MIRI Conversations: Technology Forecasting & Gradualism (Distillation)
post by CallumMcDougall (TheMcDouglas) · 2022-07-13T15:55:40.348Z · LW · GW · 1 commentsContents
TL;DR Introduction Setting the stage: gradualism & catastrophism Conversations on technology forecasting and gradualism What can history teach us? Physics doesn’t care about your feelings A bias tug-of-war Startups, secrets, and (in)efficient markets Sparse World Hypothesis Okay, so where do we go from here? Agreeing to disagree None 1 comment
This was submitted as part of UC Berkeley's distillation competition. Many thanks to everyone who gave feedback on this post – in particular Hannah Erlebach, Jamie Bernardi, Dewi Erwan, Arthur Conmy, and Peter Barnett.
Crossposted to the EA Forum [EA · GW].
TL;DR
Gradualism and catastrophism represent opposite ends of a spectrum of predictions about AI takeoff speeds. Gradualists (e.g. Paul Christiano) expect continuous progress without sharp discontinuities, whereas catastrophists (e.g. Eliezer Yudkowsky) expect there to be a phase transition around the point of human-level AGI.
In this excerpt from the Late 2021 MIRI Conversations [? · GW], the participants apply gradualist / catastrophist models to different domains, and see how the predictions generated by these models hold up to reality. In this post, I’ll focus on three domains: historical examples, startups, and peoples’ skill distributions.
I will conclude with a short retrospective on some of the MIRI conversations, and the lessons we can draw from them about the state of discourse in the alignment community.
Introduction
Flight by machines heavier than air is impractical and insignificant, if not utterly impossible.
Simon Newcomb, 1835 - 1909
If we worked on the assumption that what is accepted as true really is true, then there would be little hope for advance.
Orville Wright, 1871 - 1948
On September 14th, 2021, Paul Christiano and Eliezer Yudkowsky met in a Discord server. Their goal was to try and hash out some of their disagreements related to AI takeoff speeds. In particular, Paul was defending his “soft takeoff” view against Eliezer’s “hard takeoff”.
In brief, the “soft takeoff” / gradualist story for AI progress looks like a continuous ramping up of investment in and capabilities of AI as the field progresses. There are no huge jumps or discontinuities. By the time AGI rolls around, the world already looks pretty crazy, with AI already having had a major impact on society, and GDP having grown at a pretty wild rate over the last few years.
In contrast, in the “hard takeoff” / catastrophist scenario, the development of AGI will be a phase change (classically described as “FOOM”). There will come a point where AIs can propel themselves from “relatively unimpressive” to “strongly superhuman levels of intelligence”, and this could all happen inside a very short timeframe, maybe even a few hours.
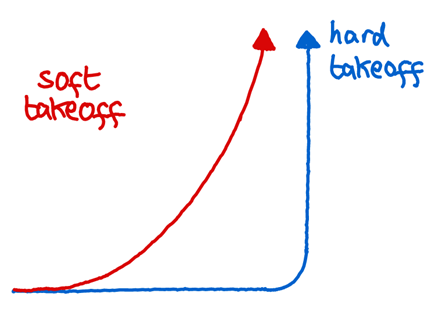
Why is this an important issue for the AI alignment community? One main reason is that it strongly informs [LW · GW] what kind of alignment work will be most useful. In the fast takeoff world, we shouldn’t expect risks from AGI to look more obvious in the future than they do right now, and so we should be focusing on tackling the difficult technical research questions in alignment. On the other hand, in the slow takeoff world we should expect the gameboard to look very different in the future than it does today, and we should be focusing more on building governance and institutional capacity.
Paul and Eliezer’s discussions (and many more) have now been published in full on LessWrong and on MIRI’s website. While they provide a fascinating insight into the views of several leading voices in AI safety research, they’re also a bit hard to decipher. This is partly because of the unfiltered format of the dialogues, but also because the participants tended to speak past each other, and found it difficult to find cruxes on which they disagree, or ways in which they could test their different worldviews by making predictions.
