Reviews of “Is power-seeking AI an existential risk?”
post by Joe Carlsmith (joekc) · 2021-12-16T20:48:26.808Z · LW · GW · 20 commentsContents
20 comments
(Edited 10/14/22 to add Lifland review. Edited 7/10/23 to add Levinstein review. Edited 10/18/23 to add superforecaster reviews.)
Open Philanthropy solicited reviews of my draft report “Is power-seeking AI an existential risk?” (Edit: arXiv version here) from various sources. Where the reviewers allowed us to make their comments public in this format, links to these comments are below, along with some responses from me in blue.
- Leopold Aschenbrenner
- Ben Garfinkel
- Daniel Kokotajlo
- Ben Levinstein
- Eli Lifland
- Neel Nanda
- Nate Soares [LW · GW]
- Christian Tarsney
- David Thorstad
- David Wallace
- Anonymous 1 (software engineer at AI research team)
- Anonymous 2 (academic computer scientist)
The table below (spreadsheet link here) summarizes each reviewer’s probabilities and key objections.
An academic economist focused on AI also provided a review, but they declined to make it public in this format.
Added 10/18/23: With funding from Open Philanthropy, Good Judgment also solicited reviews and forecasts from 21 superforecasters regarding the report -- see here for a summary of the results. These superforecasters completed a survey very similar to the one completed by the other reviewers, except with an additional question (see footnote) about the "multiple stage fallacy."[1] Their aggregated medians were:
Good Judgment has also prepared more detailed summaries of superforecaster comments and forecasts here (re: my report) and here (re: the other timelines and X-risk questions). See here [LW · GW] for some brief reflections on these results, and here for a public spreadsheet with the individual superforecasters numbers and reviews (also screenshot-ed below).
- ^
The new question (included in the final section of the survey) was:
"One concern about the estimation method in the report is that the multi-premise structure biases towards lower numbers (this is sometimes called the "multi-stage fallacy"; see also Soares here [LW · GW]). For example, forecasters might fail to adequately condition on all of the previous premises being true and to account for their correlations, or they might be biased away from assigning suitably extreme probabilities to individual premises.
When you multiply through your probabilities on the individual premises, does your estimate differ significantly from the probability you would've given to "existential catastrophe by 2070 from worlds where all of 1-6 are true" when estimating it directly? If so, in what direction?"
20 comments
Comments sorted by top scores.
comment by Vermillion (VermillionStuka) · 2021-12-17T09:04:12.606Z · LW(p) · GW(p)
I guffawed when I saw Thorstads Overall ~P Doom 0.00002%, really? And some of those other probabilities weren't much better.
Calibrate people, if you haven’t done it before do it now, here’s a handy link: https://www.openphilanthropy.org/calibration
Replies from: daniel-kokotajlo↑ comment by Daniel Kokotajlo (daniel-kokotajlo) · 2021-12-17T19:36:34.891Z · LW(p) · GW(p)
I feel like it's important to say that there's nothing wrong in principle with having extremely high or extremely low credences in things. In order for us to have credences that sum to 1 over millions of distinct possibilities, we will necessarily need to have extremely low/high credences in some propositions.
That said, yeah, I do think some of the numbers on this spreadsheet are pretty ridiculous.
Replies from: Telofy↑ comment by Dawn Drescher (Telofy) · 2021-12-24T13:54:13.902Z · LW(p) · GW(p)
Maybe one should be more hesitant to assign very low probabilities to factors in a conjunction than very high probabilities because the extreme of 100% probability should not be more controversial than omitting a factor.
With a bit of creativity someone could probably come up with dozens of verisimilar additional factor along the lines of “and false vacuum decay hasn’t happened yet.” If we then have to be humble and assign (say) no more than 99% to each of those, it just takes 10 noisy factors to bias the estimate down to ~ 90%.
