On not getting contaminated by the wrong obesity ideas
post by Natália (Natália Mendonça) · 2023-01-28T20:18:21.322Z · LW · GW · 68 commentsContents
Summary of main points It’s not clear that lab animals *or* wild animals have been getting fatter Lab mice Wild animals There were meaningful changes in obesity rates before the late 20th century Being underweight is less common than it used to be, not more The altitude effect Hypobaric confinement seems to cause a lot of weight loss: the three Operations Everest and other studies Normobaric hypoxic confinement, too, seems to cause weight loss, in both humans and animals Humans Animals Food is apparently less appetizing in hypobaric conditions The dose-response relationship between altitude and body weight seems incompatible with the contamination hypothesis Demographic factors and altitude could adequately explain the geospatial distribution of obesity in the US Foreign-born population Race and ethnicity Education High-obesity states do poorly on a lot of different health metrics, not just obesity Contaminants are not needed to explain the obesogenic effects of palatable human food On Some Conceptions Of CICO Disclaimer Overeating for weeks != overeating for decades Equivocating between different meanings of the word “overeat” is not a good argument CICO is compatible with body weight regulation Under the CICO model, a lot of things are allowed to influence body weight Conclusion Acknowledgments Addenda SMTM’s posts still have factual inaccuracies and misrepresentations of sources (Non-exhaustive) errata/changelog None 69 comments
A Chemical Hunger (a), a series by the authors of the blog Slime Mold Time Mold (SMTM), argues that the obesity epidemic is entirely caused (a) by environmental contaminants.
In my last post [LW · GW], I investigated SMTM’s main suspect (lithium).[1] This post collects other observations I have made about SMTM’s work, not narrowly related to lithium, but rather focused on the broader thesis of their blog post series.
I think that the environmental contamination hypothesis of the obesity epidemic is a priori plausible. After all, we know that chemicals can affect humans, and our exposure to chemicals has plausibly changed a lot over time. However, I found that several of what seem to be SMTM’s strongest arguments in favor of the contamination theory turned out to be dubious, and that nearly all of the interesting things I thought I’d learned from their blog posts turned out to actually be wrong. I’ll explain that in this post.
Summary of main points
- It’s not clear that either lab animals or wild animals have been getting more obese. [LW · GW]
- There were meaningful changes in obesity rates before the late 20th century [LW · GW]. People in the United States have been pretty much gradually getting heavier since the early 20th century.
- There is good evidence, which the SMTM authors have never addressed, that the lower obesity prevalence at high altitudes is caused by the lower atmospheric pressure at those altitudes [LW · GW] (rather than by the relative absence of environmental contaminants, which is their chosen explanation).
- Demographic factors and altitude might adequately explain the disparities in obesity rates across US states [LW · GW]. Contaminants don’t seem necessary to explain them.
- Being underweight is less common than it used to be, not more [LW · GW].
- CICO is compatible with body weight regulation and is consistent with the findings of overfeeding studies [LW · GW] (though CICO cannot by itself explain the obesity epidemic.)
It’s not clear that lab animals *or* wild animals have been getting fatter
When I was reading A Chemical Hunger, the argument I found most compelling was the following (from Part I: Mysteries (a)):
Humans aren’t the only ones who are growing more obese — lab animals and even wild animals are becoming more obese as well. Primates and rodents living in research colonies, feral rodents living in our cities, and domestic pets like dogs and cats are all steadily getting fatter and fatter. This can’t be attributed to changes in what they eat, because lab animals live in contained environments with highly controlled diets. They’re being fed the same foods as always, but for some reason, they’re getting fatter.
The interesting claims here are that lab animals on controlled diets and wild animals have allegedly gotten fatter. So I decided to investigate those claims.
The hyperlink in that quote leads you to a 2010 study by Klimentidis et al. which, as far as I can tell, is the only study to have investigated the issue. Googling “are lab animals getting fatter” yields a bunch of articles that all seem to cite that one paper as their source (except for a few results that don’t seem related to the question). I combed through the paper’s 179 Google Scholar citations and could not find a replication.
(By the way, contrary to what SMTM’s writing implies, this paper did not analyze the weight of wild animals, as we’ll discuss below).
Since Klimentidis et al. (2010)’s results have apparently not been replicated, I decided to attempt to replicate them myself.
Lab mice
I first thought this would be extremely hard or impossible to do, since there are way too many studies on mice. How was I supposed to find an unbiased, representative sample of mouse body weights over time?
