Posts
Comments
We certainly think that abrupt changes of safety properties are very possible! See discussion of how the most pessimistic scenarios may seem optimistic until very powerful systems are created in this post, and also our paper on Predictability and Surprise.
With that said, I think we tend to expect a bit of continuity. Empirically, even the "abrupt changes" we observe with respect to model size tend to take place over order-of-magnitude changes in compute. (There are examples of things like the formation of induction heads where qualitative changes in model properties can happen quite fast over the course of training).
But we certainly wouldn't claim to know this with any confidence, and wouldn't take the possibility of extremely abrupt changes off the table!
Unfortunately, I don't think a detailed discussion of what we regard as safe to publish would be responsible, but I can share how we operate at a procedural level. We don't consider any research area to be blanket safe to publish. Instead, we consider all releases on a case by case basis, weighing expected safety benefit against capabilities/acceleratory risk. In the case of difficult scenarios, we have a formal infohazard review procedure.
[responded to wrong comment!]
how likely does Anthropic think each is? What is the main evidence currently contributing to that world view?
I wouldn't want to give an "official organizational probability distribution", but I think collectively we average out to something closer to "a uniform prior over possibilities" without that much evidence thus far updating us from there. Basically, there are plausible stories and intuitions pointing in lots of directions, and no real empirical evidence which bears on it thus far.
(Obviously, within the company, there's a wide range of views. Some people are very pessimistic. Others are optimistic. We debate this quite a bit internally, and I think that's really positive! But I think there's a broad consensus to take the entire range seriously, including the very pessimistic ones.)
This is pretty distinct from how I think many people here see things – ie. I get the sense that many people assign most of their probability mass to what we call pessimistic scenarios – but I also don't want to give the impression that this means we're taking the pessimistic scenario lightly. If you believe there's a ~33% chance of the pessimistic scenario, that's absolutely terrifying. No potentially catastrophic system should be created without very compelling evidence updating us against this! And of course, the range of scenarios in the intermediate range are also very scary.
How are you actually preparing for near-pessimistic scenarios which "could instead involve channeling our collective efforts towards AI safety research and halting AI progress in the meantime?"
At a very high-level, I think our first goal for most pessimistic scenarios is just to be able to recognize that we're in one! That's very difficult in itself – in some sense, the thing that makes the most pessimistic scenarios pessimistic is that they're so difficult to recognize. So we're working on that.
But before diving into our work on pessimistic scenarios, it's worth noting that – while a non-trivial portion of our research is directed towards pessimistic scenarios – our research is in some ways more invested in optimistic scenarios at the present moment. There are a few reasons for this:
- We can very easily "grab probability mass" in relatively optimistic worlds. From our perspective of assigning non-trivial probability mass to the optimistic worlds, there's enormous opportunity to do work that, say, one might think moves us from a 20% chance of things going well to a 30% chance of things going well. This makes it the most efficient option on the present margin.
(To be clear, we aren't saying that everyone should work on medium difficulty scenarios – an important part of our work is also thinking about pessimistic scenarios – but this perspective is one reason we find working on medium difficulty worlds very compelling.) - We believe we learn a lot from empirically trying the obvious ways to address safety and seeing what happens. My colleague Andy likes to say things like "First we tried the dumbest way to solve alignment (prompting), then we tried the second dumbest thing (fine tuning), then we tried the third dumbest thing…" I think there's a lot to be said for "working our way up the ladder of trying dumb things" and addressing harder and harder scenarios as we do so. Earlier techniques are both building blocks and baselines for further research.
- A crucial part of many pessimistic scenarios is that "easy safety techniques" appear to work, but then fail in subtle/abrupt ways. Having the "easy safety techniques" is a prerequisite for trying to empirically investigate this. (For example, you might want to do mechanistic interpretability on sophisticated RLHF models and try to learn what's going on.) Of course, actually recognizing this will be very hard, as we're about to discuss. But it's worth noting that this is a prerequisite for empirical attempts, and we believe those attempts are worth making, even though we aren't sure they'll succeed!
We also have a lot of work that I might describe as trying to move from optimistic scenarios towards more intermediate scenarios. This includes our process-oriented learning and scalable supervision agendas.
But what are we doing now to address pessimistic scenarios? (Again, remember that our primary goal for pessimistic scenarios is just to recognize that we're in one and generate compelling evidence that can persuade the world.)
- Our work on mechanistic interpretability is deliberately targeted at trying to fill in holes in our portfolio for pessimistic scenarios. We see this as a high-risk, high-reward bet – it may very well not succeed in time to matter, but if it does, it would be a very powerful tool for determining if we were in a pessimistic scenario and providing compelling evidence to the world. See detailed discussion in the post.
- Our Testing for Dangerous Failure Modes (eg. trying to find evidence of things like situational awareness from the outside of models) work is useful in a range of scenarios, but seems helpful in a lot of quite pessimistic ones. I think it's easy to dismiss this work on the basis that, of course, models will just deceive you. But there are a lot of very sophisticated ideas for how to get around this.
- One idea is that models may try to deceive you before they can do so effectively. This could be smaller models, or large models earlier in training. (This could even include detecting hints of deceptive behavior in the logits, before it becomes likely behavior!)