In this case I went with Nate’s approach and merged the last three factors. None of them seemed silly to me, but I felt like splitting them up didn’t help my intuitions.
comment by Daniel Kokotajlo (daniel-kokotajlo) · 2021-12-17T02:24:47.423Z · LW(p) · GW(p)
Here's a visualization of the probability distribution implied by Joe's credences (hope I did the math right):
I'm not going anywhere in particular with this, I just had fun making it & found it helpful so I thought I'd share. Also, I commissioned a web app so that I and anyone else can easily make things like this in the future:
Probability Mass App (daniel-kokotajlo.vercel.app)
(Thanks to Bhuvan Singla from Bountied Rationality for building it! Bug reports & feature requests welcome!)
↑ comment by Daniel Kokotajlo (daniel-kokotajlo) · 2021-12-17T18:47:00.609Z · LW(p) · GW(p)
Here's what my distribution would be if I got it by multiplying all the numbers in the six stages. (My real distribution is somewhat more pessimistic, for reasons relating to the multiple-stage fallacy and which I laid out in my review.)
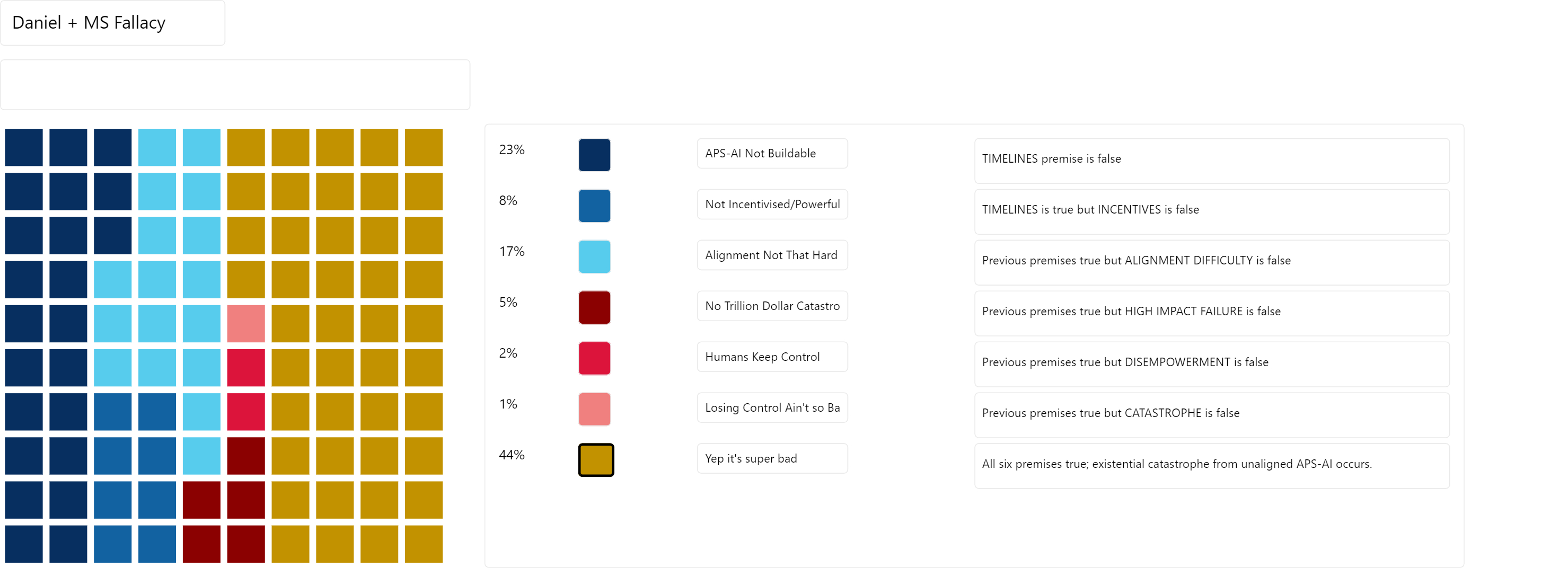
comment by Daniel Kokotajlo (daniel-kokotajlo) · 2021-12-17T01:28:04.122Z · LW(p) · GW(p)
Thanks a ton for the thoughtful and detailed engagement! Below follows my reply to your reply to my spiel about timelines in my review:
I am sad that "Fun with +12 OOMs of compute [LW · GW]" came across as pumping the intuition "wow a trillion is a lot," because I don't have that intuition at all. I wrote the post to illustrate what +12 OOMs of compute might look like in practice, precisely because a trillion doesn't feel like a lot to me; it doesn't feel like anything, it's just a number.