I first came across these discussions in Scott Alexander’s post Yudkowsky Contra Christiano On AI Takeoff Speeds. I found this summary incredibly clear and informative, and I’d had the idea of attempting something similar for other discussions in the sequence in the back of my mind for a while. When the Berkeley distillation competition was announced, I took that as my signal to give it a go. The post I’ve chosen to write a distillation of is Conversation on technology forecasting and gradualism [LW · GW], because I think it offers some new and interesting perspectives on the differences between the gradualist and catastrophist worldviews. I aim to summarise these perspectives, and connect them to some other relevant writing (e.g. Katja Grace’s study of historical discontinuities, and Peter Thiel’s book Zero to One). I also think it contains some valuable meta-level perspectives on the current state of debate in the AI safety community, which I will return to at the end of the post.
Setting the stage: gradualism & catastrophism
As mentioned above, I was inspired to write this by reading Scott Alexander’s post on Paul and Eliezer’s respective positions. I would highly recommend reading this if you haven’t already. This next section is intended as a brief summary of their respective positions as described by Scott before I dive into the main part of the post, so feel free to jump ahead if you’ve already read it.
The disagreement between Paul and Eliezer can approximately be traced to Paul’s 2018 post Takeoff speeds. In this post, Paul discusses some of the frameworks he uses for thinking about slow takeoffs, and why he doesn’t find many of the main arguments for hard takeoff convincing. For instance, one of the core arguments used by people like Eliezer is that the transition from chimps to humans took place very rapidly over an evolutionary timescale. The fact that chimps and human brains are very similar biologically speaking, but we wield intelligence in such different ways, suggests that there may be some “secret sauce” of intelligence, and once you get it, you can advance very quickly, accumulating progressively more intelligence.[1] Although Paul gives some evidential weight to this argument, in his view the key aspect missing from this story is optimisation for performance. Evolution wasn’t optimising for building organisms with high intelligence, it was simply optimising for genetic fitness. As soon as evolution stumbled into a region where these two coupled, we saw extremely fast progress. On the other hand, modern AI is being optimised for performance, with large sums of money being poured into the field, and an increasing understanding of the economic benefits of AIs capable of generality, so these discontinuities are less likely. As Scott Alexander puts it:
Imagine a company which, through some oversight, didn’t have a Sales department. They just sat around designing and manufacturing increasingly brilliant products, but not putting any effort into selling them. Then the CEO remembers they need a Sales department, starts one up, and the company goes from moving near zero units to moving millions of units overnight. It would look like the company had “suddenly” developed a “vast increase in capabilities”. But this is only possible when a CEO who is weirdly unconcerned about profit forgets to do obvious profit-increasing things for many years.
This is exactly what, according to Paul’s view, we shouldn’t expect to happen with AGI. Intense economic competition means “obvious profit-increasing things” are unlikely to be neglected. Million dollar bills tend not to lie on the sidewalk for very long.
Instead, Paul’s perspective of AI progress is based on the intuitive-seeming claim that, as he puts it:
For most X, it is easier to figure out how to do a slightly worse version of X than to figure out how to do X. If many people are trying to do X, and a slightly worse version is easier and almost-as-good, someone will figure out how to do the worse version before anyone figures out how to do the better version.
So when combined with the “optimisation for performance” argument, this would suggest that before we get superintelligent AI, we’ll get slightly-worse-than-human-level AI, which should still be enough to have radically transformative effects on the world.
One of Eliezer’s inside-view arguments for why we should expect a fast takeoff is recursive self-improvement. This is the idea that once an AI gets to a certain level, it will be able to accelerate AI research and so be able to build better AIs in a positive feedback loop that very quickly smashes up against the physical limits of intelligence. Paul’s reply to this is that - to the extent that “AIs improving AI research” is recognised as economically valuable - we should expect there to be AIs which are mediocre at self-improvement before there are AIs which are excellent at self-improvement. For example, Codex is a tool developed by OpenAI which powers GitHub Copilot, allowing programmers to write code from natural language descriptions. You could argue this is “AIs improving AI research” in some basic sense, but it definitely doesn’t represent an intelligence explosion.
As a more recent example of this idea, consider GATO [LW · GW] (the generalist agent designed by DeepMind). Its announcement sparked a lot of discussion on LessWrong, with it being described as “a gut shock [LW · GW]” and “sub-human-level AGI [LW · GW]”, and also “3 years over-due [LW · GW]”, “already priced in [LW · GW]” and “thoroughly underwhelming [LW · GW]”. On one hand, you could argue that GATO represents a weak version of generalisation, and so is consistent with Paul’s model of “impressive versions of things are usually preceded by slightly worse versions”. On the other hand, you could argue that it basically doesn’t capture generality in any important sense, so it’s meaningless to place it on a smooth trendline to AGI.
