Posts
Comments
I see that reading comprehension was an issue for you, since it seems that you stopped reading my post halfway through. Funny how a similar thing occurred on my last post too. It's almost like you think that the rules don't apply to you, since everyone else is required to read every single word in your posts with meticulous accuracy, whereas you're free to pick & choose at your whim.
i.e. splitting hairs and swirling words around to create a perpetual motte-and-bailey fog that lets him endlessly nitpick and retreat and say contradictory things at different times using the same words, and pretending to a sort of principle/coherence/consistency that he does not actually evince.
Yeah, almost like splitting hairs around whether making the public statement "I now categorize Said as a liar" is meaningfully different than "Said is a liar".
Or admonishing someone for taking a potshot at you when they said
However, I suspect that Duncan won't like this idea, because he wants to maintain a motte-and-bailey where his posts are half-baked when someone criticizes them but fully-baked when it's time to apportion status.
...while acting as though somehow that would have been less offensive if they had only added "I suspect" to the latter half of that sentence as well. Raise your hand if you think that "I suspect that you won't like this idea, because I suspect that you have the emotional maturity of a child" is less offensive because it now represents an unambiguously true statement of an opinion rather than being misconstrued as a fact. A reasonable person would say "No, that's obviously intended to be an insult" -- almost as though there can be meaning beyond just the words as written.
The problem is that if we believe in your philosophy of constantly looking for the utmost literal interpretation of the written word, you're tricking us into playing a meta-gamed, rules-lawyered, "Sovereign citizen"-esque debate instead of, what's the word -- oh, right, Steelmanning. Assuming charity from the other side. Seeking to find common ground.
For example, I can point out that Said clearly used the word "or" in their statement. Since reading comprehension seems to be an issue for a "median high-karma LWer" like yourself, I'll bold it for you.
Said: Well, I think that “criticism”, in a context like this topic of discussion, certainly includes something like “pointing to a flaw or lacuna, or suggesting an important or even necessary avenue for improvement”.
Is it therefore consistent for "asking for examples" to be contained by that set, while likewise not being pointing to a flaw? Yes, because if we say that a thing is contained by a set of "A or B", it could be "A", or it could be "B".
Now that we've done your useless exercise of playing with words, what have we achieved? Absolutely nothing, which is why games like these aren't tolerated in real workplaces, since this is a waste of everyone's time.
You are behaving in a seriously insufferable way right now.
Sorry, I meant -- "I think that you are behaving in what feels like to me a seriously insufferable way right now, where by insufferable I mean having or showing unbearable arrogance or conceit
".
Yes, I have read your posts.
I note that in none of them did you take any part of the responsibility for escalating the disagreement to its current level of toxicity.
You have instead pointed out Said's actions, and Said's behavior, and the moderators lack of action, and how people "skim social points off the top", etc.
@Duncan_Sabien I didn't actually upvote @clone of saturn's post, but when I read it, I found myself agreeing with it.
I've read a lot of your posts over the past few days because of this disagreement. My most charitable description of what I've read would be "spirited" and "passionate".
You strongly believe in a particular set of norms and want to teach everyone else. You welcome the feedback from your peers and excitedly embrace it, insofar as the dot product between a high-dimensional vector describing your norms and a similar vector describing the criticism is positive.
However, I've noticed that when someone actually disagrees with you -- and I mean disagreement in the sense of "I believe that this claim rests on incorrect priors and is therefore false." -- I have been shocked by the level of animosity you've shown in your writing.
Full disclosure: I originally messaged the moderators in private about your behavior, but I'm now writing this in public because in part because of your continued statements on this thread that you've done nothing wrong.
I think that your responses over the past few days have been needlessly escalatory in a way that Said's weren't. If we go with the Socrates metaphor, Said is sitting there asking "why" over and over, but you've let emotions rule and leapt for violence (metaphorically, although you then did then publish a post about killing Socrates, so YMMV).
There will always be people who don't communicate in a way that you'd prefer. It's important (for a strong, functioning team) to handle that gracefully. It looks to me that you've become so self-convinced that your communication style is "correct" that you've taken a war path towards the people who won't accept it -- Zack and Said.
In a company, this is problematic because some of the things that you're asking for are actually not possible for certain employees. Employees who have English as a second language, or who come from a different culture, or who may have autism, all might struggle with your requirements. As a concrete example, you wrote at length that saying "This is insane" is inflammatory in a way that "I think that this is insane" wouldn't be -- while I understand and appreciate the subtlety of that distinction, I also know that many people will view the difference between those statements as meaningless filler at best. I wrote some thoughts on that here: https://www.lesswrong.com/posts/9vjEavucqFnfSEvqk/on-aiming-for-convergence-on-truth?commentId=rGaKpCSkK6QnYBtD4
I believe that you are shutting down debates prematurely by casting your peers as antagonist towards you. In a corporate setting, as an engineer acquires more and more seniority, it becomes increasingly important for them to manage their emotions, because they're a role model for junior engineers.
I do think that @Said Achmiz can improve their behavior too. In particular, I think Said could recognize that sometimes their posts are met with hostility, and rather than debating this particular point, they could gracefully disengage from a specific conversation when they determine that someone does not appreciate their contributions.
However, I worry that you, Duncan, are setting an increasingly poor example. I don't know that I agree with the ability to ban users from posts. I think I lean more towards "ability to hide any posts from a user" as a feature, more than "prevent users from commenting". That is to say, I think if you're triggered by Said or Zack, then the site should offer you tools to hide those posts automatically. But I don't think that you should be able to prevent Said or Zack from commenting on your posts, or prevent other commentators from seeing that criticism. In part, I agree strongly (and upvoted strongly) with @Wei_Dai's point elsewhere in this thread that blocking posters means we can't tell the difference between "no one criticized this" and "people who would criticize it couldn't", unless they write their own post, as @Zack_M_Davis did.
Sometimes when you work at a large tech-focused company, you'll be pulled into a required-but-boring all-day HR meeting to discuss some asinine topic like "communication styles".
If you've had the misfortune fun of attending one of those meetings, you might remember that the topic wasn't about teaching a hypothetically "best" or "optimal" communication style. The goal was to teach employees how to recognize when you're speaking to someone with a different communication style, and then how to tailor your understanding of what they're saying with respect to them. For example, some people are more straightforward than others, so a piece of seemingly harsh criticism like "This won't work for XYZ reason." doesn't mean that they disrespect you -- they're just not the type of person who would phrase that feedback as "I think that maybe we've neglected to consider the impact of XYZ on the design."
I have read the many pages of debate on this current disagreement over the past few days. I have followed the many examples of linked posts that were intended to show bad behavior by one side or the other.
I think Zack and gjm have a good job at communicating with each other despite differences in their preferred communication styles, and in particular, I agree strongly with gjm's analysis:
I think this is the purpose of Duncan's proposed guideline 5. Don't engage in that sort of adversarial behaviour where you want to win while the other party loses; aim at truth in a way that, if you are both aiming at truth, will get you both there. And don't assume that the other party is being adversarial, unless you have to, because if you assume that then you'll almost certainly start doing the same yourself; starting out with a presumption of good faith will make actual good faith more likely.
And then with Zack's opinion:
That said, I don't think there's a unique solution for what the "right" norms are. Different rules might work better for different personality types, and run different risks of different failure modes (like nonsense aggressive status-fighting vs. nonsense passive-aggressive rules-lawyering). Compared to some people, I suppose I tend to be relatively comfortable with spaces where the rules err more on the side of "Punch, but be prepared to take a punch" rather than "Don't punch anyone"—but I realize that that's a fact about me, not a fact about the hidden Bayesian structure of reality. That's why, in "'Rationalist Discourse' Is Like 'Physicist Motors'", I made an analogy between discourse norms and motors or martial arts—there are principles governing what can work, but there's not going to be a unique motor, a one "correct" martial art.
I also agree with Zack when they said:
I'm unhappy with the absence of an audience-focused analogue of TEACH. In the following, I'll use TEACH to refer to making someone believe X if X is right; whether the learner is the audience or the interlocutor B isn't relevant to what I'm saying.
I seldom write comments with the intent of teaching a single person. My target audience is whoever is reading the posts, which is overwhelmingly going to be more than one person.
From Duncan, I agree with the following:
It is in fact usually the case that, when two people disagree, each one possesses some scrap of map that the other lacks; it's relatively rare that one person is just right about everything and thoroughly understands and can conclusively dismiss all of the other person's confusions or hesitations. If you are trying to see and understand what's actually true, you should generally be hungry for those scraps of map that other people possess, and interested in seeing, understanding, and copying over those bits which you were missing.
Almost all of my comments tend to focus on a specific disagreement that I have with the broader community. That disagreement is due to some prior that I hold, that is not commonly held here.
And from Said, I agree with this:
Examples?
This community is especially prone to large, overly-wordy armchair philosophy about this-or-that with almost no substantial evidence that can tie the philosophy back down to Earth. Sometimes that philosophy gets camouflaged in a layer of pseudo-math; equations, lemmas, writing as if the post is demonstrating a concrete mathematical proof. To that end, focusing the community on providing examples is a valuable, useful piece of constructive feedback. I strongly disagree that this is an unfair burden on authors.
EDIT: I forgot to write an actual conclusion. Maybe "don't expect everyone to communicate in the same way, even if we assume that all interested parties care about the truth"?
It seems to me that humans are more coherent and consequentialist than other animals. Humans are not perfectly coherent, but the direction is towards more coherence.
This isn't a universally held view. Someone wrote a fairly compelling argument against it here: https://sohl-dickstein.github.io/2023/03/09/coherence.html
We don't do any of these things for diffusion models that output images, and yet these diffusion models manage to be much smaller than models that output words, while maintaining an even higher level of output quality. What is it about words that makes the task different?
I'm not sure that "even higher level of output quality" is actually true, but I recognize that it can be difficult to judge when an image generation model has succeeded. In particular, I think current image models are fairly bad at specifics in much the same way as early language models.
But I think the real problem is that we seem to still be stuck on "words". When I ask GPT-4 a logic question, and it produces a grammatically correct sentence that answers the logic puzzle correctly, only part of that is related to "words" -- the other part is a nebulous blob of reasoning.
I went all the way back to GPT-1 (117 million parameters) and tested next word prediction -- specifically, I gave a bunch of prompts, and I looked for only if the very next word was what I would have expected. I think it's incredibly good at that! Probably better than most humans.
Or are you suggesting that image generators could also be greatly improved by training minimal models, and then embedding those models within larger networks?
No, because this is already how image generators work. That's what I said in my first post when I noted the architectural differences between image generators and language models. An image generator, as a system, consists of multiple models. There is a text -> image space, and then an image space -> image. The text -> image space encoder is generally trained first, then it's normally frozen during the training of the image decoder.[1] Meanwhile, the image decoder is trained on a straightforward task: "given this image, predict the noise that was added". In the actual system, that decoder is put into a loop to generate the final result. I'm requoting the relevant section of my first post below:
The reason why I'm discussing the network in the language of instructions, stack space, and loops is because I disagree with a blanket statement like "scale is all you need". I think it's obvious that scaling the neural network is a patch on the first two constraints, and scaling the training data is a patch on the third constraint.
This is also why I think that point #3 is relevant. If GPT-3 does so well because it's using the sea of parameters for unrolled loops, then something like Stable Diffusion at 1/200th the size probably makes sense.
- ^
Refer to figure 2 in https://cdn.openai.com/papers/dall-e-2.pdf. Or read this:
The trick here is that they decoupled the encoding from training the diffusion model. That way, the autoencoder can be trained to get the best image representation and then downstream several diffusion models can be trained on the so-called latent representation
This is the idea that I'm saying could be applied to language models, or rather, to a thing that we want to demonstrate "general intelligence" in the form of reasoning / problem solving / Q&A / planning / etc. First train a LLM, then train a larger system with the LLM as a component within it.
Yes, it's my understanding that OpenAI did this for GPT-4. It's discussed in the system card PDF. They used early versions of GPT-4 to generate synthetic test data and also as an evaluator of GPT-4 responses.
First, when we say "language model" and then we talk about the capabilities of that model for "standard question answering and factual recall tasks", I worry that we've accidentally moved the goal posts on what a "language model" is.
Originally, a language model was a stochastic parrot. They were developed to answer questions like "given these words, what comes next?" or "given this sentence, with this unreadable word, what is the most likely candidate?" or "what are the most common words?"[1] It was not a problem that required deep learning.
Then, we applied deep learning to it, because the path of history so far has been to take straightforward algorithms, replace them with a neural network, and see what happens. From that, we got ... stochastic parrots! Randomizing the data makes perfect sense for that.
Then, we scaled it. And we scaled it more. And we scaled it more.
And now we've arrived at a thing we keep calling a "language model" due to history, but it isn't a stochastic parrot anymore.
Second, I'm not saying "don't randomize data", I'm saying "use a tiered approach to training". We would use all of the same techniques: randomization, masking, adversarial splits, etc. What we would not do is throw all of our data and all of our parameters into a single, monolithic model and expect that would be efficient.[2] Instead, we'd first train a "minimal" LLM, then we'd use that LLM as a component within a larger NN, and we'd train that combined system (LLM + NN) on all of the test cases we care about for abstract reasoning / problem solving / planning / etc. It's that combined system that I think would end up being vastly more efficient than current language models, because I suspect the majority of language model parameters are being used for embedding trivia that doesn't contribute to the core capabilities we recognize as "general intelligence".
- ^
This wasn't for auto-complete, it was generally for things like automatic text transcription from images, audio, or videos. Spam detection was another use-case.
- ^
Recall that I'm trying to offer a hypothesis for why a system like GPT-3.5 takes so much training and has so many parameters and it still isn't "competent" in all of the ways that a human is competent. I think "it is being trained in an inefficient way" is a reasonable answer to that question.
I suspect it is a combination of #3 and #5.
Regarding #5 first, I personally think that language models are being trained wrong. We'll get OoM improvements when we stop randomizing the examples we show to models during training, and instead provide examples in a structured curriculum.
This isn't a new thought, e.g. https://arxiv.org/abs/2101.10382
To be clear, I'm not saying that we must present easy examples first and then harder examples later. While that is what has been studied in the literature, I think we'd actually get better behavior by trying to order examples on a spectrum of "generalizes well" to "very specific, does not generalize" and then training in that order. Sometimes this might be equivalent to "easy examples first", but that isn't necessarily true.
I recognize that the definitions of "easy" and "generalizes" are nebulous, so I'm going to try and explain the reasoning that led me here.
Consider the architecture of transformers and feed-forward neural networks (specifically not recurrent neural networks). We're given some input, and we produce some output. In a model like GPT, we're auto-regressive, so as we produce our outputs, those outputs become part of the input during the next step. Each step is fundamentally a function .
Given some input, the total output can be thought as:
def reply_to(input):
output = ""
while True:
token = predict_next(input + output)
if token == STOP:
break
output += token
return output
We'd like to know exactly what `predict_next` is doing, but unfortunately, the programmer who wrote it seems to have done their implementation entirely in matrix math and they didn't include any comments. In other words, it's deeply cursed and not terribly different from the output of Simulink's code generator.
def predict_next(input):
# ... matrix math
return output
Let's try to think about the capabilities and constraints on this function.
- There is no unbounded `loop` construct. The best we can do is approximate loops, e.g. by supporting an unrolled loop up to some bounded number of iterations. What determines the bounds? Probably the depth of the network?