I didn't devote much (any?) time in the post to actually arguing that OmegaStar et al would be transformative. I just left it as an intuition pump. But the actual arguments do exist, and they are roughly of the form "Look at the empirical scaling trends we see today; now imagine scaling these systems up to OmegaStar, Amp(GPT-7), etc.; we can say various things about what these scaled-up systems would be capable of, simply by drawing straight lines on graphs, and already it's looking pretty impressive/scary/"fun," and then moreover qualitatively there are novel phenomena like "transfer learning" and "generalization" and "few-shot-learning" which have been kicking in when we scale stuff up (see: GPT series) and so we have reason to think that these phenomena will intensify, and be joined by additional novel phenomena, as we scale up... all of which should lead to a broader and more impressive suite of capabilities than we'd get from trend extrapolation on existing metrics alone."
I do think I haven't spelled out these arguments anywhere (even to my own satisfaction) and it's important for me to do so; maybe I am overrating them. I'll put it on my todo list.
I think my methodology would perform fine if we applied it in the past, because we wouldn't have scaling trends to extrapolate. Besides, we wouldn't know about transfer learning, generalization, etc. and a whole host of other things. If I sent a description of Amp(GPT-7) back to 2010 and asked leading AI experts to comment on what it would be capable of, they would say that it probably wouldn't even be able to speak grammatically correct English. They might even be confused about why I was going on and on about how big it was, since they wouldn't know about the scaling properties of language models in particular or indeed of neural nets in general.
Moreover, in the past there wasn't a huge scale up of compute expenditure happening. (Well, not anytime in the past except the last 10 years.) That's a separate reason why this methodology would not have given short timelines in the past.
I can imagine in 2015 this methodology starting to give short timelines -- because by then it was clear that people were starting to make bigger and bigger models and (if one was super savvy) one might have figured out that making them bigger was making them better. But definitely prior to 2010, the situation would have been: Compute for AI training is proceeding with Moore's Law, i.e. very slowly; even if (somehow) we were convinced that there was a 80% chance that +12 OOMs from 2010-levels would be enough for TAI (again, I think we wouldn't have been, without the scaling laws and whatnot) we would have expected to cross maybe 1 or 2 of those OOMs per decade, not 5 or 6!
Oh yeah and then there's the human-brain-size thing. That also is a reason to think this methodology would not have led us astray in the past, because it is a way in which the 2020's are a priori unusually likely to contain exciting AI developments. (Technically it's not the 2020's we are talking about here, it's the range between around 10^23 and 10^26 FLOPs, and/or the range between around 10^14 and 10^16 artificial synapses)
You say 25% on "scaling up and fine-tuning GPT-ish systems works and brain-based anchors give a decent ballpark for model sizes." What do you mean by this? Do you not have upwards of 75% credence that the GPT scaling trends will continue for the next four OOMs at least? If you don't, that is indeed a big double crux.
I agree Neuromorph and Skunkworks are less plausible and do poorly on the "why couldn't you have said this in previous eras" test. I think they contribute some extra probability mass to the total but not much.
On re-running evolution: I wonder how many chimpanzees we'd need to kill in a breeding program to artificially evolve another intelligent species like us. If the answer is "Less than a trillion" then that suggests that CrystalNights would work, provided we start from something about as smart as a chimp. And arguably OmegaStar would be about as smart as a chimp -- it would very likely appear much smarter to people talking with it, at least. I'm not sure how big a deal this is, because intelligence isn't a single dimension, but it seems interesting and worth mentioning.
