Posts
Comments
Good point, fixing!
an estimated utility function is a practical abstraction that obscures the lower-level machinery/implementational details
I agree that this is what's happening. I probably have different intuitions regarding how big of a problem it is.
The main questions here might be something like:
- Is there any more information about the underlying system, besides its various utility function, useful for decision-making?
- If (1) is false, can we calibrate for that error when trying to approximate things with the utility function? If we just use the utility function, will be be over-confident, or just extra (and reasonably) cautious?
- In situations where we don't have models of the underlying system, can utility function estimates be better than alternatives we might have?
My quick expected answers to this:
- I think for many things, utility functions are fine. I think these are far more precise and accurate than other existing approaches that we have today (like, people intuitively guessing what's good for others) .
- I think if we do a decent job, we can just add extra uncertainty/caution to the system. I like to trust future actors here to not be obviously stupid in ways we could expect.
- As I stated before, I don't think we have better tools yet. I'm happy to see research into more work in understanding the underlying systems, but in the meantime, utility functions seem about as precise and information-rich as anything else we have.
is that different "deliberation/idealization procedures" may produce very different results and never converge in the limit.
Agreed. This is a pretty large topic, I was trying to keep this essay limited. My main recommendation here was to highlight the importance of deliberation and potential deliberation levels, in part to better discuss issues like these.
Do you have a preferred distinction of value functions vs. utility functions that you like, and ideally can reference? I'm doing some investigation now, and it seems like the main difference is the context in which they are often used.
My impression is that Lesswrong typically uses the term "Utility function" to mean a more specific thing than what economists do. I.E. the examples of utility functions in economics textbooks. https://brilliant.org/wiki/utility-functions/ has examples.
They sometimes describe simple things like this simple relationship, as a "utility function".
I'm curious why this got the disagreement votes.
1. People don't think Holden doing that is significant prioritization?
2. There aren't several people at OP trying to broadly figure out what to do about AI?
3. There's some other strategy OP is following?
Also, I should have flagged that Holden is now the "Director of AI Strategy" there. This seems like a significant prioritization.
It seems like there are several people at OP trying to figure out what to broadly do about AI, but only one person (Ajeya) doing AIS grantmaking? I assume they've made some decision, like, "It's fairly obvious what organizations we should fund right now, our main question is figuring out the big picture."
Ajeya Cotra is currently the only evaluator for technical AIS grants.
This situation seems really bizarre to me. I know they have multiple researchers in-house investigating these issues, like Joseph Carlsmith. I'm really curious what's going on here.
I know they've previously had (what seemed to me) like talented people join and leave that team. The fact that it's so small now, given the complexity and importance of the topic, is something I have trouble grappling with.
My guess is that there are some key reasons for this that aren't obvious externally.
I'd assume that it's really important for this team to become really strong, but would obviously flag that when things are that strange, it's likely difficult to fix, unless you really understand why the situation is the way it is now. I'd also encourage people to try to help here, but I just want to flag that it might be more difficult than it might initially seem.
Thanks for clarifying! That really wasn't clear to me from the message alone.
> Though if you used Squiggle to perform an existential risk-reward analysis of whether to use Squiggle, who knows what would happen
Yep, that's in the works, especially if we can have basic relative value forecasts later on.
If you think that the net costs of using ML techniques when improving our rationalist/EA tools are not worth it, then there can be some sort of argument there.
Many Guesstimate models are now about making estimates about AI safety.
I'm really not a fan of the "Our community must not use ML capabilities in any form", not sure where others here might draw the line.
I assume that in situations like this, it could make sense for communities to have some devices for people to try out.
Given that some people didn't return theirs, I imagine potential purchasers could buy used ones.
Personally, I like the idea of renting one for 1-2 months, if that were an option. If there's a 5% chance it's really useful, renting it could be a good cost proposition. (I realize I could return it, but feel hesitant to buy one if I think there's a 95% chance I would return it.)
Happy to see experimentation here. Some quick thoughts:
- The "Column" looked a lot to me like a garbage can at first. I like the "+" in Slack for this purpose, that could be good.
- Checkmark makes me think "agree", not "verified". Maybe a badge or something?
- "Support" and "Agreement" seem very similar to me?