Fortunately, however, some Google searches eventually led me to The Jackson Laboratory’s Mouse Phenome Database (a), which has a ton of phenotypic data of JAX® mice, which their website says (a) are the “the best characterized and most widely used mice available.” Importantly, the database includes body weight data from as far back as 2000, and you can filter the data for mice strain, so it seems like a good place to figure out whether genetically homogeneous lab mice have indeed grown fatter in tandem with humans.
Wikipedia says (a) that C57BL/6 mice are “[b]y far the most popular laboratory rodent.” So I thought that a good way of attempting to replicate Klimentidis et al.’s work would be to investigate whether C57BL/6J mice (that is (a), C57BL/6 mice from the Jackson Laboratory, the most common substrain of C57BL/6 mice[2]) have increased in body weight over time.
My analysis of the data is in this Google Colab notebook. I found positive correlations between year of measurement and average body weight of C57BL/6J mouse samples (r=0.17 for females and 0.12 for males), but this is before adjusting for age.
C57BL/6J mice grow roughly logarithmically with age; the correlation between log(age) and body weight is 0.74 for female mice and 0.70 for male mice in my sample. This is what their growth curves look like (a):
So I decided to control for log(age). After doing so, the correlations are -0.1 for both sexes. Multiple regression indicates that the average age-adjusted weight of female C57BL/6J mice has been decreasing by 0.06 grams per year, and that of male mice by 0.08 grams per year, which was not significantly different from 0.
The standard deviation of body weight has increased a bit for male mice (r = 0.09) but decreased for female mice (r = -0.18). Controlling for log(age), those correlations are 0.05 and -0.29, respectively. None of these were significant either. This trend towards a decrease in the SD of body weight among female mice, as well as the trend towards a decrease in average age-adjusted body weight among both sexes, is the opposite of what has happened among humans in the same timeframe.
My findings are in stark contrast with Klimentidis et al. (2010), which find that
Among mice in control groups in the National Toxicology Programme (NTP), there was a 11.8 per cent increase in body weight per decade from 1982 to 2003 in females coupled with a nearly twofold increase in the odds of obesity. In males there was a 10.5 per cent increase per decade.
Even more impressively, this paper looked at 24 different datasets of animals living among humans (most though not all of which seemed to have been on controlled diets) and found that age-adjusted body weights increased with time in all of them. The paper helpfully reminds us that the probability of all of those trends being in the same direction by chance is .
I... don’t know how to explain the difference. If their datasets are representative of the underlying distribution of animals living among humans, it should have been extremely unlikely for me to have found this dataset with an age-adjusted (non-significant) decrease in body weight.
Their paper did use a different statistical methodology from mine, but I honestly don’t fully understand it (it’s a lot more complicated than a simple correlation coefficient or regression model) so I wouldn’t be able to make an educated guess on how much that’s related.
Perhaps the C57BL/6J mice in my dataset have been fed progressively lower-calorie diets because researchers want them to maintain historically consistent body weights? But then why doesn’t that apply to any of the lab animal datasets in Klimentidis et al. (2010)?
Perhaps C57BL/6J mice are immune to whatever contaminant is causing obesity? But that would be weird, because Klimentidis et al. (2010) find secular increases in body weight in animals from a wide variety of different species.
Perhaps I cherry-picked my dataset? But recall that my data is from the most common substrain (C57BL/6J) of the most common strain (C57BL/6) of lab mice in the research world, and, as far as I can tell, I included all of the body weight data that the Mouse Phenome Database has on this substrain from mice undergoing no interventions. Also, I checked the next five most common strains in the dataset and replicated my findings.
So, uh, I don’t know.
I could have done something wrong in my analysis. I am not sure that my math is perfect. So it’s important to take that into account.
One consideration that complicates the interpretation of Klimentidis et al. (2010)’s findings is the fact that David B. Allison (who Nature refers to as the lead author of the study) has apparently been involved in controversies regarding his conflict of interest with the processed food and restaurant industries.[3]
Given that, and given that I could not replicate the findings with data from the Jackson Laboratory’s Mouse Phenome Database, I think it would be nice to have at least one independent replication of this study before confidently asserting (as the SMTM authors do) that lab mice have been getting fatter in tandem with humans.
Wild animals
The SMTM authors have claimed several times in their blog (a) that wild animals have been getting more obese:
From Part I: Mysteries (a):
From Part III: Environmental Contaminants (a):
Lab animals and wild animals are becoming more obese because they are exposed to the same environmental contaminants that we are.