Although some of Paul and Eliezer’s disagreements can be attributed to contingent factors (e.g. regulatory barriers, and the ability of the AI industry to snap up performance gains as soon as they appear), there are also fundamental disagreements which stem from the nature of intelligence, and the extent to which inside-view considerations should push us away from the intuition of “progress tends to be gradual and continuous”.
Conversations on technology forecasting and gradualism
Gradualism and catastrophism represent two very different frameworks with which to look at the world. In Conversations on technology forecasting and gradualism [LW · GW], the participants (which include Paul and Eliezer as well as several other alignment researchers) discuss several domains which you could apply these frameworks to in order to generate predictions, and how well these match up to reality. This involves a lot of talking past each other, because the participants often have different interpretations of the different domains, and what lessons we should draw from them.
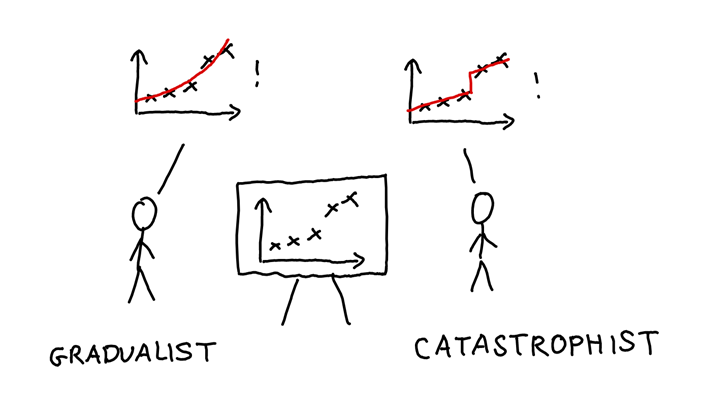
I will focus on three main domains in which the gradualist / catastrophist models are compared: historical examples of technological progress, startups and markets, and the skill distributions of people. These correspond to the three sections below. It’s worth mentioning that this decomposition is at least a little artificial, and there are situations which you could analyse through multiple different lenses.
What can history teach us?
Let’s return to Paul’s argument from earlier:
For most X, it is easier to figure out how to do a slightly worse version of X than to figure out how to do X. If many people are trying to do X, and a slightly worse version is easier and almost-as-good, someone will figure out how to do the worse version before anyone figures out how to do the better version.
One of the reasons he expects this is because it’s generally consistent with the historical record. So if you wanted to test this hypothesis, one thing you might do is study examples of discontinuous progress throughout history, examining their frequency as well as the size of the discontinuity and the reasons behind it.
Luckily, this is exactly what Katja Grace and the team at AI impacts have done! They looked for examples of discontinuously fast technological progress throughout history, where a discontinuity is measured by how many years of progress it represents, relative to the extrapolation of previous trends.
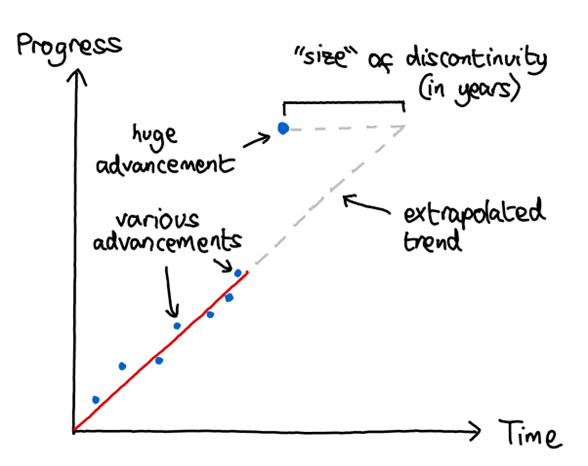
This methodology obviously has many difficulties. For instance, you need good data on what progress looked like before the trend, which isn’t always easy to find. Also, how to fit the pre-discontinuity trendline is very nontrivial: some technological metrics tend to advance at a linear rate (e.g. the ELO ratings of chess computers), whereas others tend to advance logarithmically, i.e. a certain percentage improvement over a fixed number of years (e.g. ship size). Overall, the team managed to find 10 events which produced robust and large discontinuities[2], over the 38 trends surveyed. The authors mention that this shouldn’t be taken as a prior on the rate of technological discontinuities, since their methodology was to “search for trends which might contain discontinuities” rather than to “survey trends independently and calculate frequency of discontinuities”. NunoSempere in the comments section of the MIRI dialogue attempts the latter, and arrives at a discontinuity rate of 24% - which is still higher than one might expect from the continuous change story.