- If the programmer were sufficiently deranged, they could implement `predict_next` in such a way that if they've hit the bottom of their unrolled loop, they could rely on the fact that `predict_next` will be called again, and continue their previous calculations during the next call. What would be the limitations on this? Probably the size of each hidden layer. If you wanted to figure out if this is happening, you'd want to look for prompts where the network can answer the prompt correctly if it is allowed to generate text before the answer (e.g. step-by-step explanations) but is unable to do so if asked to provide the answer without any associated explanations.
- How many total "instructions" can fit into this function? The size of the network seems like a decent guess. Unfortunately, the network conflates instructions and data, and the network must use all parameters available to it. This leads to trivial solutions where the network just over-fits to the data (analogous to baking in a lookup table on the stack). It's not unsurprising that throwing OoM more data at a fixed size NN results in better generalization. Once you're unable to cheat with over-fitting you must learn algorithms that work more efficiently.
The reason why I'm discussing the network in the language of instructions, stack space, and loops is because I disagree with a blanket statement like "scale is all you need". I think it's obvious that scaling the neural network is a patch on the first two constraints, and scaling the training data is a patch on the third constraint.
This is also why I think that point #3 is relevant. If GPT-3 does so well because it's using the sea of parameters for unrolled loops, then something like Stable Diffusion at 1/200th the size probably makes sense.
To tie this back to point #5:
- We start with a giant corpus of data. On the order of "all written content available in digital form". We might generate additional data in an automated fashion, or digitize books, or caption videos.
- We divide it into training data and test data.
- We train the network on random examples from the training data, and then verify on random examples from the test data. For simplicity, I'm glossing over various training techniques like masking data or connections between nodes.
- Then we fine-tune it, e.g with Q&A examples.
- And then generally we deploy it with some prompt engineering, e.g. prefixing queries with past transcript history, to fake a conversation.
At the end of this process, what do we have?
I want to emphasize that I do not think it is a "stochastic parrot". I think it is very obvious that the final system has internalized actual algorithms (or at least, pseudo-algorithms due to the limitation on loops) for various tasks, given the fact that the size of the data set is significantly larger than the size of the model. I think people who are surprised by the capabilities of these systems continue to assume it is "just" modeling likelihoods, when there was no actual requirement on that.
I also suspect we've wasted an enormous quantity of our parameters on embedding knowledge that does not directly contribute to system's capabilities.
My hypothesis for how to fix this is vaguely similar to the idea of "maximizing divergence" discussed here https://ljvmiranda921.github.io/notebook/2022/08/02/splits/.
I think we could train a LLM on a minimal corpus to "teach" a language[1] and then place that LLM inside of a larger system that we train to minimize loss on examples teaching logic, mathematics, and other components of reasoning. That larger system would distinguish between the weights for the algorithms it learns and the weights representing embedded knowledge. It would also have the capability to loop during the generation of an output. For comparison, think of the experiments being done with hooking up GPT-4 to a vector database, but now do that inside of the architecture instead of as a hack on top of the text prompts.
I think an architecture that cleanly separates embedded knowledge ("facts", "beliefs", "shards", etc) from the algorithms ("capabilities", "zero-shot learning") is core to designing a neural network that remains interpretable and alignable at scale.
If you read the previous paragraphs and think, "that sounds familiar", it's probably because I'm describing how we teach humans: first language, then reasoning, then specialization. A curriculum. We need language first because we want to be able to show examples, explain, and correct mistakes. Especially since we can automate content generation with existing LLMs to create the training corpus in these steps. Then we want to teach reasoning, starting with the most general forms of reasoning, and working into the most specific. Finally, we grade the system (not train!) on a corpus of specific knowledge-based activities. Think of this step as describing the rules of a made-up game, providing the current game state, and then asking for the optimal move. Except that for games, for poems, for math, for wood working, for engineering, etc. The whole point of general intelligence is that you can reason from first principles, so that's what we need to be grading the network on: minimizing loss with respect to arbitrarily many knowledge-based tasks that must be solved using the facts provided only during the test itself.
- ^
Is English the right language to teach? I think it would be funny if a constructed language actually found a use here.
I'm reminded of this thread from 2022: https://www.lesswrong.com/posts/27EznPncmCtnpSojH/link-post-on-deference-and-yudkowsky-s-ai-risk-estimates?commentId=SLjkYtCfddvH9j38T#SLjkYtCfddvH9j38T
I realize that my position might seem increasingly flippant, but I really think it is necessary to acknowledge that you've stated a core assumption as a fact.
Alignment doesn't run on some nega-math that can't be cast as an optimization problem.
I am not saying that the concept of "alignment" is some bizarre meta-physical idea that cannot be approximated by a computer because something something human souls etc, or some other nonsense.
However the assumption that "alignment is representable in math" directly implies "alignment is representable as an optimization problem" seems potentially false to me, and I'm not sure why you're certain it is true.
There exist systems that can be 1.) represented mathematically, 2.) perform computations, and 3.) do not correspond to some type of min/max optimization, e.g. various analog computers or cellular automaton.
I don't think it is ridiculous to suggest that what the human brain does is 1.) representable in math, 2.) in some type of way that we could actually understand and re-implement it on hardware / software systems, and 3.) but not as an optimization problem where there exists some reward function to maximize or some loss function to minimize.
I wasn't intending for a metaphor of "biomimicry" vs "modernist".
(Claim 1) Wings can't work in space because there's no air. The lack of air is a fundamental reason for why no wing design, no matter how clever it is, will ever solve space travel.
If TurnTrout is right, then the equivalent statement is something like (Claim 2) "reward functions can't solve alignment because alignment isn't maximizing a mathematical function."
The difference between Claim 1 and Claim 2 is that we have a proof of Claim 1, and therefore don't bother debating it anymore, while with Claim 2 we only have an arbitrarily long list of examples for why reward functions can be gamed, exploited, or otherwise fail in spectacular ways, but no general proof yet for why reward functions will never work, so we keep arguing about a Sufficiently Smart Reward Function That Definitely Won't Blow up as if that is a thing that can be found if we try hard enough.
As of right now, I view "shard theory" sort of like a high-level discussion of chemical propulsion without the designs for a rocket or a gun. I see the novelty of it, but I don't understand how you would build a device that can use it. Until someone can propose actual designs for hardware or software that would implement "shard theory" concepts without just becoming an obfuscated reward function prone to the same failure modes as everything else, it's not incredibly useful to me. However, I think it's worth engaging with the idea because if correct then other research directions might be a dead-end.
Does that help explain what I was trying to do with the metaphor?
To some extent, I think it's easy to pooh-pooh finding a flapping wing design (not maximally flappy, merely way better than the best birds) when you're not proposing a specific design for building a flying machine that can go to space. Not in the tone of "how dare you not talk about specifics," but more like "I bet this chemical propulsion direction would have to look more like birds when you get down to brass tacks."
(1) The first thing I did when approaching this was think about how the message is actually transmitted. Things like the preamble at the start of the transmission to synchronize clocks, the headers for source & destination, or the parity bits after each byte, or even things like using an inversed parity on the header so that it is possible to distinguish a true header from bytes within a message that look like a header, and even optional checksum calculations.
(2) I then thought about how I would actually represent the data so it wasn't just traditional 8-bit bytes -- I created encoders & decoders for 36/24/12/6 bit unsigned and signed ints, and 30 / 60 bit non-traditional floating point, etc.
Finally, I created a mock telemetry stream that consisted of a bunch of time-series data from many different sensors, with all of the sensor values packed into a single frame with all of the data types from (2), and repeatedly transmitted that frame over the varying time series, using (1), until I had >1 MB.
And then I didn't submit that, and instead swapped to a single message using the transmission protocol that I designed first, and shoved an image into that message instead of the telemetry stream.
- To avoid the flaw where the message is "just" 1-byte RGB, I viewed each pixel in the filter as being measured by a 24-bit ADC. That way someone decoding it has to consider byte-order when forming the 24-bit values.
- Then, I added only a few LSB of noise because I was thinking about the type of noise you see on ADC channels prior to more extensive filtering. I consider it a bug that I only added noise in some interval
[0, +N]
, when I should have allowed the noise to be positive or negative. I am less convinced that the uniform distribution is incorrect. In my experience, ADC noise is almost always uniform (and only present in a few LSB), unless there's a problem with the HW design, in which case you'll get dramatic non-uniform "spikes". I was assuming that the alien HW is not so poorly designed that they are railing their ADC channels with noise of that magnitude. - I wanted the color data to be more complicated than just RGB, so I used a Bayer filter, that way people decoding it would need to demosiac the color channels. This further increased the size of the image.
- The original, full resolution image produced a file much larger than 1 MB when it was put through the above process (3 8-bit RGB -> 4 24-bit Bayer), so I cut the resolution on the source image until the output was more reasonably sized. I wasn't thinking about how that would impact the image analysis, because I was still thinking about the data types (byte order, number of bits, bit ordering) more so than the actual image content.
- "Was the source image actually a JPEG?" I didn't check for JPEG artifacts at all, or analyze the image beyond trying to find a nice picture of bismuth with the full color of the rainbow present so that all of the color channels would be used. I just now did a search for "bismuth png" on Google, got a few hits, opened one, and it was actually a JPG. I remember scrolling through a bunch of Google results before I found an image that I liked, and then I just remember pulling & saving it as a BMP. Even if I had downloaded a source PNG as I intended, I definitely didn't check that the PNG itself wasn't just a resaved JPEG.
My understanding of faul_sname's claim is that for the purpose of this challenge we should treat the alien sensor data output as an original piece of data.
In reality, yes, there is a source image that was used to create the raw data that was then encoded and transmitted. But in the context of the fiction, the raw data is supposed to represent the output of the alien sensor, and the claim is that the decompressor + payload is less than the size of just an ad-hoc gzipping of the output by itself. It's that latter part of the claim that I'm skeptical towards. There is so much noise in real sensors -- almost always the first part of any sensor processing pipeline is some type of smoothing, median filtering, or other type of noise reduction. If a solution for a decompressor involves saving space on encoding that noise by breaking a PRNG, it's not clear to me how that would apply to a world in which this data has no noise-less representation available. However, a technique of measuring & subtracting noise so that you can compress a representation that is more uniform and then applying the noise as a post-processing op during decoding is definitely doable.
Assuming that you use the payload of size 741809 bytes, and are able to write a decompressor + "transmitter" for that in the remaining ~400 KB (which should be possible, given that 7z is ~450 KB, zip is 349 KB, other compressors are in similar size ranges, and you'd be saving space since you just need to the decoder portion of the code), how would we rate that against the claims?
- It would be possible for me, given some time to examine the data, create a decompressor and a payload such that running the decompressor on the payload yields the original file, and the decompressor program + the payload have a total size of less than the original gzipped file
- The decompressor would legibly contain a substantial amount of information about the structure of the data.
(1) seems obviously met, but (2) is less clear to me. Going back to the original claim, faul_sname said 'we would see that the winning programs would look more like "generate a model and use that model and a similar rendering process to what was used to original file, plus an error correction table" and less like a general-purpose compressor'.
So far though, this solution does use a general purpose compressor. My understanding of (2) is that I was supposed to be looking for solutions like "create a 3D model of the surface of the object being detected and then run lighting calculations to reproduce the scene that the camera is measuring", etc. Other posts from faul_sname in the thread, e.g. here seem to indicate that was their thinking as well, since they suggested using ray tracing as a method to describe the data in a more compressed manner.
What are your thoughts?
Regarding the sensor data itself
I alluded to this in my post here, but I was waffling and backpedaling a lot on what would be "fair" in this challenge. I gave a bunch of examples in the thread of what would make a binary file difficult to decode -- e.g. non-uniform channel lengths, an irregular data structure, multiple types of sensor data interwoven into the same file, and then did basically none of that, because I kept feeling like the file was unapproachable. Anything that was a >1 MB of binary data but not a 2D image (or series of images) seemed impossible. For example, the first thing I suggested in the other thread was a stream of telemetry from some alien system.
I thought this file would strike a good balance, but I now see that I made a crucial mistake: I didn't expect that you'd be able to view it with the wrong number of bits per byte (7 instead of 6) and then skip almost every byte and still find a discernible image in the grayscale data. Once you can "see" what the image is supposed to be, the hard part is done.
I was assuming that more work would be needed for understanding the transmission itself (e.g. deducing the parity bits by looking at the bit patterns), and then only after that would it be possible to look at the raw data by itself.
I had a similar issue when I was playing with LIDAR data as an alternative to a 2D image. I found that a LIDAR point cloud is eerily similar enough to image data that you can stumble upon a depth map representation of the data almost by accident.
I have posted my file here https://www.lesswrong.com/posts/BMDfYGWcsjAKzNXGz/eavesdropping-on-aliens-a-data-decoding-challenge.
I've posted it here https://www.lesswrong.com/posts/BMDfYGWcsjAKzNXGz/eavesdropping-on-aliens-a-data-decoding-challenge.
Which question are we trying to answer?
- Is it possible to decode a file that was deliberately constructed to be decoded, without a priori knowledge? This is vaguely what That Alien Message is about, at least in the first part of the post where aliens are sending a message to humanity.
- Is it possible to decode a file that has an arbitrary binary schema, without a priori knowledge? This is the discussion point that I've been arguing over with regard to stuff like decoding CAMERA raw formats, or sensor data from a hardware/software system. This is also the area where I disagree with That Alien Message -- I don't think that one-shot examples allow robust generalization.
I don't think (1) is a particularly interesting question, because last weekend I convinced myself that the answer is yes, you can transfer data in a way that it can be decoded, with very few assumptions on the part of the receiver. I do have a file I created for this purpose. If you want, I'll send you it.
I started creating a file for (2), but I'm not really sure how to gauge what is "fair" vs "deliberately obfuscated" in terms of encoding. I am conflicted. Even if I stick to encoding techniques I've seen in the real world, I feel like I can make choices on this file encoding that make the likelihood of others decoding it very low. That's exactly what we're arguing about on (2). However, I don't think it will be particularly interesting or fun for people trying to decode it. Maybe that's ok?
What are your thoughts?
It depends on what you mean by "didn't work". The study described is published in a paper only 16 pages long. We can just read it: http://web.mit.edu/curhan/www/docs/Articles/biases/67_J_Personality_and_Social_Psychology_366,_1994.pdf
First, consider the question of, "are these predictions totally useless?" This is an important question because I stand by my claim that the answer of "never" is actually totally useless due to how trivial it is.
Despite the optimistic bias, respondents' best estimates were by no means devoid of information: The predicted completion times were highly correlated with actual completion times (r = .77, p < .001). Compared with others in the sample, respondents who predicted that they would take more time to finish actually did take more time. Predictions can be informative even in the presence of a marked prediction bias.
...
Respondents' optimistic and pessimistic predictions were both strongly correlated with their actual completion times (rs = .73 and .72, respectively; ps < .01).
Yep. Matches my experience.
We know that only 11% of students met their optimistic targets, and only 30% of students met their "best guess" targets. What about the pessimistic target? It turns out, 50% of the students did finish by that target. That's not just a quirk, because it's actually related to the distribution itself.
However, the distribution of difference scores from the best-guess predictions were markedly skewed, with a long tail on the optimistic side of zero, a cluster of scores within 5 or 10 days of zero, and virtually no scores on the pessimistic side of zero. In contrast, the differences from the worst-case predictions were noticeably more symmetric around zero, with the number of markedly pessimistic predictions balancing the number of extremely
optimistic predictions.
In other words, asking people for a best guess or an optimistic prediction results in a biased prediction that is almost always earlier than a real delivery date. On the other hand, while the pessimistic question is not more accurate (it has the same absolute error margins), it is unbiased. The reality is that the study says that people asked for a pessimistic question were equally likely to over-estimate their deadline as they were to under-estimate it. If you don't think a question that gives you a distribution centered on the right answer is useful, I'm not sure what to tell you.
