AI alignment researchers don't (seem to) stack
post by So8res · 2023-02-21T00:48:25.186Z · LW · GW · 40 commentsContents
41 comments
(Status: another point I find myself repeating frequently.)
One of the reasons I suspect we need a lot of serial time [? · GW] to solve the alignment problem is that alignment researchers don't seem to me to "stack". Where “stacking” means something like, quadrupling the size of your team of highly skilled alignment researchers lets you finish the job in ~1/4 of the time.
It seems to me that whenever somebody new and skilled arrives on the alignment scene, with the sort of vision and drive that lets them push in a promising direction (rather than just doing incremental work that has little chance of changing the strategic landscape), they push in a new direction relative to everybody else. Eliezer Yudkowsky and Paul Christiano don't have any synergy between their research programs. Adding John Wentworth doesn't really speed up either of them. Adding Adam Shimi doesn't really speed up any of the previous three. Vanessa Kosoy isn't overlapping with any of the other four.
Sure, sometimes one of our visionary alignment-leaders finds a person or two that sees sufficiently eye-to-eye with them and can speed things along (such as Diffractor [LW · GW] with Vanessa, it seems to me from a distance). And with ops support and a variety of other people helping out where they can, it seems possible to me to take one of our visionaries and speed them up by a factor of 2 or so (in a simplified toy model where we project ‘progress’ down to a single time dimension). But new visionaries aren't really joining forces with older visionaries; they're striking out on their own paths.
And to be clear, I think that this is fine and healthy. It seems to me that this is how fields are often built, with individual visionaries wandering off in some direction, and later generations following the ones who figured out stuff that was sufficiently cool (like Newton or Laplace or Hamilton or Einstein or Grothendieck). In fact, the phenomenon looks even more wide-ranging than that, to me: When studying the Napoleonic wars, I was struck by the sense that Napoleon could have easily won if only he'd been everywhere at once; he was never able to find other generals who shared his spark. Various statesmen (Bismark comes to mind) proved irreplaceable. Steve Jobs never managed to find a worthy successor, despite significant effort.
Also, I've tried a few different ways of getting researchers to "stack" (i.e., of getting multiple people capable of leading research, all leading research in the same direction, in a way that significantly shortens the amount of serial time required), and have failed at this. (Which isn't to say that you can't succeed [LW · GW] where I failed!)
I don't think we're doing something particularly wrong here. Rather, I'd say: the space to explore is extremely broad; humans are sparsely distributed in the space of intuitions they're able to draw upon; people who have an intuition they can follow towards plausible alignment-solutions are themselves pretty rare; most humans don't have the ability to make research progress without an intuition to guide them. Each time we find a new person with an intuition to guide them towards alignment solutions, it's likely to guide them in a whole new direction, because the space is so large. Hopefully at least one is onto something.
But, while this might not be an indication of an error, it sure is a reason to worry. Because if each new alignment researcher pursues some new pathway, and can be sped up a little but not a ton by research-partners and operational support, then no matter how many new alignment visionaries we find, we aren't much decreasing the amount of time it takes to find a solution.
Like, as a crappy toy model, if every alignment-visionary's vision would ultimately succeed, but only after 30 years of study along their particular path, then no amount of new visionaries added will decrease the amount of time required from “30y since the first visionary started out”.
And of course, in real life, different paths have different lengths, and adding new people decreases the amount of time required at least a little in expectation. But not necessarily very much, and not linearly.
(And all this is to say nothing of how the workable paths might not even be visible to the first generation of visionaries; the intuitions that lead one to a solution might be the sort of thing that you can only see if you've been raised with the memes generated by the partial-successes and failures of failed research pathways, as seems-to-me to have been the case with mathematics and physics regularly in the past. But I digress.)
40 comments
Comments sorted by top scores.
comment by Zach Stein-Perlman · 2023-02-21T01:31:08.790Z · LW(p) · GW(p)
I largely agree. But I think not-stacking is only slightly bad because I think the "crappy toy model [where] every alignment-visionary's vision would ultimately succeed, but only after 30 years of study along their particular path" is importantly wrong; I think many new visions have a decent chance of succeeding more quickly and if we pursue enough different visions we get a good chance of at least one paying off quickly.
Edit: even if alignment researchers could stack into just a couple paths, I think we might well still choose to go wide.
Replies from: Roman Leventov, Seth Herd, firstuser-here, joshua-clymer↑ comment by Roman Leventov · 2023-02-22T10:49:05.746Z · LW(p) · GW(p)
I disagree with this view that someone's vision could "succeed" in some sense. Rather, all visions (at least if they are scientifically and methodologically rigorous), if actually applied in AGI engineering, will increase the chances that the given AGI will go well.