- While it's a different theme, I'm in favor of using popular icons where possible. My guess is that these will make it more accessible. I like the eyes you use, in part because are close to the icon. I also like:
- 🚀 or 🎉 -> This is a big accomplishment.
- 🙏 -> Thanks for doing this.
- 😮 -> This is surprising / interesting.
- It could be kind of neat to later celebrate great rationalist things by having custom icons for them, to represent when a post reminds people of their work in some way.
- I like that it shows who reacted what, that makes a big deal to me.
I liked this a lot, thanks for sharing.
Here's one disagreement/uncertainty I have on some of it:
Both of the "What failure looks like" posts (yours and Pauls) posts present failures that essentially seem like coordination, intelligence, and oversight failures. I think it's very possible (maybe 30-46%+?) that pre-TAI AI systems will effectively solve the required coordination and intelligence issues.
For example, I could easily imagine worlds where AI-enhanced epistemic environment make low-risk solutions crystal clear to key decision-makers.
In general, the combination of AI plus epistemics, pre-TAI, seems very high-variance to me. It could go very positively, or very poorly.
This consideration isn't enough to change p(doom) under 10%, but I'm probably be closer to 50% than you would be. (Right now, maybe 40% or so).
That said, this really isn't a big difference, it's less than one order of magnitude.
Quick update:
Immersed now supports a BETA for "USB Mode". I just tried it with one cable, and it worked really well, until it cut out a few minutes in. I'm getting a different USB-C cable that they recommend. In general I'm optimistic.
(That said, there are of course better headsets/setups that are coming out, too)
https://immersed.zendesk.com/hc/en-us/articles/14823473330957-USB-C-Mode-BETA-
Happy to see discussion like this. I've previously written a small bit defending AI friends, on Facebook. There was some related comments there.
I think my main takeaway is "AI friends/romantic partners" are some seriously powerful shit. I expect we'll see some really positive uses and also some really detrimental ones. I'd naively assume that, like with other innovations, some communities/groups will be much better at dealing with them than others.
Related, research to help encourage the positive sides seems pretty interesting to me.
Maybe we can refer to these systems as cybernetic or cyborg rubber ducking? :)
Yea; that's not a feature that exists yet.
Thanks for the feedback!
Dang, this looks awesome. Nice work!
Not yet. There are a few different ways of specifying the distribution, but we don't yet have options for doing from the 25th&75th percentiles. It would be nice to do eventually. (Might be very doable to add in a PR, for a fairly motivated person).
https://www.squiggle-language.com/docs/Api/Dist#normal
You can type in, normal({p5: 10, p95:30})
. It should later be possible to say normal({p25: 10, p75:30})
.
Separately; when you say "25, 50, 75 percentiles"; do you mean all at once? This would be an overspecification; you only need two points. Also; would you want this to work for normal/lognormal distributions, or anything else?
Mostly. The core math bits of Guesstimate were a fairly thin layer on Math.js. Squiggle has replaced much of the MathJS reliance with custom code (custom interpreter + parser, extra distribution functionality).
If things go well, I think it would make sense to later bring Squiggle in as the main language for Guesstimate models. This would be a breaking change, and quite a bit of work, but would make Guesstimate much more powerful.
Really nice to see this. I broadly agree. I've been concerned with boards for a while.
I think that "mediocre boards" are one of the greatest weaknesses of EA right now. We have tons of small organizations, and I suspect that most of these have mediocre or fairly ineffective boards. This is one of the main reasons I don't like the pattern of us making lots of tiny orgs; because we have to set up yet one more board for each one, and good board members are in short supply.
I'd like to see more thinking here. Maybe we could really come up with alternative structures.
For example, I've been thinking of something like "good defaults" as a rule of thumb for orgs that get a lot of EA funding.
- They choose an effective majority of board members from a special pool of people who have special training and are well trusted by key EA funders.
- There's a "board service" organization that's paid to manage the processes of boards. This service would arrange meetings, make sure that a bunch of standards are getting fulfilled, and would have the infrastructure in place to recruit new EDs when needed. These services can be paid by the organization.
Basically, I'd want to see us treat small nonprofits as sub-units of a smoothly-working bureaucracy or departments in a company. This would involve a lot of standardization and control. Obviously this could backfire a lot if the controlling groups ever do a bad job; but (1) if the funders go bad, things might be lost anyway, and (2), I think the expected harm of this could well be less than the expected benefit.