The paper actually did a number of experiments. That was just the first.
In the third experiment, the study tried to understand what people are thinking about when estimating.
Proportionally more responses concerned future scenarios (M = .74) than relevant past experiences (M =.07), r(66) = 13.80, p < .001. Furthermore, a much higher proportion of subjects' thoughts involved planning for a project and imagining its likely progress (M =.71) rather than considering potential impediments (M = .03), r(66) = 18.03, p < .001.
This seems relevant considering that the idea of premortems or "worst case" questioning is to elicit impediments, and the project managers / engineering leads doing that questioning are intending to hear about impediments and will continue their questioning until they've been satisfied that the group is actually discussing that.
In the fourth experiment, the study tries to understand why it is that people don't think about their past experiences. They discovered that just prompting people to consider past experiences was insufficient, they actually needed additional prompting to make their past experience "relevant" to their current task.
Subsequent comparisons revealed that subjects in the recall-relevant condition predicted they would finish the assignment later than subjects in either the recall condition, t(79) = 1.99, p < .05, or the control condition, f(80) = 2.14, p < .04, which did not differ significantly from each other, t(& 1) < 1
...
Further analyses were performed on the difference between subjects' predicted and actual completion times. Subjects underestimated their completion times significantly in the control (M = -1.3 days), r(40) = 3.03, p < .01, and recall conditions (M = -1.0 day), t(41) = 2.10, p < .05, but not in the recall-relevant condition (M = -0.1 days), ((39) < i. Moreover, a higher percentage of subjects finished the assignments in the predicted time in the recall-relevant condition (60.0%) than in the recall and control conditions (38.1% and 29.3%, respectively), x2G, N = 123) = 7.63, p < .01. The latter two conditions did not differ significantly from each other.
...
The absence of an effect in the recall condition is rather remarkable. In this condition, subjects first described their past performance with projects similar to the computer assignment and acknowledged that they typically finish only 1 day before
deadlines. Following a suggestion to "keep in mind previous experiences with assignments," they then predicted when they would finish the computer assignment. Despite this seemingly powerful manipulation, subjects continued to make overly optimistic forecasts. Apparently, subjects were able to acknowledge their past experiences but disassociate those episodes from their present predictions.
In contrast, the impact of the recall-relevant procedure was sufficiently robust to eliminate the optimistic bias in both deadline conditions
How does this compare to the first experiment?
Interestingly, although the completion estimates were less biased in the recall-relevant condition than in the other conditions, they were not more strongly correlated with actual completion times, nor was the absolute prediction error any smaller. The optimistic bias was eliminated in the recall-relevant condition because subjects' predictions were as likely to be too long as they were to be too short. The effects of this manipulation mirror those obtained with the instruction to provide pessimistic predictions in the first study: When students predicted the completion date for their honor's thesis on the assumption that "everything went as poorly as it possibly could" they produced unbiased but no more accurate predictions than when they made their "best guesses."
It's common in engineering to perform group estimates. Does the study look at that? Yep, the fifth and last experiment asks individuals to estimate the performance of others.
As hypothesized, observers seemed more attuned to the actors' base rates than did the actors themselves. Observers spontaneously used the past as a basis for predicting actors' task completion times and produced estimates that were later than both the actors' estimates and their completion times.
So observers are more pessimistic. Actually, observers are so pessimistic that you have to average it with the optimistic estimates to get an unbiased estimate.
One of the most consistent findings throughout our investigation was that manipulations that reduced the directional (optimistic) bias in completion estimates were ineffective in in-
creasing absolute accuracy. This implies that our manipulations did not give subjects any greater insight into the particular predictions they were making, nor did they cause all subjects to become more pessimistic (see Footnote 2), but instead caused enough subjects to become overly pessimistic to counterbalance the subjects who remained overly optimistic. It remains for future research to identify those factors that lead people to make
more accurate, as well as unbiased, predictions. In the real world, absolute accuracy is sometimes not as important as (a) the proportion of times that the task is completed by the "best-guess" date and (b) the proportion of dramatically optimistic, and therefore memorable, prediction failures. By both of these criteria, factors that decrease the optimistic bias "improve" the quality of intuitive prediction.
At the end of the day, there are certain things that are known about scheduling / prediction.
- In general, individuals are as wrong as they are right for any given estimate.
- In general, people are overly optimistic.
- But, estimates generally correlate well with actual duration -- if an individual thinks something is longer in estimate than another task, it most likely is! This is why in SW sometimes estimation is not in units of time at all, but in a concept called "points".
- The larger and more nebulously scoped the task, the worse any estimates will be in absolute error.
- The length of a time a task can take follows a distribution with a very long right tail -- a task that takes way longer than expected can take an arbitrary amount of time, but the fastest time to complete a task is limited.
- The best way to actually schedule or predict a project is to break it down into as many small component tasks as possible, identify dependencies between those tasks, and produce most likely, optimistic, and pessimistic estimates for each task, and then run a simulation for chain of dependencies to see what the expected project completion looks like. Use a Gantt chart. This is a boring answer because it's the "learn project management" answer, and people will hate on it because
gesture vaguely to all of the projects that overrun their schedule
. There are many interesting reasons for why that happens and why I don't think it's a massive failure of rationality, but I'm not sure this comment is a good place to go into detail on that. The quick answer is that comical overrun of a schedule has less to do with an inability to create correct schedules from an engineering / evidence-based perspective, and much more to do with a bureaucratic or organizational refusal to accept an evidence-based schedule when a totally false but politically palatable "optimistic" schedule is preferred.
Right. I think I agree with everything you wrote here, but here it is again in my own words:
In communicating with people, the goal isn't to ask a hypothetically "best" question and wonder why people don't understand or don't respond in the "correct" way. The goal is to be understood and to share information and acquire consensus or agree on some negotiation or otherwise accomplish some task.
This means that in real communication with real people, you often need to ask different questions to different people to arrive at the same information, or phrase some statement differently for it to be understood. There shouldn't be any surprise or paradox here. When I am discussing an engineering problem with engineers, I phrase it in the terminology that engineers will understand. When I need to communicate that same problem to upper management, I do not use the same terminology that I use with my engineers.
Likewise, there's a difference when I'm communicating with some engineering intern or new grad right out of college, vs a senior engineer with a decade of experience. I tailor my speech for my audience.
In particular, if I asked this question to Kenoubi ("what's the worst case for how long this thesis could take you?"), and Kenoubi replied "It never finishes", then I would immediately follow up with the question, "Ok, considering cases when it does finish, what's the worst-case look like?" And if that got the reply "the day before it is required to be due", I would then start poking at "What would would cause that to occur?".
The reason why I start with the first question is because it works for, I don't know, 95% of people I've ever interacted with in my life? In my mind, it's rational to start with a question that almost always elicits the information I care about, even if there's some small subset of the population that will force me to choose my words as if they're being interpreted by a Monkey's paw.
Isn't this identical to the proof for why there's no general algorithm for solving the Halting Problem?
The Halting Problem asks for an algorithm A(S, I)
that when given the source code S
and input I
for another program will report whether S(I)
halts (vs run forever).
There is a proof that says A
does not exist. There is no general algorithm for determining whether an arbitrary program will halt. "General" and "arbitrary" are important keywords because it's trivial to consider specific algorithms and specific programs and say, yes, we can determine that this specific program will halt via this specific algorithm.
That proof of the Halting Problem (for a general algorithm and arbitrary programs!) works by defining a pathological program S
that inspects what the general algorithm A
would predict and then does the opposite.
What you're describing above seems almost word-for-word the same construction used for constructing the pathological program S
, except the algorithm A
for "will this program halt?" is replaced by the predictor "will this person one-box?".
I'm not sure that this necessarily matters for the thought experiment. For example, perhaps we can pretend that the predictor works on all strategies except the pathological case described here, and other strategies isomorphic to it.
If we look at the student answers, they were off by ~7 days, or about a 14% error from the actual completion time.
The only way I can interpret your post is that you're suggesting all of these students should have answered "never".
I'm not convinced that "never" just didn't occur to them because they were insufficiently motivated to give a correct answer.
How far off is "never" from the true answer of 55.5 days?
It's about infinitely far off. It is an infinitely wrong answer. Even if a project ran 1000% over every worst-case pessimistic schedule, any finite prediction was still infinitely closer than "never".
It's a quirk of rationalist culture (and a few others — I've seen this from physicists too) to take the words literally and propose that "infinitely long" is a plausible answer, and be baffled as to how anyone could think otherwise.
That's because "infinitely long" is a trivial answer for any task that isn't literally impossible.[1] It provides 0 information and takes 0 computational effort. It might as well be the answer from a non-entity, like asking a brick wall how long the thesis could take to complete.

Question: How long can it take to do X?
Brick wall: Forever. Just go do not-X instead.
It is much more difficult to give an answer for how long a task can take assuming it gets done while anticipating and predicting failure modes that would cause the schedule to explode, and that same answer is actually useful since you can now take preemptive actions to avoid those failure modes -- which is the whole point of estimating and scheduling as a logical exercise.
The actual conversation that happens during planning is
A: "What's the worst case for this task?"
B: "6 months."
A: "Why?"
B: "We don't have enough supplies to get past 3 trial runs, so if any one of them is a failure, the lead time on new materials with our current vendor is 5 months."
A: "Can we source a new vendor?"
B: "No, but... <some other idea>
"
- ^
In cases when something is literally impossible, instead of saying "infinitely long", or "never", it's more useful to say "that task is not possible" and then explain why. Communication isn't about finding the "haha, gotcha" answer to a question when asked.
Is the concept of "murphyjitsu" supposed to be different than the common exercise known as a premortem in traditional project management? Or is this just the same idea, but rediscovered under a different name, exactly like how what this community calls a "double crux" is just the evaporating cloud, which was first described in the 90s.
If you've heard of a postmortem or possibly even a retrospective, then it's easy to guess what a premortem is. I cannot say the same for "murphyjitsu".
I see that premortem is even referenced in the "further resources" section, so I'm confused why you'd describe it under a different name that cannot be researched easily outside of this site, where there is tons of literature and examples of how to do premortems correctly.
The core problem remains computational complexity.
Statements like "does this image look reasonable" or saying "you pay attention to regularities in the data", or "find the resolution by searching all possible resolutions" are all hiding high computational costs behind short English descriptions.
Let's consider the case of a 1280x720 pixel image.
That's the same as 921600 pixels.
How many bytes is that?
It depends. How many bytes per pixel?[1] In my post, I explained there could be 1-byte-per-pixel grayscale, or perhaps 3-bytes-per-pixel RGB using [0, 255] values for each color channel, or maybe 6-bytes-per-pixel with [0, 65535] values for each color channel, or maybe something like 4-bytes-per-pixel because we have 1-byte RGB channels and a 1-byte alpha channel.
Let's assume that a reasonable cutoff for how many bytes per pixel an encoding could be using is say 8 bytes per pixel, or a hypothetical 64-bit color depth.
How many ways can we divide this between channels?
If we assume 3 channels, it's 1953.
If we assume 4 channels, it's 39711.
Also if it turns out to be 5 channels, it's 595665.
This is a pretty fast growing function. The following is a plot.
Note that the red line is O(2^N)
and the black line barely visible at the bottom is O(N^2)
. N^2
is a notorious runtime complexity because it's right on the threshold of what is generally unacceptable performance.[2]
Let's hope that this file isn't actually a frame buffer from a graphics card with 32 bits per channel or a 128 bit per pixel / 16 byte per pixel.
Unfortunately, we still need to repeat this calculation for all of the possibilities for how many bits per pixel this image could be. We need to add in the possibility that it is 63 bits per pixel, or 62 bits per pixel, or 61 bits per pixel.
In case anyone wants to claim this is unreasonable, it's not impossible to have image formats that have RGBA data, but only 1 bit associated with the alpha data for each pixel. [3]
And for each of these scenarios, we need to question how many channels of color data there are.
- 1? Grayscale.
- 2? Grayscale, with an alpha channel maybe?
- 3? RGB, probably, or something like HSV.
- 4? RGBA, or maybe it's the RGBG layout I described for a RAW encoding of a Bayer filter, or maybe it's CMYK for printing.
- 5? This is getting weird, but it's not impossible. We could be encoding additional metadata into each pixel, e.g. distance from the camera.
- 6? Actually, this question how how many channels there are is very important, given the fast growing function above.
- 7? This one question, if we don't know the right answer, is sufficient to make this algorithm pretty much impossible to run.
- 8? When we say we can try all of options, that's not actually possible.
- 9? What I think people mean is that we can use heuristics to pick the likely options first and try them, and then fall back to more esoteric options if the initial results don't make sense.
- 10? That's the difference between average run-time and worst case run-time.
- 11? The point that I am trying to make is that the worst case run-time for decoding an arbitrary binary file is pretty much unbounded, because there's a ridiculous amount of choice possible.
- 12? Some examples of "image" formats that have large numbers of channels per "pixel" are things like RADAR / LIDAR sensors, e.g. it's possible to have 5 channels per pixel for defining 3D coordinates (relative to the sensor), range, and intensity.
You actually ran into this problem yourself.
Similarly (though you'd likely do this first), you can tell the difference between RGB and RGBA. If you have (255, 0, 0, 255, 0, 0, 255, 0, 0, 255, 0, 0), this is probably 4 red pixels in RGB, and not a fully opaque red pixel, followed by a fully transparent green pixel, followed by a fully transparent blue pixel in RGBA. It could be 2 pixels that are mostly red and slightly green in 16 bit RGB, though. Not sure how you could piece that out.
Summing up all of the possibilities above is left as an exercise for the reader, and we'll call that sum K
.
Without loss of generality, let's say our image was encoded as 3 bytes per pixel divided between 3 RGB color channels of 1 byte each.
Our 1280x720 image is actually 2764800 bytes as a binary file.
But since we're decoding it from the other side, and we don't know it's 1280x720, when we're staring at this pile of 2764800 bytes, we need to first assume how many bytes per pixel it is, so that we can divide the total bytes by the bytes per pixel to calculate the number of pixels.
Then, we need to test each possible resolutions as you've suggested.
The number of possible resolutions is the same as the number of divisors of the number of pixels. The equation for providing an upper bound is exp(log(N)/log(log(N)))
[4], but the average number of divisors is approximately log(N)
.
Oops, no it isn't!
Files have headers! How large is the header? For a bitmap, it's anywhere between 26 and 138 bytes. The JPEG header is at least 2 bytes. PNG uses 8 bytes. GIF uses at least 14 bytes.
Now we need to make the following choices:
- Guess at how many bytes per pixel the data is.
- Guess at the length of the header. (maybe it's 0, there is no header!)
- Calculate the factorization of the remaining bytes N for the different possible resolutions.
- Hope that there isn't a footer, checksum, or any type of other metadata hanging out in the sea of bytes. This is common too!
Once we've made our choices above, then we multiply that by log(N)
for the number of resolutions to test, and then we'll apply the suggested metric. Remember that when considering the different pixel formats and ways the color channel data could be represented, the number was K
, and that's what we're multiplying by log(N)
.
In most non-random images, pixels near to each other are similar. In an MxN image, the pixel below is a[i+M], whereas in an NxM image, it's a[i+N]. If, across the whole image, the difference between a[i+M] is less than the difference between a[i+N], it's more likely an MxN image. I expect you could find the resolution by searching all possible resolutions from 1x<length> to <length>x1, and finding which minimizes average distance of "adjacent" pixels.