However, at this stage (AGI very soon, race between AGI labs) it's now the time to convince AGI labs to use any of the existing visions, apart from (and in parallel with, not instead) their own. While simultaneously, trying to make these visions more mature. In other words: researchers shouldn't "spread" and try to "crack alignment" independently from each other. Rather, they should try to reinforce existing visions (while people with social capital and political skill should try to convince AGI labs to use these visions).
The multi-disciplinary (and multi-vision!) view on AI safety [LW · GW] includes the model above, however, I haven't elaborated on this exact idea yet (the linked post describes the technical side of the view, and the social/strategic/timelines-aware argument for the multi-disciplinary view is yet to be written).
↑ comment by Seth Herd · 2023-02-22T01:42:28.870Z · LW(p) · GW(p)
This seems like a better model of the terrain: we don't know how far down which path we need to get to find a working alignment solution. So the strategy "let's split up to search, gang; we'll cover more ground" actually makes sense before trying to stack efforts in the same direction.
Replies from: NicholasKross↑ comment by Nicholas / Heather Kross (NicholasKross) · 2023-03-03T23:53:23.761Z · LW(p) · GW(p)
Yeah, basically explore-then-exploit. (I do worry that the toy model is truer IRL though...)
↑ comment by 1stuserhere (firstuser-here) · 2023-02-23T13:41:37.080Z · LW(p) · GW(p)
I agree. It seems like striking a balance between exploration and exploitation. We're barely entering the 2nd generation of alignment researchers. It's important to generate new directions of approaching the problem especially at this stage, so that we have a better chance of covering more of the space of possible solutions before deciding to go in deeper. The barrier to entry also remains slightly lower in this case for new researchers. When some research directions "outcompete" other directions, we'll naturally see more interest in those promising directions and subsequently more exploitation, and researchers will be stacking.
↑ comment by joshc (joshua-clymer) · 2023-03-14T05:55:10.122Z · LW(p) · GW(p)
+1. As a toy model, consider how the expected maximum of a sample from a heavy tailed distribution is affected by sample size. I simulated this once and the relationship was approximately linear. But Soares’ point still holds if any individual bet requires a minimum amount of time to pay off. You can scalably benefit from parallelism while still requiring a minimum amount of serial time.
comment by Jacob_Hilton · 2023-02-21T17:28:33.507Z · LW(p) · GW(p)
I think the examples you give are valid, but there are several reasons why I think the situation is somewhat contingent or otherwise less bleak than you do:
- Counterexamples: I think there are research agendas that are less pre-paradigmatic than the ones you're focused on that are significantly more (albeit not entirely) parallelizable. For example, mechanistic interpretability and scalable oversight both have multiple groups focused on them and have grown substantially over the last couple of years. I'm aware that we disagree about how valuable these directions are.
- Survival of the fittest: Unfortunately I think in cases where an individual has been pursuing a research direction for many years and has tried but failed to get anyone else on board with it, there is some explanatory power to the hypothesis that the direction is not that productive. Note that I'm not claiming to have a strong view on any particular agenda, and there are of course other possibilities in any given case. On the flip side, I expect promising directions to gain momentum over time, even if only gradually, and I consider the counterexamples from point 1 to be instances of this effect.
- Fixable coordination/deference failures: I think it would be a mistake for absolutely everyone to go off and try to develop their own alignment strategy from scratch, and it's plausible that the group you're focused on is erring too far in this direction. My own strategy has been to do my best to develop my own inside view (which I think is important for research prioritization and motivation as well from a group epistemics perspective), use this to narrow down my set of options to agendas I consider plausible, but be considerably more willing to defer when it comes to making a final call about which agenda to pursue.
- Clarity from AI advances: If the risk from AI is real, then I expect the picture of it to become clearer over time as AI improves. As a consequence, it should become clearer to people which directions are worth pursuing, and theoretical approaches should evolve into practical ones than can be iterated on empirically. This should both cause the field to grow and lead to more parallelizable work. I think this is already happening, and even the public at large is picking up on the spookiness of current alignment failures (even though the discourse is unsurprisingly very muddled).
comment by Jan_Kulveit · 2023-02-22T11:45:18.961Z · LW(p) · GW(p)
Yes, the non-stacking issue in the alignment community is mostly due to the nature of the domain
But also partly due to the LessWrong/AF culture and some rationalist memes. For example, if people had stacked on Friston et. al., the understanding of agency and predictive systems (now called "simulators") in the alignment community could have advanced several years faster. However, people seem to prefer reinventing stuff, and formalizing their own methods. It's more fun... but also more karma.