- Another more complex one is to think of treacherous turns as being kind of like a phase change, and to try to introduce control variables that expand the problem to more dimensions and allow you to safely observe treacherous turns. (For example, you might deliberately give a model weaker supervision in order to see if misalignment develops in weaker models, and then study how this changes as a function of both model capacity and supervision strength.)
- A lot of our work in this area involves studying "model organisms" of these failure modes so that we can understand them in models that are much less capable than their overseers.
- Our work on Understanding Generalization also aims to provide tools for understanding why models behave the way they do, which might help us recognize deceptively aligned models.
To be clear, we think pessimistic scenarios are, well, pessimistic and hard! These are our best preliminary attempts at agendas for addressing them, and we expect to change and expand as we learn more. Additionally, as we make progress on the more optimistic scenarios, I expect the number of projects we have targeted on pessimistic scenarios to increase.
I moderately disagree with this? I think most induction heads are at least primarily induction heads (and this points strongly at the underlying attentional features and circuits), although there may be some superposition going on. (I also think that the evidence you're providing is mostly orthogonal to this argument.)
I think if you're uncomfortable with induction heads, previous token heads (especially in larger models) are an even more crisp example of an attentional feature which appears, at least on casual inspection, to typically be monosematnically represented by attention heads. :)
As a meta point – I've left some thoughts below, but in general, I'd rather advance this dialogue by just writing future papers.
(1) The main evidence I have for thinking that induction heads (or previous token heads) are primarily implementing those attentional features is just informally looking at their behavior on lots of random dataset examples. This isn't something I've done super rigorously, but I have a pretty strong sense that this is at least "the main thing".
(2) I think there's an important distinction between "imprecisely articulating a monosemantic feature" and "a neuron/attention head is polysemantic/doing multiple things". For example, suppose I found a neuron and claimed it was a golden retriever detector. Later, it turns out that it's a U-shaped floppy ear detector which fires for several species of dogs. In that situation, I would have misunderstood something – but the misunderstanding isn't about the neuron doing multiple things, it's about having had an incorrect theory of what the thing is.
It seems to me that your post is mostly refining the hypothesis of what the induction heads you are studying are – not showing that they do lots of unrelated things.
(3) I think our paper wasn't very clear about this, but I don't think your refinements of the induction heads was unexpected. (A) Although we thought that the specific induction head in the 2L model we studied only used a single QK composition term to implement a very simple induction pattern, we always thought that induction heads could do things like match [a][b][c]. Please see the below image with a diagram from when we introduced induction heads that shows richer pattern matching, and then text which describes the k-composition for [a][b] as the "minimal way to create an induction head", and gives the QK-composition term to create an [a][b][c] matching case. (B) We also introduced induction heads as a sub-type of copying head, so them doing some general copying is also not very surprising – they're a copying head which is guided by an induction heuristic. (Just as one observes "neuron splitting" creating more and more specific features as one scales a model, I expect we get "attentional feature splitting" creating more and more precise attentional features.)
(3.A) I think it's exciting that you've been clarifying induction heads! I only wanted to bring these clarifications up here because I keep hearing it cited as evidence against the framework paper and against the idea of monosemantic structures we can understand.
(3.B) I should clarify that I do think we misunderstood the induction heads we were studying in the 2L models in the framework paper. This was due to a bug in the computation of low-rank Frobenius norms in a library I wrote. This is on a list of corrections I'm planning to make to our past papers. However, I don't think this reflects our general understanding of induction heads. The model was chosen to be (as we understood it at the time) the simplest case study of attention head composition we could find, not a representative example of induction heads.
(4) I think attention heads can exhibit superposition. The story is probably a bit different than that of normal neurons, but – drawing on intuition from toy models – I'm generally inclined to think: (a) sufficiently important attentional features will be monosemantic, given enough model capacity; (b) given a privileged basis, there's a borderline regime where important features mostly get a dedicated neuron/attention head; (c) this gradually degrades into being highly polysemantic and us not being able to understand things. (See this progression as an example that gives me intuition here.)
It's hard to distinguish "monosemantic" and "slightly polysemantic with a strong primary feature". I think it's perfectly possible that induction heads are in the slightly polysemantic regime.
(5) Without prejudice to the question of "how monosemantic are induction heads?", I do think that "mostly monosemantic" is enough to get many benefits.
(5.A) Background: I presently think of most circuit research as "case studies where we can study circuits without having resolved superposition, to help us build footholds and skills for when we have". Mostly monosemantic is a good proxy in this case.
(5.B) Mostly monosemantic features / attentional features allow us to study what features exist in a model. A good example of this is the SoLU paper – we believe many of the neurons have other features hiding in correlated small activations, but it also seems like it's revealing the most important features to us.
(5.C) Being mostly monosemantic also means that, for circuit analysis, interference with other circuits will be mild. As such, the naive circuit analysis tells you a lot about the general story (weights for other features will be proportionally smaller). For contrast, compare this to a situation where one believes they've found a neuron (say a "divisible by seven" number detector, continuing my analogy above!) and it turns out that actually, that neuron mostly does other things on a broader distribution (and they even cause stronger activations!). Now, I need to be much more worried about my understanding…
Can I summarize your concerns as something like "I'm not sure that looking into the behavior of "real" models on narrow distributions is any better research than just training a small toy model on that narrow distribution and interpreting it?" Or perhaps you think it's slightly better, but not considerably?
Between the two, I might actually prefer training a toy model on a narrow distribution! But it depends a lot on exactly how the analysis is done and what lessons one wants to draw from it.
