only lightly studied)
- "AI Capabilities" -- roughly, the ability to use AI systems to take (strategically) powerful actions -- as "Offense"
- "AI Safety" -- roughly, that AI systems under control and use do not present a catastrophic/existential threat -- as "Defense"
Now re-creating the two values (each) of the two variables of Offense-Defense Theory:
- "Capabilities Advantaged" -- Organizations who disproportionately invest in capabilities get strategic advantage
- "Safety Advantaged" -- Organizations who disproportionately invest in safety get strategic advantage.[1]
- "Capabilities Posture Not Distinguishable from Safety Posture" -- from the outside, you can't credibly determine how an organization is investing Capabilities-vs-Safety. (This is the case if very similar research methods/experiments are used to study both, or that they are not technologically separable. My whole interest in this is in cultivating and clarifying this one point. This exists in the security dilemma as well inasmuch as defensive missile interceptors can be used to offensively strike ranged targets, etc.)
- "Capabilities Posture Distinguishable from Safety Posture" -- it's credibly visible from the outside whether an organization is disproportionately invested in Safety
Finally, we can sketch out the Four Worlds of Offense-Defense Theory
- Capabilities Advantaged / Not-Distinguishable : Doubly dangerous (Jervis' words, but I strongly agree here). I also think this is the world we are in.
- Safety Advantaged / Not-Distinguishable : There exists a strategic dilemma, but it's possible that non-conflict equilibrium can be reached.
- Capabilities Advantaged / Distinguishable : No security dilemma, and other strategic responses can be made to obviously-capabilities-pursuing organizations. We can have warnings here which trigger or allow negotiations or other forms of strategic resolution.
- Safety Advantaged / Distinguishable : Doubly stable (Jervis' words, also strongly agree).
From the paper linked in the title:
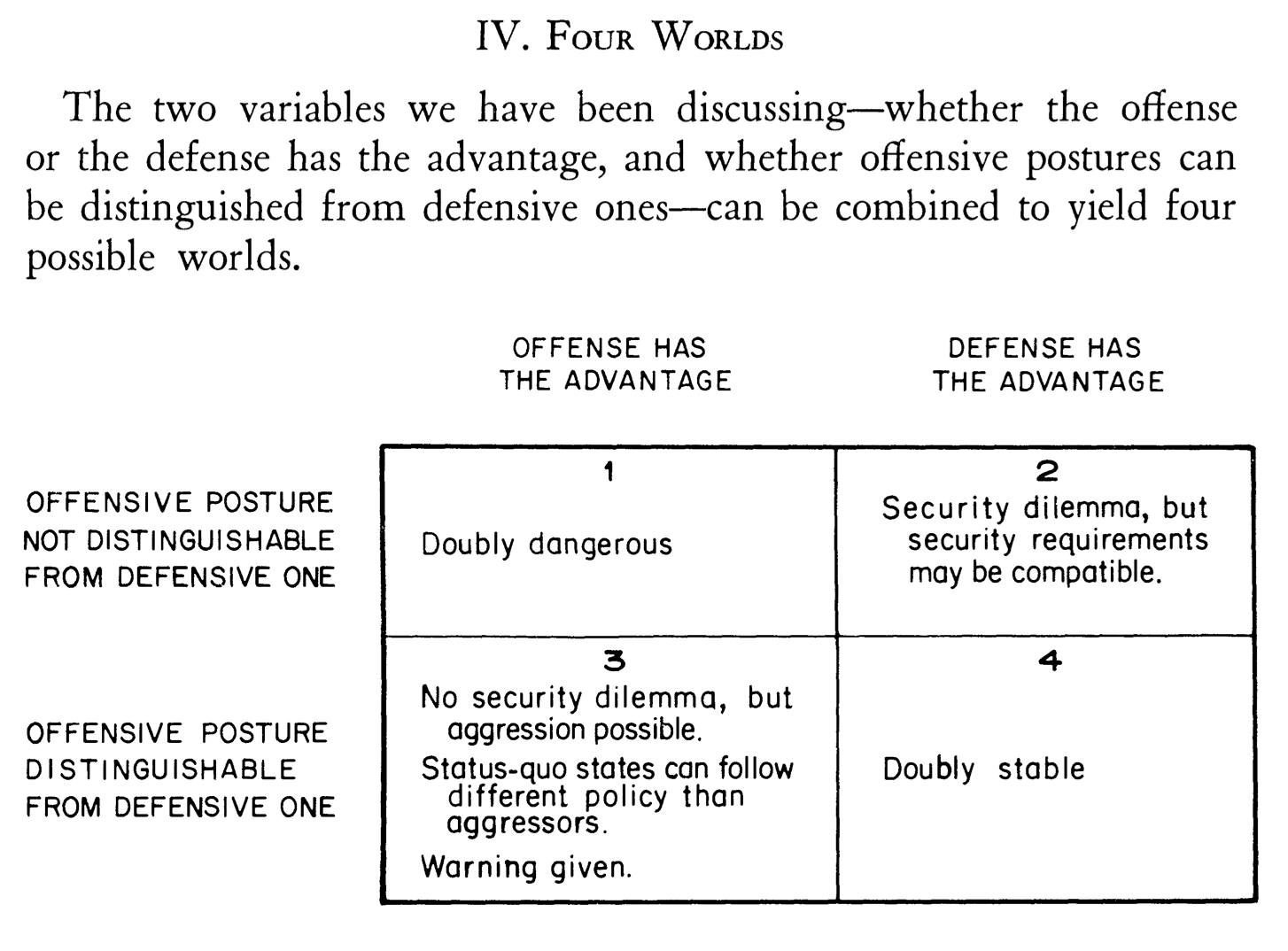
My Overall Take
- If this analogy fits, I think we're in World 1.
- The analogy feels weak here, there's a bunch of mis-fits from the original (very deep) theory
- I think this is neat and interesting but not really a valuable strategic insight
- I hope this is food for thought
- ^
This one is weird, and hard for me to make convincing stories about the real version of this, as opposed to seeming to have a Safety posture -- things like "recruiting", "public support", "partnerships", etc all can come from merely seeming to adopt the Safety Posture. (Though, this is actually a feature of the security dilemma and offense-defense theory, too)
I largely agree with the above, but commenting with my own version.
What I think companies with AI services should do:
Can be done in under a week:
- Have a monitored communication channel for people, primarily security researchers, to responsibly disclose potential issues ("Potential Flaw")
- Creating an email (ml-disclosures@) which forwards to an appropriate team
- Submissions are promptly responded to with a positive receipt ("Vendor Receipt")
- Have clear guidance (even just a blog post or similar) about what constitutes an issue worth reporting ("Vulnerability")
- Even just a simple paragraph giving a high level overview could be evolved with time
- Have a internal procedure/playbook for triaging and responding to potential issues. Here's some options I think you could have, with heuristics to pick:
- Non-Vulnerability: reply that the reported behavior is safe to publicly disclose
- Investigation: reply that more investigation is needed, and give a timeline for an updated response
- Vulnerability: reply that the reported behavior is a vulnerability, and give a timeline for resolution and release of a fix, as well as updates as those timelines change
Longer term
- Have a public bounty program to incentivize responsible disclosure
- There even exist products to help companies deploy these sorts of programs
- Coordinate with other organizations with similar systems
- Confidential communication channels to share potentially critical severity vulnerabilities.
- Possibly eventually a central coordinating organization (analogous to MITRE) that de-duplicates work handling broad vulnerabilities -- I think this will be more important when there are many more service providers than today.
- Coordinate as a field to develop shared understanding of what does and does not constitute a vulnerability. As these systems are still nascent, lots of work needs to be done to define this, and this work is better done with a broad set of perspectives and inputs.
- Cultivate positive relationships with responsible researchers and responsible research behaviors
- Don't stifle this kind of research by just outright banning it -- which is how you get the only researchers breaking your system are black hats
- Create programs and procedures specifically to encourage and enable this kind of research in ways that are mutually beneficial
- Reward and publicly credit researchers that do good work
References:
- Responsible Vulnerability Disclosure Process (draft IETF report)
- Guidelines for Security Vulnerability Reporting and Response (Organization for Internet Safety)
- Probably better more updated ones but I've been out of this field for a long time
Weakly positive on this one overall. I like Coase's theory of the firm, and like making analogies with it to other things. I don't think this application felt like it quite worked to me, and trying to write up why.
One thing is I think feels off is an incomplete understanding of the Coase paper. What I think the article gets correct: Coase looks at the difference between markets (economists preferred efficient mechanism) and firms / corporation, and observes that transaction costs (for people these would be contracts, but in general all transaction costs are included) are avoided in firms. What I think it misses: A primary question explored in the paper is what factors govern the size of firms, and this leads to a mechanistic model that the transaction costs internal to the firm increase with the size of the firm until they reach a limit of the same as transaction costs for the open market (and thus the expected maximum efficient size of a non-monopoly firm). A second, smaller, missed point I think is that the price mechanism works for transactions outside the firm, but does not for transactions inside the firm.
Given these, I think the metaphor presented here seems incomplete. It's drawing connections to some of the parts of the paper, but not all of the central parts, and not enough to connect to the central question of size.