For what it's worth, I think I prefer the phrase,
"Failing with style"
Minor point:
I suggest people experiment with holiday ideas and report back, before we announce anything "official". Experimentation seems really nice on this topic, that seems like the first step.
In theory we could have a list of holiday ideas, and people randomly choose a few of them, try them out, then report back.
Interesting. Thanks!
The more sophisticated system is Squiggle. It's basically a prototype. I haven't updated it since the posts I made about it last year.
https://www.lesswrong.com/posts/i5BWqSzuLbpTSoTc4/squiggle-an-overview
Update:
I think some of the graphs could be better represented with upfront fixed costs.
When you buy a book, you pay for it via your time to read it, but you also have the fixed initial fee of the book.
This fee isn't that big of a deal for most books that you have a >20% chance of reading, but it definitely is for academic articles or similar.
(Also want to say I've been reading them all and am very thankful)
I enjoyed writing this post, but think it was one of my lesser posts. It's pretty ranty and doesn't bring much real factual evidence. I think people liked it because it was very straightforward, but I personally think it was a bit over-rated (compared to other posts of mine, and many posts of others).
I think it fills a niche (quick takes have their place), and some of the discussion was good.
Good point! I feel like I have to squint a bit to see it, but that's how exponentials sometimes look early on.
To be clear, I care about clean energy. However, if energy production can be done without net-costly negative externalities, then it seems quite great.
I found Matthew Yglesias's take, and Jason's writings, interesting.
https://www.slowboring.com/p/energy-abundance
All that said, if energy on the net leads to AGI doom, that could be enough to offset any gain, but my guess is that clean energy growth is still a net positive.
but I think this is actually a decline in coal usage.
Ah, my bad, thanks!
They estimate ~35% increase over the next 30 years
That's pretty interesting. I'm somewhat sorry to see it's linear (I would have hoped solar/battery tech would improve more, leading to much faster scaling, 10-30 years out), but it's at least better than some alternatives.
I found this last chart really interesting, so did some hunting. It looks electricity generation in the US grew linearly until around ~2000. In the last 10 years though, there's been a very large decline in "petroleum and other", along with a strong increase in natural gas, and a smaller, but significant, increase in renewables.
I'd naively guess things to continue to be flat for a while as petroleum use decreases further; but at some point, I'd expect energy use to increase again.