From Part IX: Anorexia in Animals (a):
even wild animals have gotten more obese over the past several decades
From Interlude E: Bad Seeds (a):
Seed oils have a hard time accounting for patterns like obesity being related to altitude, and wild animals also becoming obese.
The only source they mention in their blog for that claim is the study above by Klimentidis et al. However, that study only examined animal populations living in close proximity to humans; the “wildest” animal population they include is Norway rats living in human towns (and thus likely eating human food), which is probably not what people have in mind (a) when they think of “wild animals.” None of the animals in the study were in the wilderness or had wild diets.
When Divia Caroline pointed that out to them on Twitter (a), they brought up studies (a) showing that populations of white-tailed deer experiencing increasing predation from humans have been getting heavier:
However, this is really not great evidence that “wild animals are becoming more obese.” Both of the studies they mention in those tweets — Wolverton et al. (2008) and Stout et al. (2013) — examined populations of white-tailed deer under increasing predation from humans. Predation decreases their population density, which increases the amount of energy available for each individual deer in their habitat. Both papers explicitly talk about the increase in average body weight as an expected, direct consequence of the increase in hunting. Further supporting this explanation is the fact that modern-day deer populations that aren’t hunted by humans have much lower average weights than those that are (p < 0.0001) according to Wolverton et al. (2007).
Additionally, these studies do not even attempt to measure the body fat of the deer, so it’s not as if they find that white-tailed deer have been accumulating more adipose tissue over time or anything like that. We don’t even know whether the deer equivalent of BMI has increased over time in those populations or not. In fact, given that Wolverton et al. (2008) report an increase in the mean size of the astragalus (a type of ankle bone (a)) in deer populations in which the average weight has increased, these deer may be simply getting taller and gaining a lot of lean mass.
So it seems to me inappropriate to bring up those studies as evidence that wild animals are getting more obese; doing so essentially amounts to taking advantage of the fact that people will often confuse increased body weight with increased adiposity. And it completely ignores the fact that the change in average weight in those populations can be explained by known factors unrelated to the obesity epidemic in humans.
There were meaningful changes in obesity rates before the late 20th century
From A Chemical Hunger – Part I: Mysteries:
Mystery 2: An Abrupt Shift
Another thing that many people are not aware of is just how abrupt this change was. Between 1890 and 1976, people got a little heavier. The average BMI went from about 23 to about 26. This corresponds with rates of obesity going from about 3% to about 10%. The rate of obesity in most developed countries was steady at around 10% until 1980, when it suddenly began to rise.
I think this understates the meaningfulness and extent of the changes in average BMI and obesity rates that occurred before 1980.
In the United States, the obesity rate among adults 20-74 years old was already 13.4% in 1960-1962 (a), 18-20 years before 1980. We don’t have nationally representative data for the obesity rate in the early 20th or late 19th centuries, but it might have been as low as ~1.5% or as high as 3%, indicating that the obesity rate in the US increased by a factor of >4x from ~1900 to ~1960.
John Komlos and Marek Brabec have investigated the trajectory of BMI deciles by birth cohort in the United States by using fancy statistical modeling on survey data collected between 1959 and 2006 by the National Center for Health Statistics (NCHS). According to their statistical model, BMI deciles have been increasing fairly smoothly across birth cohorts from 1886 to 1986 (note that the x-axes are each cohort’s birth year, not the year their BMI was measured):
(Those lines are the 10th, 20th, …, 90th BMI percentiles. The figures for white females and black males look similar.)
Note that we don’t have nationally representative BMI data before 1959 for the US; those lines are estimates based on cohort- and age-associated changes in BMI.
It does look like there has been an acceleration at the later birth cohorts for the few highest BMI percentiles, but a minor acceleration is arguably not the same thing as “an abrupt shift.” And in fact, the paper points out that for some groups, the rate of change in BMI never actually surpassed its pre-WWII peak.
Another way to demonstrate this: the median weight of a 5’10” 18-year-old white male student at the Citadel Military Academy in Charleston increased by 7 kg (or 15.4 lb) from 1888-1927 to 1938-1957. This change was greater than the change in median weight of US males of the same age/height/race from the 1980s to the 2000s:
(Again, the x-axis here is each cohort’s birth year. These are all 18-year-olds, so you can add 18 to get the measurement year range of each bucket.)
Further evidence of great changes in BMI before 1980 can be found in this Twitter thread (a), which shows, among other things, that men at elite colleges (a) (source) and veterans all started getting substantially fatter in the early 20th century.