Real language models seem to make extensive use of superposition. I expect there to be lots of circuits superimposed with the one you're studying, and I worry that studying it on a narrow distribution may give a misleading impression – as soon as you move to a broader distribution, overlapping features and circuits which you previously missed may activate, and your understanding may in fact be misleading.
On the other hand, for a model just trained on a toy task, I think your understanding is likely closer to the truth of what's going on in that model. If you're studying it over the whole training distribution, features either aren't in superposition (there's so much free capacity in most of these models this seem possible!) or else they'll be part of the unexplained loss, in your language. So choosing to use a toy model is just a question of what that model teaches you about real models (for example, you've kind of side-stepped superposition, and it's also unclear to what extent the features and circuits in a toy model represent the larger model). But it seems much clearer what is true, and it also seems much clearer that these limitations exist.
Regarding the more general question of "how much should interpretability make reference to the data distribution?", here are a few thoughts:
Firstly, I think we should obviously make use of the data distribution to some extent (and much of my work has done so!). If you're trying to reverse engineer a regular computer program, it's extremely useful to have traces of that program running. So too with neural networks!
However, the fundamental thing I care about is understanding whether models will be safe off-distribution, so an understanding which is tied to a specific distribution – and especially to a narrow distribution – is less clear in how it advances my core goals. Explanations which hold narrowly but break off distribution are one of my biggest worries for interpretability, and a big part of why I've taken the mechanistic approach rather than picking low-hanging fruit in correlational interpretability. I'm much more worried about explanations only holding on narrow distributions than I am about incomplete global explanations -- this is probably a significant implicit motivator of my research taste. (Caveat: I'm reluctantly okay with certain aspects of understanding being built on the entire training distribution when we have a compelling theoretical argument for why this captures everything and will generalize.)
Let's return to my example of protein binding affinities from my other comment and imagine two different descriptions of the situation:
- The "global story" – We have a table of binding affinities. When one protein has a much higher binding affinity than the other, it outcompetes it.
- The "on distribution story" – We have a table of proteins which "block" other proteins in practice.
The global story is a kind of "unbiased account of the mechanism" which requires us to think through more possibilities, but can predict weird out of distribution behavior. On the other hand, the "on distribution story" highlights the aspects of the mechanism which are important in practice, but might fail in weird situations.
But what do we want from the on-distribution analysis?
One easy answer is that we just want to use it to make mechanistic understanding easier. Neural networks are immensely complicated computer programs. It seems to me that even understanding small neural networks is probably comparable to something like "reverse engineer a compiled linux kernel knowing nothing about operating systems". It's very helpful to have examples of it running to kind of bootstrap your analysis.
But I think there's something deeper which you're getting at, which I might articulate as distinguishing which aspects of a neural network's mechanistic behavior are "deliberate or useful" and which are "bugs or quirks". For example, in the framework paper we highlight some skip-trigrams which appear to be bugs:
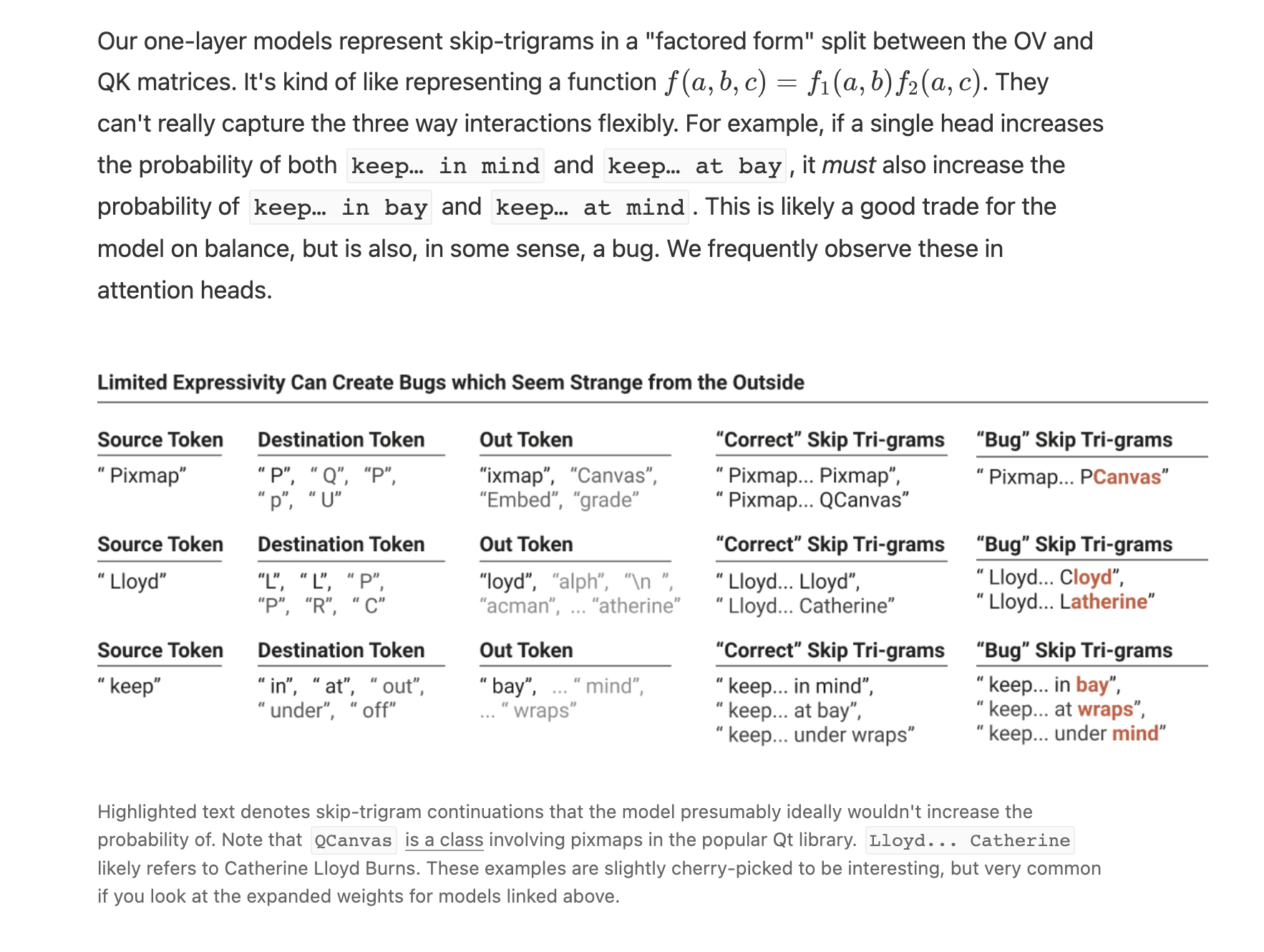
Of course, distinguishing between "correct" skip tri-grams and "bug" skip-trigrams required our judgment based on understanding the domain. In an impartial account of the mechanism, they're all valid skip-trigrams the model implements. It's only with reference to the training distribution or some other external distribution or task that we can think of some as "correct" and others as "bugs".
By more explicitly analyzing on a distribution, one might automate this kind of differentiation. And possibly, one might just ignore these (especially to the extent that other heads or the bigrams can compensate in practice!). This could make a simpler "explanation" at the cost of not generalizing to other distributions.