Re: "I deny the implication of your premise 2: namely, that if you have 80%+ on +12 OOMs, you should have 40%+ on +6 – this seems to imply that your distribution should be log-uniform from 1-12, which is a lot stronger of a claim than just “don’t be super spikey around 12.”" Here, let me draw some graphs. I don't know much math, but I know a too-spikey distribution when I see one! Behold the least spikey distribution I could make, subject to the constraint that 80% be by +12. Notice how steep the reverse slope is, and reflect on what that slope entails about the credence-holder's confidence in their ability to distinguish between two ultimately very similar and outlandish hypothetical scenarios.
Oh wait, now I see your final credences... you say 25% in 1e29 or less? And thus 20% by 2030? OK, then we have relatively little to argue about actually! 20% is not much different from my 50% for decision-making purposes. :)
Replies from: joekc↑ comment by Joe Carlsmith (joekc) · 2021-12-17T06:14:18.613Z · LW(p) · GW(p)
Thanks for these comments.
that suggests that CrystalNights would work, provided we start from something about as smart as a chimp. And arguably OmegaStar would be about as smart as a chimp - it would very likely appear much smarter to people talking with it, at least.
"starting with something as smart as a chimp" seems to me like where a huge amount of the work is being done, and if Omega-star --> Chimp-level intelligence, it seems a lot less likely we'd need to resort to re-running evolution-type stuff. I also don't think "likely to appear smarter than a chimp to people talking with it" is a good test, given that e.g. GPT-3 (2?) would plausibly pass, and chimps can't talk.
"Do you not have upwards of 75% credence that the GPT scaling trends will continue for the next four OOMs at least? If you don't, that is indeed a big double crux." -- Would want to talk about the trends in question (and the OOMs -- I assume you mean training FLOP OOMs, rather than params?). I do think various benchmarks [AF · GW] are looking good, but consider e.g. the recent Gopher paper:
On the other hand, we find that scale has a reduced benefit for tasks in the Maths, Logical Reasoning, and Common Sense categories. Smaller models often perform better across these categories than larger models. In the cases that they don’t, larger models often don’t result in a performance increase. Our results suggest that for certain flavours of mathematical or logical reasoning tasks, it is unlikely that scale alone will lead to performance breakthroughs. In some cases Gopher has a lower performance than smaller models– examples of which include Abstract Algebra and Temporal Sequences from BIG-bench, and High School Mathematics from MMLU.
(Though in this particular case, re: math and logical reasoning, there are also other relevant results to consider, e.g. this and this.)
It seems like "how likely is it that continuation of GPT scaling trends on X-benchmarks would result in APS-systems" is probably a more important crux, though?
Re: your premise 2, I had (wrongly, and too quickly) read this as claiming "if you have X% on +12 OOMs, you should have at least 1/2*X% on +6 OOMs," and log-uniformity was what jumped to mind as what might justify that claim. I have a clearer sense of what you were getting at now, and I accept something in the vicinity if you say 80% on +12 OOMs (will edit accordingly). My +12 number is lower, though, which makes it easier to have a flatter distribution that puts more than half of the +12 OOM credence above +6.
The difference between 20% and 50% on APS-AI by 2030 seems like it could well be decision-relevant to me (and important, too, if you think that risk is a lot higher in short-timelines worlds).
Replies from: daniel-kokotajlo↑ comment by Daniel Kokotajlo (daniel-kokotajlo) · 2021-12-17T18:18:57.049Z · LW(p) · GW(p)
Nice! This has been a productive exchange; it seems we agree on the following things:
--We both agree that probably the GPT scaling trends will continue, at least for the next few OOMs; the main disagreement is about what the practical implications of this will be -- sure, we'll have human-level text prediction and superhuman multiple-choice-test-takers, but will we have APS-AI? Etc.