In conventional academia, researchers are typically forced to stack. If progress is in principle stackable, and you don't do it, it won't be published. This means that even if your reinvention of a concept is slightly more elegant or intuitive to you, you still need to stack. This seems to go against what's fun: I think I don't know any researcher who would be really excited about literature reviews and prefer that over thinking and writing their own ideas. In the absence of incentives for stacking ... or actually presence of incentives against stacking ... you get a lot of non-stacking AI alignment research.
↑ comment by Noosphere89 (sharmake-farah) · 2023-02-22T15:20:23.986Z · LW(p) · GW(p)
comment by Adele Lopez (adele-lopez-1) · 2023-02-21T01:38:21.573Z · LW(p) · GW(p)
It does seem like a bad sign to me if the insights generated by these visionaries don't seem to be part of the same thing enough that they build off each other at least a little bit. Which makes me wonder what an elementary textbook coauthored by all the major AI alignment researchers would look like... what are the core things they all agree it's important to know?
Replies from: DragonGod, tamgent, Aprillion↑ comment by Aprillion · 2023-02-23T12:39:45.842Z · LW(p) · GW(p)
Building a tunnel from 2 sides is the same thing even if those 2 sides don't see each other initially. I believe some, but not all, approaches will end up seeing each other, that it's not a bad sign if we are not there yet.
Since we don't seem to have time to build 2 "tunnels" (independent solutions to alignment), a bad sign would be if we could prove all of the approaches are incompatible with each other, which I hope is not the case.
Replies from: firstuser-here↑ comment by 1stuserhere (firstuser-here) · 2023-02-23T13:46:02.255Z · LW(p) · GW(p)
In this analogy, the trouble is, we do not know whether we're building tunnels in parallel (same direction) or the opposite, or zig zag. The reason for that is a lack of clarity about what will turn out to be a fundamentally important approach towards building a safe AGI. So, it seems to me that for now, exploration for different approaches might be a good thing and the next generation of researchers does less digging and is able to stack more on the existing work
comment by Past Account (zachary-robertson) · 2023-02-21T17:31:43.107Z · LW(p) · GW(p)
[Deleted]
comment by Thane Ruthenis · 2023-02-21T02:33:15.801Z · LW(p) · GW(p)
I think it's mostly right, in the sense that any given novel research artifact produced by Visionary A is unlikely to be useful for whatever research is currently pursued by Visionary B. But I think there's a more diffuse speed-up effect from scale, based on the following already happening:
the intuitions that lead one to a solution might be the sort of thing that you can only see if you've been raised with the memes generated by the partial-successes and failures of failed research pathways
The one thing all the different visionaries pushing in different directions do accomplish is mapping out the problem domain. If you're just prompted with the string "ML research is an existential threat", and you know nothing else about the topic, there's a plethora of obvious-at-first-glance lines of inquiry you can go down. Would prosaic alignment somehow not work, and if yes, why? How difficult would it be to interpret a ML model's internals? Can we prevent a ML model from becoming an agent? Is there some really easy hack to sidestep the problem? Would intelligence scale so sharply that the first AGI failure kills us all? If all you have to start with is just "ML research is an existential threat", all of these look... maybe not equally plausible, but not like something you can dismiss without at least glancing in that direction. And each glance takes up time.
On the other hand, if you're entering the field late, after other people have looked in these directions already, surveying the problem landscape is as easy as consuming their research artifacts. Maybe you disagree with some of them, but you can at least see the general shape of the thing, and every additional bit of research clarifies that shape even further. Said "clarity" allows you to better evaluate the problem, and even if you end up disagreeing with everyone else's priorities, the clearer the vision, the better you should be able to triangulate your own path.
So every bit of research probabilistically decreases the "distance" between the solution and the point at which a new visionary starts. Orrr, maybe not decreases the distance, but allows a new visionary to plot a path that looks less like a random walk and more like a straight line.
comment by Raemon · 2023-03-03T05:34:28.785Z · LW(p) · GW(p)
Curated.
This seems like a really important crux for alignment strategy. It seems like there are some influential people who disagree with this, and I'd really like them to lay out the counter-case.
I'd also be interested in historian/freelance-researcher types digging into the evidence here – something like, making a list of important discoveries, and looking into how those discoveries got made, and seeing whether the parallel/serial ontology seems like a useful framework. (I'm not sure of a good methodology for finding 'important discoveries', but, seems like the sort of thing AI Impacts or similar researchers could figure out)
comment by Christopher King (christopher-king) · 2023-02-21T05:30:32.900Z · LW(p) · GW(p)
Like, as a crappy toy model, if every alignment-visionary's vision would ultimately succeed, but only after 30 years of study along their particular path, then no amount of new visionaries added will decrease the amount of time required from “30y since the first visionary started out”.