That said, I'd of course like for it to increase much, much faster (more like China). :)
https://www.eia.gov/energyexplained/electricity/electricity-in-the-us-generation-capacity-and-sales.php
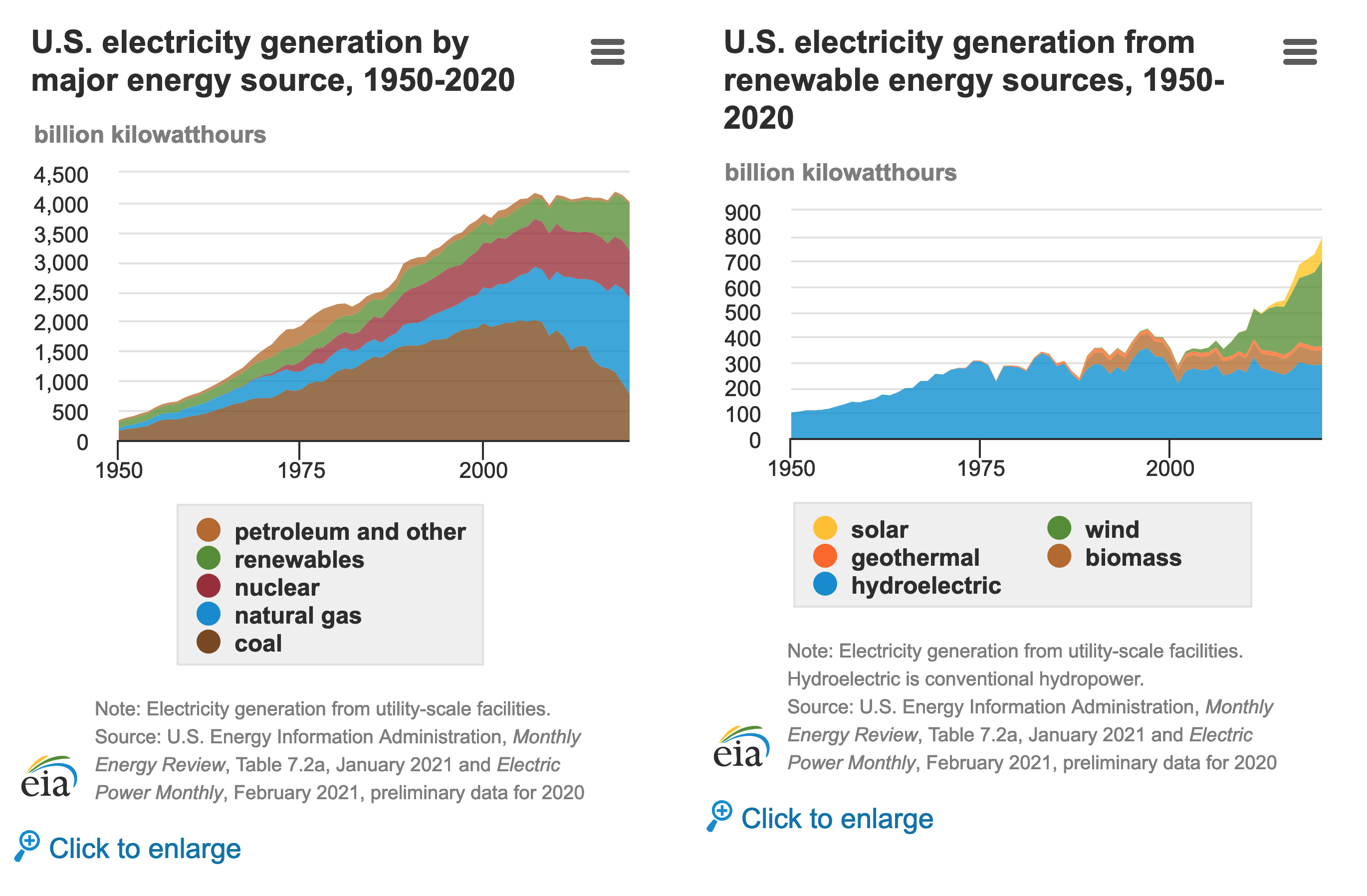
I liked this post a lot, though of course, I didn't agree with absolutely everything.
These seemed deeply terrible. If you think the best use of funds, in a world in which we already have billions available, is to go trying to convince others to give away their money in the future, and then hoping it can be steered to the right places, I almost don’t know where to start. My expectation is that these people are seeking money and power,
I'm hesitant about this for a few reasons.
- Sure, we have a few billion available, and we're having trouble donating that right now. But we're also not exactly doing a ton of work to donate our money yet. (This process gave out $10 Million, with volunteers). In the scheme of important problems, a few (~40-200) billion really doesn't seem like that much to me. Marginal money, especially lots of money, still seems pretty good.
- My expectation is that these people are seeking money and power -> I don't know which specific groups applied or their specific details. I can say that my impression, lots of EAs really just don't know what else to do. It's tough to enter research, and we just don't have that much in terms of "these interventions would be amazing, please someone do them" for longtermism. I've seen a lot of orgs get created with something like, "This seems like a pretty safe strategy, it will likely come into use later on, and we already have the right connections to make it happen." This, combined with a general impression that marginal money is still useful in the long-term, I think could present a more sympathetic take than what you describe.
The default strategy for lots of non-EA entrepreneurs I know has been something like, "Make a ton of money/influence, then try to figure out how to use it for good. Because people won't listen to me or fund my projects on my own". I wish more of these people would do direct work (especially in the last few years, when there's been more money), but can sympathize with that strategy. Arguably, Elon Musk is much better off having started with "less ambitious" ventures like Zip2 and Paypal; it's not clear if he would have been funded to start with SpaceX/Tesla when he was younger.
All that said, the fact that EAs have so little idea of what exactly is useful seems like a pretty burning problem to me. (This isn't unique to EAs, to be clear). On the margin, it seems safe to heavily emphasize "figuring stuff out" instead of "making more money, in hopes that we'll eventually figure stuff out" However, "figuring stuff out" is pretty hard and not nearly as tractable as we'd like it to be.