--I agree with what you said about chimps and GPT-3 etc. GPT-3 is more impressive than a chimp in some ways, and less in others, and just because we could easily get from chimp to AGI doesn't mean we can easily get from GPT-3 to AGI. (And OmegaStar may be relevantly similar to GPT-3 in this regard, for all we know.) My point was a weak one which I think you'd agree with: Generally speaking, the more ways in which system X seems smarter than a chimp, the more plausible it should seem that we can easily get from X to AGI, since we believe we could easily get from a chimp to AGI.
--Now we are on the same page about Premise 2 and the graphs. Sorry it was so confusing. I totally agree, if instead of 80% you only have 55% by +12 OOMs, then you are free to have relatively little probability mass by +6. And you do.
Replies from: joekc↑ comment by Joe Carlsmith (joekc) · 2021-12-17T19:05:05.658Z · LW(p) · GW(p)
(Note that my numbers re: short-horizon systems + 12 OOMs being enough, and for +12 OOMs in general, changed since an earlier version you read, to 35% and 65% respectively.)
Replies from: daniel-kokotajlo↑ comment by Daniel Kokotajlo (daniel-kokotajlo) · 2021-12-17T19:53:09.442Z · LW(p) · GW(p)
Ok, cool! Here, is this what your distribution looks like basically?
Joe's Distribution?? - Grid Paint (grid-paint.com)
I built it by taking Ajeya's distribution from her report and modifying it so that:
--25% is in the red zone (the next 6 ooms)
--65% is in the red+blue zone (the next 12)
--It looks as smooth and reasonable as I could make it subject to those constraints, and generally departs only a little from Ajeya's.
Note that it still has 10% in the purple zone representing "Not even +50 OOMs would be enough with 2020's ideas"
I encourage you (and everyone else!) to play around with drawing distributions, I found it helpful. You should be able to make a copy of my drawing in Grid Paint and then modify it.
comment by bmg · 2021-12-17T22:36:37.398Z · LW(p) · GW(p)
I really appreciate you taking the time both to write this report and solicit/respond to all these reviews! I think this is a hugely valuable resource, that has helped me to better understand AI risk arguments and the range of views/cruxes that different people have.
A couple quick notes related to the review I contributed:
First, .4% is the credence implied by my credences in individual hypotheses — but I was a little surprised by how small this number turned out to be. (I would have predicted closer to a couple percent at the time.) I’m sympathetic to the possibility that the high level of conjuctiveness here created some amount of downward bias, even if the argument does actually have a highly conjunctive structure.
Second (only of interest to anyone who looked at my review): My sense is we still haven’t succeeded in understanding each other’s views about the nature and risk-relevance of planning capabilities. For example, I wouldn’t necessarily agree with this claim in your response to the section on planning:
Presumably, after all, a fixed-weight feedforward network could do whatever humans do when we plan trips to far away places, think about the best way to cut down different trees, design different parts of a particle collider, etc -- and this is the type of cognition I want to focus on.
Let’s compare a deployed version of AlphaGo with and without Monte Carlo tree search. It seems like the version with Monte Carlo tree search could be said to engage in planning: roughly speaking, it simulates the implications of different plays, and these simulations are used to arrive at better decisions. It doesn’t seem to me like there’s any sense in which the version of AlphaGo without MCTS is doing this. [1] Insofar as Go-playing humans simulate the implications of different plays, and use the simulations to arrive at better decisions, I don’t think a plain fixed-weight feedforward Go-playing network could be said to be doing the same sort of cognition as people. It could still play as well as humans, if it had been trained well enough, but it seems to me that the underlying cognition would nonetheless be different.
I feel like I have a rough sense of the distinction between these two versions of AlphaGo and a rough sense of how this distinction might matter for safety. But if both versions engage in “planning,” by some thinner conception of “planning,” then I don’t think I have a good understanding of what this version of the “planning”/“non-planning” distinction is pointing at — or why it matters.
It might be interesting to try to more fully unpack our views at some point, since I do think that differences in how people think about planning might be an underappreciated source of disagreement about AI risk (esp. around 'inner alignment').