A deterministic model seems a bit weird 🤔. I'm imagining something like an exponential distribution. In that case, if every visionary's project has an expected value of 30 years, and there are n visionaries, then the expected value for when the first one finishes is 30/n years. This is exactly the same as if they were working together on one project.
You might be able to get a more precise answer by trying to statistically model the research process (something something complex systems theory). But unfortunately, determining the amount of research required to solve alignment seems doubtful, which hampers the usefulness. :P
comment by Aprillion · 2023-02-23T16:32:42.489Z · LW(p) · GW(p)
I agree with the explicitly presented evidence and reasoning steps, but one implied prior/assumption seems to me so obscenely wrong (compared to my understanding about social reality) that I have to explain myself before making a recommendation. The following statement:
“stacking” means something like, quadrupling the size of your team of highly skilled alignment researchers lets you finish the job in ~1/4 of the time
implies a possibility that approximately neg-linear correlation between number of people and time could exist (in multidisciplinary software project management in particular and/or in general for most collective human endeavors). The model of Nate that I have in my mind believes that reasonable readers ought to believe that:
- as a prior, it's reasonable to expect more people will finish a complex task in less time than fewer people would, unless we have explicit reasons to predict otherwise
- Brooks's law is a funny way to describe delayed projects with hindsight, not a powerful predictor based on literally every single software project humankind ever pursued
I am making a claim about the social norm that it's socially OK to assume other people can believe in linear scalability, not a belief whether other people actually believe that 4x the people will actually finish in 1/4 time by default.
Individually, we are well calibrated to throw a TypeError at the cliche counterexamples to the linear scalability assumption like "a pregnant woman delivers one baby in 9 months, how many ...".
And professional managers tend to have an accurate model of applicability of this assumption, individually they all know how to create the kind of work environment that may open the possibility for time improvements (blindly quadrupling the size of your team can bring the project to a halt or even reverse the original objective, more usually it will increase the expected time because you need to lower other risks, and you have to work very hard for a hope of 50% decrease in time - they are paid to believe in the correct model of scalability, even in cases when they are incentivized to say more optimistic professions of belief in public).
Let's say 1000 people can build a nuclear power plant within some time unit. Literally no one will believe that one person will build it a thousand times slower or that a million people will build it a thousand times faster.
I think it should not be socially acceptable to say things that imply that other people can assume that others might believe in linear scalability for unprecedented large complex software projects. No one should believe that only one person can build Aligned AGI or that a million people can build it thousand times faster than a 1000 people. Einstein and Newton were not working "together", even if one needed the other to make any progress whatsoever - the nonlinearity of "solving gravity" is so qualitatively obvious, no one would even think about it in terms of doubling team size or halving time. That should be the default, a TypeError law of scalability.
If there is no linear scalability by default, Alignment is not an exception to other scalability laws. Building unaligned AGI, designing faster GPUs, physically constructing server farms, or building web apps ... none of those are linearly scalable, it's always hard management work to make a collective human task faster when adding people to a project.
Why is this a crux for me? I believe the incorrect assumption leads to rationally-wrong emotions in situations like these:
Also, I've tried a few different ways of getting researchers to "stack" (i.e., of getting multiple people capable of leading research, all leading research in the same direction, in a way that significantly shortens the amount of serial time required), and have failed at this.
Let me talk to you (the centeroid of my models of various AI researchers, but not any one person in particular). You are a good AI researcher and statistically speaking, you should not expect you to also be an equally good project manager. You understand maths and statistically speaking, you should not expect you to also be equally good at social skills needed to coordinate groups of people. Failling at a lot of initial attempts to coordinate teams should be the default expectation - not one or two attempts and then you will nail it. You should expect to fail more ofthen than the people who are getting the best money in the world for aligning groups of people towards a common goal. If those people who made themselves successful in management initially failed 10 times before they became billionaires, you should expect to fail more times than that.
Recommendation
You can either dilute your time by learning both technical and social / management skills or you can find other experts to help you and delegate the coordination task. You cannot solve Alignment alone, you cannot solve Alignment without learning, and you cannot learn more than one skill at a time.
The surviving worlds look like 1000 independent alignment ideas, each pursued by 100 different small teams. Some of the teams figured out how to share knowledge between some of the other teams and connect one or two ideas and merge teams iff they figure out explicit steps how to shorten time by merging teams.
We don't need to "stack", we need to increase the odds of a positive black swan.
Yudkowsky, Christiano, and the person who has the skills to start figuring out the missing piece to unify their ideas are at least 10,000 different people.
comment by trevor (TrevorWiesinger) · 2023-02-21T01:25:35.955Z · LW(p) · GW(p)
I've actually worked on a toy model related to producing alignment researchers, although the visionary-focused angle is a new one and I'd like to update it so it puts more emphasis on sourcing high-impact individuals and less on larger numbers of better rank-and-file workers. I'm definitely interested in improving it, especially if it's wildly inaccurate.