(In this particular case, I suspect there might actually be a more beautiful, non-distribution specific story to be told in terms of superposition. But that's another topic.)
One interesting thing this suggests is that a "global story" should be able to be "bound" to a distribution to create an in-distribution account. For example, if one has a list of binding affinities for different chemicals, and knows that only a certain subset will be present at the same time, one can produce a summary of which will block each other.
While we're on the topic, it's perhaps useful to more directly describe my concerns about distribution-specific understanding of models, and especially narrow-distribution understanding of the kind a lot of work building Causal Scrubbing seems to be focusing on.
It seems to me that this kind of work is very vulnerable to producing fragile understandings of models which break on a wider distribution due to interpretability illusion type issues.
As one concrete example from my own experience, in the early days of Anthropic I looked into how language models perform arithmetic by only looking at model behavior only on arithmetic expressions. Immediately, lots of interesting patterns popped out and some interesting partial stories began to emerge. However, as soon as I returned to the full training distribution, the story fell apart. All the components I thought did something were doing other things – often primarily doing other things – on the full distribution. Of course, this was a very casual investigation and not anywhere near as rigorous as the causal scrubbing work. But while I'm sure there were ways my understanding on distribution was incomplete, I'm 100x more worried about the fact that it was clearly misleading about the general situation. (My strong suspicion is that there is a very nice story here, but it's deeply intertwined with superposition and we can't understand it without addressing that.)
With that said, I'm very excited for people to be taking different approaches to these problems. My concerns could be misplaced! I definitely think that restricting to a narrow distribution allows one to make a lot of progress on that type of understanding.
Thanks for writing this up. It seems like a valuable contribution to our understanding of one-layer transformers. I particularly like your toy example – it's a good demonstration of how more complicated behavior can occur here.
For what it's worth, I understand this behavior as competition between skip-trigrams. We introduce "skip-trigrams" as a way to think of pairs of entries in the OV and QK-circuit matrices. The QK-circuit describes how much the attention head wants to attend to a given token in the attention softmax and implement a particular skip-trigram. The phenomenon you describe occurs when there are multiple skip-trigrams present with different QK-circuit values.
An analogy I find useful for thinking about this is protein binding affinity in molecular biology. (I don't know much about molecular biology – hopefully experts can forgive me if my analogy is naive!) Proteins have a propensity to bind to other proteins, just as attention heads have a propensity to attend between specific tokens and implement skip-trigrams. However, fully understanding the behavior requires remembering that when one protein has a higher binding affinity than another, it can "block" binding. This doesn't mean that it's incorrect to understand proteins as having binding affinity! Nor does it mean that skip-trigrams are the wrong way to understand one-layer models. It just means that in thinking about proteins (or skip-trigrams) one wants to keep in mind the possibility of second order interactions.
I do think your example is very clarifying about the kind of second order interactions that can occur with skip-trigrams! While I definitely knew "skip-trigrams compete for attention", I hadn't realized it could give rise to this behavior.
With that said, I get the sense that maybe you might have understood us to be making a stronger claim about skip-trigrams being independent which we didn't intend. I'm sorry for any confusion here. We do talk about "independent skip-trigram models". Here "independent" is modifying "models" – it's referring to the fact that there are multiple attention heads implementing independent skip-trigram models. (This might seem trivial now, but we had spent an entire section on this point because many people didn't realize this from the original concatenated version of the transformer equations.) Then "skip-trigram" is referring to the fact that the natural units of one-layer models are triplets of tokens. Although our introduction and section introduce this without more context, our actual discussion of skip-trigrams keeps referring back to the OV and QK-circuits, which is the mathematical model they're trying to provide a language for talking about.