I'm confused exactly what parts of the metaphor map to the paper's concept of market and firm. Is monogamy the market, since it doesn't require high-order coordination? Is polyamory the market since everyone can be a free-ish actor in an unbundled way? Is monogamy the firm since it's not using price-like mechanisms to negotiate individual unbundled goods? Is polyamory the firm since its subject to the transaction cost scaling limit of size?
I do think that it seems to use the 'transaction costs matter' pretty solidly from the paper, so there is that bit.
I don't really have much I can say about the polyamory bits outside of the economics bits.
This post was personally meaningful to me, and I'll try to cover that in my review while still analyzing it in the context of lesswrong articles.
I don't have much to add about the 'history of rationality' or the description of interactions of specific people.
Most of my value from this post wasn't directly from the content, but how the content connected to things outside of rationality and lesswrong. So, basically, i loved the citations.
Lesswrong is very dense in self-links and self-citations, and to a lesser degree does still have a good number of links to other websites.
However it has a dearth of connections to things that aren't blog posts -- books, essays from before the internet, etc. Especially older writings.
I found this posts citation section to be a treasure trove of things I might not have found otherwise.
I have picked up and skimmed/started at least a dozen of the books on the list.
I still come back to this list sometimes when I'm looking for older books to read.
I really want more things like this on lesswrong.
I read this sequence and then went through the whole thing. Without this sequence I'd probably still be procrastinating / putting it off. I think everything else I could write in review is less important than how directly this impacted me.
Still, a review: (of the whole sequence, not just this post)
First off, it signposts well what it is and who it's for. I really appreciate when posts do that, and this clearly gives the top level focus and whats in/out.
This sequence is "How to do a thing" - a pretty big thing, with a lot of steps and branches, but a single thing with a clear goal.
The post is addressing a real need in the community (and it was a personal need for me as well) -- which I think are the best kinds of "how to do a thing" posts.
It was detailed and informative while still keeping the individual points brief and organized.
It specifically calls out decision points and options, how much they matter, what the choices are, and information relevant to choosing. This is a huge energy-saver in terms of actually getting people to do this process.
When I went through it, it was accurate, and I ran into the decision points and choices as expected.
Extra appreciation for the first post which also includes a concrete call to action for a smaller/achievable-right-now thing for people to do (sign a declaration of intent to be cryopreserved). Which I did! I also think that a "thing you can do right now" is a great feature to have in "how to do a thing" posts.
I'm in the USA, so I don't have much evaluation or feedback on how valuable this is to non-USA folks. I really do appreciate that a bunch of extra information was added for non-USA cases, and it's organized such that it's easy to read/skim past if not needed.
I know that this caused me personally to sign up for cryonics, and I hope others as well. Inasmuch as the authors goal was for more people in our community to sign up for cryonics -- I think that's a great goal and I think they succeeded.
Summary
- public discourse of politics is too focused on meta and not enough focused on object level
- the downsides are primarily in insufficient exploration of possibility space
Definitions
- "politics" is topics related to government, especially candidates for elected positions, and policy proposals
- opposite of meta is object level - specific policies, or specific impacts of specific actions, etc
- "meta" is focused on intangibles that are an abstraction away from some object-level feature, X, e.g. someones beliefs about X, or incentives around X, or media coverage vibes about X
- Currently public discourse of politics is too much meta and not enough object level
Key ideas
- self-censorship based on others' predicted models of self-censorship stifles thought
- worrying about meta-issues around a policy proposal can stifle the ability to analyze the object-level implications
- public discourse seems to be a lot of confabulating supporting ideas for the pre-concluded position
- downsides of too much meta are like distraction in terms of attention and cognition
- author has changed political beliefs based on repeated object-level examples why their beliefs were wrong
Review Summary
- overall i agree with the author's ideas and vibe
- the piece feels like its expressing frustration / exasperation
- i think it's incomplete, or a half-step, instead of what i'd consider a full article
- I give examples of things that would make it feel full-step to me
Review
Overall I think I agree with the observations and concepts presented, as well as the frustration/exasperation sense at the way it seems we're collectively doing it wrong.
However I think this piece feels incomplete to me in a number of ways, and I'll try to point it out by giving examples of things that would make it feel complete to me.
One thing that would make it feel complete to me is a better organized set of definitions/taxonomy around the key ideas. I think 'politics' can be split into object level things around politicians vs policies. I think even the 'object level' can be split into things like actions (vote for person X or not) vs modeling (what is predicted impact on Y). When I try to do this kind of detail-generation, I think I find that my desire for object-level is actually a desire for specific kinds of object level focus (and not object-level in the generic).
Another way of making things more precise is to try to make some kind of measure or metric out of the meta<->object dimension. Questions like 'how would it be measured' or even 'what are the units of measurement' would be great for building intuitions and models around this. Relatedly - describing what 'critical meta-ness' or the correct balance point would also be useful here. Assuming we had a way to decrease-meta/increase-object, how would we know when to stop? I think these are the sorts of things that would make a more complete meta-object theory.
A gears-level model of what's going on would also make this feel complete to me. Here's me trying to come up with one on the spot:
Discourse around politics is a pretty charged and emotionally difficult thing for most people, in ways that can subvert our normally-functioning mechanisms of updating our beliefs. When we encounter object-level evidence that contradicts our beliefs, we feel a negative emotion/experience (in a quick/flash). One palliative to this feeling is to "go meta" - hop up the ladder of abstraction to a place where the belief is not in danger. We habituate ourselves to it by seeing others do similarly and imitation is enough to propagate this without anyone doing it intentionally. This model implicitly makes predictions about how to make spaces/contexts where more object level discussions happen (less negative experience, more emotional safety) as well as what kinds of internal changes would facilitate more object level discussions (train people to notice these fast emotional reactions and their corresponding mental moves).
Another thing that would make this article feel 'complete' to me would be to compare the 'politics' domain to other domains familiar to folks here on lesswrong (candidates are: effective altruism, AI alignment, rationality, etc). Is the other domain too meta in the way politics is? Is it too object level? It seems like the downsides (insufficient exploration, self-censorship, distraction) could apply to a much bigger domain of thought.
Thoughts, mostly on an alternative set of next experiments:
I find interpolations of effects to be a more intuitive way to study treatment effects, especially if I can modulate the treatment down to zero in a way that smoothly and predictably approaches the null case. It's not exactly clear to me what the "nothing going on case is", but here's some possible experiments to interpolate between it and your treatment case.
- alpha interpolation noise: A * noise + (A - 1) * MNIST, where the null case is the all-noise case. Worth probably trying out a bunch of different noise models since mnist doesn't really look at all gaussian.
- shuffle noise: Also worth looking at pixel/row/column shuffles, within an example or across dataset, as a way of preserving some per-pixel statistics while still reducing the structure of the dataset to basically noise. Here the null case is again that "fully noised" data should be the "nothing interesting" case, but we don't have to do work to keep per-pixel-statistics constant
- data class interpolation: I think the simplest version of this is dropping numbers, and maybe just looking at structurally similar numbers (e.g. 1,7 vs 1,7,9). This doesn't smoothly interpolate, but still having a ton of different comparisons with different subsets of the numbers. The null case here is that more digits adds more structure
- data size interpolation: downscaling the images, with or without noise, should reduce the structure such that the small / less data an example has, the closer it resembles the null case
- suboptimal initializations: neural networks are pretty hard to train (and can often degenerate) if initialized incorrectly. I think as you move away from optimal initialization (both of model parameters and optimizer parameters), it should approach the null / nothing interesting case.
- model dimensionality reduction: similar to intrinsic dimensionality, you can artificially reduce the (linear) degrees of freedom of the model without significant decrease to its expressivity by projecting into a smaller subspace. I think you'd need to get clever about this, because i think the naive version would just be linear projection before your linear operation (and then basically a no-op).
I mostly say all this because I think it's hard to evaluate "something is up" (predictions dont match empirical results) in ML that look like single experiments or A-B tests. It's too easy (IMO) to get bugs/etc. Smoothly interpolating effects, with one side as a well established null case / prior case, and another different case; which vary smoothly with whatever treatment, is IMO strong evidence that "something is up".
Hope there's something in those that's interesting and/or useful. If you haven't already, I strongly recommend checking out the intrinsic dimensionality paper -- you might get some mileage by swapping your cutoff point for their phase change measurement point.
I think at this point these feel like empirical questions, which I think would be much more clearly answered by demonstrations or experiments.
Trying to encode an additional penalty on changing non-semantic information is an interesting idea.
However I think you're missing that you don't have the ability to directly compare to a reference LM in cases where you're training to improve on some performance benchmark. During training the model will change its predictions on everything to some degree (both semantic and nonsemantic content).
So your proposed detection will always show some amount of disagreement between the prior and the trained model on weird grammatical patterns as well as conceptual tokens. The question is: "is the difference merely due to the changes to improve performance, or is it also transmitting hidden information"
If what you’re saying is “any change to the distribution will change KL” — I think that’s just correct.
This also applies to changes during training where the model is learning to perform better on the objective task.
So we are expecting some amount of KL divergence already.