As you can see, there was a significant slowdown in the increase of BMIs around the middle of the twentieth century. Because of this, looking only at post-war BMI data can give the impression that the rate of change in BMI in the late twentieth century was more unusual than it actually was.
Being underweight is less common than it used to be, not more
The SMTM authors have made (a) the following claim:
In humans, the obesity epidemic has been matched by an increase in the variance of BMI, which means more people who are overweight AND more people who are underweight
They say that this is evidence of the contamination theory, claiming (a) that “[o]ne obvious prediction of the theory that obesity is caused by a contaminant in our environment is that we should also expect to see paradoxical reactions to that contaminant" i.e. in this case, that contaminants that cause most people to gain weight should also cause some people to lose weight.
However, as you can easily figure out by Googling, the prevalence of underweight has been decreasing over time, not increasing, as they claimed. This article in The Lancet (which the SMTM authors have cited once but don’t seem to have read[4]) says that:
[From 1975 to 2014], age-standardised global prevalence of underweight decreased from 13·8% (10·5–17·4) to 8·8% (7·4–10·3) in men and from 14·6% (11·6–17·9) to 9·7% (8·3–11·1) in women.
Figure 6 from the article shows the trends in age-standardized prevalence of BMI categories by region, in women (Figure 5, which shows the same thing for men, looks similar):
Underweight people are too rare in high-income countries for any trend to be easily discernable from that figure, so let us zoom in on US data. In the US, the prevalence of underweight among adults aged 20-74 has decreased almost monotonically (a) over the past several decades:
Likewise, data from the United Kingdom show a consistent decrease (a) in the prevalence of underweight among adults from 2000 to 2016:
It is true that, as the SMTM authors point out, the variance in BMI has increased, but that is entirely due to higher BMIs being more common (at least in the countries for which I have data, which are the UK and the US). Here is how the distribution of BMI has changed from the first NHANES (in the early 70s (orange)) to the last one (from 2017 to 2020, in blue)[5]:
The code used to make this plot is here.
And here are BMI distributions from the UK in 1993 and 2018, from the UK Health Security Agency (a):
The altitude effect
When you look at county-level or state-level US obesity data, it’s very clear (a) that altitude is (negatively) associated with obesity. Notably, the association remains after controlling for several relevant variables, as shown in Voss et al. (2013). And we have evidence that the effect is causal: Voss et al. (2014) found that members of the US Army or Air Force were less likely to become obese if they were assigned to live in a high-altitude county. Moreover, it is well-established in the literature that ascending to high altitudes causes weight loss in the short term.
So why is this a thing?
A good starting point is to see what the 2016 Slate Star Codex post about the phenomenon (a) has to say about its cause:
The active ingredient of altitude seems to be hypoxia. The air is thin at high altitudes so the body gets less oxygen. Being in low oxygen conditions in normal pressure seems to cause weight loss too – see here and here for studies of people exercising in low oxygen conditions. I don’t know of any studies where people were just kept in low-oxygen environments for a long time without exercise to see what happened to their weight. It’s not really clear how reduced oxygen makes people eat less.
The SMTM authors disagree, claiming that the effect of altitude on obesity cannot be explained by anything about the atmosphere. What is their argument, you ask? Well, this is it (a):
We know that oxygen and carbon dioxide vary with elevation, so you might expect that this is attributable to these differences. But the evidence is pretty thin. Combined with a low-calorie diet, exercise in a low-oxygen environment does seem to reduce weight more than exercise in normal atmospheric conditions, but not by much. Submarines have CO2 levels about 10 times higher than usual, but a US Navy study didn’t find evidence of consistent weight gain. The atmosphere itself can’t explain this.
I don’t think this does a good job at all of addressing the evidence for the role of the atmosphere. The participants in the low-oxygen study they cited spent only 3.6% of their time (6 hours per week) in hypoxia, for only four weeks. That is clearly different from spending all of your time for decades in a hypoxic environment, as people who live in high altitudes do. Also, it’s unclear why they imply that the effect wasn’t large: the study they cited said that the hypoxia group lost 2.6 kg (5.7 lb) more than the control group over 4 weeks.
Moreover, that study only changed the oxygen content of the air subjects were breathing. It exposed them to what is called “normobaric” hypoxia in the research literature. “Normobaric” means that the ambient air pressure was unchanged. People who live in high altitudes are subject to hypobaric hypoxia instead, which seems to cause more severe effects than normobaric hypoxia for the same oxygen levels.