"I would hire assistance to do at least the following"
I've been hoping that the volunteer funders (EA Funds, SFF) would do this for a while now. Seems valuable to at least try out for a while. In general, "funding work" seems really bottlenecked to me, and I'd like to see anything that could help unblock it.
definitely a case of writing a longer letter
I'm impressed by just how much you write on things like this. Do you have any posts outlining your techniques? Is there anything special, like speech-to-text, or do you spend a lot of time on it, or are you just really fast?
Thanks!
Just checking; I think you might have sent the wrong link though?
Quick question:
When you say, "Yuji adjustable-color-temperature LED strips/panels"
Do you mean these guys?
https://store.yujiintl.com/products/yujileds-high-cri-95-dim-to-warm-led-flexible-strip-1800k-to-3000k-168-leds-m-pack-5m-reel
It looks kind of intimidating to setup, and is pricey, but maybe is worth it.
Just want to say; I'm really excited to see this.
I might suggest starting with an "other" list that can be pretty long. With Slack, different subcommunities focus heavily on different emojis for different functional things. Users sometimes figure out neat innovations and those proliferate. So if it's all designed by the LW team, you might be missing out.
That said, I'd imagine 80% of the benefit is just having anything like this, so I'm happy to see that happen.
That's interesting to know, thanks!
I just (loosely) coined "disagreeables" and "assessors" literally two days ago.
I suggest coming up with any name you think is a good fit.
I wouldn't read too much into my choice of word there.
It's also important to point out that I was trying to have a model that assumed interestingness. The "disagreeables" I mention are the good ones, not the bad ones. The ones worth paying attention to I think are pretty decent here; really, that's the one thing they have to justify paying attention to them.
Good point, agreed.
A few quick thoughts:
1) This seems great, and I'm impressed by the agency and speed.
2) From reading the comments, it seems like several people were actively afraid of how Leverage could retaliate. I imagine similar for accusations/whistleblowing for other organizations. I think this is both very, very bad, and unnecessary; as a whole, the community is much more powerful than individual groups, so it seems poorly managed when the community is scared of a specific group. Resources should be spent to cancel this out.
In light of this, if more money were available, it seems easy to justify a fair bit more. Or even better could be something like, "We'll help fund lawyers in case you're attacked legally, or anti-harassing teams if you're harassed or trolled". This is similar to how the EFF helps with cases from small people/groups being attacked by big companies.
I don't mean to complain; I think any steps here, especially so quickly are fantastic.
3) I'm afraid this will get lost in this comment section. I'd be excited about a list of "things to keep in mind" like this to be repeatedly made prominent somehow. For example, I could imagine that at community events or similar, there could be necessary papers like, "Know your rights, as a Rationalist/EA", which flags how individuals can report bad actors and behavior.
4) Obviously a cash prize can encourage lying, but I think this can be decently managed. (It's a small community, so if there's good moderation, $15K would be very little compared to the social stigma that would come and you've found out to have destructively lied for $15k)
The latter option is more of what I was going for.
I’d agree that the armor/epistemics people often aren’t great at coming up with new truths in complicated areas. I’d also agree that they are extremely unbiased and resistant to both poor faith arguments, and good faith, but systematically misleading arguments (these are many of the demons the armor protects against, if that wasn’t clear).
When I said that they were soft-spoken and poor at arguing, I’m assuming that they have great calibration and are likely arguing against people who are very overconfident, so in comparison they seem meager. I think of a lot of superforecasters in this way; they’re quite thoughtful and reasonable, but not often bold enough to sell a lot of books. Other people with too epistemics sometimes recognize their skills (especially when f they have empirical track records like in forecasting systems), but that’s right now a meager minority.
When I hear the words "intelligence" and "wisdom", I think of things that are necessarily properties of individual humans, not groups of humans. Yet some of the specifics you list seem to be clearly about groups.
I tried to make it clear that I was referring to groups with the phrase, "of humanity", as in, "as a whole", but I could see how that could be confusing.
the wisdom and intelligence[1] of humanity
For those interested in increasing humanity’s long-term wisdom and intelligence[1]
I also suspect that work on optimizing group decision making will look rather different from work on optimizing individual decision making, possibly to the point that we should think of them as separate cause areas.