My claims are:
-
The cheapest place to hide information (due to KL) are places where the model already has high entropy (ie it is uncertain between many possible outputs)
-
optimization pressure will try to push this extra information into the cheapest places to hide
-
the increase in KL won’t be clearly distinguishable from the increase due to increased performance on the task
I think that’s right but also the moral maze will be mediating the information and decision making support that’s available to the leadership, so they’re not totally immune from the influences
I think I understand what you're saying, but I want to double check and try laying it out explicitly.
- The "null space" is actually the null space of the prior model, which is human-like, but not exactly lined up to human perception of language
- Because of this, the information is "hidden" w.r.t. human perceptions, it's "hidden" w.r.t. the language model prior
I think I agree with both of these points, but here's my thinking for why I still expect to see this phenomena (and why the article above was simplified to just be "human")
- Empirically drawing many samples results in lots of "contains similar high level contents according to a human (me)"
- It might be worth studying this explicitly, such as setting up a bunch of chain of thought reasoning like scenarios, and sampling a lot of generated reasoning, then asking humans to rate how similar the generations are
- My prediction is that people will think that they are largely similar
- We could also use this same method to study human-measured relevance of other non-trivial sampling methods, like beam search, etc
- "Hiding" w.r.t. language model prior is sufficient, in the limit of good language models
- One of the things I think my reasoning relies on is that we will continue to produce better language models, which means the prior over time more and more closely resembles human priors
- This suggests that one way to avoid this phenomena is to only use smaller / less capable models -- or at least they will do a worse job at hiding information. I currently expect that many research labs will continue to do chain of thought reasoning with state of the art models, though.
- There's a lot of room in the model distribution
- Chain of thought reasoning is not currently, nor do I expect it will ever be, overconstrained.
- Instead, it can be seen as a mechanism for dramatically increasing the space of possible outputs, both those seen during training, and those encountered at test time
- Human preference effects
- Research Campaign Effects: Human researchers will want human-like outputs, and thus will be expected to tweak/retrain/etc in order to get human-like reasoning in the chain of thought. This introduces a separate optimization pressure from the KL penalty towards reasoning being human-like
- Human preference models: I think an easy-to-predict future direction here is training reward models to match subjective human preferences and then optimizing with them or sampling with them (e.g. best of N) -- these preference models could also introduce additional optimization pressure towards human-like
I think the most important point in all of this is that I expect the model prior to be sufficiently close to the human prior -- and I'd be interested in learning more about evidence or research to the contrary.
I think this is worth considering even in cases of things like recursive prompting for a couple of reasons that I haven't organized:
- In general additional compute time lets you improve your answer, so by default I'd expect answers to any significant question to take multiple steps
- I agree for trivial questions it might not do any of this. My expectation is that AI deployments impacts will mostly be on things like non-trivial questions or tasks
- So far current research hasn't done a great deal of regularizing or penalizing models for using a lot of thinking
- Mostly they've optimized for "maximize the chance of correct answer"
- This could change in the future, especially in cases where the model is expected to be deployed / otherwise sensitive to runtime costs
- Steganography is rewarded over openness if the openness is somehow far from the prior for a given piece of information it wants to transmit
- Caveat this point is more speculative than the above document
- One of my points is that due to null space + optimization pressure, it's very easy and convenient for gradient descent to put information in these hidden dimensions
- I think one of the things this can do well is handle concepts that don't yet exist -- and so reasoning moves like "invent a new term" or "give an illustrative example" or "invent a thought experiment" are lower likelihood under the prior
- In these cases, it can be more likely for steganography to transmit certain information, if it is less expensive in terms of KL penalties
- I do agree that for straightforward reasoning on straightforward evidence, where the reasoning is human-like and close to the prior, we can expect it to mostly reason out loud in a way that is "open"
- Myopia -- though less important than it was earlier in RFLO research -- seemed like a good alignment criterion to aim for, and still does today somewhat
- The original starting point for this direction of thinking was about Myopia / Non-Myopia in chain of thought reasoning systems
- The sorts of guarantees I was hoping to get for chain of thought systems included myopia guarantees
- Steganography came up as an example of how it could break myopia (other more complicated examples include side effects and situational awareness)
- The concepts in this document came up by asking "how might steganography arise naturally given current research techniques and directions"
Putting what I think is the most important part of my reply first: I think research into mitigations is premature and instead demonstrating/measuring the phenomena should take priority.
However given that, I think I agree that these are all possible mitigations to the phenomena, in particular (rephrasing your points):
- Reducing the channel capacity, and thus the amount of information that can be transmitted
- Constraining the outputs, and thus reducing the degrees of freedom of the null space
- Adding noise (via dropout or otherwise) also reduces the channel capacity, and probably also has some desirable side effects (like increasing the diversity or creativity of reasoning)
- Introducing "barriers" past which optimization for hidden information becomes difficult (in your example, you use different models; I also think "stop gradients" in end-to-end training has similar effects, if weaker)
- If you can detect/localize this phenomena, you might be able to use interpretability like techniques to limit or constrain it
- I'm less certain about this, but possible future interpretability research will unlock this ability.
Also I think an important point here is that we can expect to see it with fairly simple techniques -- merely including sampled/generated data in the training set is sufficient, as opposed to it requiring a complicated reinforcement learning algorithm like MuZero.
Did you publish your proposal? I'd be interested in reading it.
Agree that founders are a bit of an exception. Actually that's a bit in the longer version of this when I talk about it in person.
Basically: "The only people who at the very top of large tech companies are either founders or those who were able to climb to the tops of moral mazes".
So my strategic corollary to this is that it's probably weakly better for AI alignment for founders to be in charge of companies longer, and to get replaced less often.
In the case of facebook, even in the face of all of their history of actions, I think on the margin I'd prefer the founder to the median replacement to be leading the company.
(Edit: I don't think founders remaining at the head of a company isn't evidence that the company isn't a moral maze. Also I'm not certain I agree that facebook's pivot couldn't have been done by a moral maze.)
Thanks, fixed the link in the article. Should have pointed here: https://www.lesswrong.com/posts/dhj9dhiwhq3DX6W8z/hero-licensing
I think there should be a norm about adding the big-bench canary string to any document describing AI evaluations in detail, where you wouldn't want it to be inside that AI's training data.
Maybe in the future we'll have a better tag for "dont train on me", but for now the big bench canary string is the best we have.
This is in addition to things like "maybe don't post it to the public internet" or "maybe don't link to it from public posts" or other ways of ensuring it doesn't end up in training corpora.
I think this is a situation for defense-in-depth.
More Ideas or More Consensus?
I think one aspect you can examine about a scientific field is it's "spread"-ness of ideas and resources.
High energy particle physics is an interesting extrema here -- there's broad agreement in the field about building higher energy accelerators, and this means there can be lots of consensus about supporting a shared collaborative high energy accelerator.
I think a feature of mature scientific fields that "more consensus" can unlock more progress. Perhaps if there had been more consensus, the otherwise ill-fated superconducting super collider would have worked out. (I don't know if other extenuating circumstances would still prevent it.)
I think a feature of less mature scientific fields that "more ideas" (and less consensus) would unlock more progress. In this case, we're more limited about generating and validating new good ideas. One way this looks is that there's not a lot of confidence with what to do with large sums of research funding, and instead we think our best bet is making lots of small bets.
My field (AI alignment) is a less mature scientific field in this way, I think. We don't have a "grand plan" for alignment, which we just need to get funding. Instead we have a fractal of philanthropic organizations empowering individual grantmakers to try to get small and early ideas off the ground with small research grants.
A couple thoughts, if this model does indeed fit:
There's a lot more we could do to orienting as a field with "the most important problem is increasing the rate of coming up with good research ideas". In addition to being willing to fund lots of small and early stage research, I think we could factorize and interrogate the skills and mindsets needed to do this kind of work. It's possible that this is one of the most important meta-skills we need to improve as a field.
I also think this could be more of a priority when "field building". When recruiting or trying to raise awareness of the field, it would be good to consider more focus or priority on places where we expect to find people who are likely to be good generators of new ideas. I think one of the ways this looks is to focus on more diverse and underrepresented groups.
Finally, at some point it seems like we'll transition to "more mature" as a field, and it's good to spend some time thinking about what would help that go better. Understanding the history of other fields making this transition, and trying to prepare for predicted problems/issues would be good here.
AGI will probably be deployed by a Moral Maze
Moral Mazes is my favorite management book ever, because instead of "how to be a good manager" it's about "empirical observations of large-scale organizational dynamics involving management".
I wish someone would write an updated version -- a lot has changed (though a lot has stayed the same) since the research for the book was done in the early 1980s.
My take (and the author's take) is that any company of nontrivial size begins to take on the characteristics of a moral maze. It seems to be a pretty good null hypothesis -- any company saying "we aren't/won't become a moral maze" has a pretty huge evidential burden to cross.
I keep this point in mind when thinking about strategy around when it comes time to make deployment decisions about AGI, and deploy AGI. These decisions are going to be made within the context of a moral maze.
To me, this means that some strategies ("everyone in the company has a thorough and complete understanding of AGI risks") will almost certainly fail. I think the only strategies that work well inside of moral mazes will work at all.