So I don’t think they’ve presented enough evidence in that paragraph to completely rule out the idea that the atmosphere is responsible for the altitude effect.[6]
Neither the 2016 SSC blog post nor the SMTM authors ever mention the studies that seem most relevant for figuring out whether hypobaric hypoxia is responsible for the altitude effect: the ones that confine people to hypobaric chambers continuously for weeks.
Hypobaric confinement seems to cause a lot of weight loss: the three Operations Everest and other studies
When researching experimental hypobaric confinement for this blog post, I came across some of the most dangerous studies on healthy humans I’ve ever heard of: the three Operations Everest.
As the title of this section suggests, those three studies involved putting people inside hypobaric chambers, and, as the study names suggest, those chambers eventually reached an air pressure close to that of the summit of Mount Everest. So let’s take a look at those studies.
Operation Everest I (conducted in 1946 by the US Navy):
4 healthy young men were selected [...]. They lived in a decompression chamber [...] for 32 days during which the chamber was gradually decompressed to a simulated altitude of 22,000 ft [6,705.6 m] (350 torr) where they remained for 4 days.
[...]
Appetites declined and all subjects lost weight (average 6 pounds in the last 2 weeks).
Unfortunately, I could find no further information about body weight changes.
Operation Everest II (conducted in 1985, also by the US Navy):
[S]ix men, provided with a palatable ad libitum diet, were studied during progressive decompression to 240 Torr [equivalent to 8,848 m] over 40 days in a hypobaric chamber where hypoxia was the major environmental variable. Caloric intake decreased 43.0% from 3,136 to 1,789 kcal/day (P < 0.001). [...] Over the 40 days of the study the subjects lost 7.4 ± 2.2 (SD) kg [16.28 ± 4.8 lb] and 1.6% (2.5 kg) of the total body weight as fat. Computerized tomographic scans indicated that most of the weight loss was derived from fat-free weight.
This corresponded to 8.9 ± 2.0% (mean ± SD) of participants’ starting body weight.
Operation Everest III:
Energy and water balance was studied over 31 days in a hypobaric chamber at 452–253 Torr (corresponding to 4,500–8,848 m altitude), after 7 days acclimatization at 4,350 m. Subjects were eight men, age 27±4 years (mean±SD), body mass index 22.9±1.5 kg/.
[...]
Body mass changed throughout the experiment. The changes from normoxia to day 2, over days 2–17 and over days 17–31 were –1.4±0.8 (P<0.01), –2.5±1.1 (P<0.001) and –1.2±0.6 kg (P<0.01), respectively
I calculated that, as a proportion of starting body weight, the weight loss was 6.9 ± 2.1% in this study.
There have been shorter and less extreme hypobaric hypoxia studies.
Greenleaf et al. (1987) found that participants lost on average about 1% of their body weight after 8 days at a simulated altitude of 2,287 m. Interestingly, this is around the same average % weight loss as that found in Lippl et al. (2010), a real-altitude study that took people to an altitude with a similar barometric pressure (2,650 m) for a similar period of time (7 days).
Gatterer et al. (2021) confined 12 young women to a hypobaric chamber twice, once with the chamber “turned on” at a pressure of 493 mmHg (equivalent to 3,500 m) and once with it “turned off.” The women, who at the start of the experiment weighed an average of 60.6 kg (133.3 lb), lost on average 0.71 kg (1.56 lb) in four days during the hypobaric hypoxia period, while their weight remained stable during the control period.
Buchzik et al. (2014) is a double-blind, placebo-controlled, reverse simulated altitude study. It took all subjects to a high altitude (2,760 m) for seven days, but gave supplemental oxygen to the control group. I can’t find the full text, but the abstract says that the “intervention group weighed significantly less than the control group who gained weight at D14” i.e. after the high-altitude stay.
Those were all of the studies of hypobaric hypoxia confinement in humans I’ve found that reported changes in body weight.
Normobaric hypoxic confinement, too, seems to cause weight loss, in both humans and animals
Humans
Studies of normobaric hypoxic confinement in humans likewise find a trend towards weight loss, though none of those studies have been as extreme as the Operations Everest, as far as I can tell.
Mekjavic et al. (2021) confined 8 obese men to both a hypoxic chamber and a normoxic (normal-oxygen) chamber in a crossover manner. During hypoxic confinement, they lost on average 0.7 ± 0.2 kg, and during normoxic confinement, they gained 1.0 ± 0.2 kg.
Mekjavic et al. (2016) found 0.2 kg greater weight loss in hypoxic confinement than in normoxic confinement after 10 days among a group of 11 thin men. Mean daily energy intake was substantially lower in hypoxia (2472 ± 251 kcal) than in normoxia (2847 ± 241 kcal).