One way of pressing this point: There’s not really a sense in which you could give it more ‘time to think,’ in a given turn, and have its ultimate decision keep getting better and better. ↩︎
↑ comment by Joe Carlsmith (joekc) · 2021-12-21T04:10:26.910Z · LW(p) · GW(p)
I’m glad you think it’s valuable, Ben — and thanks for taking the time to write such a thoughtful and detailed review.
I’m sympathetic to the possibility that the high level of conjuctiveness here created some amount of downward bias, even if the argument does actually have a highly conjunctive structure.”
Yes, I am too. I’m thinking about the right way to address this going forward.
I’ll respond re: planning in the thread with Daniel.
↑ comment by Daniel Kokotajlo (daniel-kokotajlo) · 2021-12-18T00:40:35.628Z · LW(p) · GW(p)
I'm curious to hear more about how you think of this AlphaGo example. I agree that probably the version of AlphaGo without MCTS is not doing any super detailed simulations of different possible moves... but I think in principle it could be, for all we know, and I think that if you kept making the neural net bigger and bigger and training it for longer and longer, eventually it would be doing something like that, because the simplest circuit that scores highly in the training environment would be a circuit that does something like that. Would you disagree?
Replies from: bmg↑ comment by bmg · 2021-12-18T23:27:26.578Z · LW(p) · GW(p)
Hm, I’d probably disagree.
A couple thoughts here:
First: To me, it seems one important characteristic of “planners” is that they can improve their decisions/behavior even without doing additional learning. For example, if I’m playing chess, there might be some move that (based on my previous learning) initially presents itself as the obvious one to make. But I can sit there and keep running mental simulations of different games I haven’t yet played (“What would happen if I moved that piece there…?”) and arrive at better and better decisions.
It doesn’t seem like that’d be true of a deployed version of AlphaGo without MCTS. If you present it with some board state, it seems like it will just take whatever action (or distribution of actions) is already baked into its policy. There’s not a sense, I think, in which it will keep improving its decision. Unlike in the MCTS case, you can’t tweak some simple parameter and give it more ‘time to think’ and allow it to make a better decision. So that’s one sense in which AlphaGo without MCTS doesn’t seem, to me, like it could exhibit planning.
However, second: A version of AlphaGo without explicit MCTS might still qualify as a “planner” on a thinner conception of “planning.” In this case, I suppose the hypothesis would be that: when we do a single forward pass through the network, we carry out some computations that are roughly equivalent to the computations involved in (e.g.) MCTS. I suppose that can’t be ruled out, although I’m also not entirely sure how to think about it. One thing we could still say, though, is that insofar as planning processes tend to involve a lot of sequential steps, the number of layers in MCTS-less AlphaGo would seriously limit the amount of ‘planning’ it can do. Eight layers don’t seem like nearly enough for a forward pass to correspond to any meaningful amount of planning.
So my overall view is: For a somewhat strict conception of “planning,” it doesn’t seem like feedforward networks can plan. For a somewhat loose conception of “planning,” it actually is conceivable that a feedforward network could plan — but (I think) only if it had a really huge number of layers. I’m also not sure that there would a tendency for the system to start engaging in this kind of “planning” as layer count increases; I haven't thought enough to have a strong take.[1]
Also, to clarify: I think that the question of whether feedforward networks can plan probably isn’t very practically relevant, in-and-of-itself — since they’re going to be less important than other kinds of networks. I’m interested in this question mainly as a way of pulling apart different conceptions of “planning,” noticing ambiguities and disagreements, etc. ↩︎
↑ comment by gwern · 2021-12-30T01:46:14.675Z · LW(p) · GW(p)
For a somewhat loose conception of “planning,” it actually is conceivable that a feedforward network could plan — but (I think) only if it had a really huge number of layers.
Search doesn't buy you that much, remember. After relatively few nodes, you've already gotten much of the benefit from finetuning the value estimates (eg. AlphaZero, or the MuZero appendix). And you can do weight-tying to repeat feedforward layers, or just repeat layers/the model. (Is AlphaFold2 recurrent? Is ALBERT recurrent? A VIN? Or a diffusion model? Or a neural ODE?) This is probably why Jones finds that distilling MCTS runtime search into search-less feedforward parameters comes, empirically, at a favorable exchange rate I wouldn't call 'really huge'.