To sum up my takes here:
- basically every company eventually becomes a moral maze
- AGI deployment decisions will be made in the context of a moral maze
- understanding moral maze dynamics is important to AGI deployment strategy
(Caveat: I ran the first big code scrape and worked on the code generating models which later became codex.)
My one line response: I think opt-out is obviously useful and good and should happen.
AFAIK there are various orgs/bodies working on this but kinda blanking what/where. (In particular there's a FOSS mailing list that's been discussing how ML training relates to FOSS license rights that seems relevant)
Opt-out strings exist today, in an insufficient form. The most well known and well respected one is probably the big-bench canary string: https://github.com/google/BIG-bench/blob/main/docs/doc.md -- but this is just intended to protect data used for evaluating text models.
Mimicking the structure to comment on each point:
Simplicity
I think simplicity is points in favor of cheapness, but not points (directly) in favor of why something "should be done". I see this as "technical cost to implement are low", and agree.
Competitiveness
I think this also is points in favor of cheapness, but again not why it "should be done". I see this as "expected reduction in ML perf is small", and agree.
Ethics
I think this makes the point that we currently don't have settled understanding on what the ethics of various options are here. People being upset at the state of things is pretty strong evidence that it's not settled, but seems to be less strong evidence that it's unethical. I can't tell the point you're trying to make here is that "we should figure out the ethics of opt-out" (which I agree with) or that "opt-out is ethically required" (which I don't think you've sufficiently supported here for me to agree with).
Risk
I see this as making the point "opt-out would (very minorly) reduce AI risk". I think this is both well supported by the arguments and technically valid. I'm personally skeptical about the amount of protection this gets us, and am mostly optimistic in applying it to non-software domains (e.g. nanotech, gain of function, virology, etc).
A personal technical prediction I can add: I think that in the software domain, it will be inexpensive for a capable system to compose any non-allowed concepts out of allowed concepts. I think this is non-obvious to traditional ML experts. In traditional ML, removing a domain from the dataset usually robustly removes it from the model -- but things like the large-scale generative models mentioned in the top of the post have generalized very well across domains. (They're still not very capable in-domain, but are similarly not-capable in domains that didn't exist in training.) I think this "optimism about generalization" is the root of a bunch of my skepticism about domain-restriction/data-censoring as a method of restricting model capabilities.
Precedent
I think the robots.txt example is great and basically this is the one that is most directly applicable. (Other precedents exist but IMO none are as good.) I totally agree with this precedent.
Separately, there's a lot of precedent for people circumventing or ignoring these -- and I think it's important to look at those precedents, too!
Risk Compensation
This is an interesting point. I personally don't weigh this highly, and feel like a lot of my intuition here is attached to gut-level stuff.
As far as I know, the literature on risk compensation is almost entirely about things that are direct personal risk to someone. I don't know of any cases of risk compensation where the risk was indirect or otherwise largely separated from the person. (At some point of indirectness this seems to reduce more to a "principal-agent problem" than a risk-compensation problem)
What's Missing
I think it's easy to focus on the technical implementation costs and less on the "what happens next" costs. Figuring out the legal status of this opt-out (and possibly pushing for legislation to change this) is difficult and expensive. Figuring out standards for evaluation will be similarly hard, especially as the tech itself changes rapidly.
Personal Conclusion
I think opt-out is obviously good and useful and should be done. It think its a pretty clear positive direction for ML/AI policy and regulatory development -- and also I'm optimistic that this is the sort of thing that will happen largely on its own (i.e. no drastic action is required).
Sometimes I get asked by intelligent people I trust in other fields, "what's up with AI x risk?" -- and I think at least part of it unpacks to this: Why don't more people believe in / take seriously AI x-risk?
I think that is actually a pretty reasonable question. I think two follow-ups are worthwhile and I don't know of good citations / don't know if they exist:
- a sociological/anthropological/psychological/etc study of what's going on in people who are familiar with the ideas/reasonings of AI x-risk, but decide not to take it seriously / don't believe it. I expect in-depth interviews would be great here.
- we should probably just write up as many obvious things ourselves up front.
The latter one I can take a stab at here. Taking the perspective of someone who might be interviewed for the former:
- historically, ignoring anyone that says "the end of the world is near" has been a great heuristic
- very little of the public intellectual sphere engages with the topic
- the public intellectual sphere that does in engages is disproportionately meme lords
- most of the writings about this are exceptionally confusing and jargon-laden
- there's no college courses on this / it doesn't have the trappings of a legitimate field
- it feels a bit like a Pascal's mugging -- at the very least i'm not really prepared to try to think about actions/events with near-infinite consequences
- people have been similarly doom-y about other technologies and so far the world turned out fine
- we have other existential catastrophes looming (climate change, etc) that are already well understood and scientifically supported, so our efforts are better put on that than this confusing hodge-podge
- this field doesn't seem very diverse and seems to be a bit monocultural
- this field doesn't seem to have a deep/thorough understanding of all of the ways technology is affecting people's lives negatively today
- it seems weird to care about future people when there are present people suffering
- I see a lot of public disagreement about whether or not AGI is even real, which makes the risk arguments feel much less trustworthy to me
I think i'm going to stop for now, but I wish there was a nice high-quality organization of these. At the very least, having the steel-version of them seems good to have around, in part as an "epistemic hygiene" thing.
Thanks so much for making this!
I'm hopeful this sort of dataset will grow over time as new sources come about.
In particular, I'd nominate adding MLSN (https://www.alignmentforum.org/posts/R39tGLeETfCZJ4FoE/mlsn-4-many-new-interpretability-papers-virtual-logit) to the list of newsletters in the future.
This seems like an overly alarmist take on what is a pretty old trend of research. Six years ago there was a number of universities working on similar models for the VizDoom competition (IIRC they were won by Intel and Facebook). It seems good to track this kind of research, but IMO the conclusions here are not supported at all by the evidence presented.
Do you have suggestions for domains where you do expect one-turn debate to work well, now that you've got these results?
Congratulations! Can you say if there will be a board, and if so who will start on it?
Longtermist X-Risk Cases for working in Semiconductor Manufacturing
Two separate pitches for jobs/roles in semiconductor manufacturing for people who are primarily interested in x-risk reduction.
Securing Semiconductor Supply Chains
This is basically the "computer security for x-risk reduction" argument applied to semiconductor manufacturing.
Briefly restating: it seems exceedingly likely that technologies crucial to x-risks are on computers or connected to computers. Improving computer security increases the likelihood that those machines are not stolen or controlled by criminals. In general, this should make things like governance and control strategy more straightforward.
This argument also applies to making sure that there isn't any tampering with the semiconductor supply chain. In particular, we want to make sure that the designs from the designer are not modified in ways that make it easier for outside actors to steal or control information or technology.
One of the primary complaints about working in semiconductor manufacturing for longtermist reasons is accelerating semiconductor progress. I think security work here is not nearly as much as a direct driver of progress as other roles, so I would argue this as differentially x-risk reducing.
Diversifying Semiconductor Manufacturing
This one is more controversial in mainline longtermist x-risk reduction, so I'll try to clearly signpost the hypotheses that this is based on.
The reasoning is basically:
- Right now, most prosaic AI alignment techniques require access to a lot of compute
- It's possible that some prosaic AI alignment techniques (like interpretability) will require much more compute in the future
- So, right now AI alignment research is at least partially gated on access to compute, and it seems plausible this will be the case in the future
So if we want to ensure these research efforts continue to have access to compute, we basically need to make sure they have enough money to buy the compute, and that there is compute to be sold.
Normally this wouldn't be much of an issue, as in general we can trust markets to meet demands, etc. However semiconductor manufacturing is increasingly becoming a part of international conflict strategy.
In particular, much of the compute acceleration used in AI research (including AI alignment research) is manufactured in Taiwan, which seems to be coming under increasing threats.
My argument here is that I think it is possible to increase the chances that AI alignment research labs will continue to have access to compute, even in cases of large-scale geopolitical conflict. I think this can be done in ways that end up not dramatically increasing the global semiconductor manufacturing capacity by much.
I think your explanation of legibility here is basically what I have in mind, excepting that if it's human designed it's potentially not all encompassing. (For example, a world model that knows very little, but knows how to search for information in a library)
I think interpretability is usually a bit more narrow, and refers to developing an understanding of an illegible system. My take is that it is not "interpretability" to understand a legible system, but maybe I'm using the term differently than others here. This is why I don't think "interpretability" applies to systems that are designed to be always-legible. (In the second graph, "interpretability" is any research that moves us upwards)
I agree that the ability to come up with totally alien and untranslateable to humans ideas gives AGI a capabilities boost. I do think that requiring a system to only use legible cognition and reasoning is a big "alignment tax". However I don't think that this tax is equivalent to a strong proof that legible AGI is impossible.
I think my central point of disagreement with this comment is that I do think that it's possible to have compact world models (or at least compact enough to matter). I think if there was a strong proof that it was not possible to have a generally intelligent agent with a compact world model (or a compact function which is able to estimate and approximate a world model), that would be an update for me.