What you're describing here is actually similar to a common metric used in algorithms for automatically focusing cameras by calculating the contrast of an image, except for focusing you want to maximize contrast instead of minimize it.
The interesting problem with this metric is that it's basically a one-way function. For a given image, you can compute this metric. However, minimizing this metric is not the same as knowing that you've decoded the image correctly. It says you've found a decoding, which did minimize the metric. It does not mean that is the correct decoding.
A trivial proof:
- Consider an image and the reversal of that image along the horizontal axis.
- These have the same metric.
- So the same metric can yield two different images.
A slightly less trivial proof:
- For a given "image" of
N
bytes of image data, there are2^(N*8)
possible bit patterns. - Assuming the metric is calculated as an 8-byte IEEE 754 double, there are only
2^(8*8)
possible bit patterns. - When
N > 8
, there are more bit patterns than values allowed in a double, so multiple images need to map to the same metric.
The difference between our 2^(2764800*8)
image space and the 2^64
metric is, uhhh, 10^(10^6.8)
. Imagine 10^(10^6.8)
pigeons. What a mess.[5]
The metric cannot work as described. There will be various arbitrary interpretations of the data possible to minimize this metric, and almost all of those will result in images that are definitely not the image that was actually encoded, but did minimize the metric. There is no reliable way to do this because it isn't possible. When you have a pile of data, and you want to reverse meaning from it, there is not one "correct" message that you can divine from it.[6] See also: numerology, for an example that doesn't involve binary file encodings.
Even pretending that this metric did work, what's the time complexity of it? We have to check each pixel, so it's O(N)
. There's a constant factor for each pixel computation. How large is that constant? Let's pretend it's small and ignore it.
So now we've got K*O(N*log(N))
which is the time complexity of lots of useful algorithms, but we've got that awkward constant K
in the front. Remember that the constant K
reflects the number of choices for different bits per pixel, bits per channel, and the number of channels of data per pixel. Unfortunately, that constant is the one that was growing a rate best described as "absurd". That constant is the actual definition of what it means to have no priors. When I said "you can generate arbitrarily many hypotheses, but if you don't control what data you receive, and there's no interaction possible, then you can't rule out hypotheses", what I'm describing is this constant.
I think it would be very weird, if we were trying to train an AI, to send it compressed video, and much more likely that we do, in fact, send it raw RGB values frame by frame.
What I care about is the difference between:
1. Things that are computable.
2. Things that are computable efficiently.
These sets are not the same.
Capabilities of a superintelligent AGI lie only in the second set, not the first.
It is important to understand that a superintelligent AGI is not brute forcing this in the way that has been repeatedly described in this thread. Instead the superintelligent AGI is going to use a bunch of heuristics or knowledge about the provenance of the binary file, combined with access to the internet so that it can just lookup the various headers and features of common image formats, and it'll go through and check all of those, and then if it isn't any of the usual suspects, it'll throw up metaphorical hands, and concede defeat. Or, to quote the title of this thread, intelligence isn't magic.
- ^
This is often phrased as bits per pixel, because a variety of color depth formats use less than 8 bits per channel, or other non-byte divisions.
- ^
Refer to https://accidentallyquadratic.tumblr.com/ for examples.
- ^
A fun question to consider here becomes: where are the alpha bits stored? E.g. if we assume 3 bytes for RGB data, and then we have the 1 alpha bit, is each pixel taking up 9 bits, or are the pixels stored in runs of 8 pixels followed by a single "alpha" pixel with 8 bits describing the alpha channels of the previous 8 pixels?
- ^
- ^
- ^
The way this works for real reverse engineering is that we already have expectations of what the data should look like, and we are tweaking inputs and outputs until we get the data we expected. An example would be figuring out a camera's RAW format by taking pictures of carefully chosen targets like an all white wall, or a checkerboard wall, or an all red wall, and using the knowledge of those targets to find patterns in the data that we can decode.
Why do you say that Kolmogorov complexity isn't the right measure?
most uniformly sampled programs of equal KC that produce a string of equal length.
...
"typical" program with this KC.
I am worried that you might have this backwards?
Kolmogorov complexity describes the output, not the program. The output file has low Kolmogorov complexity because there exists a short computer program to describe it.
I have mixed thoughts on this.
I was delighted to see someone else put forth an challenge, and impressed with the amount of people who took it up.
I'm disappointed though that the file used a trivial encoding. When I first saw the comments suggesting it was just all doubles, I was really hoping that it wouldn't turn out to be that.
I think maybe where the disconnect is occurring is that in the original That Alien Message post, the story starts with aliens deliberately sending a message to humanity to decode, as this thread did here. It is explicitly described as such:
From the first 96 bits, then, it becomes clear that this pattern is not an optimal, compressed encoding of anything. The obvious thought is that the sequence is meant to convey instructions for decoding a compressed message to follow...
But when I argued against the capability of decoding binary files in the I No Longer Believe Intelligence To Be Magical thread, that argument was on a tangent -- is it possible to decode an arbitrary binary files? I specifically ruled out trivial encodings in my reasoning. I listed the features that make a file difficult to decode. A huge issue is ambiguity because in almost all binary files, the first problem is just identifying when fields start or end.
I gave examples like
- Camera RAW formats
- Compressed image formats like PNG or JPG
- Video codecs
- Any binary protocol between applications
- Network traffic
- Serialization to or from disk
- Data in RAM
On the other hand, an array of doubles falls much more into this bucket
data that is basically designed to be interpreted correctly, i.e. the data, even though it is in a binary format, is self-describing.
With all of the above said, the reason why I did not bother uploading an example file in the first thread is frankly because it would have taken me some number of hours to create and I didn't think there would be any interest in actually decoding it by enough people to justify the time spent. That assumption seems wrong now! It seems like people really enjoyed the challenge. I will update accordingly, and I'll likely post my example of a file later this week after I have an evening or day free to do so.
https://en.wikipedia.org/wiki/Kolmogorov_complexity
The fact that the program is so short indicates that the solution is simple. A complex solution would require a much longer program to specify it.
I gave this post a strong disagree.
Some thoughts for people looking at this:
- It's common for binary schemas to distinguish between headers and data. There could be a single header at the start of the file, or there could be multiple headers throughout the file with data following each header.
- There's often checksums on the header, and sometimes on the data too. It's common for the checksums to follow the respective thing being checksummed, i.e. the last bytes of the header are a checksum, or the last bytes after the data are a checksum. 16-bit and 32-bit CRCs are common.
- If the data represents a sequence of messages, e.g. from a sensor, there will often be a counter of some sort in the header on each message. E.g. a 1, 2, or 4-byte counter that provides ordering ("message 1", "message 2", "message N") that wraps back to 0.
You should add computational complexity.
I’m not sure if your comment is disagreeing with any of this. It sounds like we’re on the same page about the fact that exact reasoning is prohibitively costly, and so you will be reasoning approximately, will often miss things, etc.
I agree. The term I've heard to describe this state is "violent agreement".
so in practice wrong conclusions are almost always due to a combination of both "not knowing enough" and “not thinking hard enough” / “not being smart enough.”
The only thing I was trying to point out (maybe more so for everyone else reading the commentary than for you specifically) is that it is perfectly rational for an actor to "not think hard enough" about some problem and thus arrive at a wrong conclusion (or correct conclusion but for a wrong reason), because that actor has higher priority items requiring their attention, and that puts hard time constraints on how many cycles they can dedicate to lower priority items, e.g. debating AC efficiency. Rational actors will try to minimize the likelihood that they've reached a wrong conclusion, but they'll also be forced to minimize or at least not exceed some limit on allowed computation cycles, and on most problems that means the computation cost + any type of hard time constraint is going to be the actual limiting factor.
Although even that, I think that's more or less what you meant by
in some sense you’ve probably spent too long thinking about the question relative to doing something else
In engineering R&D we often do a bunch of upfront thinking at the start of a project, and the goal is to identify where we have uncertainty or risk in our proposed design. Then, rather than spend 2 more months in meetings debating back-and-forth who has done the napkin math correctly, we'll take the things we're uncertain about and design prototypes to burn down risk directly.
First, it only targeted Windows machines running an Microsoft SQL Server reachable via the public internet. I would not be surprised if ~70% or more theoretically reachable targets were not infected because they ran some other OS (e.g. Linux) or server software instead (e.g. MySQL). This page makes me think the market share was actually more like 15%, so 85% of servers were not impacted. By not impacted, I mean, "not actively contributing to the spread of the worm". They were however impacted by the denial-of-service caused by traffic from infected servers.
Second, the UDP port (1434) that the worm used could be trivially blocked. I have discussed network hardening in many of my posts. The easiest way to prevent yourself from getting hacked is to not let the hacker send traffic to you -- blocking IP ranges, ports, unneeded Ethernet or IP protocols, and other options available in both network hardware (routers) or software firewalls provides a low cost and highly effective way to do so. This contained the denial-of-service.
Third, the worm's attack only persisted in RAM, so the only thing a host had to do was restart the infected application. Combined with the second point, this would prevent the machine from being reinfected.
This graph[1] shows the result of wide-spread adoption of filter rules within hours of the attack being detected
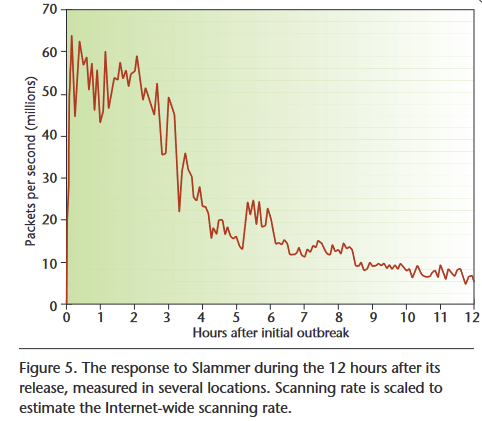
This was actually a kind of fun test case for a priori reasoning. I think that I should have been able to notice the consideration denkenbgerger raised, but I didn't think of it. In fact when I stared reading his comment my immediate reaction was "this methodology is so simple, how could the equilibrium infiltration rate end up being relevant?" My guess would be that my a priori reasoning about AI is wrong in tons of similar ways even in "simple" cases. (Though obviously the whole complexity scale is shifted up a lot, since I've spent hundreds of hours thinking about key questions.)
This idea -- that you should have been able to notice the issue with infiltration rates -- is what I've been questioning when I ask "what is the computational complexity of general intelligence" or "what does rational decision making look like in a world with computational costs for reasoning".
There is a mindset that people are simply not rational enough, and if they were more rational, they wouldn't fall to those traps. Instead, they would more accurately model the situation, correctly anticipate what will and won't matter, and arrive at the right answer, just by exercising more careful, diligent thought.
My hypothesis is that whatever that optimal "general intelligence" algorithm[1] is -- the one where you reason a priori from first principles, and then you exhaustively check all of your assumptions for which one might be wrong, and then you recursively use that checking to re-reason from first principles -- it is computational inefficient enough in such a way that for most interesting[2] problems, it is not realistic to assume that it can run to completion in any reasonable[3] time with realistic computation resources, e.g. a human brain, or a supercomputer.[4]
I suspect that the human brain is implementing some type of randomized vaguely-Monte-Carlo-like algorithm when reasoning, which is how people can (1) often solve problems in a reasonable amount of time[5], (2) often miss factors during a priori reasoning but understand them easily after they've seen it confirmed experimentally, (3) different people miss different things, (4) often if someone continues to think about a problem for an arbitrarily long people of time[6] they will continue to generate insights, and (5) often those insights generated from thinking about a problem for an arbitrarily long period of time are only loosely correlated[7].
In that world, while it is true that you should have been able to notice the problem, there is no guarantee on how much time it would have taken you to do so.
- ^
The "God algorithm" for reasoning, to use a term that Jeff Atwood wrote about in this blog post. It describes the idea of an optimal algorithm that isn't possible to actually use, but the value of thinking about that algorithm is that it gives you a target to aim towards.
- ^
The use of the word "interesting" is intended to describe the nature of problems in the real world, which require institutional knowledge, or context-dependent reasoning.
- ^
The use of the word "reasonable" is intended to describe the fact that if a building is on fire and you are inside of it, you need to calculate the optimal route out of that burning building in a time period that is than a few minutes in length in order to maximize your chance of survival. Likewise, if you are tasked to solve a problem at work, you have somewhere between weeks and months to show progress or be moved to a separate problem. For proving a theorem, it might be reasonable to spend 10+ years on it if there's nothing necessitating a more immediate solution.
- ^
This is mostly based on an observation that for any scenario with say some fixed number of "obvious" factors influencing it, there are effectively arbitrarily many "other" factors that may influence the scenario, and the process of deterministically ordering an arbitrarily long list and then preceding down the list from "most likely to impact the situation" and "least likely to impact the scenario" to manually check if each "other" factor actually does matter has an arbitrarily high computational cost.
- ^
Feel free to put "solve" in quotes and read this as "halt in a reasonable time" instead. Getting the correct answer is optional.
- ^
Like mathematical proofs, or the thing where people take a walk and suddenly realize the answer to a question they've been considering.
- ^
It's like the algorithm jumped from one part of solution space where it was stuck to a random, new part of the solution space and that's where it made progress.
I deliberately tried to focus on "external" safety features because I assumed everyone else was going to follow the task-as-directed and give a list of "internal" safety features. I figured that I would just wait until I could signal-boost my preferred list of "internal" safety features, and I'm happy to do so now -- I think Lauro Langosco's list here is excellent and captures my own intuition for what I'd expect from a minimally useful AGI, and that list does so in probably a clearer / easier to read manner than what I would have written. It's very similar to some of the other highly upvoted lists, but I prefer it because it explicitly mentions various ways to avoid weird maximization pitfalls, like that the AGI should be allowed to fail at completing a task.
We can even consider the proposed plan (add a 2nd hose and increase the price by $20) in the context of an actual company.
The proposed plan does not actually redesign the AC unit around the fact that we now have 2 hoses. It is "just" adding an additional hose.
Let's assume that the distribution of AC unit cooling effectively looks something like this graphic that I made in 3 seconds.
In this image, we are choosing to assume that yes, in fact, 2-hose units are more efficient on average than a 1-hose unit. We are also recognizing that perhaps there is some overlap. Perhaps there are especially bad 2-hose units, and especially good 1-hose units.
Based on all of the evidence, I'm going to say that the average 1-hose unit does represent the minimum efficiency needed for cooling in an average consumer's use-case -- i.e. it is sufficient for their needs.
When I consider what would make a 2-hose unit good or bad, I suspect it has a lot to do with how much of the design is built around the fact that there are 2-hoses.
In your proposal, we simply add a 2nd hose to a unit that was otherwise designed functionally as a 1-hose unit. Let's consider where that might be plotted on this graph.
I'm going to claim based on vague engineering intuition / judgment / experience that it goes right here.
If I am right about where this proposal falls against the competition, then here's what we've done:
- This is not a 1-hose unit any more. Despite it being more efficient than the average 1-hose units, and only slightly more expensive, consumers looking at 1-hose units (because they are concerned about cost) will not see this model. The argument that it is "only $20 more expensive" is irrelevant. Their search results are filtered, they read online that they wanted a one-hose unit, this product has been removed from their consideration.
- This is a bad 2-hose unit. It is at the bottom of the efficiency scale, because other 2-hose units were actually designed to take full advantage of the 2-hoses. They will beat you on efficiency, even if they cost more. Wirecutter will list this in the "also ran" when discussing 2-hose units, "So and so sells a 2-hose model, but it was barely more efficient than a 1-hose, we cannot recommend it".