What’s going on here? One possible explanation for the discontinuities is that most of them seem to fall into the category of “features” in Katja’s classification. Here, a feature should be understood as a characteristic which is generally good, but not close to capturing all the value produced. A problem with features relative to value proxies is that features are easier to Goodhart [? · GW]. For example, one discontinuity that made it onto the list was the Paris Gun: a long-range siege gun used by the Germans in WWI for the long-range bombardment of Paris (image below)[3]. It could launch a projectile over 40 kilometres into the air; six times the previous record for a height achieved by man-made means. However, it seems to have been more effective as a psychological tool than a practical weapon, and was generally not considered a military success. Despite the literal arms race going on, it seems to have been much easier to build a weapon that performed well on somewhat inconsequential metrics than one representing a phase change in the effectiveness of waging war. One could argue that we currently see these effects playing out for AI. It’s much easier to optimise for some particular AI performance metric than for making a breakthrough with a meaningful impact on the entire world economy.
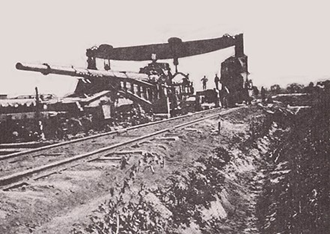
Another theme from the study was that many discontinuities come from “thresholds”. The basic idea here is that, if some new technology requires some minimum capability level to be viable, then the first time it becomes viable it outclasses all previous technologies. For example, the first non-stop transatlantic flight (1909) registered as a discontinuity in the fastest time to cross the Atlantic, because the first plane capable of this journey was much faster than the fastest ships at the time. If the Wright Flyer had the range to cross the Atlantic in 1903, then it probably wouldn’t have registered as a discontinuity, because it wouldn’t have been much faster than ships at the time. Should we expect something like this in AI, where we get a zero-one discontinuity in capabilities once a certain threshold has been crossed? Possibly. Although most metrics of AI performance on human tasks seem to have proceeded relatively continuously so far (even AlexNet, which was investigated by the AI Impacts team and is often cited as a discontinuity, didn’t make the list), if you agree with the “secret sauce” argument then you might expect AI to eventually cross some “generality threshold”, allowing it to perform multiple different tasks at human or superhuman levels.
Physics doesn’t care about your feelings
Before we get carried away with generalising from historical examples, one question needs to be answered: what can all these examples actually teach us? Maybe using them as reference classes for technological progress in AI is totally the wrong approach, because the similarities are either completely absent or small enough to make inside-view considerations about AI the dominant factor. This point is also made by Rob Bensinger in the discussion, who responds to Paul’s prior of “things are shitty / low-impact when they happen for the first time” with the following argument:
As I see it, physics 'doesn't care' about human conceptions of impactfulness, and will instead produce AGI prototypes, aircraft prototypes, and nuke prototypes that have as much impact as is implied by the detailed case-specific workings of general intelligence, flight, and nuclear chain reactions respectively.
When it comes to reasoning about intelligence, we’re already dealing with something that has been known to take over the world on at least one occasion - something you decidedly can’t say about most other technologies throughout history! So maybe we should just throw out base rates from historical progress, and focus on inside-view arguments about CS and human cognition.
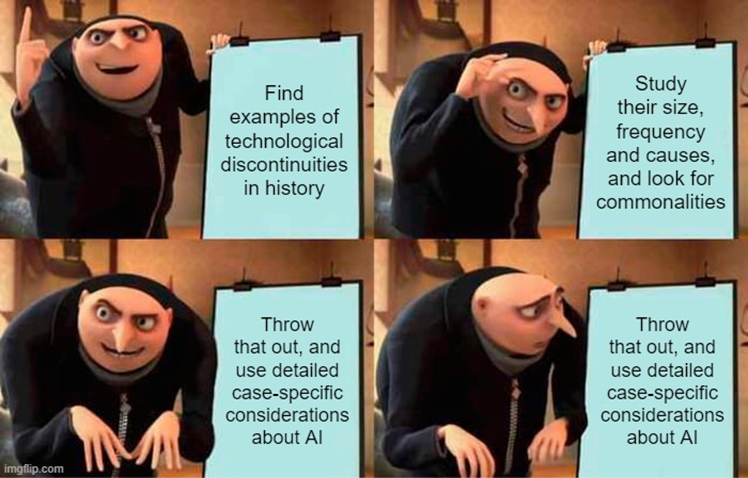
Rob uses nuclear weapons as an analogy:
If there's a year where explosives go from being unable to destroy cities to being able to destroy cities, we should estimate the impact of the first such explosives by drawing on our beliefs about how (current or future) physics might allow a city to be destroyed, and what other effects or side-effects such a process might have. We should spend little or no time thinking about the impactfulness of the first steam engine or the first telescope.