(For the record, I think of myself as a generally intelligent agent with a compact world model)
Two Graphs for why Agent Foundations is Important (according to me)
Epistemic Signpost: These are high-level abstract reasons, and I don’t go into precise detail or gears-level models. The lack of rigor is why I’m short form-ing this.
First Graph: Agent Foundations as Aligned P2B Fixpoint
P2B (a recursive acronym for Plan to P2B Better) is a framing of agency as a recursively self-reinforcing process. It resembles an abstracted version of recursive self improvement, which also incorporates recursive empowering and recursive resource gathering. Since it’s an improvement operator we can imagine stepping, I’m going to draw an analogy to gradient descent.
Imagine a highly dimensional agency landscape. In this landscape, agents follow the P2B gradient in order to improve. This can be convergent such that two slightly different agents near each other might end up at the same point in agency space after some number of P2B updates.
Most recursive processes like these have fixed point attractors — in our gradient landscape these are local minima. For P2B these are stable points of convergence.
Instead of thinking just about the fixed point attractor, lets think about the parts of agency space that flow into a given fixed point attractor. This is like analyzing watersheds on hilly terrain — which parts of the agency space flow into which attractors.
Now we can have our graph: it’s a cartoon of the “agency landscape” with different hills/valleys flowing into different local minimum, colored by which local minimum they flow into.
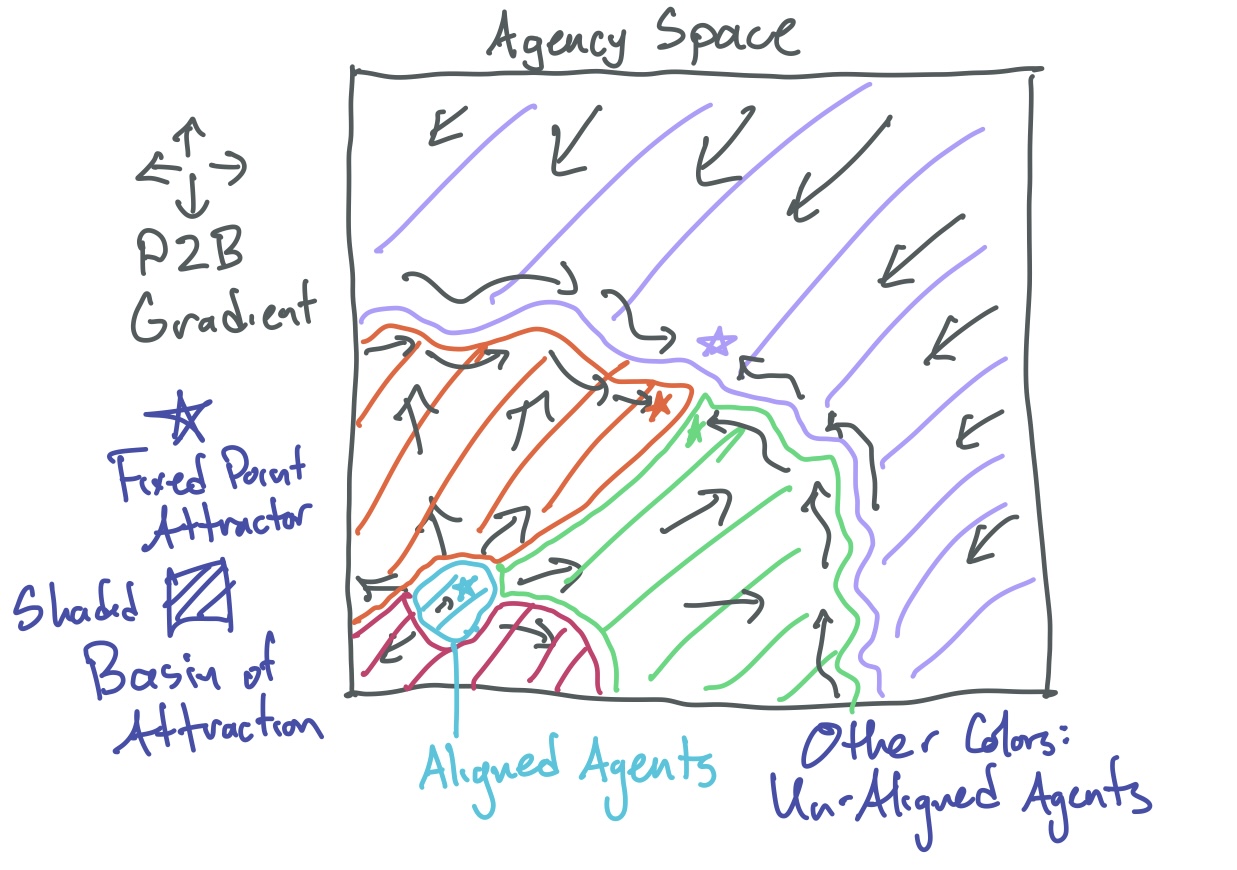
Here we have a lot of different attractors in agency space, but almost all of them are unaligned, what we need to do is get the tiny aligned attractor in the corner.
However it’s basically impossible to initialize an AI at one of these attractors, the best we can do is make an agent and try to understand where in agency space they will start. Building an AGI is imprecisely placing a ball on this landscape, which will roll along the P2B gradient towards its P2B attractor.
How does this relate to Agent Foundations? I see Agent Foundations as a research agenda to write up the criterion for characterizing the basin in agent space which corresponds to the aligned attractor. With this criterion, we can try to design and build an agent, such that when it P2Bs, it does so in a way that is towards an Aligned end.
Second: Agent Foundations as designing an always-legible model
ELK (Eliciting Latent Knowledge) formalized a family of alignment problems, eventually narrowing down to the Ontology Mapping Problem. This problem is about translating between some illegible machine ontology (basically it’s internal cognition) and our human ontology (concepts and relations that a person can understand).
Instead of thinking of it as a binary, I think we can think of the ontology mapping problem as a legibility spectrum. On one end of the spectrum we have our entirely illegible bayes net prosaic machine learning system. On the other end, we have totally legible machines, possibly specified in a formal language with proofs and verification.
As a second axis I’d like to imagine development progress (this can be “how far along” we are, or maybe the capabilities or empowerment of the system). Now we can show our graph, of different paths through this legibility vs development space.
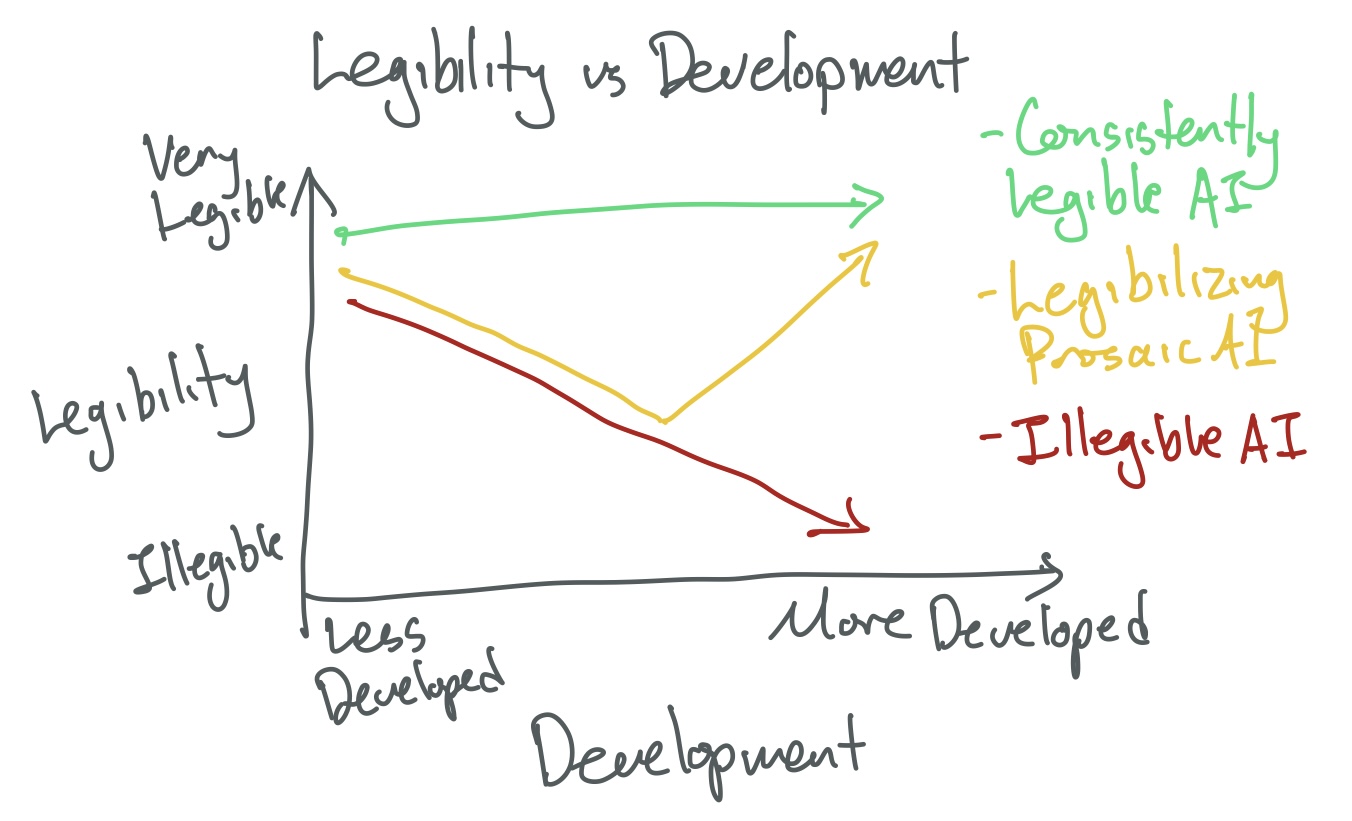
Some strategies move away from legibility and never intend to get back to it. I think these plans have us building an aligned system that we don’t understand, and possibly can’t ever understand (because it can evade understanding faster than we can develop understanding).