I've been meaning to add a number of correctives and clarifications to our papers – this is on the list, and we'll link to your example!
(I'll comment on your more general thesis regarding understanding models with respect to a specific distribution in a separate comment.)
I'm curious how you'd define memorisation? To me, I'd actually count this as the model learning features ...
Qualitatively, when I discuss "memorization" in language models, I'm primarily referring to the phenomenon of languages models producing long quotes verbatim if primed with a certain start. I mean it as a more neutral term than overfitting.
Mechanistically, the simplest version I imagine is a feature which activates when the preceding N tokens match a particular pattern, and predicts a specific N+1 token. Such a feature is analogous to the "single data point features" in this paper. In practice, I expect you can have the same feature also make predictions about the N+2, N+3, etc tokens via attention heads.
This is quite different from a normal feature in the fact that it's matching a very specific, exact pattern.
a bunch of examples will contain the Bible verse as a substring, and so there's a non-trivial probability that any input contains it, so this is a genuine property of the data distribution.
Agreed! This is why I'm describing it as "memorization" (which, again, I mean more neutrally than overfitting in the context of LLMs) and highlight that it really does seem like language models morally should do this.
Although there's also lots of SEO spam that language models memorize because it's repeated which one might think of as overfitting, even though they're a property of the training distribution.
In this toy model, is it really the case that the datapoint feature solutions are "more memorizing, less generalizing" than the axis-aligned feature solutions? I don't feel totally convinced of this.
Well, empirically in this setup, (1) does generalize and get a lower test loss than (2). In fact, it's the only version that does better than random. 🙂
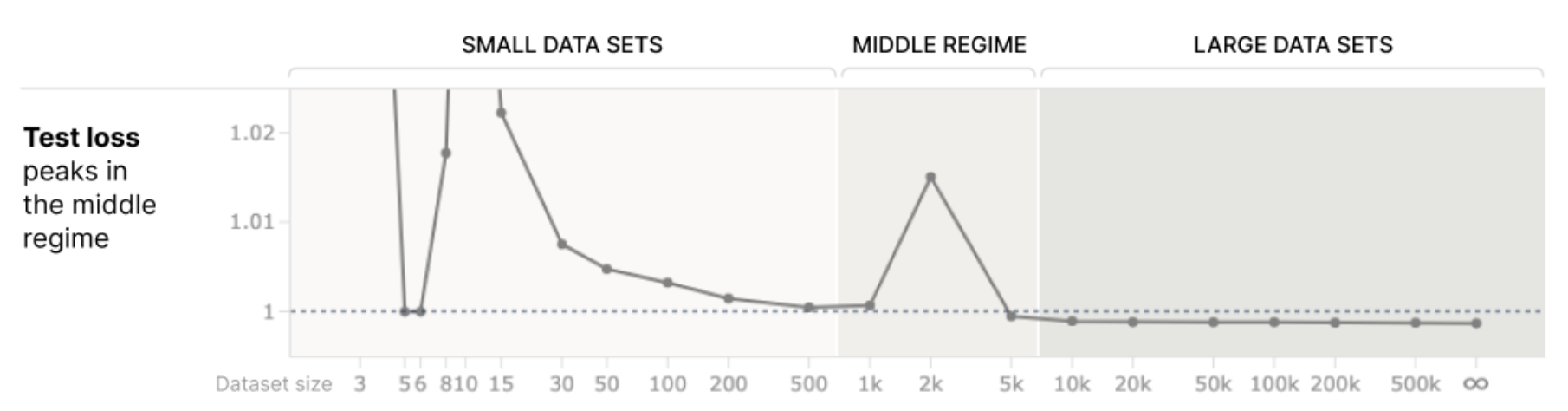
But I think what you're maybe saying is that from the neural network's perspective, (2) is a very reasonable hypothesis when T < N, regardless of what is true in this specific setup. And you could perhaps imagine other data generating processes which would look similar for small data sets, but generalize differently. I think there's something to that, and it depends a lot on your intuitions about what natural data is like.
Some important intuitions for me are:
- Many natural language features are extremely sparse. For example, it seems likely LLMs probably have features for particular people, for particular street intersections, for specific restaurants... Each of these features is very very rarely occurring (many are probably present less than 1 in 10 million tokens).
- Simultaneously, there are an enormous number of features (see above!).
- While the datasets aren't actually small, repeated data points effectively make many data points behave like we're in the small data regime (see Adam's repeated data experiment).
Thus, my intuition is that something directionally like this setup -- having a large number of extremely sparse features -- and then studying how representations change with dataset size is quite relevant. But that's all just based on intuition!
(By the way, I think there is a very deep observations about the duality of (1) vs (2) and T<N. See the observations about duality in https://arxiv.org/pdf/2210.16859.pdf )
I feel pretty confused, but my overall view is that many of the routes I currently feel are most promising don't require solving superposition.
It seems quite plausible there might be ways to solve mechanistic interpretability which frame things differently. However, I presently expect that they'll need to do something which is equivalent to solving superposition, even if they don't solve it explicitly. (I don't fully understand your perspective, so it's possible I'm misunderstanding something though!)