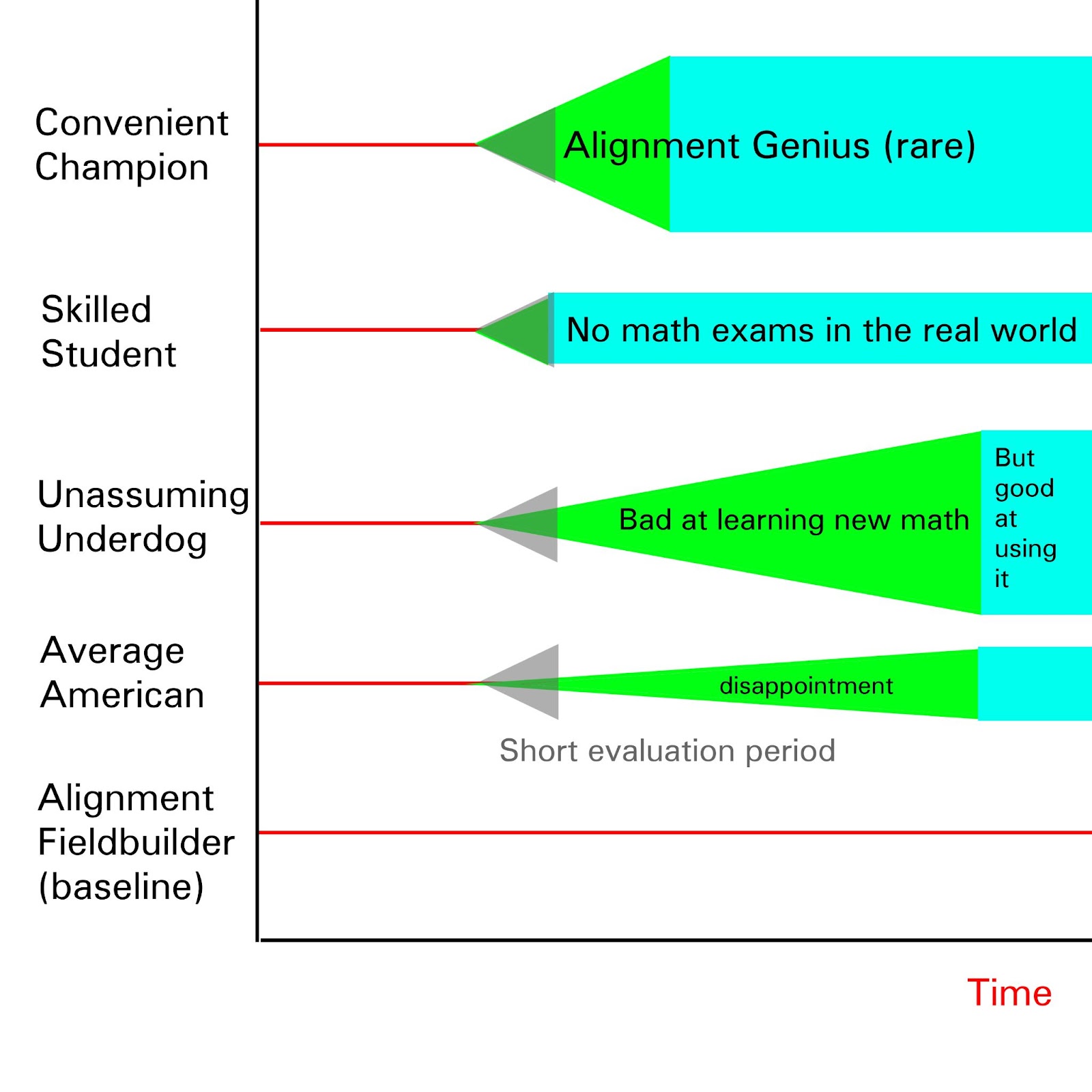
My understanding is that attempting to find people who have high output per year requires knowing the ratio of Convenient Champions to Skilled Students to Unassuming Underdogs to Average Americans. Then, after finding their relative frequency in the pool, the hard part is during the evaluation period, where it's a question of how good you can get at distinguishing Skilled Students and Convenient Champions, who learn math quickly and distinguish themselves on their own, and how well you can distinguish Unassuming Underdogs and Average Americans, who learn new kinds of quantitative thinking more slowly but the unassuming underdogs end up very skilled at applying them and end up as high-impact champions after a long waiting period.