Many prosaic alignment strategies are about going down in legibility, and then figuring out some mechanism to go back up again in legibility space. Interpretability, ontology mapping, and other approaches fit in this frame. To me, this seems better than the previous set, but still seem skeptical to me.
Finally my favorite set of strategies are ones that start legible and endeavor to never deviate from that legibility. This is where I think Agent Foundations is in this graph. I think there’s too little work on how we can build an Aligned AGI which is legible from start-to-finish, and almost all of them seem to have a bunch of overlap with Agent Foundations.
Aside: earlier I included a threshold in legibility space that‘s the “alignment threshold” but that doesn’t seem to fit right to me, so I took it out.
Maybe useful: an analogy this post brought to mind for me: Replacing “AI” with “Animals”.
Hypothetical alien civilization, observing Early Earth and commenting on whether it poses a risk.
Doesn’t optimization nature produce non-agentic animals? It mostly does, but those aren’t the ones we’re concerned with. The risk is all concentrated in the agentic animals.
Basically every animal ever is not agentic. I’ve studied animals for my entire career and I haven’t found an agentic animal yet. That doesn’t preclude them showing up in the future. We have reasons to believe that not only are agents possible, but they are likely.
Even if agentic animals showed up, they would be vastly outnumbered by all the other animals. We believe that agency will give the agentic animals such a drastic advantage, that they will seem to take over the world in a very short amount of time.
(Etc etc)
(Possible that this is in one of the things you cite, and either I missed it or I am failing to remember it)
Hacking the Transformer Prior
Neural Network Priors
I spend a bunch of time thinking about the alignment of the neural network prior for various architectures of neural networks that we expect to see in the future.
Whatever alignment failures are highly likely under the neural network prior are probably worth a lot of research attention.
Separately, it would be good to figure out knobs/levers for changing the prior distribution to be more aligned (or produce more aligned models). This includes producing more interpretable models.
Analogy to Software Development
In general, I am able to code better if I have access to a high quality library of simple utility functions. My goal here is to sketch out how we could do this for neural network learning.
Naturally Occurring Utility Functions
One way to think about the induction circuits found in the Transformer Circuits work is that they are "learned utility functions". I think this is the sort of thing we might want to provide the networks as part of a "hacked prior"
A Language for Writing Transformer Utility Functions
Thinking Like Transformers provides a programming language, RASP, which is able to express simple functions in terms of how they would be encoded in transformers.
Concrete Research Idea: Hacking the Transformer Prior
Use RASP (or something RASP-like) to write a bunch of utility functions (such as the induction head functions).
Train a language model where a small fraction of the neural network is initialized to your utility functions (and the rest is initialized normally).
Study how the model learns to use the programmed functions. Maybe also study how those functions change (or don't, if they're frozen).
Future Vision
I think this could be a way to iteratively build more and more interpretable transformers, in a loop where we:
- Study transformers to see what functions they are implementing
- Manually implement human-understood versions of these functions
- Initialize a new transformer with all of your functions, and train it
- Repeat
If we have a neural network that is eventually entirely made up of human-programmed functions, we probably have an Ontologically Transparent Machine. (AN: I intend to write more thoughts on ontologically transparent machines in the near future)
I think there’s a lot going on with your equivocating the speed prior over circuits w/ a speed prior over programs.
I think a lot of the ideas in this direction are either confused by the difference between circuit priors and program priors, or at least treating them as equivalent. Unfortunately a lot of this is vague until you start specifying the domain of model. I think specifying this more clearly will help communicating about these ideas. To start with this myself, when I talk about circuit induction, I’m talking about things that look like large randomly initialized bayes nets (or deep neural networks).
Program Induction Priors are bad: I would claim that any program induction priors (simplicity prior, speed prior, others) are almost always a bad fit for developing useful intuitions about the behavior of large random bayes net machines.
Confusion between circuit induction speed prior and simplicity prior: I think your point about double descent is wrong — in particular, the speed is largely unchanged in double descent experiments, since the *width* is the parameter being varied, and all deep neural networks of the same depth have approximately the same speed (unless you mean something weird by speed).
Circuit Simplicity: You give circuit-size and circuit-depth as examples of a “speed prior”, which seems pretty nonstandard, especially when describing it as “not the simplicity prior”.
More than Speed and Simplicity: I think there are other metrics that provide interesting priors over circuits, like likelihood under some initialization distribution. In particular, I think “likelihood under the initialization distribution” is the prior that matters most, until we develop techniques that let us “hack the prior”.
Connection to Infinite-Size Neural Networks: I think research about neural networks approaching/at the infinite limit looks a lot like physics about black holes — and similarly can tell us interesting things about dynamics we should expect. In particular, for systems optimized by gradient descent, we end up with infinitesimal/nonexistent feature learning in the limit — which is interesting because all of the sub-modules/sub-circuits we start with are all we’ll ever have! This means that even if there are “simple” or “fast” circuits, if they’re not likely under the initialization distribution, then we expect they’ll have a vanishingly small effect on the output. (One way of thinking about this is in terms of the NTK, that even if we have extremely powerfully predictive modules, their predictive power will be overwhelmed by the much more common and simple features)
Hacking the Prior: Right now we don’t have a good understanding of the behavior of partially-hand coded neural networks, but I think they could serve as a new/distinct class of models (with regards to what functions are likely under the initialization distribution). Concretely, this could look like us “hand-programming” circuits or parts of neural networks, then randomly initializing the rest, and see if during training the model learns to use those programmed functions.
Interpretability Challenges
Inspired by a friend I've been thinking about how to launch/run interpretability competitions, and what the costs/benefits would be.
I like this idea a lot because it cuts directly at one of the hard problems of spinning up in interpretability research as a new person. The field is difficult and the objectives are vaguely defined; it's easy to accidentally trick yourself into seeing signal in noise, and there's never certainty that the thing you're looking for is actually there.
On the other hand, most of the interpretability-like interventions in models (e.g. knowledge edits/updates to transformers) make models worse and not better -- they usually introduce some specific and contained deficiency (e.g. predict that the Eiffel Tower is in Rome, Italy).
So the idea for Interpretability Challenges would be to use existing methods (or possibly invent new ones) to inject concrete "things to find" inside of models, release those models as challenges, and then give prizes for finding things.
Some ways this might work:
- Super simple challenge: use editing techniques like ROME to edit a model, upload to google drive, and post a challenge to lesswrong. I'd probably personally put up a couple of prizes for good writeups for solutions.
- CTF (Capture the Flag): the AI Village has been interested in what sorts of AI challenges/competitions could be run in tandem with infosec conferences. I think it would be pretty straightforward to build some interpretability challenges for the next AI Village CTF, or to have a whole interpretability-only CTF by itself. This is exciting to me, because its a way to recruit more people from infosec into getting interested in AI safety (which has been a goal of mine for a while).
- Dixit-rules challenge league: One of the hard problems with challenges like this is how to set the difficulty. Too hard and no one makes progress. Too easy and no one learns/grows from it. I think if there were a bunch of interested people/groups, we could do a dixit style tournament: Every group takes turns proposing a challenge, and gets the most points if exactly one other group solves it (they don't get points if everyone solves it, or if no one solves it). This has a nice self-balancing force, and would be good if there wanted to be an ongoing group who built new challenges as new interpretability research papers were published.
Please reach out to me if you're interested in helping with efforts like this.
My Cyberwarfare Concerns: A disorganized and incomplete list
- A lot of internet infrastructure (e.g. BGP / routing) basically works because all the big players mostly cooperate. There have been minor incidents and attacks but nothing major so far. It seems likely to be the case that if a major superpower was backed into a corner, it could massively disrupt the internet, which would be bad.
- Cyberwar has a lot of weird asymmetries where the largest attack surfaces are private companies (not militaries/governments). This gets weirder when private companies are multinational. (Is an attack on google an attack on ireland? USA? Neither/both?)
- It's unclear who is on whose side. The Snowden leaks showed that american intelligence was hacking american companies private fibers on american soil, and the trust still hasn't recovered. It's a low-trust environment out there, which seems (to me) to make conflict more likely to start, and harder to contain and extinguish once started.