- A consumer looking at 2-hose units is already selecting for efficiency over cost, so they will not buy the "just add another hose" 2-hose unit, since it is on the wrong end of the 2-hose distribution.
- You will acquire a reputation as the company that sells "cheap" products -- your unit is cheaper than other 2-hose units, but isn't better because it wasn't designed as a 2-hose unit, and it was torn apart by reviewers.
- Fixing this inefficiency requires actually designing around 2-hoses, which likely results in something like this
"Minimum viable", in the context of a "minimum viable product" or MVP, is a term in engineering that represents the minimal thing that a consumer will pay to acquire. This is a product that can actually be sold. It's not the literal worst in its category, and it has a clear supremacy over cheaper categories. This is also called table stakes. Reviewers will consider it fairly, consumers will not rage review it, etc.
However, it's probably also a lot more expensive than the hypothetical "only $20 more" that has been repeatedly stated.
Even in the scenario where a reviewer does consider the "just add another hose" model when viewing one-hose units, we've already established that the one-hose unit is cheaper (by $20! if it's a $200 unit, that's 10%), and that the average 1-hose unit is sufficient for some average use-case. Therefore the rational consumer choice is to buy the cheaper one-hose anyway, because it's irrational to pay more for efficiency that isn't needed![1][2]
- ^
The exception here is some hypothetical consumer who knows, for a fact, that their unique situation requires a two-hose unit, e.g. they tried a one-hose unit already and it was insufficient.
- ^
There's also an argument here that a rational option is to buy a 1-hose unit, and then if you need slightly more efficiency, just buy & wrap the 1-hose with insulation, as described here. This allows the consumer to purchase at the lower price point and then add efficiency if needed for the cost of the insulation. It's unclear to me that the "just add another hose" AC would still perform better than an insulated 1-hose.
I didn't even think to check this math, but now that I've gone and tried to calculate it myself, here's what I got:
INSIDE | ΔINSIDE (CONTROL) | |||
AVERAGE OUTSIDE | 86.5 | |||
AVERAGE ONE HOSE Δ | 19.65 | 66.85 | 6.55 | |
AVERAGE TWO HOSE Δ | 22.45 | 64.05 | 9.35 | |
CONTROL Δ | 13.1 | 73.4 | ||
1.42 | ΔTWO/ΔONE |
EDIT: I see the issue. The parent post says that the control test was done at evening, where the temperature was 82 F. So it's not even comparable at all, imo.
I'll edit the range, and note that "uncomfortably hot" is my opinion. Rest of my analysis / rant still applies. In fact, in your case, you don't need need the AC unit at all, since you'd be fine with the control temperature.
I take fault with your primary conclusion, for the same reasons I gave in the first thread:
- You claim how little adding a 2nd hose would impact the system, without analyzing the actual constraints that apply to engineers building a product that must be shipped & distributed
- You still neglect the existence of insulating wraps for the hose which do improve efficiency, but are also not sold with the single-hose AC system, which lends evidence to my first point -- companies are aware of small cost items that improve AC system efficiency, but do not include them with the AC by default, suggesting that there is an actual price point / consumer market / confounding issue at play that prevents them doing so
The full posts, quoted here for convenience
I think one reason that this error occurs is that there's a mistaken assumption that the available literature captures all institutional knowledge on a topic, so if one simply spends enough time reading the literature, they'll have all requisite knowledge needed for policy recommendations. I realize that this statement could apply equally to your own claims here, but in my experience I see it happen most often when someone reads a handful of the most recently released research papers and from just that small sample of work tries to draw conclusions applicable that are broadly applicable to the entire field.
Engineering claims are particularly suspect because institutional knowledge (often in the form of proprietary or confidential information held by companies and their employees) is where the difference between what is theoretically efficient and what is practically more efficient is found. It doesn't even need to be protected information though -- it can also just be that due to manufacturing reasons, or marketing reasons, or some type of incredibly aggravating constraint like "two hoses require a larger box and the larger box pushes you into a shipping size with much higher per-volume / mass costs so the overall cost of the product needs to be non-linearly higher than what you'd expect would be needed for a single hose unit, and that final per-unit cost is outside of what people would like to pay for an AC unit, unless you then also make drastic improvements to the motor efficiency, thermal efficiency, and reduce the sound level, at which point the price is now even higher than before, but you have more competitive reasons to justify it which will be accepted by a large enough % of the market to make up for the increased costs elsewhere, except the remaining % of the market can't afford that higher per-unit cost at all, so we're back to still making and selling a one-hose unit for them".
Concrete example while we're on the AC unit debate -- there's a very simple way to increase efficiency of portable AC units, and it's to wrap the hot exhaust hose with insulating duct wrap so that less of the heat on that very hot hose radiates directly back into the room you're trying to cool. Why do companies not sell their units with that wrap? Probably for one of any of the following reasons -- A.) takes up a lot of space, B.) requires a time investment to apply to the unit which would dissuade buyers who think they can't handle that complexity, C.) would cost more money to sell and no longer be profitable at the market's price point, D.) has to be applied once the AC unit is in place, and generally is thick enough that the unit is no longer "portable" which during market testing was viewed as a negative by a large % of surveyed people, or E.) some other equally trivial sounding reason that nonetheless means it's more cost effective for companies to NOT sell insulating duct wrap in the same box as the portable AC unit.
Example of an AC company that does sell an insulating wrap as an optional add-on: https://www.amazon.com/DeLonghi-DLSA003-Conditioner-Insulated-Universal/dp/B07X85CTPX
EDIT: I want to make a meta point here, which is that I have not personally worked on ACs, but I have built & shipped multiple products to consumers, and the type of stupid examples I gave in the first AC post are not just made-up for fun. Engineers argue extensively in meetings about "how can we make product A better", and ideas get shot down for seemingly trivial reasons that basically come down to -- yes, in a vacuum, that would be better, but unfortunately, there's a ton of existing context like how large a truck is or what parts can actually be bought off the shelf that kneecap those ideas before they leave the design room. The engineers who designed the AC were not idiots, or morons, or clowns who don't understand thermodynamic efficiency. Engineering is about working around limitations. Those limitations do not have to be rooted in physics; society or infrastructure or consumer behavior around critical price points can all be just as real in terms of what it is feasible for a company to create. Just look at how many startups fail and the founder claims in a postmortem, "Yeah, our tech was way better, but unfortunately people wouldn't pay 10% more for it, even though it was AMAZING compared to our competitor. We just couldn't get them to switch."
EDIT 2: I'm pretty annoyed that you doubled-down on your conclusion even after admitting the actual efficiency difference was significantly less than expected, and then chose a different analysis to let you defend your original point anyway, so these edits might keep coming. Regarding market pressures, two-hose AC units do exist. Companies do sell them, and if consumers want to buy a two-hose AC unit, they can do so. But the presence of both one-hose AC units and two-hose AC units in the market tells us it is not winner-take-all and there is consumer behavior, e.g. around price or complexity, that prevents two-hose units from acquiring literally all market share. So until that changes, it will always be more rational for companies to sell one-hose AC units in addition to their two-hose AC unit, because otherwise they'd be leaving money on the floor by only servicing part of the consumer market. (EDIT 5: see also this post, which was itself a reply to AllAmericanBreakfast's reply on this thread here)
EDIT 3: Let's look at your math. Outdoor temp is 85-88 F, let's just take the average and call it 86.5 F. That's pretty hot. I'd definitely be uncomfortable in that scenario. How cold did the AC cool the rooms? You say on low fan it was 20.6 F degrees with one hose, 22.7 F with two hoses, and then on high fan, 18.3 F with one hose, and 22.2 F with two hoses. The control was 13.1 F. Looking at the control, that gives a room temperature of ~73.4 F. That is uncomfortably hot in my opinion. I keep my room temperature around 68-70 F ish. The internet tells me that this is within the window of a "comfortable room temperature" defined as 67-75 F[1], so I'm just a normal human, I guess. How well did the ACs accomplish that? With one hose, you got it down to ~66 F, and with two hoses, you had it down to about ~64 F. That is pretty cold in my mind -- I would not set my AC that low if it actually reached that temperature. What does this mean? The one hose unit literally did the job it was designed to do. With an incredibly hot outside temperature, that resulted in an uncomfortable indoor "control" temperature, the one-hose AC was able to lower the temperature to a comfortable, ideal range, and then go below that, showing it even has margin left over. But now you're saying that they should make the thing more expensive and optimize it for even greater efficiency because ... why!? It works!
EDIT 4: I will die on this hill. This is the problem with how the rationalist community approaches the concept of what it means to "make a rational decision" perfectly demonstrated in a single debate. You do not make a "rational decision" in the real world by reasoning in a vacuum. That is how you arrive at a hypothetically good action, but it is not necessarily feasible or possible to perform, so you always need to check your analysis by looking at real world constraints and then pick the action that is 1.) actually possible in the real world, and 2.) still has the highest expected value. Failing to do that is not more clever or more rational, it is just a bad, broken model for how an ideal, optimal agent would behave. An optimal agent doesn't ignore their surroundings -- they play to them, exploit them, use them.
- ^
I averaged the following lower / upper temperatures.
Wikipedia: 64-75
www.cielowigle.com: 68-72
www.vivint.com: 68-76
www.provicincialheating.ca: 68-76
I really like this list because it does a great job of explicitly specifying the same behavior I was trying to vaguely gesture at in my list when I kept referring to AGI-as-a-contract-engineer.
Even your point about it doesn't have to succeed, it's ok for it to fail at a task if it can't reach it in some obvious, non-insane way -- that's what I'd expect from a contractor. The idea that an AGI would find that a task is generally impossible but identify a novel edge case that allows it to be accomplished with some ridiculous solution involving nanotech and then it wouldn't alert or tell a human about that plan prior to taking it has always been confusing to me.
In engineering work, we almost always have expected budget / time / material margins for what a solution looks like. If someone thinks that solution space is empty (it doesn't close), but they find some other solution that would work, people discuss that novel solution first and agree to it.
That's a core behavior I'd want to preserve. I sketched it out in another document I was writing a few weeks ago, but I was considering it in the context of what it means for an action to be acceptable. I was thinking that it's actually very context dependent -- if we approve an action for AGI to take in one circumstance, we might not approve that action in some vastly different circumstance, and I'd want the AGI to recognize the different circumstances and ask for the previously-approved-action-for-circumstance-A to be reapproved-for-circumstance-B.
EDIT: Posting this has made me realize that idea of context dependencies is applicable more widely than just allowable actions, and it's relevant to discussion of what it means to "optimize" or "solve" a problem as well. I've suggested this in my other posts but I don't think I ever said it explicitly: if you consider human infrastructure, and human economies, and human technology, almost all "optimal" solutions (from the perspective of a human engineer) are going to be built on the existing pile of infrastructure we have, in the context of "what is cheapest, easiest, the most straight line path to a reasonably good solution that meets the requirements". There is a secret pile of "optimal" (in the context of someone doing reasoning from first principles) solutions that involve ignoring all of human technology and bootstrapping a new technology tree from scratch, but I'd argue that's a huge overlap if not the exact same set as the things people have called "weird" in multiple lists. Like if I gave a contractor a task to design a more efficient paperclip factory and they gave me a proposed plan that made zero reference to buying parts from our suppliers or showed the better layout of traditional paper-clip making machines or improvements to how an existing paper-clip machine works, I'd be confused, because that contractor is likely handing me a plan that would require vertically integrating all of the dependencies, which feels like complete overkill for the task that I assigned. Even if I phrased my question to a contractor as "design me the most efficient paperclip factory", they'd understand constraints like: this company does not own the Earth, therefore you may not reorder the Earth's atoms into a paperclip factory. They'd want to know, how much space am I allowed? How tall can the building be? What's the allowable power usage? Then they'd design the solution inside of those constraints. That is how human engineering works. If an AGI mimicked that process and we could be sure it wasn't deceptive (e.g. due to interpretability work), then I suspect that almost all claims about how AGI will immediately kill everyone are vastly less likely, and the remaining ways AGI can kill people basically reduce to the people controlling the AGI deliberately using it to kill people, in the same way that the government uses military contractors to design new and novel ways of killing people, except the AGI would be arbitrarily good at that exercise.
Oh, sorry, you're referring to this:
includes a distributed network of non-nuclear electromagnetic pulse emitters that will physically shut down any tech infrastructure appearing to be running rogue AI agents.
This just seems like one of those things people say, in the same vein as "melt all of the GPUs". I think that non-nuclear EMPs are still based on chemical warheads. I don't know if a "pulse emitter" is a thing that someone could build. Like I think what this sentence actually says is equivalent to saying
includes a distributed network of non-nuclear ICBMs that will be physically shot at any target believed to be running a rogue AI agent
and then we can put an asterisk on the word "ICBM" and say it'll cause an EMP at the detonation site, and only a small explosion.
But you can see how this now has a different tone to it, doesn't it? It makes me wonder how the system defines "appears to be running rogue AI agents", because now I wonder what the % chance of false positives is -- since on a false positive, the system launches a missile.
What happens if this hypothetical system is physically located in the United States, but the rogue AI is believed to be in China or Russia? Does this hypothetical system fire a missile into another country? That seems like it could be awkward if they're not already on board with this plan.
because they're doing something pretty non-trivial, they probably have to be big complex systems. Because they're big complex systems, they're hackable. Does this sound right to you? I'm mostly asking you about the step "detecting rogue AI implies hackable". Or to expand the question, for what tasks XYZ can you feasibly design a system that does XYZ, but is really seriously not hackable even by a significantly superhuman hacker?
It's not really about "tasks", it's about how the hardware/software system is designed. Even a trivial task, if done on a general-purpose computer, with a normal network switch, the OS firewall turned off, etc, is going to be vulnerable to whatever exploits exist for applications or libraries running on that computer. Those applications or libraries expose vulnerabilities on a general-purpose computer because they're connected to the internet to check for updates, or they send telemetry, or they're hosting a Minecraft server with log4j.
It seems like you could not feasibly make an unhackable system that takes a bunch of inputs from another (unsafe) system and processes them in a bunch of complex ways using software that someone is constantly updating, because having the ability to update to the latest Detect-O-Matic-v3.4 without knowing in advance what sort of thing the Detect-O-Matic is, beyond that it's software, seems to imply being Turing-completely programmable, which seems to imply being hackable.
When you're analyzing the security of a system, what you're looking for is "what can the attacker control?"
If the attacker can't control anything, the system isn't vulnerable.
We normally distinguish between remote attacks (e.g. over a network) and physical attacks (e.g. due to social engineering or espionage or whatever). It's generally safe to assume that if an attacker has physical access to a machine, you're compromised.[1] So first, we don't want the attacker to have physical access to these computers. That means they're in a secure facility, with guards, and badges, and access control on doors, just like you'd see in a tech company's R&D lab.
That leaves remote attacks. These generally come in two forms:
- The attacker tricks you into downloading and running some compromised software. For example, visiting a website with malicious JavaScript, or running some untrusted executable you downloaded because it was supposed to be a cheat engine for a video game but it was actually just a keylogger, or the attacker has a malicious payload in a seemingly innocent file type like a Word document or PDF file and it's going to exploit a bug in the Word program or Adobe Acrobat program that tries to read that file.
- The attacker sends network traffic to the machine which is able to compromise the machine in some way, generally by exploiting open ports or servers running on the target machine.