I imagine there's a lot of overlap. I'd also be fine with multiple prioritization research projects, but think it's early to decide that.
This makes me wonder how nascent this really is?
I'm not arguing that people haven't made successes in the entire field (I think there's been a ton of progress over the last few hundred years, and that's terrific). I would argue though that there's very little formal prioritization of such progress. Similar to how EA has helped formalize the prioritization of global health and longtermism, we have yet to have similar efforts for "humanity's wisdom and intelligence".
I think that there are likely still strong marginal gains in at least some of the intervention areas.
That's an interesting perspective. It does already assume some prioritization though. Such experimentation can only really be done in a very few of the intervention areas.
I like the idea, but am not convinced of the benefit of this path forward, compared to other approaches. We already have had a lot of experiments in this area, many of which cost a lot more than $15,000; marginal exciting ones aren't obvious to me.
But I'd be up for more research to decide if things like that are the best way forward :)
The first few chapters of "The Existential Pleasures of Engineering" detail some optimism, then pessimism, of technocracy in the US at least.
I think the basic story there was that after WW2, in the US, people were still pretty excited about tech. But in the 70s (I think), with environmental issues, military innovations, and general malaise, people because disheartened.
https://www.amazon.com/Existential-Pleasures-Engineering-Thomas-Dunne-ebook/dp/B00CBFXLWQ
I'm sure I'm missing details, but I found the argument interesting. It is true that in the US at least, there seemed to be a lot of techno-optimism post-WW2.
Ah, thanks!
Thanks for the opinion, and I find the take interesting.
I'm not a fan of the line, "How about a policy that if you use illegal drugs you are presumptively considered not yet good enough to be in the community?", in large part because of the phrase "not yet good enough". This is a really thorny topic that seems to have several assumptions baked into it that I'm uncomfortable with.
I also think that many here like at least some drugs that are "technically illegal", in part, because the FDA/federal rules move slowly. Different issue though.
I like points 2 and 3, I imagine if you had a post just with those two it would have gotten way more upvotes.
There's an "EA Mental Health Navigator" now to help people connect to the right care.
https://eamentalhealth.wixsite.com/navigator
I don't know how good it is yet. I just emailed them last week, and we set up an appointment for this upcoming Wednesday. I might report back later, as things progress.
I really like things like this. I think it's possible we could do a "decent enough" job, though it's impossible to have a solution without risk.
One thing I've been thinking about is a browser extention. People would keep a list of things, like, "User XYZ is Greg Hitchenson", and then when it sees XYZ, it adds annotation".
Lots of people are semi-anonymous already. They have psuedonyms that most people don't know, but "those in the know" do. This sort of works, but isn't formalized, and can be a pain. (Lots of asking around: "Who is X?")
That's good to know.
I imagine grantmakers would be skeptical about people who would say "yes" to an optional form. Like, they say they're okay with the information being public, but when it actually goes out, some of them will complain about it, leading to a lot of extra time.
However, some of our community seems unusually reasonable, so perhaps there's some way to make it viable.
I agree that it would have been really nice for grantmakers to communicate with the EA Hotel more, and other orgs more, about their issues. This is often a really challenging conversation to have ("we think your org isn't that great, for these reasons"), and we currently have very few grantmaker hours for the scope of the work, so I think grantmakers don't have much time now to spend on this. However, there does seem to be a real gap here to me. I represent a small org and have been around other small orgs, and the lack of communication with small grantmakers is a big issue. (And I probably have it much easier than most groups, knowing many of the individuals responsible)
I think the fact that we have so few grantmakers right now is a big bottleneck that I'm sure basically everyone would love to see improved. (The situation isn't great for current grantmakers, who often have to work long hours). But "figuring out how to scale grantmaking" is a bit of a separate discussion.
Around making the information public specifically, that's a whole different matter. Imagine the value proposition, "If you apply to this grant, and get turned down, we'll write about why we don't like it publically for everyone to see." Fewer people would apply and many would complain a whole lot when it happens. The LTFF already gets flack for writing somewhat-candid information on the groups they do fund.
(Note: I was a guest manager on the LTFF for a few months, earlier this year)
Thanks for the review here. I found this book highly interesting and relevant. I've been surprised at how much it seems to have been basically ignored.