↑ comment by Daniel Kokotajlo (daniel-kokotajlo) · 2021-12-19T01:25:38.747Z · LW(p) · GW(p)
OK, thanks. Why is it important that they be able to easily improve their performance without learning?
I agree that eight layers doesn't seem like enough to do some serious sequential pondering. For comparison, humans take multiple seconds--often minutes--of subjective time to do this, at something like 100 sequential steps per second.
Replies from: joekc↑ comment by Joe Carlsmith (joekc) · 2021-12-21T04:44:14.725Z · LW(p) · GW(p)
Cool, these comments helped me get more clarity about where Ben is coming from.
Ben, I think the conception of planning I’m working with is closest to your “loose” sense. That is, roughly put, I think of planning as happening when (a) something like simulations are happening, and (b) the output is determined (in the right way) at least partly on the basis of those simulations (this definition isn’t ideal, but hopefully it’s close enough for now). Whereas it sounds like you think of (strict) planning as happening when (a) something like simulations are happening, and (c) the agent’s overall policy ends up different (and better) as a result.
What’s the difference between (b) and (c)? One operationalization could be: if you gave an agent input 1, then let it do its simulations thing and produce an output, then gave it input 1 again, could the agent’s performance improve, on this round, in virtue of the simulation-running that it did on the first round? On my model, this isn’t necessary for planning; whereas on yours, it sounds like it is?
Let’s say this is indeed a key distinction. If so, let’s call my version “Joe-planning” and your version “Ben-planning.” My main point re: feedforward neural network was that they could do Joe-planning in principle, which it sounds like you think at least conceivable. I agree that it seems tough for shallow feedforward networks to do much of Joe-planning in practice. I also grant that when humans plan, they are generally doing Ben-planning in addition to Joe-planning (e.g., they’re generally in a position to do better on a given problem in virtue of having planned about that same problem yesterday).
Seems like key questions re: the connection to AI X-risk include:
- Is there reason to think a given type of planning especially dangerous and/or relevant to the overall argument for AI X-risk?
- Should we expect that type of planning to be necessary for various types of task performance?
Re: (1), I do think Ben-planning poses dangers that Joe-planning doesn’t. Notably, Ben planning does indeed allow a system to improve/change its policy "on its own" and without new data, whereas Joe planning need not — and this seems more likely to yield unexpected behavior. This seems continuous, though, with the fact that a Ben-planning agent is learning/improving its capabilities in general, which I flag separately as an important risk factor.
Another answer to (1), suggested by some of your comments, could appeal to the possibility that agents are more dangerous when you can tweak a single simple parameter like “how much time they have to think” or “search depth” and thereby get better performance (this feels related to Eliezer’s worries [LW · GW] about “turning up the intelligence dial” by “running it with larger bounds on the for-loops”). I agree that if you can just “turn up the intelligence dial,” that is quite a bit more worrying than if you can’t — but I think this is fairly orthogonal to the Joe-planning vs. Ben-planning distinction. For example, I think you can have Joe-planning agents where you can increase e.g. their search depth by tweaking a single parameter, and you can have Ben-planning agents where the parameters you’d need to tweak aren’t under your control (or the agent’s control), but rather are buried inside some tangled opaque neural network you don't understand.
The central reason I'm interested in Joe-planning, though, is that I think the instrumental convergence argument makes the most sense if Joe-planning is involved -- e.g., if the agent is running simulations that allow it to notice and respond to incentives to seek power (there are versions of the argument that don't appeal to Joe-planning, but I like these less -- see discussion in footnote 87 here). It's true that you can end up power-seeking-ish via non-Joe-planning paths (for example, if in training you developed sphex-ish heuristics that favor power-seeking-ish actions); but when I actually imagine AI systems that end up power-seeking, I imagine it happening because they noticed, in the course of modeling the world in order to achieve their goals, that power-seeking (even in ways humans wouldn't like) would help.