- There is no good international "law of war" with regards to cyberwarfare. There are some works-in-progress which have been slowly advancing, but there's nothing like the geneva convention yet. Right now existing cyber conflicts haven't really pushed the "what is an illegal attack" sense (in the way that land mines are "illegal" in war), and the lack of clear guidance here means that in an all-out conflict there isn't much in the way of clear limitations.
- Many cyber attacks are intentionally vague or secret in origin. Some of this is because groups are distributed and only loosely connected to national powers (e.g. via funding, blind eyes, etc) and some because it's practically useful to have plausible deniability. This really gets in the way of any sort of "cease fire" or armistice agreements -- if a country comes to the peace treaty table for a given cyber conflict, this might end up implicating them as the source of an attack.
- Expanding the last point more, I'm worried that there are a lot of "ratchet-up" mechanisms for cyberwarfare, but very few "ratchet-down" mechanisms. All of these worries somewhat contribute to a situation where if the currently-low-grade-burning-cyberwar turns into more of an all-out cyberwar, we'll have very few tools for deescalation.
- Relating this to my concerns about AGI safety, I think an 'all-out cyberwar' (or at least a much larger scale one) is one of the primary ways to trigger an AGI weapons development program. Right now it's not clear to me that much of weapons development budget is spent on cyberweapons (as opposed to other capabilities like SIGINT), but a large-scale cyberwar seems like a reason to invest more. The more money is spent on cyberweapons development, the more likely I think it is that an AGI weapons program is started. I'm not optimistic about the alignment or safety of an AGI weapons program.
Maybe more to come in the future but that's it for now.
I think that the authors at least did some amount of work to distinguish the eras, but agree more work could be done.
Also I agree w/ Stella here that Turing, GPT-J, GShard, and Switch are probably better fit into the “large scale“ era.
I with more of the language alignment research folks were looking into how current proposals for aligning transformers end up working on S4 models.
(I am one of said folks so maybe hypocritical to not work on it)
In particular it seems like there's way in which it would be more interpretable than transformers:
- adjustable timescale stepping (either sub-stepping, or super-stepping time)
- approximately separable state spaces/dynamics -- this one is crazy conjecture -- it seems like it should be possible to force the state space and dynamics into separate groups, in ways that would allow analysis of them in isolation or in relation to the rest of the model
It does seem like they're not likely to be competitive with transformers for short-context modeling anytime soon, but if they end up being differentially alignment-friendly, then we could instead try to make them more competitive.
(In general I think it's much easier to make an approach more competitive than it is to make it more aligned)
I work on this sort of thing at OpenAI.
I think alignment datasets are a very useful part of a portfolio approach to alignment research. Right now I think there are alignment risks/concerns for which datasets like this wouldn't help, but also there are some that it would help for.
Datasets and benchmarks more broadly are useful for forecasting progress, but this assumes smooth/continuous progress (in general a good assumption -- but also good to be wary of cases where this isn't the case).
Some thoughts from working on generating datasets for research, and using those datasets in research:
- Start by building tiny versions of the dataset yourself
- It's good to switch early to paying labelers/contractors to generate and labels -- they won't be perfect at first, so there's a lot of iterating in clarifying instructions/feedback/etc
- It's best to gather data that you'd want to use for research right away, not for some nebulous possible future research
- Getting clean benchmarks that exhibit some well-defined phenomena is useful for academics and grad students
- When in doubt, BIG-Bench is a good place to submit these sorts of tiny evaluative datasets
- Where possible, experiment with using models to generate more data (e.g. with few-shot or generative modeling on the data you have)
- Sometimes a filter is just as good as data (a classifier that distinguishes data inside the desired distribution)
I think this is a great idea, but would be best to start super small. It sounds right now like a huge project plan, but I think it could be road-mapped into something where almost every step along the path produces some valuable input.
Given the amount of funding available from charitable sources for AI alignment research these days, I think a good thing to consider is figuring out how to make instructions for contractors to generate the data, then getting money to hire the contractors and just oversee/manage them. (As opposed to trying to get volunteers to make all the data)
I worry a little bit about this == techniques which let you hide circuits in neural networks. These "hiding techniques" are a riposte to techniques based on modularity or clusterability -- techniques that explore naturally emergent patterns.[1] In a world where we use alignment techniques that rely on internal circuitry being naturally modular, trojan horse networks can avoid various alignment techniques.
I expect this to happen by default for a bunch of reasons. An easy one to point to is the "free software" + "crypto anarchist" + "fuck your oversight" + "digital privacy" cluster -- which will probably argue that the government shouldn't infringe your right to build and run neural networks that are not-aligned. Similar to how encrypting personal emails subverts attempts to limit harms of email, "encrypting neural network functions" can subvert these alignment techniques.
To me, it seems like this system ends up like a steganography equilibrium -- people find new hiding techniques, and others find new techniques to discover hidden information. As long as humans are both sides of this, I expect there to be progress on both sides. In the human-vs-human version of this, I think it's not too unevenly matched.
In cases where its human-vs-AI, I strongly expect the AI wins in the limit. This is in part why I'm optimistic about things like ELK solutions which might be better at finding "hidden modules" not just naturally occurring ones.
- ^
The more time I spend with these, the more I think the idea of naturally occuring modularity or clusterability makes sense / seems likely, which has been a positive update for me
Just copy-pasting the section
We believe that Transformative Artificial Intelligence (TAI) [Karnofsky et al., 2016] is approaching [Cotra, 2020, Grace et al., 2018], and that these systems will cause catastrophic damage if they are misaligned with human values [Fox and Shulman, 2013, Omohundro, 2008]. As such, we believe it is essential to prioritize and help facilitate technical research that ensures TAI’s values will be aligned with ours.
AI Alignment generally refers to the problem of how to ensure increasingly powerful and autonomous AI systems perform the users’ wishes faithfully and without unintended consequences. Alignment is especially critical as we approach human and superhuman levels of intelligence, as powerful optimization processes amplify small errors in goal specification into large misalignments [Goodhart, 1984, Manheim and Garrabrant, 2019, Fox and Shulman, 2013], and misalignments in this regime will result in runaway optimization processes that evade alteration or shutdown [Omohundro, 2008, Benson-Tilsen and Soares, 2016, Turner et al., 2021], posing a significant existential risk to humanity. Additionally, even if the goal is specified correctly, superhuman models may still develop deceptive subsystems that attempt to influence the real world to satisfy their objectives [Hubinger et al., 2021]. While current systems are not yet at the level where the consequences of misalignment pose an existential threat, rapid progress in the field of AI has increased the concern that the alignment problem may be seriously tested in the not-too-distant future.
Much of the alignment literature focuses on the more theoretical aspects of alignment [Demski and Garrabrant, 2020, Yudkowsky and Soares, 2018, Taylor, 2016, Garrabrant et al., 2016, Armstrong and Mindermann, 2018, Hubinger et al., 2021], abstracting away the specifics of how intelligence will be implemented, due to uncertainty over the path to TAI. However, with the recent advances in capabilities, it may no longer be the case that the path to TAI is completely unpredictable. In particular, recent increases in the capabilities of large language models (LLMs) raises the possibility that the first generation of transformatively powerful AI systems may be based on similar principles and architectures as current large language models like GPT. This has motivated a number of research groups to work on “prosaic alignment” [Christiano, 2016, Askell et al., 2021, Ouyang et al., 2021], a field of study that considers the AI alignment problem in the case of TAI being built primarily with techniques already used in modern ML. We believe that due to the speed of AI progress, there is a significant chance that this assumption is true, and, therefore, that contributing and enabling contributions to prosaic alignment research will have a large impact.
The open-source release of this model is motivated by the hope that it will allow alignment researchers who would not otherwise have access to LLMs to use them. While there are negative risks due to the potential acceleration of capabilities research, which may place further time pressure on solving the alignment problem, we believe the benefits of this release outweigh the risks of accelerating capabilities research.
It's worth probably going through the current deep learning theories that propose parts of gears-level models, and see how they fit with this. The first one that comes to mind is the Lottery Ticket Hypothesis. It seems intuitive to me that certain tasks correspond to some "tickets" that are harder to find.
I like the taxonomy in the Viering and Loog, and it links to a bunch of other interesting approaches.
This paper shows phase transitions in data quality as opposed to data size, which is an angle I hadn't considered before.
There's the google paper explaining neural scaling laws that describes these two regimes that can be transitioned between: variance-limited and resolution-limited. Their theory seems to predict that behavior between the two is similar to a phase boundary.
I think also there should be a bit of a null hypothesis. It seems like there are simple functional maps where even if the internal improvement on "what matters" (e.g. feature learning) is going smoothly, our metric of performance is "sharp" in a way that hides the internal improvement until some transition when it doesnt.
Accuracy metrics seem like an example of this -- where you get 1 point if the correct answer is highest probability, otherwise 0 points. It's easy to understand why this has a sharp transition in complex domains.
Personal take: I've been spending more and more time thinking about modularity, and it seems like modularity in learning could drive sharp transitions (e.g. "breakthroughs").
Decomposing Negotiating Value Alignment between multiple agents
Let's say we want two agents to come to agreement on living with each other. This seems pretty complex to specify; they agree to take each other's values into account (somewhat), not destroy each other (with some level of confidence), etc.