Debevec et al. (2014a) measured body weight changes in 11 healthy men after three 21-day interventions: bed rest, hypoxia and bed rest with hypoxia. The men lost 1% and 2% more of their body weight on the hypoxic and hypoxic bed rest interventions, respectively, than on the normoxic bed rest intervention.
Debevec et al. (2014b) confined 14 healthy male participants to a normobaric hypoxic chamber for 10 days, and randomized them to either exercise or not. Both groups lost weight, by an average of 1.4 kg.
Those were all of the studies of normobaric hypoxia confinement in humans I’ve found that reported changes in body weight.
Animals
J. Argyll Campbell was a late 19th-century and early 20th-century scientist who was academically interested in the physiological effects of hypoxia. He conducted dozens of studies in which he confined animals to low-oxygen environments for several weeks or months. As far as I could tell, in all of them[7], the poor animals rapidly lost a lot of weight, and many of those were young and supposed to be growing. Here is a sample chart from one of his papers, Campbell (1934) (a), showing that young mice in hypoxic environments completely fail to grow up (the arrow labeled “21” denotes when the mice in the experimental condition returned to an environment with the normal partial pressure of oxygen at sea-level, which is ~21% of a standard atmosphere):
Food is apparently less appetizing in hypobaric conditions
Apparently, airlines have found that odor and taste perceptions get worse inside airplanes at cruising altitude (whose cabin pressures correspond to an altitude of ~6,000 ft to ~8,000 [~2,000 m to ~2,500 m] according to a quick Google search). See this Business Insider article and this BBC article. The articles mention this study, which found that odor and taste perception get worse inside simulated aircraft cabins, i.e. hypobaric chambers with a bit more noise and air dryness.
Relatedly, Matu et al. (2018), a systematic review and meta-analysis on the effect of hypoxia on hunger perceptions, found that actual high altitude in general doesn’t seem to affect hunger-related variables more than simulated altitude does (see their subgroup analysis on Table 1), though there were a small number of studies involved. In fact, studies that simulate high altitude with hypobaria (as opposed to normobaric hypoxia) seem to show greater effects on hunger perception than studies that actually take people to high altitudes.
The dose-response relationship between altitude and body weight seems incompatible with the contamination hypothesis
Most industrial water contamination in the US probably happens at relatively low altitudes, since that’s where most people live. A very small fraction of the US population lives more than 1,000 m (3,280.84 ft) above sea level:
The x-axis is in meters.
So, if the altitude effect were entirely caused by contaminants, we would expect moving from 0 m to 1,000 m to have a much greater effect on body weight than moving from 2,000 m (6,561.68 ft) to 3,000 m (9,842.52 ft) in the US.
But the information we have about this topic seems to suggest that the opposite is true. Voss et al. (2013) found that the altitude effect accelerates with increasing altitude. The prevalence of obesity is barely different at 1,000 m than at 0 m across United States counties, and then there is a sharp drop-off from 2,000 m upwards:
Likewise, Voss et al. (2014) found that US military members assigned to high-altitude locations (>1,960 m) had a 41% (95% CI 35%-46%) lower chance of becoming obese than those assigned to low-altitude locations (<980 m), but that those assigned to medium-altitude locations (980 m-1,960 m) only had a 5% (95% CI 0%-10%) lower chance of becoming obese.
Moreover, by the same logic, the contamination theory would predict that, at altitude ranges with very little or no industrial contamination, altitude should stop affecting body weight altogether. If you are on a mountaineering expedition, why should being at, say, 6,000 m rather than 4,000 m make any difference to your body weight? There is barely any industrial activity above 4,000 m, as far as I am aware, and exactly zero of it in most mountains people climb. And yet, as far as I can tell, exposure to extreme altitudes seems to cause more weight loss than exposure to very high but lower altitudes.
Demographic factors and altitude could adequately explain the geospatial distribution of obesity in the US
The distribution of obesity across US states looks something like this:
(I’m using state-level instead of county-level data because county-level obesity datasets don’t correlate well with each other. [LW(p) · GW(p)])
Here’s a choropleth I created myself, using the same data as the one above but on a continuous color scale:
As I’ve mentioned before, you can see that altitude seems to be negatively associated with obesity.
But it does not seem to be the only obvious factor at play — after all, states like West Virginia (high-ish average altitude) are a lot more obese than states like Massachusetts (low average altitude). I did a simple linear regression and found that population-weighted average altitude explains about 7% of the variation in obesity rates across US states, leaving a lot unexplained.