My understanding of the current strategy described here is that we wait for people to get good at alignment on their own, even though there's only ~300 people on earth [EA · GW] who end up working on tasks that vaguely sound like AI safety. This implies that we have a pool of <500 people currently skilling up to work on AI safety (probably significantly less), and we... just... wait for geniuses to figure things out on their own, get good at distinguishing themselves on their own, do everything right, pop out of the pool on their own, all with zero early detection or predictive analytics, and we got ~5 people that way? Even though there's like 200,000 people on earth with +4 SD? And we're just crossing our fingers hoping for summoned heroes to show up at berkeley and save the day?
Replies from: None↑ comment by [deleted] · 2023-02-21T06:42:29.993Z · LW(p) · GW(p)
Also look at the inverse direction. Right now, we finally found a trick that works well enough to form a useful AI. And it has serious issues (with hallucination, being threatening or creepy, or making statements that disagree with a web search or writing code that doesn't compile and work). So to fix those issues, now that we know about them, there are straightforward things to try to fix the issues.*
...Would an alignment genius of any stripe, without knowing the results, have been able to predict the issues current models have and design algorithms for the countermeasures?
This is a testable query. Did anyone in the alignment field predict (1) the current problems with hallucination** (2) propose a solution?
*recursion. It appears that current models can examine text that happens to be their own output and can be prompted to inspect it for errors in logic and fact. This suggests a way to fix this particular issue with current scale llms.
**how did they do it. The field of mathematics doesn't cover complex networks of arbitrary learned functions hallucinating information does it...
Another point: for fission, there are high performance reactor designs that can never be safe. Possibly for AGI this is the same, that only very restricted, specific designs are mostly safe, and anything else is not. There may not exist a general alignment solution. There is not for fission, and high performance reactors (like nuclear salt water rocket engines) are absurdly unsafe.
For fission it also took years and thousands of people working on it, armed with data from previous reactors and previous meltdowns, before they arrived at Mostly Safe designs.
comment by TekhneMakre · 2023-02-21T02:17:27.917Z · LW(p) · GW(p)
Einstein or Grothendieck
My passing impression was that both of these were very greatly amplified by collaborations with mathematicians.
comment by joshc (joshua-clymer) · 2023-03-14T06:16:36.921Z · LW(p) · GW(p)
The analogy between AI safety and math or physics is assumed it in a lot of your writing and I think it is a source of major disagreement with other thinkers. ML capabilities clearly isn’t the kind of field that requires building representations over the course of decades.
I think it’s possible that AI safety requires more conceptual depth than AI capabilities; but in these worlds, I struggle to see how the current ML paradigm coincides with conceptual ‘solutions’ that can’t be found via iteration at the end. In those worlds, we are probably doomed so I’m betting on the worlds in which you are wrong and we must operate within the current empirical ML paradigm. It’s odd to me that you and Eliezer seem to think the current situation is very intractable, and yet you are confident enough in your beliefs to where you won’t operate on the assumption that you are wrong about something in order to bet on a more tractable world.
Replies from: sharmake-farah↑ comment by Noosphere89 (sharmake-farah) · 2023-09-28T16:25:02.629Z · LW(p) · GW(p)
This is definitely an underrated point. In general, I tend to think that worlds where Eliezer/Nate Soares/Connor Leahy/very doomy people are right are worlds where humans just can't do much of anything around AI extinction risks, and that the rational response is essentially to do what Elizabeth's friend did here in the link below, which is to leave AI safety/AI governance and do something else worthwile, as you or anyone else can't do anything:
https://www.lesswrong.com/posts/tv6KfHitijSyKCr6v/?commentId=Nm7rCq5ZfLuKj5x2G [LW · GW]
In general, I think you need certain assumptions in order to justify working either on AI safety or AI governance, and that will set at least a soft ceiling on how doomy you can be. One of those is the assumption of feedback loops are available, which quite obviously rules out a lot of sharp left turns, and in general there's a limit to how extreme your scenarios for difficulty of safety have to be before you can't do anything at all, and I think a lot of classic Lesswrong people like Nate Soares and Eliezer Yudkowsky, as well as more modern people like Connor Leahy are way over the line of useful difficulty.
comment by Dave Kinard (dave-kinard) · 2023-03-12T12:23:21.616Z · LW(p) · GW(p)
Hi. First time commenter.
Not sure where "newbie" questions go, but this was a post about alignment. I had a basic question I posted in a group- I was wondering about this and figured others here would know the answer.