In response, Rohin Shah pushes back slightly on the nuclear weapons / AI analogy. He argues that there might have been good inside-view reasons to expect a discontinuity in nuclear weapons, before you tested them for the first time. In particular, nuclear weapons are based on “entirely new physics”, which should raise our priors for the existence of a discontinuity in explosive effectiveness, even if we don’t yet know how to perform the exact calculations. Does the “entirely new physics” analogy work for AIs? Rohin doesn’t think so:
I don't really see any particular detail for AI that I think I should strongly update on. My impression is that Eliezer thinks that "general intelligence" is a qualitatively different sort of thing than that-which-neural-nets-are-doing, and maybe that's what's analogous to "entirely new physics". I'm pretty unconvinced of this, but something in this genre feels quite crux-y for me.
On this view, it seems more reasonable to take the empirical observation of “historical discontinuities are infrequent” as an initial prior. Rohin replies to NunoSempere’s comment (where he suggests a discontinuity rate of 24%), pointing out some other reasons why it might be reasonable to have a lower prior than this for the specific case of AI, for instance:
- We can’t point to anything in the future analogous to “entirely new physics”
- The field of AI has existed for 60 years, so the low-hanging fruit might already have been picked[4]
- Expecting discontinuity around the point where AI reaches human-level intelligence is a more specific prediction than discontinuity somewhere along the curve
For these reasons, he argues that considerations like this should push our priors down much lower than 24%, maybe even as low as 5% (if ignoring meta-level uncertainty about the model).
A bias tug-of-war
So far, we’ve mainly discussed the importance of historical examples through the lens of domain similarities. In other words, why did this technological discontinuity happen, and are there any lessons from it which can generalise to AI progress? Richard Ngo argues that there are other ways in which historical examples can be informative. In particular, they can provide lessons about:
- Human effort and insight
- Human predictive biases
I’ll defer discussion of the first of these to the next section; for now let’s consider the second. The basic idea here is that if we have a systematic tendency to make incorrect predictions about technological progress, then studying historical examples can help inform us about this bias, and maybe the directions in which we should try and adjust our predictions. For instance, one plausible-sounding story is that we tend to overestimate the importance of prototypes and therefore the probability of discontinuities, maybe because of some kind of availability heuristic [? · GW]. Rob disagrees, arguing that this bias is unlikely to exist, and even if it did, we should try and stick to the object level. He returns to the example of trying to perform a priori reasoning about the effectiveness of nuclear weapons:
my intuition is still that you're better off … focusing on physics calculations rather than the tug-of-war 'maybe X is cognitively biasing me in this way, no wait maybe Y is cognitively biasing me in this other way, no wait...'
I think even if this is true, it’s important to be aware of biases because they can help improve rational discourse around this topic. For instance, after the announcement of GATO, Metaculus’ median prediction of the arrival time of AGI dropped by about a decade. On the other hand, many people on LessWrong argue that the advances GATO represents should have already been priced into prediction markets. If historical examples can hold lessons for us about biases like these, then it seems unwise to let those lessons go to waste.
Startups, secrets, and (in)efficient markets
Another lens that is used to analyse technological progress during this discussion is Peter Thiel’s concept of secrets. Secrets in this sense means important information which is knowable but not widely known, which you can use to get an edge in something. Thiel expands on this concept in his book Zero to One, where he describes loss of belief in secrets as an unfortunate trend in modern society, particularly for would-be entrepreneurs. From Scott Alexander’s book review:
Good startups require a belief in secrets, where “secret” is equivalent to “violation of the efficient market hypothesis”. You believe you’ve discovered something that nobody else has: for example, that if you set up an online bookstore in such-and-such a way today, in thirty years you’ll be richer than God.