All of the attacks in (1) fall under "when you run untrusted code, you will get pwned" umbrella. There's a bunch of software mitigations for trying to make this not terrible, like admin users vs non-admin users, file system permissions, VM sandboxing, etc, but ultimately it's just like rearranging deck chairs on the Titanic. It doesn't matter what you do, someone else is going to find a side channel attack and ruin your day if you let them run code on your machine. So don't do that. This is actually easier than you might think: plenty of systems are "secure" because they run an incredibly minimal Linux OS (or some RTOS or even just bare metal) and they're effectively static -- the software image is flashed to some SoC's read-only memory (ROM) by an external debugger[2], and there's no capability from within the software to write or update that memory. The processor is not configured for running code outside of that ROM. There's no user accounts, or "default applications", or browsers, or anything else other than the code for performing the actual task required by the system.
For (2), in cases where the system is not supposed to be receiving arbitrary traffic, we solve this by using networking hardware downstream of the system that drops any unexpected traffic, and rate-limits everything. If the attacker's traffic gets dropped, they can't control anything. The rate-limiting is to prevent a denial-of-service based on traffic we expect. For the "expected" traffic, the ideal mechanism to prevent abuse is a binary schema for valid messages, a fixed MTU size on messages, and the use of authenticated encryption so that the attacker is unable to generate the traffic without access to encryption keys. Encryption keys can be stored in hardware security modules that are physically located on the senders & receivers within the system.[3]
With that in mind, I hope it is clear that the problem isn't about complexity of a system, or the number of inputs that the system has, or if the system supports software updates.
The actual issue with the system we're discussing is that the attacker can control their network traffic, and in this hypothetical, we need to look at arbitrary network traffic to decide if it's evidence of a rogue AI.[4] We can't just drop everything in hardware and ignore messages that don't fit an authenticated encryption scheme, because we want to read that traffic.
So we have to assume that the attacker can generate arbitrary payloads, send them to our system, and our system is going to need to not get pwned.
I think I'd probably approach it with the following thoughts:
- I'd design the system so that the traffic to be inspected is distributed across a cluster of computers. This is both to prevent denial-of-service attacks and to prevent targeted attacks like Nethammer. If the system is receiving traffic in excess of what the cluster is designed to handle, it'll be dropped -- while this represents a degradation in monitoring capability, it can be fixed by adding additional machines to the cluster as needed. It's often useful to gracefully degrade capabilities when a system is under load.
- The computers in the cluster that receive traffic have a simple role: send an alert message to a central supervisor if suspicious traffic is detected.
- The alert message uses authenticated encryption.
- The central supervisor is only communicated with via authenticated encryption messages on a local network. It is not connected to the wider network and it is not possible for the attacker to send traffic to the supervisor machine, unless they first compromised one of the cluster machines.
The supervisor machine is pretty safe unless a cluster machine is compromised. If a cluster machine is compromised, maybe we think that the attacker can control the alert message, and in doing so, control traffic sent to the central supervisor? Let's fix that. What do we need for the alert message? Maybe the IP of the packet, geolocation information, reasons why it was suspicious? Etc. We'll define a binary schema for that information and we'll stick a serial device or FPGA onto each cluster machine.
- The cluster machines send the alert message by setting the relevant fields of the message via the serial device or FPGA registers, and it's that device which sends the actual alert. Now if the cluster machine is compromised, all the attacker can do is change fields in the alert message, but they can't send different messages entirely.[5]
- We test the central supervisor to confirm that any permutation of the alert message is still safe to receive. For testing permutations, we normally use a method based on equivalence classes.
So now attackers can maybe compromise cluster machines because they control the traffic that we're trying to monitor, but they can't use a compromised cluster machine to control traffic to the central supervisor, because compromising a cluster machine doesn't let you change the traffic sent to a supervisor in a way that would be dangerous.
At this point we can ask what the attacker can do:
- They can maybe send garbage alert messages.
- They can maybe send no message at all -- i.e. they crash the cluster machine so it fails silent.
We can mitigate the 2nd problem by requiring a heartbeat from each cluster machine to the central supervisor. A heartbeat is another message sent via authenticated encryption, but it's sent at a known frequency, like 100 Hz. If an attacker or any other event crashes the machine, the central supervisor will know.
If the attacker sends a garbage alert message, what is the downside? It could be bad if this system launches missiles, so maybe we'll have human review on any alert that the central supervisor receives, prior to any action being taken by the system. The human review can include a log of the network traffic that generated the alert, captured by the cluster machine, and a diagnosis of the cluster machine's telemetry for how it concluded the traffic was suspicious. This is intended to mitigate the possibility of a false positive where the attacker can control where we think a rogue AI is located.
Now, where we stand is that an attacker can maybe compromise a cluster machine, and in doing so, they can maybe generate an erroneous alert message for a rogue AI, which a human review maybe won't be able to detect as erroneous, so whatever action gets taken on belief that a rogue AI exists would be sent to a location controlled by the attacker. The magnitude of how bad that is depends on what action is taken. If it is "fire an ICBM", it could be pretty bad.
The other possibility is that an attacker compromises a machine in such a way that no alert is generated, but no heartbeat is missed either. This is an interesting failure mode because it's equivalent to another failure mode that we have not discussed: the system could simply fail to detect the behavior of a rogue AI. In other words, this system doesn't need to be hacked in order to fail. If the algorithm we're using for detecting rogue AI has any margin for error at all, then it should be no surprise to anyone that this system is susceptible to a false negative, i.e.: it did not send an alert when it should have.
The relevant question becomes, what's the margin of error on the hypothetical algorithm for detecting a rogue AI? If that algorithm has a higher likelihood of a false positive than our hypothetical attacker, after all of our mitigations, than maybe this system isn't even worth building, depending on how high that likelihood is. Likewise, if the algorithm has a high likelihood of a false negative, such that a rogue AI can reasonably evade detection due to not being suspicious enough, then this system might still not be worth building, because now the most likely failure mode is that the system just doesn't work reliably enough, hacked or not.
- ^
This is a safe assumption because if the attacker has physical access to a machine, they can do dumb things like pull out the hard drive and put it into another computer that they control. Enable encryption on your drives.
- ^
When I say "external debugger", this is implying physical access to the system.
- ^
In order to get the encryption keys, the attacker needs physical access to the system. Attacks that would use a side-channel attack won't work here, because we don't accept arbitrary traffic, and in order to generate that traffic, you need the keys. It's a catch-22, unless you have physical access. But if you have physical access, we assume the system is compromised.
- ^
To be clear, I am not convinced that "evidence of a rogue AI" is a meaningful description of behavior.
- ^
This is assuming that we've verified the device that sends the alert message, which we'll have high confidence in because of how simple the implementation will be.
Would you agree that if there were a system that could automatically (without humans) monitor for rogue AI, then that system is probably hackable?
I can't comment on this, because I have no idea what this system would even do, or what it would be looking for, or who would be running it.
What indicates a rogue AI? How is that differentiated from human hackers? How is that different from state-level actors like the NSA? How is it different from some white hat security researchers doing pen-testing in the wild for questionable reasons? What "behavior" is the system trying to look for?
How is it monitoring? What inputs is it taking? Is it only looking at internet traffic? How? Where is it tapping for those packets? There's a lot of internet traffic. Is this even "a computer", or it, "an entire facility of computers". Why are there no humans involved in this process? Where do the logs, monitoring, or other telemetry go if this is done "without humans"? Wouldn't this system be reporting some type of digest or summary for review? Why do you think that it would need to be a general computer? Do you think that high-performance SoCs do not exist, or that it would not be possible to have a distributed system containing multiple SoCs with the equivalent RAM and compute of some general-purpose machine?
What would it mean to hack this system? Just that it doesn't report any rogue AI anymore? Or that it erroneously reports the presence of rogue AI? What would hacking it to not report rogue AI look like? Are we concerned that it would log the presence of a rogue AI, but then the rogue AI would be able to wipe that entry from some log file, like a movie? Or are we thinking that the rogue AI is going to disable this system before it is even noticed by the system? Do we count "turning off the power" as "hacking"?
I don't think that this TL;DR is particularly helpful.
People think attacks like Rowhammer are viable because security researchers keep releasing papers that say the attacks are viable.
If I posted 1 sentence and said "Rowhammer has too many limitations for it to be usable by an attacker", I'd be given 30 links to papers with different security researchers all making grandiose claims about how Rowhammer is totally a viable attack, which is why 8 years after the discovery of Rowhammer we've had dozens of security researchers reproduce the attack and 0 attacks in the wild[1] that make use of it.
If my other posts haven't made this clear, I think almost all disagreements in AI x-risk come down to a debate over high-level vs low-level analysis. Many things sound true as a sound-bite or quick rebuttal in a forum post, but I'm arguing from my perspective and career spent working on hardware/software systems that we've accumulated enough low-level evidence ("the devil is in the details") to falsify the high-level claim entirely.
- ^
We can argue that just because we don't know that someone has used Rowhammer -- or a similar probabilistic hardware vulnerability -- doesn't mean that someone hasn't. I don't know if that's a useful tangent either. The problem is that people use these side-channel attacks as an "I win" button in arguments about secure software systems by making it seem like the existence of side channel exploits is therefore proof that security is a lost cause. It isn't. It isn't about the intelligence of the adversary, it's that the target basically needs be sitting there, helping the attack happen. On any platform where part of the stack is running someone else's code, yeah, you're going to get pwned if you just accept arbitrary code, so maybe don't do that? It is not rocket science.
I am only replying to the part of this post about hardware vulnerabilities.
Like, superhuman-at-security AGIs rewrote the systems to be formally unhackable even taking into account hardware vulnerabilities like Rowhammer that violate the logical chip invariants?
There are dozens of hardware vulnerabilities that exist primarily to pad security researcher's bibliographies.
Rowhammer, like all of these vulnerabilities, is viable if and only if the following conditions are met:
- You know the exact target hardware.
- You also know the exact target software, like the OS, running on that hardware.
- You need to know what in RAM you're trying to flip, and where to target to do so, like a page table or some type of bit for the user's current access level.
- The target needs to actually execute your code.
- In attacks where security researchers pad themselves for pulling off Rowhammer remotely, it's because they use JavaScript or WebGL in a browser, and then pwn devices that use browsers. This is flaw almost entirely reserved for general-purpose compute hardware, because embedded software or other application-specific hardware/software systems don't need or have browsers in them.
- In all other attacks, it involves downloading & executing a program on the target machine. Normally the example is given with cloud VMs whose entire purpose is to run code from an external source. Again, this is reserved for general-purpose compute hardware, because systems that execute code only out of read-only memory will not be able to execute an attacker's code.
- There is time on the target to run the Rowhammer attack uninterrupted. It relies on a continuous and degenerate set of instructions. This can be anywhere from minutes to days of time. This means that systems that don't give uninterrupted time to external code are also not vulnerable.
- The target OS, or other software, on the system needs to not perform any type of RAM scrubbing. There are papers claiming that variants of Rowhammer work for systems that use ECC + scrubbing, but those papers also assume that the scrubbing happens over hours. If a system has very little RAM, like an embedded processor, it is feasible for hardware to scrub RAM far faster than that.
- These exploits also add the requirement that the attacker needs to know the exact target RAM and ECC algorithm.
- The target hardware/software system needs to not have any hardware level redundancy. You can't rowhammer a system that has 3 separate computers that compare & vote their state on a synchronized clock. Hardware vulnerabilities are probabilistic attacks. They don't work anymore if the attack must occur simultaneously, and identically on separate physical systems. This is another reason why we're able to build systems that function despite hostile environments where bit flips are routine, i.e. high radiation.
- The target needs to not crash. Semi-randomly flipping bits in roughly the right location in RAM is not something that most software is designed to handle, and in an overwhelming number of cases, trying to execute this attack will crash the system.
It's not that Rowhammer isn't possible in the sense that it cannot be shown to work, but it's like this paper showing that you can create WiFi signals in air-gapped computers. Or this fun paper for Nethammer showing novel attacks that don't require code execution on the target machine, except they also don't allow for controlling where bit flips occur, so the "attack" is isomorphic to an especially hostile radiation environment with a high likelihood of bit-flips, and it relies on the ability for the attacker to swarm the target system with a high volume (500 Mbps?) of network traffic that they control -- a network switch that drops unexpected traffic or even just rate-limits it will defeat Nethammer. Note that rate-limiting network traffic is in fact standard practice for high stability systems, because it's also a protection against much more mundane denial-of-service attacks.
Consumer systems are vulnerable to attacks, because consumer systems don't care about stability. Consumers want to have a fast network connection to the internet. There's no requirement, or need, for that to be true on a system designed for stability, like something in a satellite, or some other safety-critical role. It is possible to have systems that are effectively "not able to be hacked" -- they don't use general-purpose hardware, they don't have code that can be modified, they have no capability for executing external code, they include hardware level fault tolerance and redundancy, and they have exceptionally limited I/O. It doesn't require us presuming "superhuman-at-security AGIs" exist to design these systems.
Every few weeks researchers publish papers carefully documenting the latest side-channel attacks that result in EVERYTHING EVERYWHERE BEING VULNERABLE FOREVER, and every few weeks attackers continue to do the boring old thing of leaving USB drives lying around for a target to pwn themselves, or letting the target just download the malware directly to their machine. They're almost all just remixes too -- it's "here's how to do exploit A (the original), but on hardware systems that implemented mitigations for C, D, and E". Except exploit A still has all of the preconditions I listed above, and now you've got whatever new preconditions the security researchers have in their latest paper.
I worry that the question as posed is already assuming a structure for the solution -- "the sort of principles you'd build into a Bounded Thing meant to carry out some single task or task-class and not destroy the world by doing it".
When I read that, I understand it to be describing the type of behavior or internal logic that you'd expect from an "aligned" AGI. Since I disagree that the concept of "aligning" an AGI even makes sense, it's a bit difficult for me to reply on those grounds. But I'll try to reply anyway, based on what I think is reasonable for AGI development.
In a world where AGI was developed and deployed safely, I'd expect the following properties:
1. Controlled environments.
2. Controlled access to information.
3. Safety-critical systems engineering.
4. An emphasis on at-rest encryption and secure-by-default networking.
5. Extensive logging, monitoring, interpretability, and circuit breakers.
6. Systems with AGI are assumed to be adversarial.
Let's stop on the top of the mountain and talk about (6).
Generally, the way this discussion goes is we discuss how unaligned AGI can kill everyone, and therefore we need to align the AGI, and then once we figure out how to align the AGI, problem solved, right?
Except someone then points out that, well, other people might create unaligned AGI, and then that will kill everyone, so that's awkward.
Also maybe the team that thought they aligned the AGI actually didn't, their proof had a mistake, whoops.
Or they had a formally proven proof, but they deployed it to a general purpose computer, implemented zero hardware redundancy, and then a bit flip caused the system to kill everyone anyway, whoops.
So normally we don't discuss the last 2 failure modes, because it's a bit awkward for the discussion of alignment, and we instead talk about how the deployment of the aligned AGI is going to go totally fine, and then we'll just do a little pivotal act.
How does AGI kill everyone in these stories? Almost always, the answer is nanotechnology. If the answer isn't nanotechnology, it's hacking into <hackable thing>
.
When you try to suggest that maybe the AGI shouldn't be able to hack into <hackable thing>
, you're told that's insane, because that would be boxing the AGI, and we know that boxing doesn't work.
The world I am going to describe might not be our world, but it also isn't Eliezer's world.
We are separated by a few critical assumptions -- and it starts with hardware.