"I'm not a programmer, but I had a question regarding AI alignment. I read the example on ACT of the AI assigned to guard a diamond and how it could go for tricking the sensory apparatus. What if you different AIs that had different goals, i.e., different ways of detecting the diamond's presence, and a solution was only approved if it met all the AIs goals? For other things, you could have one goal be safety that is given higher priority, a second AI with the original goal, and a third to "referee" edge cases? In general, the problem seems to be that AIs are good at doing what we tell them but we're not good at telling them what we want. What about "Balance of powers"? Different AIs with different, competing goals, where a solution only "works" when it satisfies the goals of each who are wary of being fooled by other ais? It seems to be that balancing of goals is more akin to "actual" intelligence. You could even then have top AIs to evaluate how the combined processes of the competing AI worked and have it able to alter their program within parameters but have that Top AI checked by other AIs. I'm sure I'm not the first person to have thought of this, as the adversarial process is used in other ways. Why wouldn't this work for alignment?"
comment by the gears to ascension (lahwran) · 2023-02-21T09:18:21.474Z · LW(p) · GW(p)
They stack a little, but it's fine, the winning ticket through the alignment research task is pretty damn short. We just need to find the combination of people who have the appropriate insights and connect them together with the research that gives them the context they need to understand it.
comment by Gunnar_Zarncke · 2023-02-21T12:11:58.673Z · LW(p) · GW(p)
This suggests that there should be a focus on fostering the next generation of AI researchers by
- making the insights and results from the pioneers accessible and interesting and
- making it easy to follow in the footsteps with a promise to reach the edge quickly.
comment by bvbvbvbvbvbvbvbvbvbvbv · 2023-02-21T14:57:25.738Z · LW(p) · GW(p)
My first thought : examples that come to my mind of brilliant minds stacked against a problem were incredibly successful :
- Manhattan project
- Moon race
↑ comment by Carl Feynman (carl-feynman) · 2023-02-23T14:47:21.352Z · LW(p) · GW(p)
You’re preferentially remembering programs that came to a successful conclusion. Counterbalance the Apollo Project with Project Pluto, Project Orion, the X-33, and the National Aero-Space Plane, which consumed lots of effort and never resulted in flyable products. Camp Century and the larger Project Iceworm turned out not to be a good idea once they tried it. The Japanese Fifth Generation project was a total washout. Also consider the War on Cancer and the Space Shuttle, which produced results, but far less than the early proponents imagined.
None of these seemed like dumb ideas going in.
Replies from: lcmgcd↑ comment by lemonhope (lcmgcd) · 2023-03-05T17:41:29.210Z · LW(p) · GW(p)
Do you think there might be a simple difference between the successes and failures here that we could learn from?
Replies from: carl-feynman↑ comment by Carl Feynman (carl-feynman) · 2023-05-04T15:44:27.868Z · LW(p) · GW(p)
If there was a simple difference, it would already have been noticed and acted on.
Replies from: lcmgcd↑ comment by lemonhope (lcmgcd) · 2023-05-04T18:47:45.483Z · LW(p) · GW(p)
Some things are easy to notice and hard to replicate
comment by Linda Linsefors · 2023-03-11T21:28:38.271Z · LW(p) · GW(p)
Like, as a crappy toy model, if every alignment-visionary's vision would ultimately succeed, but only after 30 years of study along their particular path, then no amount of new visionaries added will decrease the amount of time required from “30y since the first visionary started out”.
I think that a closer to true model is that most current research directions will lead approximately no-where but we don't know until someone goes and check. Under this model adding more researchers increases the probability that at least someone is working on fruitful research direction. And I don't think you (So8res) disagree, at least not completely?
I don't think we're doing something particularly wrong here. Rather, I'd say: the space to explore is extremely broad; humans are sparsely distributed in the space of intuitions they're able to draw upon; people who have an intuition they can follow towards plausible alignment-solutions are themselves pretty rare; most humans don't have the ability to make research progress without an intuition to guide them. Each time we find a new person with an intuition to guide them towards alignment solutions, it's likely to guide them in a whole new direction, because the space is so large. Hopefully at least one is onto something.
I do think that researchers stack, because there are lots of different directions that can and should be explored in parallel. So maybe the crux is to what fraction of people can do this? Most people I talk to do have research intrusions. I think it takes time and skill to cultivate one's intuition into an agenda that one can communicate to others, but just having enough intuition to guide one self is a much lower bar. However most people I talk to think they have to fit into someone else's idea of what AIS research look like in order to get paid. Unfortunately I think this is a correct belief for everyone without exceptional communication skills and/or connections. But I'm honestly uncertain about this, since I don't have a good understanding of the current funding landscape.
A side from money there are also imposter-syndrom type effects going on. A lot of people I talk to don't feel like they are allowed to have their own research direction, for vague social reasons. Some things that I have noticed sometimes helps:
- Telling them "Go for it!", and similar things. Repletion helps.
- Talking about how young AIS is as a field, and the implications of this, including the fact that their intrusions about the importance of expertise is probably wrong when applied to AIS.