In a follow up to an earlier MIRI dialogue [? · GW], Eliezer suggests that Paul’s “takeoff speeds” post is implicitly assuming a “perfectly anti-Thielian” worldview. In other words, he interprets Paul as saying that the path to AGI will be widely known, and widely recognised to be profitable. Almost all the major players will be pouring money and resources into it. Low hanging fruit will be rare. Eliezer thinks this is a possible way the world could have been, but it’s not the world we live in. Rather, we live in the world which failed to make the necessary preparations for Covid-19, which didn’t incentivise Japanese central bankers to have adequate predictions about monetary policy, and so on, through roughly a thousand more examples.
In contrast, Eliezer’s model assigns a much higher probability that Thielian secrets exist on the path to AGI. When the first AI project stumbles onto it, they will gain a decisive advantage over all other competitors, and we might just FOOM all the way up to superintelligence.
How accurately does this “anti-Thielian" interpretation actually represent Paul’s views? It’s difficult to say for sure, since Paul says during the discussion that he hasn’t read Thiel’s writing extensively, and just has a “vibe” of what Thielian secrets are. He summarises his beliefs on the topic as:
- Generally it takes a lot of work to do stuff, as a strong empirical fact about technology.
- Generally if the returns are bigger there are more people working on it, as a slightly-less-strong fact about sociology.
When combined, this seems to support a weak anti-Thielian position in which secrets do exist, but they are rare, and become even rarer in more competitive industries. For instance, you could argue that the Wright brothers discovered a secret, but this was possible largely because so few people were working on the problem of manned powered flight, and there wasn’t much economic value you could capture directly from being the first to crack it. In contrast, the secrets of AI are so economically valuable that everyone is competing to find them.
How efficient are markets, in the presence of large amounts of competition? Are there situations where we might expect them to fail to find secrets? It’s impossible to do justice to this question in a single post, ideally you’d need an entire book. One domain you might draw evidence from is the world of startups. Elon Musk has founded PayPal, SpaceX and Tesla - all highly successful companies which have disrupted their respective industries. Other examples of entrepreneurs who’ve had repeated success include Steve Jobs (who funded the group that was to become Pixar before he left for Apple), and Peter Thiel himself (who has had repeated investment success, and executed several grand long-term plans). In an anti-Thielian world, you might expect the occasional entrepreneur to hit on success by chance, but these examples are all repeat offenders. This is of course just anecdotal evidence, as Thiel himself admits. Returning to the original dialogue, Holden Karnofsky observes that Thiel has invested in many startups with grand plans and visions, and these have generally not come to fruition (or at least when they did, it was in a much smaller and less impressive form).
Regardless of the true story here, it seems dangerous to give too much evidential weight to arguments about market efficiency. Rob comments:
I can imagine an inside-view model … that tells you 'AGI coming from nowhere in 3 years is bonkers'; I can't imagine an ML-is-a-reasonably-efficient-market argument that does the same, because even a perfectly efficient market isn't omniscient and can still be surprised by undiscovered physics facts ...
Rob later clarifies that he does think this type of argument has some validity, and so depending on your beliefs about the efficiency of markets this might cause you to update towards one particular class of takeoff scenarios. It’s just that this argument fails to get you all the way to bonkers, in either direction. [5]
Sparse World Hypothesis
One way of framing gradualism is “with enough competition / optimisation for performance, discontinuities tend to get smoothed over”. In the first section, we talked about whether competition between designers of new technologies can smooth over discontinuities in history. In the second section, we talked about whether market competition in the world of startups and investors can smooth over discontinuities in financial success. Finally, we’ll test out gradualism in a third domain, by examining whether competition between individuals can smooth over discontinuities in the space of peoples’ intelligence, abilities, and accomplishments.
Eliezer opens this part of the discussion with the example of Steve Jobs trying to find a successor to take over Apple. According to this account, Jobs searched hard for someone who could rival him along important dimensions like UI taste, and even though he was able to pay a virtually unlimited amount of money as an incentive, his search was essentially unsuccessful.[6] A few other examples:
- Von Neumann - a polymath who made contributions in the fields of automata theory, economics, ergodic theory, digital computers, fluid dynamics, foundations of mathematics, and functional analysis (and that’s just up to “f”!)