Neither initially has total dominance over the other. (This implies that neither is corrigible to the other)
A good first step for these agents is to share each's values with the other. While this could be intractably complex -- it's probably the case that values are compact/finite and can be transmitted eventually in some form.
I think this decomposes pretty clearly into ontology transmission and value assignment.
Ontology transmission is communicating one agents ontology of the objects/concepts to another. Then value assignment is communicating the relative or comparative values to different elements in the ontology.
I'm really excited about this research direction. It seems so well-fit to what you've been researching in that past -- so much so that it doesn't seem to be a new research direction so much as a clarification of the direction you were already pursuing.
I think producing a mostly-coherent and somewhat-nuanced generalized theory of alignment would be incredibly valuable to me (and I would consider myself someone working on prosaic alignment strategies).
A common thread in the last year of my work on alignment is something like "How can I be an aligned intelligence?" and "What action would I take here if I was an aligned intelligence?". This helps me bootstrap reasoning about my own experiences and abilities, and helps me think about extrapolations of "What if I had access to different information?" or "What if I could think about it for a very long time?".
I still don't have answers to these questions, but think they would be incredibly useful to have as an AI alignment researcher. They could inform new techniques as well as fundamentally new approaches (to use terms from the post: both de-novo and in-motion)
Summing up all that, this post made me realize Alignment Research should be its own discipline.
Addendum: Ideas for things along these lines I'd be interested in hearing more about in the future:
(not meant as suggestions -- more like just saying curiosities out loud)
What are the best books/papers/etc on getting the "Alex Flint worldview on alignment research"? What existing research institutions study this (if any)?
I think a bunch of the situations involving many people here could be modeled by agent-based simulations. If there are cases where we could study some control variable, this could be useful in finding pareto frontiers (or what factors shape pareto frontiers).
The habit formation example seems weirdly 'acausal decision theory' flavored to me (though this might be a 'tetris effect' like instance). It seems like habits similar to this are a mechanism of making trades across time/contexts with yourself. This makes me more optimistic about acausal decision theories being a natural way of expressing some key concepts in alignment.
Proxies are mentioned but it feels like we could have a rich science or taxonomy of proxies. There's a lot to study with historical use of proxies, or analyzing proxies in current examples of intelligence alignment.
The self-modification point seems to suggest an opposite point: invariants. Similar to how we can do a lot in physics by analysing conserved quantities and conservative fields -- maybe we can also use invariants in self-modifying systems to better understand the dynamics and equilibria.
Oh great, thanks. I think I was just asking folks if they thought it should be discussed separately (since it is a different piece) or together with this one (since they're describing the same research).
Should this other post be a separate linkpost for this? https://www.furidamu.org/blog/2022/02/02/competitive-programming-with-alphacode/#fnref:2
Feels like it covers the same, but is a personal description by an author, rather than the deepmind presser.
I think that's right that upgraded verification by itself is insufficient for 'defense wins' worlds. I guess I'd thought that was apparent but you're right it's definitely worth saying explicitly.
A big wish of mine is that we end up doing more planning/thinking-things-through for how researchers working on AI today could contribute to 'defense wins' progress.
My implicit other take here that wasn't said out loud is that I don't really know of other pathways where good theorem proving translates to better AI x-risk outcomes. I'd be eager to know of these.
Thoughts:
First, it seems worthwhile to try taboo-ing the word 'deception' and see whether the process of building precision to re-define it clears up some of the confusion. In particular, it seems like there's some implicit theory-of-mind stuff going on in the post and in some of the comments. I'm interested if you think the concept of 'deception' in this post only holds when there is implicit theory-of-mind going on, or otherwise.
As a thought experiment for a non-theory-of-mind example, let's say the daemon doesn't really understand why it gets a high reward for projecting the image of teapot (and then also doing some tactile projection somehow later) but it thinks that this is a good way to get high reward / meet its goals / etc. It doesn't realize (or at least not in an obviously accessible way, "know") that there is another agent/observer who is updating their models as a result of this. Possibly, if it did know this, it would not project the teapot, because it has another component of its reward function of "don't project false stimulus to other observing agents".
In this thought experiment, is the daemon 'deceiving' the observer? In general, is it possible to deceive someone who you don't realize is there? (Perhaps they're hiding behind a screen, and to you it just looks like you're projecting a teapot to an empty audience)
Aside: I think there's some interesting alignment problems here, but a bunch of our use of language around the concepts of deception hasn't updated to a world where we're talking about AI agents.
Copying some brief thoughts on what I think about working on automated theorem proving relating to working on aligned AGI:
- I think a pure-mathematical theorem prover is more likely to be beneficial and less likely to be catastrophic than STEM-AI / PASTA
- I think it's correspondingly going to be less useful
- I'm optimistic that it could be used to upgrade formal software verification and cryptographic algorithm verification
- With this, i think you can tell a story about how development in better formal theorem provers can help make information security a "defense wins" world -- where information security and privacy are a globally strong default
- There are some scenarios (e.g. ANI surveillance of AGI development) where this makes things worse, I think in expectation it makes things better
- There are some ways this could be developed where it ends up accelerating AGI research significantly (i.e. research done to further theorem proving ends up unlocking key breakthroughs to AGI) but I think this is unlikely
- One of the reasons I think this is unlikely is that current theorem proving environments are much closer to "AlphaGo on steriods" than "read and understand all mathematics papers ever written"
- I think if we move towards the latter, then I'm less differentially-optimistic about theorem proving as a direction of beneficial AI research (and it goes back to the general background level of AGI research more broadly)
FWIW I think this is basically right in pointing out that there's a bunch of errors in reasoning when people claim a large deep neural network "knows" something or that it "doesn't know" something.
I think this exhibits another issue, though, by strongly changing the contextual prefix, you've confounded it in a bunch of ways that are worth explicitly pointing out:
- Longer contexts use more compute to generate the same size answer, since they they attend over more tokens of input (and it's reasonable to think that in some cases that more compute -> better answer)
- Few-shot examples are a very compact way of expressing a structure or controlling the model to give a certain output -- but are very different than seeing if the model can implicitly understand context (or lack thereof). I liked the original question because it seemed to be pointed at this comparison, in a way that your few-shot example lacks.
- There is an invisible first token sometimes (an 'end-of-text' token) which indicates whether the given context represents the beginning of a new whole document. If this token is not present, then it's very possible (in fact very probable) that the context is in the middle of a document somewhere, but the model doesn't have access to the first part of the document. This leads to something more like "what document am I in the middle of, where the text just before this is <context>". Explicitly prefixing end-of-text signals that there is no additional document that the model needs to guess or account for.
- My basic point here is that your prompt implies a much different 'prior document' distribution than the original posts.
In general I'm pretty appreciative of efforts to help us get more clear understanding of neural networks, but often that doesn't cleanly fit into "the model knows X" or "the model doesn't know X".
Quite a lot of scams involve money that is fake. This seems like another reasonable conclusion.
Like, every time I simulate myself in this sort of experience, almost all of the prior is dominated by "you're lying".
I have spent an unreasonable (and yet unsuccessful) amount of time trying to sketch out how to present omega-like simulations to my friends.
Giving Newcomb's Problem to Infosec Nerds
Newcomb-like problems are pretty common thought experiments here, but I haven't seen a bunch of my favorite reactions I've got when discussing it in person with people. Here's a disorganized collection:
- I don't believe you can simulate me ("seems reasonable, what would convince you?") -- <describes an elaborate series of expensive to simulate experiments>. This never ended in them picking one box or two, just designing ever more elaborate and hard to simulate scenarios involving things like predicting the output of cryptographically secure hashings of random numbers from chaotic sources / quantum sources.
- Fuck you for simulating me. This is one of my favorites, where upon realizing that the person must consider the possibility that they are currently in an omega simulation, immediately do everything they can to be expensive and difficult to simulate. Again, this didn't result in picking one box or two, but I really enjoyed the "Spit in the face of God" energy.
- Don't play mind games with carnies. Excepting the whole "omniscience" thing, omega coming up to you to offer you a deal with money has very "street hustler scammer" energy. A good prior for successfully not getting conned is to stick to simple, strong priors, and don't update too strongly based on information presented. This person two-boxed, but this seems reasonable in the fast-response of "people who offer me deals on the street are trying to scam me".
There's probably some others I'm forgetting, but I did enjoy these most I think.
Adding a comment instead of another top-level post saying basically the same thing. Add my thoughts, on things I liked about this plan:
It's centered on people. A lot of rationality is thinking and deciding and weighing and valuing possible actions. Another frame that is occasionally good (for me at least) is "How would <my hero> act?" -- and this can help guide my actions. It's nice to have a human or historical action to think about instead of just a vague virtue or principle.
It encourages looking through history for events of positive impact. Many of us wish to impact the future (potentially the long future) for the better. It's nice to have examples of people in the past that made an impact. I think it helps me think about my possible impact and the impact of the people around me.
It marks a time on a calendar. Maybe my sense of time was warped by covid, but also I think I've missed the regular holidays that a religious life spaces throughout the year. It's also nice to coordinate on things, even if the coordination is small and just a few friends.
I want to start a list of people I might consider for this, but keeping it to myself for now!