- Handing over a post-it note with the text "Hero Licence".
comment by Adam Zerner (adamzerner) · 2023-03-03T06:18:53.350Z · LW(p) · GW(p)
Does intellectual progress stack in other fields? If so, I'd think that that would point decently strongly towards it stacking in AI alignment, in which case the question becomes how to get it to stack here. If not it points towards it perhaps not being worth pursuing further. If we don't know or feel confident, it seems like something worth researching. And funnily enough, that is something that seems pretty easily stackable!
comment by Review Bot · 2024-03-12T20:04:45.115Z · LW(p) · GW(p)
The LessWrong Review [? · GW] runs every year to select the posts that have most stood the test of time. This post is not yet eligible for review, but will be at the end of 2024. The top fifty or so posts are featured prominently on the site throughout the year.
Hopefully, the review is better than karma at judging enduring value. If we have accurate prediction markets on the review results, maybe we can have better incentives on LessWrong today. Will this post make the top fifty?
comment by metacoolus · 2023-06-08T22:25:11.394Z · LW(p) · GW(p)
That's an interesting perspective. This suggests that rather than creating research teams that are doing the same thing together, it would be better to encourage a wider diversity of approaches in attempts to get different intuition.
This might raise the question of what’s more valuable to the field - researchers with the ability to expand upon specific, targeted direction or researchers with new ideas that open up additional areas?
Perhaps part of the solution is recognizing that both attributes can be mutually beneficial in different ways. While efficiency is certainly a concern, exclusivity and narrow scope may be a more significant limiting factor. This reminds me of the principle that the pursuit of rationality should encompass a broad range of topics beyond just its own sake. Exploration and experimentation may be more valuable than strictly being directed in a single channel. The idea that people can only be sped up to a certain extent is also worth noting. At some point, adding more people to an existing vision loses effectiveness.
comment by Charlie Sanders (charlie-sanders) · 2023-03-12T22:33:08.451Z · LW(p) · GW(p)
Just to call it out, this post is taking the Great Man Theory of historical progress as a given, whereas my understanding of the theory is that it’s highly disputed/controversial in academic discourse.
Replies from: sharmake-farah↑ comment by Noosphere89 (sharmake-farah) · 2023-03-12T23:02:19.789Z · LW(p) · GW(p)
I'll admit, my prior expectations on Great Man Theory is that it's probably right, if not in every detail, due to the heavy tail of impact, where a few people generate most of the impact.
comment by Htarlov (htarlov) · 2023-03-07T01:22:35.769Z · LW(p) · GW(p)
I don't think "stacking" is a good analogy. I see this process as searching through some space of the possible solutions and non-solutions to the problem. Having one vision is like quickly searching from one starting point and one direction. This does not guarantee that the solution will be found more quickly as we can't be sure progress won't be stuck in some local optimum that does not solve the problem, no matter how many people work on that. It may go to a dead end with no sensible outcome.
For a such complex problem, this seems pretty probable as the space of problem solutions is likely also complex and it is unlikely that any given person or group has a good guess on how to find the solution.
On the other hand, starting from many points and directions will make each team/person progress slower but more of the volume of the problem space will be probed initially. Possibly some teams will sooner reach the conclusion that their vision won't work or is too slow to progress and move to join more promising visions.
I think this is more likely to converge on something promising in a situation when it is hard to agree on which vision is the most sensible to investigate.
comment by qjh (juehang) · 2023-02-21T15:38:24.408Z · LW(p) · GW(p)
But, while this might not be an indication of an error, it sure is a reason to worry. Because if each new alignment researcher pursues some new pathway, and can be sped up a little but not a ton by research-partners and operational support, then no matter how many new alignment visionaries we find, we aren't much decreasing the amount of time it takes to find a solution.
I'm not really convinced by this! I think a way to model this would be to imagine the "key" researchers as directed MCMC agents exploring the possible solution space. Maybe something like HMC, their intuition is modelled by the fact that they have a hamiltonian to guide them instead of being random-walk MCMC. Even then, having multiple agents would allow for the relevant minima to be explored much more quickly.
Taking this analogy further, there is a maximum number of agents beyond which you won't be mapping out the space more quickly. This is because chains need some minimum length for burn-in, to discard correlated samples, etc. In the research world, I think it just means people take a while to get their ideas somewhere useful, and subsequent work tends to be evolutionary instead of revolutionary over short time-scales; only over long time-scales does work seem revolutionary. The question then is this: are we in the sparse few-agents regime, or in the crowded many-agents regime? This isn't my field, but if I were to hazard a guess as an outsider, I'd say it sure feels like the former. In the latter, I'd imagine most people, even extremely productive researchers, would routinely find their ideas to have already been looked at before. It feels like that in my field, but I don't think I am a visionary my ideas are likely more "random-walk" than "hamiltonian".