- Peter Thiel and Elon Musk - as discussed in the previous section, they aren’t just one-time successes, they’re serial disruptors
- Eliezer himself - according to his own account, he searched very hard for people who would be able to progress beyond his contributions to the alignment problem (this was the main purpose of writing The Sequences), and he considers this endeavour as “having largely failed”
What should we learn from these examples? Eliezer suggests a “Sparse World Hypothesis”. As he describes it:
The shadow of an Earth with eight billion people, projected into some dimensions, is much sparser than plausible arguments might lead you to believe. Interesting people … cannot find other people who are 80% as good as what they do (never mind being 80% similar to them as people).
To understand this statement, first suppose we lived in a world where this hypothesis was false. You could imagine “ability to run a company” as being determined by some combination of skills, all of which are densely clustered around the population average. In this model, any datapoint you choose is likely to have several neighbours close to them in “personspace”, who can either exceed or closely approximate their skills in many different fields. This remains true even if certain skills are positively correlated - sampling from the upper end of the distribution will generate more impressive people, but nobody orders of magnitude more impressive than anyone else.
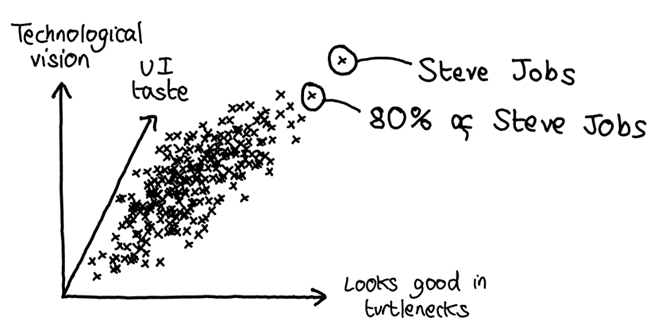
On the other hand, in a “Sparse World”, we could model peoples’ skills as a compounding process. Maybe there is a certain threshold which, once surpassed, allows you to gain compound interest in many different areas, in a way vaguely analogous to recursive self-improvement.
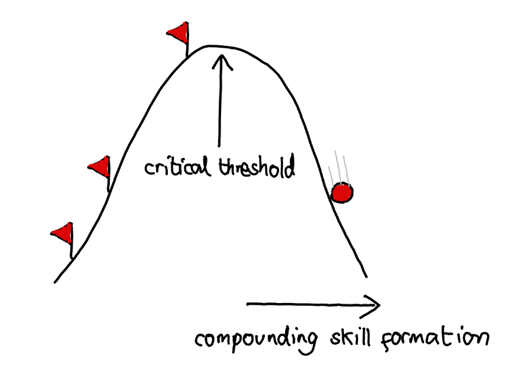
Eliezer argues that the “Sparse World” observation seems surprising, and isn’t something you’d predict in advance (and in fact he didn’t predict it; his writing of The Sequences represented a significant bet against it). Why would it have been hard to predict? The primary reason is that humans occupy a very small range of mind design space [LW · GW]. Biologically speaking, we are all very similar to each other, and so you might expect divergences between different humans’ intelligence and abilities to be relatively small. In other words, if gradualism holds up anywhere you’d expect it to hold up here - and yet gradualism makes the wrong predictions. As Eliezer puts it:
This is part of the story for why I put gradualism into a mental class of "arguments that sound plausible and just fail in real life to be binding on reality; reality says 'so what' and goes off to do something else".
What is Paul’s reply to this? Mainly, he says that gradualism in the space of humans doesn’t seem like the kind of gradualism which we can generalise to reason about AI progress. He suggests that the disagreement over which domains to apply gradualism to probably stem from fundamental disagreements about the nature of intelligence, which Eliezer seems to agree with.[7]
Unfortunately, this is a difficult issue to get past (and indeed the conversation between them doesn’t go much further after this point). In the LessWrong classic Is That Your True Rejection? [LW · GW], Eliezer suggests a few reasons why two rationalists might fail to resolve a disagreement which has persisted past their first discussion. Some of these include “long inferential distances [? · GW]”, “hard-to-verbalise intuitions” and “patterns perceptually recognised from experience” - the common theme being that they are all hard to communicate or expose. “Fundamental disagreements about the nature of intelligence” seems to fall squarely within this set.
Okay, so where do we go from here?