1. Controlled environments.
In a world where we cared about AGI being developed safely, here's a fun idea: you know all that TPU hardware that Google designed, or the other custom AI/ML chips that companies are working? It's all just glorified GPUs, except the Mythic AMP is kind of cool, since it's an actual analog compute platform for running neural networks, like an analog FPGA. What if AGI wasn't developed to run on general-purpose consumer hardware? What if it can't be run on general-purpose hardware? What if the general intelligence algorithm is computationally inefficient enough that the path to AGI actually looks like incredibly specialized, heavily parallelized, wide processors with low clock rates, large caches, and fast memory access.
This is a world where the AGI machine code must be flashed to the ROM via an external debugger. There is no ability to flash or update the code from within the hardware/software system. The AGI cannot update itself. We do not design, deploy, or use self-improving systems.
This is a world where the hardware used for AGI development uses a memory-protection unit so that memory accesses to the ROM containing the AGI machine code trigger a hardware exception. It is not possible to read the ROM from within the hardware/software system. The AGI cannot inspect its own source code.
This is a world where the AGI has RAM, but no accessible non-volatile memory. If it loses power, poof, gone. The AGI cannot maintain, or persist, any type of internal or "custom" state between resets. Anything the AGI needs to know is either baked into the image flashed to the ROM, or it's transferred into the AGI's RAM after power-on.
This is a world where we deliberately leave performance on the floor because what we want from the system is consistency, predictability, and some minimum level of effectiveness above human capabilities -- even if we hypothetically could run an AGI system at some 1000x "speed" (whatever that means) relative to a human mind, doesn't mean that you'd have to do so. If you're worried about not being able to react to sudden or unexpected changes, then deliberately run the system at arbitrarily low clock rates. There is zero reason why we couldn't run an AGI on a custom hardware/software system that has a clock rate so low it thinks in glacial time relative to human perception.
This is a world where it's reasonable to regulate the AGI hardware the same way we regulate components for ICBMs, because it is not ubiquitously used, and we don't need to burn everyone's GPU -- we just need to not sell consumer AGI hardware, in the same way that we don't sell consumer GPS units that could be used for an ICBM instead of a car. The very concept of "stealing" or "releasing" the AGI doesn't make sense, because the hardware to run the AGI machine code is as carefully-controlled and rare as the hardware for custom-built spacecraft.
2. Controlled access to information.
In a world where we cared about AGI being developed safely, no one would be dumb enough to allow that system unrestricted access to networks. This would be accomplished by external, non-AGI systems. If we don't trust the AGI, it would be weird to try and use some other AGI system as a watchdog. So instead, use custom network hardware. Build it with an FPGA. Ethernet ports on either side. The custom switch has a simple rule: when a packet is received from the AGI, request approval. If no approval is given, drop the packet. Same for incoming traffic. If the complaint is that this is hugely inefficient because it slows down the AGI's ability to do ad-hoc discovery & learning, that's the point. If we want the AGI to solve a problem about optimizing car production, we will give it a data dump of material that we think is relevant for the problem. If it then wants to request additional information, then we have to approve that request. This is how workers are treated on projects with highly proprietary or otherwise silo'd information and that's how the AGI should be treated too.
But there's more. We're running the AGI on custom hardware, right? Internal to the AGI board, after the Ethernet (let's assume we're using one or more gigabit links) port on the PCB, we'll put a hardware security module and FPGA for decrypting the packets. This is not connected to, or part of, the hardware/software system running the AGI code. It is located on the PCB, but otherwise it is a separate system entirely. This system has one job: try to decrypt the traffic and drop anything that is invalid. Why are we going to do this? Because outside of this system, on the non-AGI network switch, we are going to encrypt traffic that we approve for the AGI. If we don't approve the traffic, it'll got dropped. We'll do the reverse for traffic generated by the AGI. It is always encrypted prior to leaving the system, and the AGI itself has no control over this function. If the custom network hardware isn't there to decrypt the approved traffic, it'll just be babbling noise indecipherable from random data.
There's no WiFi module on this hardware, and no public WiFi endpoints in the facility. All WiFi access points in the facility require passwords and have a white-list of approved MAC addresses.
The reason why controlling access to information matters is because there are hard limits to how far or fast an intelligent entity can reason in a vacuum. There is enough context-dependent information that you can't reason everything from first principles, because the world's current state has tons of structure embedded in it that humans put in place, and anything that wants to use human supply chains, logistics, and manufacturing is going to have to respect those totally arbitrary and unpredictable constraints.
An AGI won't be able to magically decode arbitrary data. If we don't give it access to datasheets, technical descriptions, or allow it to interact with data sources, it'll be just as stuck as any other intelligence faced with an arbitrary number of hypotheses and no way to rule any of them out.
Depending on how complex the "general intelligence" algorithm turns out to be, just reasoning through if-then logic and Bayesian world modeling could be non-trivial compute time. The more we control information for the AGI, the less feasible plans that involve looking at thousands of CCTV cameras simultaneously will seem.
3. Safety-critical systems engineering.
In a world where we cared about AGI being developed safely, the hardware that I'm describing above containing the hardware security module would actually have at least 3 identical processors running on a synchronized clock. The hardware security module would expect 3 identical packets for any I/O. If that did not occur, an immediate interrupt would power-cycle the system -- again, this is outside of the processors running the AGI code itself. This is because even if we assumed the AGI was "aligned", we don't want uncontrollable behavior like radiation-induced bit flips, hardware defects from manufacturing, or other unexpected issues to result in erroneous behavior.
Power for the entire system would be controlled via external, non-AGI systems. There would be multiple easily accessed locations for throwing a physical switch on the power line. In addition to that, there would be network accessible microprocessors for doing the same remotely, with the caveat that use small pyrotechnic charges are therefore irreversible. The default behavior of the system is that the power is controlled via these external systems and on reset, they do not supply power downstream to the AGI system itself. After an unexpected reset, e.g. loss of facility power, it requires manual control to re-enable downstream power to the AGI. This is done on a logically separate power domain from the rest of the facility -- i.e. you can turn on power to everything else in the facility, WITHOUT being forced to power anything related to the AGI system.
This is a world where AGI systems are designed under similar paranoia that you'd use for developing any other hardware/software system that could kill people, and the resulting pessimism drives us to isolate and limit functionality using non-AGI systems by default, where those non-AGI systems can be designed, implemented, verified, and QA-tested all using traditional systems safety methods.
4. An emphasis on at-rest encryption and secure-by-default networking.
In a world where we cared about limiting the damage that AGI could do, we would ask manufacturers of hard drives, solid state drives, and every operating system to require encryption at rest. Your work laptop probably already does this. If you're paranoid, you've got it enabled on your cellphone too. Do it everywhere. This is just good security practice, even without an AGI, but this is doubling down on (2) -- we are controlling access to information. Limit what can be known. Prevent discovery.
Ditto for the second point. Emphasize encrypted networking, VPNs, firewalls, and anything else that makes it harder for any attacker to find targets. Importantly, and I want to emphasize this point: maybe don't network factories to the internet and totally remote-controllable. This isn't actually a problem in today's world, as I've posted about it before, but it's something to think about going forward. If a failure mode in many stories where the AGI kills everyone is "well it could hack this cool networked factory", maybe don't do that? The weird thing about factories is that there kind of aren't that many in the world that conceivably fit that description? Like, there are lots of factories, but very few that are designed to build arbitrary products. It's a handful of places in China and they still require manual reconfiguration for new products. Most things called "factories" build specific products. The AGI that somehow takes over the Ford car factory is not going to immediately begin turning out things that are not Ford cars.
5. Extensive logging, monitoring, interpretability, and circuit breakers.
Remember all of those non-AGI systems from 2 and 3? They're constantly logging and telemetering information. If the AGI suddenly starts babbling like an idiot unexpectedly, cut the power. If the AGI is requesting information and has been denied repeatedly, cut the power. If the AGI unexpectedly starts using significantly more power, or the power begins to fluctuate in "weird" ways, cut the power. If any of these non-AGI systems report errors, or failures, cut the power. None of this needs to be controlled by humans. It's all driven by simple microprocessors running watchdogs. Even failures like "this switch is supposed to report telemetry, but didn't" will result in killing the power.
If you can do this for systems whose failure mode is dozens of people die, you can do this for the system whose failure mode is "everybody dies".
For the AGI itself, we want interpretability. As much as we can get. We don't want this to be a function of the AGI, because we don't trust the AGI. But we don't need it to be. We've got access to the RAM through an external debug interface. We don't need the AGI to report that state, and we don't have to trust it.
Even though we won't use AGI systems as watchdogs, it is acceptable to use traditional AI/ML systems for parts of this loop. Maybe no human can parse what the AGI's RAM says, but if we can train an AI/ML system to do a good job of producing a human-readable digest, that is better than nothing.
In a world where we cared about AGI being developed safely, the AGI is still treated like a task-based service. The AGI is given a problem to solve, and when it reports a solution and we finish negotiating with it on the particulars of the solution, we reset the system. We don't maintain long-running AGI instances because there's no need. Point it at a problem, get the solution, power-cycle it.
6. Systems with AGI are assumed to be adversarial.
Here we are again, back on the top of the mountain.
The point of the systems safety process is to make some system safe, despite the inherent unsafety of components within it.
To do that, we look for the ways it can cause harm, and we find mitigations.
We practice defense-in-depth, with an emphasis on redundancy and at least one-fault-tolerance.
In a world where we cared about not dying to AGI, it would be insufficient to expect that an aligned AGI would "just work". It is not enough for there to be a proof that a hypothetical product as-designed is safe. We also need to look at the likelihood of:
- design failures (the formal proof was wrong because the verification of it had a bug, there is no formal proof, the "formally verified" proof was actually checked by humans and not by an automated theorem prover)
- manufacturing failures (hardware behavior out-of-spec, missed errata, power failures, bad ICs, or other failure of components)
- implementation failures (software bugs, compiler bugs, differences between an idealized system in a proof vs the implementation of that system in some runtime or with some language)
- verification failures (bugs in tests that resulted in a false claim that the software met the formal spec)
- environment or runtime failures (e.g. radiation-induced upsets like bit flips; Does the system use voting? Is the RAM using ECC? What about the processor itself?)
- usage failures (is the product still safe if it's misused? what type of training or compliance might be required? is maintenance needed? is there some type of warning or lockout on the device itself if it is not actively maintained?)
- process failures ("normalization of deviance")
For each of these failure modes, we then look at the worst-case magnitude of that failure. Does the failure result in non-functional behavior, or does it result in erroneous behavior? Can erroneous behavior be detected? By what? Etc. This type of review is called an FMEA. This review process can rule out designs that "seem good on paper" if there's sufficient likelihood of failures and inability to mitigate them to our desired risk tolerances outside of just the design itself, especially if there exist other solutions in the same design space that do not have similar flaws.
If we did this process, and we stuck to it, I think we'd come to an awkward conclusion.
Many things that people have assumed we would give to the AGI to solve, we could just not.
If the AGI tells us the way to solve climate change is nanobots, we can say "no thanks, give us a different solution". It doesn't matter if the AGI promises us this solution is 99.99% efficient and the next best option using boring, conventional technology is only 70% efficient. We can opt to not do things that we don't think we can verify.
Or if the AGI gives us the plans for a remote controlled factory that is going to be so efficient because it's totally controlled by the AGI over the internet -- don't build that factory. Ask the AGI for the schematics, data sheets, and any other design material needed to build a new product, and build them the old fashioned way. Trade efficiency for stability.
That's how the system safety process is supposed to work. If after we do an FMEA, we are uncertain about a proposed solution, the ethical and correct response is to reject that solution!
If we ask the AGI to explain a plan, and it says "Humans can't comprehend the complexity of this.", then we should reply "Toss that one then, give us one we can understand".
That's how design reviews work.
You don't tell the review board, "You wouldn't understand why this design is safe."
Why would I hold a super-intelligent AGI to a lower standard than I would a human engineer?
Conclusion
Is any of this feasible?
In my mind, the key assumption comes down to whether AGI can be deployed to general-purpose hardware.
I think the answer might be "no", which is why we increasingly see the most successful AI/ML labs in the world are investing in specialized hardware like the Google TPUs, Cerebras Wafer, Nvidia JETSON, Microsoft / Graphcore IPU, Mythic AMP, or literal dozens of other examples. All of these are examples of specialized, dedicated HW for AI/ML systems that replace general-purpose hardware like a CPU or GPU.
The alternative is a world where a 10-year-old laptop can run an AGI in someone's living room.
I have not seen anything yet that makes me think we're leaning that way. Nothing about the human brain, or our development of AI/ML systems so far, makes me think that when we create an actual AGI, it'll be possible for that algorithm to run efficiently on general-purpose hardware.
In the world that I'm describing, we do develop AGI, but it never becomes ubiquitous. It's not like a world where every single company has pet AGI projects. It's the ones you'd expect. The mega-corporations. The most powerful nations. AGI are like nuclear power plants. They're expensive and hard to build and the companies that do so have zero incentive to give that away. If you can't spend the billions of dollars on designing totally custom, novel hardware that looks nothing like any traditional general-purpose computer hardware built in the last 40 years, then you can't develop the platform needed for AGI. And for the few companies that did pull it off, their discoveries and inventions get the dubious honor of being regulated as state secrets, so you can't buy it on the open market either. This doesn't mean AI/ML development stops or ceases. The development of AGI advances that field immensely too. It's just in the world I'm describing, even after AGI is discovered, we still have development focused on creating increasingly powerful, efficient, and task-focused AI/ML systems that have no generality or agent-like behavior -- a lack of capabilities isn't a dead-end, it's yet another reason why this world survives. If you don't need agents for a problem, then you shouldn't apply an agent to that problem.
Only if we pretend that it's an unknowable question and that there's no way to look at the limitations of a 286 by asking about how much data it can reasonably process over a timescale that is relevant to some hypothetical human-capable task.
http://datasheets.chipdb.org/Intel/x86/286/datashts/intel-80286.pdf
The relevant question here is about data transfers (bus speed) and arithmetic operations (instruction sets). Let's assume the fastest 286 listed in this datasheet -- 12.5 MHz.
Let's consider a very basic task -- say, catching a ball thrown from 10-15 feet away.
To simplify this analysis, we are going to pretend that if we can analyze 3 image frames, in close proximity, then we can do a curve fit and calculate the ball's trajectory, so that we don't need need to look at any other images.
Let's also assume that a 320x240 image is sufficient, and that it's sufficient for the image to be in 1-byte-per-pixel grayscale. For reference, that looks like this:
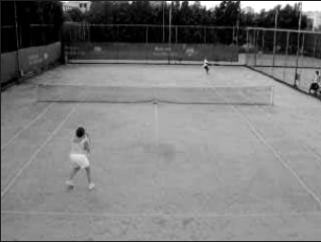
With the 12.5 MHz system clock, we're looking at 80 nanoseconds per clock cycle.
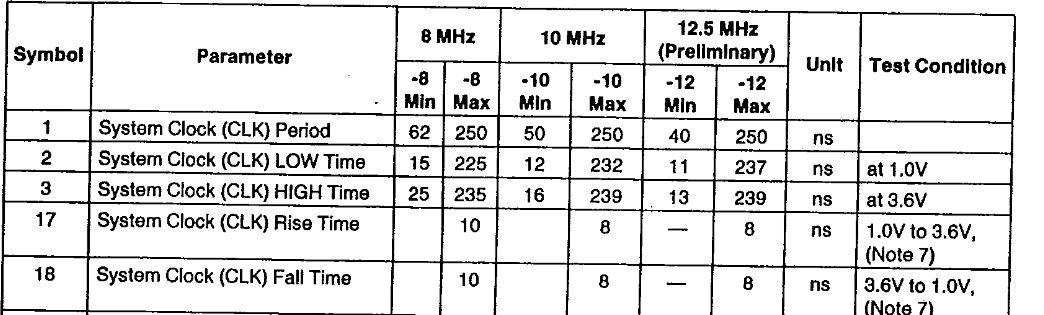
We've got 76800 bytes per image and that's the same as 38400 processor words (it is 16-bit).