The SMTM authors claim (a) that that is because of environmental contaminants:
It’s important to note that altitude itself doesn’t affect obesity directly. Instead, altitude is a proxy for how high an area is in the watershed, which is itself a proxy for how badly the local water supply is contaminated. This is why Mississippi is more obese than low-lying areas of California. In California the water supply hasn’t traveled nearly as far in its path to the ocean, and has traveled past fewer farms, highways, cities, and factories.
But there are very important differences between high- and low-obesity states that I think must be taken into account.
Foreign-born population
US states vary widely in the proportion of their population that is foreign-born. The differences are really not subtle. In California, 1 in 3.7 people was born outside of the United States, compared to 1 in 67 in West Virginia (a).
You can see that the highest-obesity states tend to have fewer foreign-born people as a proportion of their population:
A multiple linear regression model with population-weighted average altitude and proportion of the population that is foreign-born as independent variables explains 45.5% of the variation in obesity rates across US states, so this is a way better model than altitude alone.
This makes sense because most countries have a lower obesity rate than the US (a). And although immigrants to the US do gain weight with time, they remain leaner than the native population for more than a decade after arrival.
The SMTM authors haven’t addressed the possibility that immigration could play a role in explaining the obesity rate differences between US states. They’ve written a post (a) claiming that those differences cannot be explained by demographic factors, but don’t mention immigration anywhere in it.
Race and ethnicity
There are racial and ethnic disparities in the prevalence of obesity in the United States (see this page on the CDC website (a) for more information). Accordingly, a linear regression model with “% (non-Hispanic) Asian,” “% (non-Hispanic) Black” and “% Hispanic” variables (chosen because those are the three largest racial/ethnic minority groups) on top of the variables we’ve discussed above (population-weighted average altitude and proportion of the population that is foreign-born) explains 74% of the variation in state obesity rates.
Education
It turns out that education is strongly negatively associated with obesity across US states:
I used this obesity dataset from the CDC and this educational attainment dataset from the USDA to create this chart.
Here’s the data in choropleth form:
A Chemical Hunger talks a lot about how Colorado (the #1 leanest state) is a lot less obese than any other US state, and how that is probably explained by its high average altitude and thus low exposure to environmental contaminants in water. But one thing the SMTM authors never mention about the state is that it is suspiciously #2 in the nation in its proportion of adults with college degrees, behind only Massachusetts (which also happens to be the #2 leanest state).
At the individual level, the obesity rate of adults without a college degree in the US is around 40%, compared to about 28% for those with a degree – though earlier educational milestones (e.g. completing high school, or attending college but not graduating) don’t have a consistent association with obesity.
Adding education to our linear regression model takes the from 0.74 to 0.82.
Independent variables | adjusted
| Leave-one-out negative mean absolute error | |
Population-weighted average altitude | 0.07 | 0.05 | -3.3 |
Population-weighted average altitude + % foreign-born | 0.455 | 0.407 | -2.9 |
Population-weighted average altitude + % foreign-born + % Asian + % Black + % Hispanic | 0.74 | 0.71 | -2.1 |
Population-weighted average altitude + % foreign-born + % Asian + % Black + % Hispanic + % with college degree | 0.82 | 0.79 | -1.9 |
(Code here.)
Anyway. It is definitely plausible that environmental contaminants are responsible for the geographical disparities in obesity rates in the US. But I don’t see any indication that environmental contaminants are necessary to explain them, as the SMTM authors claim they are.
High-obesity states do poorly on a lot of different health metrics, not just obesity
Consider the following scatterplots:
(States are colored according to the Democratic vote margin in the 2020 general presidential election. My code is in this Colab notebook.)
Racial and ethnic minorities face unique challenges in accessing healthcare, so we should control for race and ethnicity. Doing so, this is what we get:
Variable | Partial correlation with obesity rate, controlling for race and ethnicity |
---|---|
COVID-19 vaccination rate | -0.59, 95% CI [-0.75, -0.36], p = 0.000013 |
Opioid dispensing rate | 0.52, 95% CI [0.27, 0.7], p = 0.000177 |
Democratic vote margin in 2020 | -0.62, 95% CI [-0.77, -0.41], p = 0.000003 |
Permanent tooth removal rate | 0.40, 95% CI [0.13, 0.62], p = 0.004976 |
Smoking rate | 0.60, 95% CI [0.38, 0.76], p = 0.000007 |
Percentage of adults with a Bachelor’s degree or higher | -0.69, 95% CI [-0.82, -0.5], p = 8.220840e-08 |
It just seems that there’s a group of states that do poorly on a lot of health metrics. They have higher rates of smoking, permanent tooth removal, and opioid dispensing, along with lower rates of COVID-19 vaccination, and they have higher obesity rates. Also, this seems really quite strongly related to partisanship for some reason.