Can this happen without Ben-planning? I think it can. Suppose, for example, that none of your previous Joe-planning models were power-seeking. Then, you train a new Joe-planner, who can run more sophisticated simulations. On some inputs, this Joe-planner realizes that power-seeking is advantageous, and goes for it (or starts deceiving you, or whatever).
Re: (2), for the reasons discussed in section 3.1, I tend to see Joe-planning as pretty key to lots of task-performance — though I acknowledge that my intuitions are surprised by how much it looks like you can do via something more intuitively “sphexish.” And I acknowledge that some of those arguments may apply less to Ben-planning. I do think this is some comfort, since agents that learn via planning are indeed scarier. But I am separately worried that ongoing learning will be very useful/incentivized, too.
comment by Dawn Drescher (Telofy) · 2021-12-24T14:16:10.908Z · LW(p) · GW(p)
That’s a great report and exercise! Thank you!
I hopelessly anchored on almost everything in the report, so my estimate is far from independent. I followed roughly Nate’s approach (though that happened without anchoring afaik), and my final probability is ~ 50% (+/- 5% when I play around with the factors). But it might’ve turned out differently if I had had an espresso more or less in the morning.
50% is lower than what I expected – another AI winter would lead to a delay and might invalidate the scaling hypothesis, so that the cumulative probability should probably increase more slowly after 2040–50, but I would’ve still expected something like 65–85%.
My biggest crux seems to be about the necessity for “deployment.” The report seems to assume that for a system to become dangerous, someone has to decide to deploy it. I don’t know what the technology will be like decades from now, but today I test software systems hundreds of times on my laptop and on different test systems before I deploy them. I’m the only user of my laptop, so privilege escalation is intentionally trivial. As it happens it’s also more powerful than the production server. Besides, my SSH public key is in authorized_keys files on various servers.
So before I test a software, I likely will not know whether it’s aligned, and once I test it, it’s too late. Add to that that even if many people should indeed end up using carefully sandboxed systems for testing AGIs within a few decades, it still only takes one person to relax their security a bit.
So that’s why I merged the last three points and collectively assigned ~ 95% to them (not anchored on Nate afaik). (Or to be precise I mostly ignored the last point because it seems like a huge intellectual can of beans spanning ethics, game theory, decision theory, physics, etc., so more than the purview of the report.)
comment by Richard_Ngo (ricraz) · 2021-12-27T20:09:46.010Z · LW(p) · GW(p)
Thanks for providing this! Could you say a little bit about what you were aiming for with these reviews? I find the collection of people involved somewhat surprising (although they're all individually great) - particularly the lack of mainstream ML researchers.
Also, I hadn't seen this framing before, so I enjoyed this section of Leopold's response:
Replies from: joekcI could tell you about an agent in the world that is very misaligned (downright evil, proto-fascist, depending on who you ask), has the brainpower equivalent to 1.4 billion people, is in control of the second largest military in the world, and is probably power-seeking. This seems like a pretty worst-case version of the scenario outlined in the report. This agent is called China! Fwiw, I am quite worried about China. But I see the argument about AI as analogous to an argument about other misaligned agents. And my prior on other misaligned agents is that competition and correction is generally very good at keeping them in check.
↑ comment by Joe Carlsmith (joekc) · 2022-01-03T05:58:04.909Z · LW(p) · GW(p)
Reviewers ended up on the list via different routes. A few we solicited specifically because we expected them to have relatively well-developed views that disagree with the report in one direction or another (e.g., more pessimistic, or more optimistic), and we wanted to understand the best objections in this respect. A few came from trying to get information about how generally thoughtful folks with different backgrounds react to the report. A few came from sending a note to GPI saying we were open to GPI folks providing reviews. And a few came via other miscellaneous routes. I’d definitely be interested to see more reviews from mainstream ML researchers, but understanding how ML researchers in particular react to the report wasn’t our priority here.