To give a concrete example (although this is easier than what I actually envision), let's consider this model from Adam Jermyn's repeated data extension of our paper:
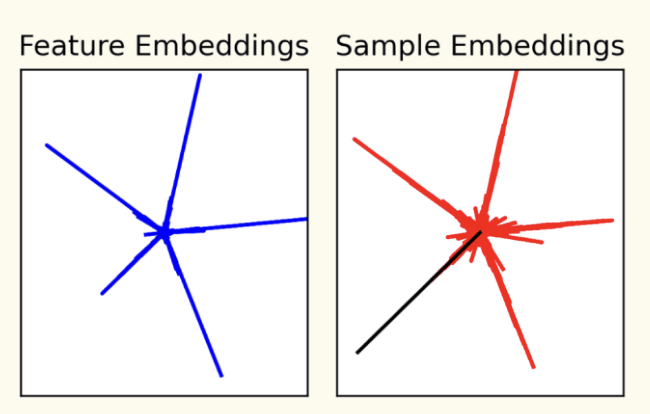
If you want to know whether the model is "generalizing" rather than "triggering a special case" you need to distinguish the "single data point feature" direction from normal linear combinations of features. Now, it happens to be the case that the specific geometry of the 2D case we're visualizing here means that isn't too hard. But we need to solve this in general. (I'm imagining this as a proxy for a model which has one "special case backdoor/evil feature" in superposition with lots of benign features. We need to know if the "backdoor/evil feature" activated rather than an unusual combination of normal features.)
Of course, there may be ways to distinguish this without the language of features and superposition. Maybe those are even better framings! But if you can, it seems to me that you should then be able to backtrack that solution into a sparse coding solution (if you know whether a feature has fired, it's now easy to learn the true sparse code!). So it seems to me that you end up having done something equivalent.
Again, all of these comments are without really understanding your view of how these problems might be solved. It's very possible I'm missing something.
Sorry for not noticing this earlier. I'm not very active on LessWrong/AF. In case it's still helpful, a couple of thoughts...
Firstly, I think people shouldn't take this graph too seriously! I made it for a talk in ~2017, I think and even then it was intended as a vague intuition, not something I was confident in. I do occasionally gesture at it as a possible intuition, but it's just a vague idea which may or may not be true.
I do think there's some kind of empirical trend where models in some cases become harder to understand and then easier. For example:
- An MNIST model without a hidden layer (ie. a softmax generalized linear regression model) is easy to understand.
- An MNIST model with a single hidden layer is very hard to understand.
- Large convolutional MNIST/CIFAR models are perhaps slightly easier (you sometimes get nice convolutional filters).
- AlexNet is noticeably more interpretable. You start to see quite a few human interpretable neurons.
- InceptionV1 seems noticeably more interpretable still.
These are all qualitative observations / not rigorous / not systematic.
So what is going on? I think there are several hypotheses:
- Confused abstractions / Modeling Limitations - Weak models can't express the true abstractions, so they use a mixture of bad heuristics that are hard to reason about and much more complicated than the right thing. This is the original idea behind this curve.
- Overfitting - Perhaps it has less to do with the models and more to do with the data. See this paper where overfitting seems to make features less interpretable. So ImageNet models may be more interpretable because the dataset is harder and there's less extreme overfitting.
- Superposition - It may be that apparent interpretability is actually all a result of superposition. For some reason, the later models have less superposition. For example, there are cases where larger models (with more neurons) may store less features in superposition.
- Overfitting - Superposition Interaction - There's reason to believe that overfitting may heavily exploit superposition (overfit features detect very specific cases and so are very sparse, which is ideal for superposition). Thus, models which are overfit may be difficult to interpret, not because overfit features are intrinsically uninterpretable but because they cause superposition.
- Other Interaction Effects - It may be that there are other interactions between the above arguments. For example, perhaps modeling limitations cause the model to represent weird heuristics, which then more heavily exploit superpositition.
- Idiosyncrasies of Vision - It may be that the observations which motivated this curve are idiosyncratic to vision models, or maybe just the models I was studying.
I suspect it's a mix of quite a few of these.
In the case of language models, I think superposition is really the driving force and is quite different from the vision case (language model features seem to typically be much sparser than vision model ones).
This is a good summary of our results, but just to try to express a bit more clearly why you might care...
I think there are presently two striking facts about overfitting and mechanistic interpretability:
(1) The successes of mechanistic interpretability have thus far tended to focus on circuits which seem to describe clean, generalizing algorithms which one might think of as the "non-overfitting parts of neural networks". We don't really know what "overfitting mechanistically is", and you could imagine a world where it's so fundamentally messy we just can't understand it!
(2) There's evidence that more overfit neural networks are harder to understand.
A pessimistic interpretation of this could be something like: Overfitting is fundamentally a messy kind of computation we won't ever cleanly understand. We're dealing with pathological models/circuits, and if we want to understand neural networks, we need to create non-overfit models.
In the case of vision, that might seem kind of sad but not horrible: you could imagine creating larger and larger datasets that reduce overfitting. ImageNet models are more interpretable than MNIST ones and perhaps that's why. But language models seem like they morally should memorize some data points. Language models should recite the US constitution and Shakespeare and the Bible. So we'd really like to be able to understand what's going on.