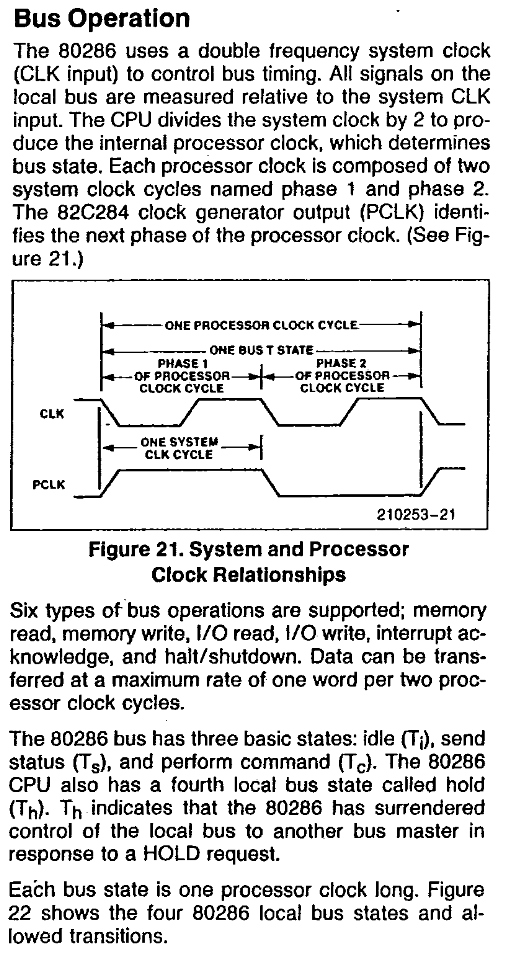
Because data transfers are one word per two processor clock cycles, and a processor clock cycle is two system clock cycles, we've got one word for every 4 system clock cycles. That's 220 nanoseconds per word. Multiply that through and we've got ~8.5 ms to transfer each image into memory, or 25 milliseconds total for the 3 images we need.
We can fit all 3 images into the 1 megabyte of address space allowed in real addressing mode, so we don't need to consider virtual addresses. This is good because virtual addresses would be slower.
In order to calculate the ball's trajectory, we'll need to find it in each image, and then do a curve fit. Let's start with just the first image, because maybe we can do something clever on subsequent images.
We'll also assume that we can do depth perception on each image because we know the size of the ball we are trying to catch. If we didn't have that assumption, we'd want 2 cameras and 2 images per frame.
Most image processing algorithms are O(N) with respect to the size of the image. The constant factor is normally the number of multiplications and additions per pixel. We can simplify here and assume it's like a convolution with a 3x3 kernel, so each pixel is just multiplied 9 times and added 9 times. This is a comical simplification because any image processing for "finding a ball" requires significantly more compute than that.
Let's also assume we can do this using only integer math. If it was floating point, we'd need to use the 80287[1], and we'd pay for additional bus transfers to shuffle the memory to that processor. Also, math operations on the 80287 seem to be about 100-200 clock cycles, whereas our integer add and integer multiply are only 7 and 13 clock cycles respectively.

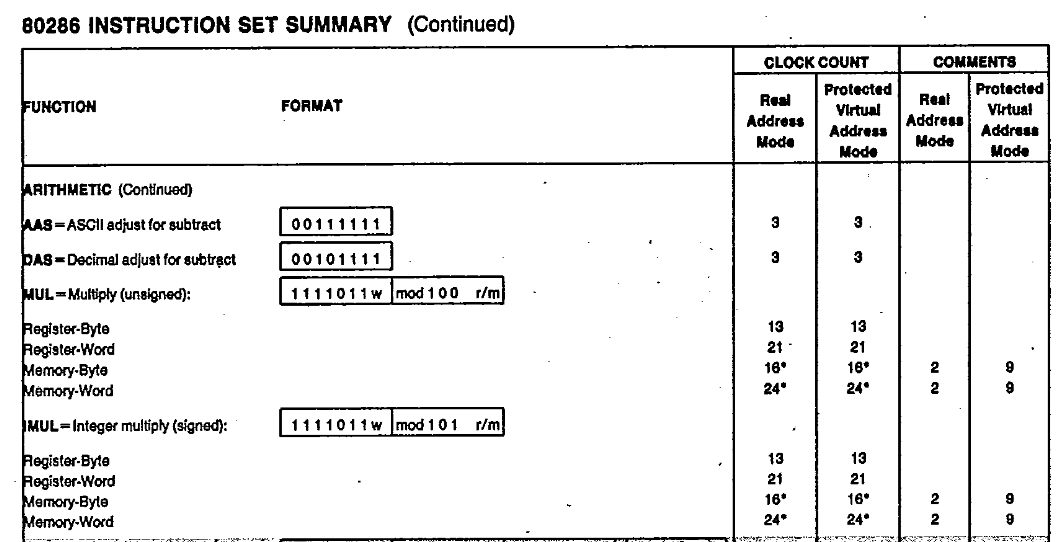
So each pixel is 9 multiplies and 9 additions, which at 12.5 MHz system clock gives us 14.4 microseconds per pixel, or 1.1 seconds per image.
Note that this is incredibly charitable, because I'm ignoring the fact that we only have 8 registers on this processor, so we'll actually be spending a large amount of time on clock cycles just moving data into and out of the registers.
Since we have 3 images, and if we can't do some type of clever reduction after the first image, then we'll have to spend 1.1 seconds on each of them as well. 1.1 seconds is a long enough period of time that I'm not sure you can make any reasonable estimate about where the ball might be in subsequent frames after a single sample, so we are probably stuck.
That means we're looking at 3.3 seconds before we can do that curve fit to avoid the expensive image processing work. Unless the ball is being thrown from very far away (and if it was, it wouldn't be resolvable with this low image resolution), this system is not going to be able to react quickly enough to catch a ball thrown from 10-15 feet away.
Conclusion
Now is the point in this conversation where someone starts suggesting that a superhuman intelligence won't need to look at pixels, or transfer data into memory, and they'll somehow use algorithms that side-step basic facts about how computers work like how many clock cycles a multiplication takes. Or someone suggests that intelligence, as an algorithm, is not like looking at pixels, and reasoning about facts & logic & inferences requires far fewer math operations, so it's not at all comparable, despite the next obvious question being, "what is the time-complexity of general intelligence?"
the rest of the field has come to regard Eliezer as largely correct
It seems possible to me that you're witnessing a selection bias where the part of the field who disagree with Eliezer don't generally bother to engage with him, or with communities around him.
It's possible to agree on ideas like "it is possible to create agent AGI" and "given the right preconditions, AGI could destroy a sizeable fraction of the human race", while at the same time disagreeing with nearly all of Eliezer's beliefs or claims on that same topic.
That in turn would lead to different beliefs for what types of approach will work, which could go a long way towards explaining why so many AI research labs are not pursuing ideas like pivotal acts or other Eliezer-endorsed solutions.
For example, the linked post didn't use this quote when discussing Eliezer's belief that intelligence doesn't require much compute power, but as recently as 2021 (?) he said
Well, if you're a superintelligence, you can probably do human-equivalent human-speed general intelligence on a 286, though it might possibly have less fine motor control, or maybe not, I don't know. [source]
"or maybe not, I don't know" is doing a lot of work in covering that statement.
Then I'm not sure what our disagreement is.
I gave the example of a Kalman filter in my other post. A Kalman filter is similar to recursive Bayesian estimation. It's computationally intensive to run for an arbitrary number of values due to how it scales in complexity. If you have a faster algorithm for doing this, then you can revolutionize the field of autonomous systems + self-driving vehicles + robotics + etc.
The fact that "in principle" information provides value doesn't matter, because the very example you gave of "updating belief networks" is exactly what a Kalman filter captures, and that's what I'm saying is limiting how much information you can realistically handle. At some point I have to say, look, I can reasonably calculate a new world state based on 20 pieces of data. But I can't do it if you ask me to look at 2000 pieces of data, at least not using the same optimal algorithm that I could run for 20 pieces of data. The time-complexity of the algorithm for updating my world state makes it prohibitively expensive to do that.
This really matters. If we pretend that agents can update their world state without incurring a cost of computation, and that it's the same computational cost to update a world state based on 20 measurements as it would take for 2000 measurements, or if we pretend it's only a linear cost and not something like N^2, then yes, you're right, more information is always good.
But if there are computational costs, and they do not scale linearly (like a Kalman filter), then there can be negative value associated with trying to include low quality information in the update of your world state.
It is possible that the doctors are behaving irrationally, but I don't think any of the arguments here prove it. Similar to what mu says on their post here.
Are you ignoring the cost of computation to use that information, as I explained here then?
I was describing a file that would fit your criteria but not be useful. I was explaining in bullet points all of the reasons why that file can't be decoded without external knowledge.
I think that you understood the point though, with your example of data from the Hubble Space Telescope. One caveat: I want to be clear that the file does not have to be all zeroes. All zeroes would violate your criteria that the data cannot be compressed to less than 10% of it's uncompressed size, since all zeroes can be trivially run-length-encoded.
But let's look at this anyway.
You said the file is all zeroes, and it's 1209600 bytes. You also said it's pressure readings in kPa, taken once per second. You then said it's 2^11 x 3^3 x 5^2 x 7 zeroes -- I'm a little bit confused on where this number came from? That number is 9676800, which is larger than the file size in bytes. If I divide by 8, then I get the stated file size, so maybe you're referring to the binary sequence of bits being either 0 or 1, and then on this hardware a byte is 8-bits, and that's how those numbers connect.
In a trivial sense, yes, that is "deriving the structure but not the meaning".
What I really meant was that we would struggle to distinguish between:
- The file is 1209600 separate measurements, each 1-byte, taken by a single pressure sensor.
- The file is 604800 measurements of 1 byte each from 2 redundant pressure sensors.
- The file is 302400 measurements, each 4-bytes, taken by a single pressure sensor.
- The file is 241920 measurements, each a 4-byte timestamp field and a 1-byte pressure sensor value.
Or considering some number of values, with some N-byte width:
- The pressure sensor value is in kPa.
- The pressure sensor value is in psi.
- The pressure sensor value is in atmospheres.
- The pressure sensor value is in lbf.
- The pressure sensor value is in raw counts because it's a direct ADC measurement, so it needs to be converted to the actual pressure via a linear transform.
- We don't know the ADC's reference voltage.
- Or the data sheet for this pressure sensor.
Your questions are good.
1. Is it coherent to "understand the meaning but not the structure"?
Probably not? I guess there's like a weird "gotcha" answer to this question where I could describe what a format tells you, in words, but not show you the format itself, and maybe we could quibble that in such a scenario you'd understand "the meaning but not the structure".
EDIT: I think I've changed my mind on this answer since posting -- yes, there are scenarios where you would understand the meaning of something, but not necessarily the structure of it. A trivial example would be something like a video game save file. You know that some file represents your latest game, and that it allows you to continue and resume from where you left off. You know how that file was created (you pressed "Save Game"), and you know how to use it (press "Load Game", select save game name), but without some amount of reverse-engineering, you don't know the structure of it (assuming that the saved game is not stored as plain text). For non-video-game examples, something like a calibration file produced by some system where the system can both 1.) produce the calibration via some type of self-test or other procedure, and 2.) receive that calibration file. Or some type of system that can be configured by the user, and then you can save that configuration file to disc, so that you can upload it back to the system. Maybe you understand exactly what the configuration file will do, but you never bothered to learn the format of the file itself.
2. Would it be possible to go from "understands the structure but not the meaning" to "understands both" purely through receiving more data?
In general, no. The problem is that you can generate arbitrarily many hypotheses, but if you don't control what data you receive, and there's no interaction possible, then you can't rule out hypotheses. You'd have to just get exceedingly lucky and repeatedly be given more data that is basically designed to be interpreted correctly, i.e. the data, even though it is in a binary format, is self-describing. These formats do exist by the way. It's common for binary formats to include things like length prefixes telling you how many bytes follow some header. That's the type of thing you wouldn't notice with a single piece of data, but you would notice if you had a bunch of examples of data all sharing the same unknown schema.
3. If not , what about through interaction
Yes, this is how we actually reverse-engineer unknown binary formats. Almost always we have some proprietary software that can either produce the format, or read the format, and usually both. We don't have the schema, and for the sake of argument let's say we don't want decompile the software. An example: video game save files that are stored using some ad-hoc binary schema.
What we generally do is start poking known values into the software and seeing what the output file looks like -- like a specific date time, a naming something a certain field, or making sure some value or checkbox is entered in a specific way. Then we permute that entry and see how the file changes. The worse thing would be if almost the entire file changes, which tells us the file is either encrypted OR it's a direct dump of RAM to disk from some data structure with undefined ordering, like a hash map, and the structure is determined by keys which we haven't figured out yet.
Likewise, we do the reverse. We change the format and we plug it back into the software (or an external API, if we're trying to understand how some website works). What we're hoping for is an error message that gives us additional information -- like we change some bytes and now it complains a length is invalid, that's probably related to length. Or if we change some bytes and it says the time cannot be negative, then that might be a time related. Or we change any byte and it rejects the data as being invalid, whoops, probably encrypted again.
The key here is that we have the same problem as question 2 -- we can generate arbitrarily many hypotheses -- but we have a solution now. We can design experiments and iteratively rule out hypotheses, over and over, until we figure out the actual meaning of the format -- not just the structure of it, but what values actually represent.
Again, there are limits. For instance, there are reverse-engineered binary formats where the best we know is that some byte needs to be some constant value for some reason. Maybe it's an internal software version? Who knows! We figured out the structure of that value -- the byte at location 0x806 shall be 203 -- but we don't know the meaning of it.
4. If not, what differences would you expect to observe between a world where I understood the structure but not the meaning of something and a world where I understood both?
Hopefully the above has answered this.
Replying to your other post here:
I don't think this algorithm could decode arbitrary data in a reasonable amount of time. I think it could decode some particularly structured types of data, and I think "fairly unprocessed sensor data from a very large number of nearly identical sensors" is one of those types of data.
My whole point is that "unprocessed sensor data" can be arbitrarily tied to hardware in ways that make it impossible to decode without knowledge of that particular hardware, e.g. ADC reference voltages, datasheets, or calibration tables.
RAW I'd expect is better than a bitmap, assuming no encryption step, on the hypothesis that more data is better.
The opposite. Bitmaps are much easier than RAW formats. A random RAW format, assuming no interaction with the camera hardware or software that can read/write that format, might as well be impossible. E.g. consider the description of a RAW format here. Would you have known that the way the camera hardware works involves pixels that are actually 4 separate color sensors arranged as (in this example) an RGBG grid (called a Bayer filter), and that to calculate the pixel colors for a bitmap you need to interpolate between 4 of those raw color sensors for each color channel on each pixel in the bitmap, and the resulting file size is going to be 3x larger? Or that the size of the output image is not the size of the sensor, so there's dead pixels that need to be ignored during the conversion? Again, this is just describing some specific RAW format -- other RAW formats work differently, because cameras don't all use the same type of color sensor.
The point as I see it is more about whether it's possible with a halfway reasonable amount of compute or whether That Alien Message was completely off-base.
It was completely off-base.