If you’ve read this far into the post, then you’ve probably noticed that the participants in these discussions often found it difficult to reach agreement. There was disagreement over interpretations of different domains such as technological progress and market efficiency, disagreement over those domains’ relevance for AI safety, and disagreement over the kinds of questions we should even be asking about AI takeoff speeds. Nate Soares makes the following observation at the end of the discussion:
It seems to me that another revealed intuition-difference is in the difficulty that people have operating each other's models. This is evidenced by, eg, Eliezer/Rob saying things like "I don't know how to operate the gradualness model without making a bunch of bad predictions about Steve Jobs", and Paul/Holden responding with things like "I don't know how to operate the secrets-exist model without making a bunch of bad predictions about material startups".
In a sense, it’s an unfortunate inevitability that these kinds of communication problems will exist. AI is still a relatively young field when viewed on the timeline of technological progress, while the difficulties of predicting the future are as old as history. The Paulverse and Eliezerverse differ in many ways, and offer few testable predictions that can help us determine which one (if either) we live in.
In “Everything Everywhere All At Once” (which has to be the strangest movie I’ve seen all year), the main character learns how to perform “verse-jumping” - tapping into one of many alternate universes in order to access her doppelganger’s knowledge and skills. But in doing so, she gradually loses her grip on reality, to the point where all the universes start to blur together, and she finds it increasingly hard to tell which one she’s currently inhabiting. If the alignment community can’t do a better job at figuring out what it means to live in the Paulverse or the Eliezerverse, then maybe we’ll end up stuck in limbo, in an epistemic state where we’re unable to decide what new developments tell us about future AI progress … and also maybe have hot-dogs for fingers. (This metaphor may have gotten away from me slightly.)
Agreeing to disagree
At the start of this post, I mentioned that one of the reasons the hard / soft takeoff picture matters is that it helps inform which directions in AI safety research are more valuable. But there is another reason: understanding different perspectives and frameworks on this issue can help build better inside views of AI safety, and what researchers’ theories of change are. From my own experience, I’ve spent the last year trying to engage with AI safety and the arguments surrounding it, and I’ve often found it very confusing how people can hold such differing opinions on which kinds of work are valuable. This is partly what we should expect with a pre-paradigmatic field, where we not only don’t have all the answers, but we don’t even know how to frame the questions. I hope that better understanding of where opinions differ in the alignment community, such as in dialogues like this, can help people in different fields of AI safety understand each other a little better.
- ^
Another perspective you could have here, which isn’t brought up as much during these discussions, comes from cultural evolution. This is explored more in Joseph Heinrich’s book The Secret of Our Success, where he argues that cultural transmission was the key to humans’ dominance in the natural world, rather than our individual intelligence. Compelling evidence for this comes from experiments in childhood learning, where human toddlers are close to apes on most intellectual performance measures, but absolutely clean up when it comes to social learning (ability to learn from others). One could argue that the speedup in human history occurred when we reached the threshold for cultural transmission, at which point cultural rather than genetic evolution drove most of our subsequent progress. For more on this topic, I highly recommend Scott Alexander’s book review.
- ^
Here, “large” here means a discontinuity of at least 100 years, and “robust” is a subjective judgement based on the quality of available trend data.
- ^
According to historical records, when the guns were first used, Parisians believed they were being bombed by a high-altitude Zeppelin, as the sound of neither an aeroplane nor a gun could be heard.
- ^
See the section “Startups, secrets, and (in)efficient markets” for a more thorough treatment of this line of argument.
- ^
It’s worth mentioning here that Paul agrees this argument shouldn’t be enough to update you to “AGI coming from nowhere in 3 years is bonkers”. In Takeoff speeds, he gives ~30% as his probability for fast takeoff.
- ^
One could of course dispute this particular case, although this isn’t explored much in the discussion, mainly because it doesn’t seem like a significant crux for either side.
- ^
Suggested drinking game: take a shot every time a disagreement caches out at “hard-to-verbalise disagreements about the fundamental nature of intelligence.” Proceed at your own peril...
1 comments
Comments sorted by top scores.
comment by Noosphere89 (sharmake-farah) · 2022-07-15T14:53:31.963Z · LW(p) · GW(p)
One important datapoint is progress on AI using various metrics has usually been the first time discontinuous, but after that things start decelerating into continuous growth. So that's a point in favor of gradualism.