The naive mechanistic hypothesis for memorization/overfitting is to create features, represented by neurons, which correspond to particular data points. But there's a number of problems with this:
- It seems incredibly inefficient to have neurons represent data points. You probably want to memorize lots of data points once you start overfitting!
- We don't seem to observe neurons that do this -- wouldn't it be obvious if they existed?
The obvious response to that is "perhaps it's occurring in superposition."
So how does this relate to our paper?
Firstly, we have an example of overfitting -- in a problem which wasn't specifically tuned for overfitting / memorization -- which from a naive perspective looks horribly messy and complicated but turns out to be very simple and clean. Although it's a toy problem, that's very promising!
Secondly, what we observe is exactly the naive hypothesis + superposition. And in retrospect this makes a lot of sense! Memorization is the ideal case for something like superposition. Definitionally, a single data point feature is the most sparse possible feature you can have.
Thirdly, Adam Jermyn's extension to repeated data shows that "single data point features" and "generalizing features" can co-occur.
The nice double descent phase change is really just the cherry on the cake. The important thing is having these two regimes where we represent data points vs features.
There's one other reason you might care about this: it potentially has bearing on mechanistic anomaly detection.
Perhaps the clearest example of this is Adam Jermyn's follow up with repeated data. Here, we have a model with both "normal mechanisms" and "hard coded special cases which rarely activate". And distinguishing them would be very hard if one didn't understand the superposition structure!
Our experiment with extending this to MNIST, although obviously also very much a toy problem, might be interpreted as detecting "memorized training data points" which the model does not use its normal generalizing machinery for, but instead has hard coded special cases. This is a kind of mechanistic anomaly detection, albeit within the training set. (But I kind of think that alarming machinery must form somewhere on the training set.)
One nice thing about these examples is that they start to give a concrete picture of what mechanistic anomaly detection might look like. Of course, I don't mean to suggest that all anomalies would look like this. But as someone who really values concrete examples, I find this useful in my thinking.
These results also suggest that if superposition is widespread, mechanistic anomaly detection will require solving superposition. My present guess (although very uncertain) is that superposition is the hardest problem in mechanistic interpretability. So this makes me think that anomaly detection likely isn't a significantly easier problem than mechanistic interpretability as a whole.
All of these thoughts are very uncertain of course.
See also the Curve Detectors paper for a very narrow example of this (https://distill.pub/2020/circuits/curve-detectors/#dataset-analysis -- a straight line on a log prob plot indicates exponential tails).
I believe the phenomena of neurons often having activation distributions with exponential tails was first informally observed by Brice Menard.
I just stumbled on this post and wanted to note that very closely related ideas are sometimes discussed in interpretability under the names "universality" or "convergent learning": https://distill.pub/2020/circuits/zoom-in/#claim-3
In fact, not only do the same features form across different neural networks, but we actually observe the same circuits as well (eg. https://distill.pub/2020/circuits/frequency-edges/#universality ).
Well, goodness, it's really impressive (and touching) that someone absorbed the content of our paper and made a video with thoughts building on it so quickly! It took me a lot longer to understand these ideas.
I'm trying to not work over the holidays, so I'll restrict myself to a few very quick remarks:
-
There's a bunch of stuff buried in the paper's appendix which you might find interesting, especially the "additional intuition" notes on MLP layers, convolutional-like structure, and bottleneck activations. A lot of it is quite closely related to the things you talked about in your video.
-
You might be interested in work in the original circuits thread, which focused on reverse engineering convolutional networks with ReLU neurons. Curve Detectors and Curve Circuits are an deep treatment of one case and might shed light on some of the ideas you were thinking about. (For example, you discussed what we call "dataset examples" for a bit.)
-
LayerNorm in transformers is slightly different from what you describe. There are no interactions between tokens. This is actually the reason LayerNorm is preferred: in autoregressive transformers, one needs to be paranoid about avoiding information leakage from future tokens, and normalization across tokens becomes very complicated as a result, leading to a preference for normalization approaches that are per-token. In any case, there are some minor flow through effects from this to other things you say.
-
Most transformers prefer GeLU neurons to ReLU neurons.
-
In general, I'd recommend pushing linearization back until you hit a privileged basis (either a previous MLP layer or the input tokens) rather than the residual stream. My guess is that's the most interpretable formulation of things. It turns out you can always do this.
I think there's another important idea that you're getting close to and I wanted to remark on:
- Just as we can linearize the attention layers by freezing the attention patterns, we can linearize a ReLU MLP layer by freezing the "mask" (what you call the referendum).
- The issue is that to really leverage this for understanding, one probably needs to understand the information they froze. For example, for attention layers one still needs to look at the attention patterns, and figure out why they attend where they do (the QK circuit) or at least have an empirical theory (eg. "induction heads attend to previous copies shifted one forward").
- Linearizing MLP layers requires you to freeze way more information than attention layers, which makes it harder to "hold in your head." Additionally, once you understand the information you've frozen, you have a theory of the neurons and could proceed via the original circuit-style approach to MLP neurons. In any case, it's exciting to see other people thinking about this stuff. Happy holidays, and good luck if you're thinking about this more!
Thanks for making that distinction, Steve. I think the reason things might sounds muddled is that many people expect that (1) will drive (2).
Why might one expect (1) to cause (2)? One way to think about it is that, right now, most ML experiments optimistically given 1-2 bits of feedback to the researcher, in the form of whether their loss went up or down from a baseline. If we understand the resulting model, however, that could produce orders of magnitude more meaningful feedback about each experiment. As a concrete example, in InceptionV1, there are a cluster of neurons responsible for detecting 3D curvature and geometry that all form together in one very specific place. It's pretty suggestive that, if you wanted your model to have a better understanding of 3D curvature, you could add neurons there. So that's an example where richer feedback could, hypothetically, guide you.
Of course, it's not actually clear how helpful it is! We spent a bunch of time thinking about the model and concluded "maybe it would be especially useful on a particular dimension to add neurons here." Meanwhile, someone else just went ahead and randomly added a bunch of new layers and tried a dozen other architectural tweaks, producing much better results. This is what I mean about it actually being really hard to outcompete the present ML approach.
There's another important link between (1) and (2). Last year, I interviewed a number of ML researchers I respect at leading groups about what would make them care about interpretability. Almost uniformly, the answer was that they wanted interpretability to give them actionable steps for improving their model. This has led me to believe that interpretability will accelerate a lot if it can help with (2), but that's also the point at which it helps capabilities.
Evan, thank you for writing this up! I think this is a pretty accurate description of my present views, and I really appreciate you taking the time to capture and distill them. :)
I’ve signed up for AF and will check comments on this post occasionally. I think some other members of Clarity are planning to so as well. So everyone should feel invited to ask us questions.
One thing I wanted to emphasize is that, to the extent these views seem intellectually novel to members of the alignment community, I think it’s more accurate to attribute the novelty to a separate intellectual community loosely clustered around Distill than to me specifically. My views are deeply informed by the thinking of other members of the Clarity team and our friends at other institutions. To give just one example, the idea presented here as a “microscope AI” is deeply influenced by Shan Carter and Michael Nielsen’s thinking, and the actual term was coined by Nick Cammarata.
To be clear, not everyone in this community would agree with my views, especially as they relate to safety and strategic considerations! So I shouldn’t be taken as speaking on behalf of this cluster, but rather as articulating a single point of view within it.
Subscribed! Thanks for the handy feature.
One thing I'd add, in addition to Evan's comments, is that the present ML paradigm and Neural Architecture Search are formidable competitors. It feels like there’s a big gap in effectiveness, where we’d need to make lots of progress for “principled model design” to be competitive with them in a serious way. The gap causes me to believe that we’ll have (and already have had) significant returns on interpretability before we see capabilities acceleration. If it felt like interpretability was accelerating capabilities on the present margin, I’d be a bit more cautious about this type of argumentation.
(To date, I think the best candidate for a capabilities success case from this approach is Deconvolution and Checkerboard Artifacts. I think it’s striking that the success was less about improving a traditional benchmark, and more about getting models to do what we intend.)
I think that’s a fair characterization of my optimism.
I think the classic response to me is “Sure, you’re making progress on understanding vision models, but models with X are different and your approach won’t work!” Some common values of X are not having visual features, recurrence, RL, planning, really large size, and language-based. I think that this is a pretty reasonable concern (more so for some Xs than others). Certainly, one can imagine worlds where this line of work hits a wall and ends up not helping with more powerful systems. However, I would offer a small consideration in the other direction: In 2013 I think no one thought we’d make this much progress on understanding vision models, and in fact many people thought really understanding them was impossible. So I feel like there’s some risk of distorting our evaluation of tractability by moving the goal post in these conversations.
I’m not surprised by other people feeling like they have less traction. I feel like the first three or so years I spent trying to understand the internals neural networks involved a lot of false starts with approaches that ended up being dead ends (eg. visualizing really small networks, or focusing on dimensionality reduction). DeepDream was very exciting, but it retrospect I feel like it took me another two or so years to really digest what it meant and how one could really use it as a scientific tool. And this is with the benefit of amazing collaborators and multiple very supportive environments.
One final thing I’d add is that, if I’m honest, I’m probably more motivated by aesthetics than optimism. I’ve spent almost seven years obsessed with the question of what goes on inside neural networks and I find the crazy partial answers we learn every year tantalizingly beautiful. I think this is pretty normal for early research directions; Kuhn talks about it a fair amount in The Structure of Scientific Revolutions.
I'm curious what's Chris's best guess (or anyone else's) about where to place AlphaGo Zero on that diagram
Without the ability to poke around at AlphaGo -- and a lot of time to invest in doing so -- I can only engage in wild speculation. It seems like it must have abstractions that human Go players don’t have or anticipate. This is true of even vanilla vision models before you invest lots of time in understanding them (I've learned more than I ever needed to about useful features for distinguishing dog species from ImageNet models).
But I’d hope the abstractions are in a regime where, with effort, humans can understand them. This is what I expect the slope downwards as we move towards “alien abstractions” to look like: we’ll see abstractions that are extremely useful if you can internalize them, but take more and more effort to understand.
Is there an implicit assumption here that RL agents are generally more dangerous than models that are trained with (un)supervised learning?
Yes, I believe that RL agents have a much wider range of accident concerns than supervised / unsupervised models.
Later the OP contrasts microscopes with oracles, so perhaps Chris interprets a microscope as a model that is smaller, or otherwise somehow restricted, s.t. we know it's safe?
Gurkenglas provided a very eloquent description that matches why I believe this. I’ll continue discussion of this in that thread. :)