This seems to be a classic case of a lot of variables being correlated with each other, with the underlying cause being difficult to disentangle. I don’t see any indication that environmental contaminants — rather than more pedestrian things like differences in cultural or socioeconomic factors — must be the underlying cause.
Contaminants are not needed to explain the obesogenic effects of palatable human food
One intuitive explanation of the obesity epidemic is that it is caused by exposure to ultra-processed/hyper-palatable/“cafeteria diet”-like human food. And, perhaps unexpectedly to a lot of people, the SMTM authors acknowledge (a) the obesogenic properties of that type of food:
Mystery 6: Palatable Human Food
Lab rats gain some weight on high-fat diets, but they gain much more weight on a “cafeteria diet” of human foods like Froot Loops [sic] and salami (see also here).
[...]
Rodents eating diets that are only high in fat or only high in carbohydrates don’t gain nearly as much weight as rodents eating the cafeteria diet. And this isn’t limited to lab rats. Raccoons and monkeys quickly grow fat on human food as well.
We see a similar pattern of results in humans. With access to lots of calorie-dense, tasty foods, people reliably overeat and rapidly gain weight. But again, it’s not just the contents. For some reason, eating more fat or sugar by itself isn’t as fattening as the cafeteria diet. Why is “palatable human food” so much worse for your waistline than its fat and sugar alone would suggest?
The SMTM authors think this happens because processed food has more contaminants:
Lab rats gain more weight from human foods than they do from rat chow with similar nutritional properties because obesity doesn’t come from fat or carbohydrate content, but from contaminants in the food, and human food has more contaminants than the rat chow does, likely from packaging and processing.
But there is an alternative hypothesis in the academic world, explained in Stephan Guyenet’s The Hungry Brain, and summarized in Scott Alexander’s review of the book. The TL;DR is that the rewarding qualities of modern human food damage your brain’s weight-regulation mechanism. It makes sense to be skeptical of this explanation if you believe that lab or wild animals are getting fatter, or that contaminants are needed to explain geospatial discrepancies in obesity rates. But, as I explained in earlier sections of this post, neither of those two things is clear, which makes this explanation more plausible than it would otherwise be.
On Some Conceptions Of CICO
Disclaimer
CICO is an extremely touchy subject, and in this section I will criticize SMTM’s criticisms of CICO. So, before I do that, I’d like to make a few things clear:
- Most popular conceptions of CICO do not describe a law of physics. For example, if you interpret CICO as meaning that weight changes are purely a function of calories consumed minus calories burned, that is wrong because it doesn’t take into account that your body can get rid of calories without necessarily burning them. People with untreated diabetes mellitus pee out a lot of sugar, and the calories in the sugar they pee out are obviously neither burned nor stored as energy in their bodies. For more examples of things like this, see the comments in this Facebook post by Spencer Greenberg. See also Eliezer Yudkowsky on this topic.
- Obesity is not caused by a lack of willpower. Your brain has a lipostat that employs an array of different mechanisms for keeping body fat at a certain level. It is so sophisticated that even exercising more often just results in your body burning less energy in maintenance and repair (a) to compensate (yes, seriously). Some particularly unsophisticated conceptions of CICO that are not taken seriously in the research world do not take this into account, and they are wrong.
- Even smart conceptions of CICO that take the points above into account have limited usefulness in explaining the obesity epidemic. They leave open the question of why people are consuming more calories (or burning/wasting fewer calories) than they were several decades ago.
With that out of the way, I'll explain why I think SMTM's arguments against CICO were weak.
Overeating for weeks != overeating for decades
The SMTM authors say that overfeeding studies are evidence against CICO because people don’t gain that much weight in them (a):
Overfeeding in controlled environments does make people gain weight. But they don’t gain enough weight to explain the obesity epidemic. If you eat 10,000 calories per day, you might be able to gain 20 or 30 pounds, but most Americans aren’t eating 10,000 calories per day.
We can compare these numbers to the increases in average calories per day we reviewed earlier. Sure, consumption in the US went from 2,025 calories per day in 1970 to 2,481 calories per day in 2010, a difference of 456 calories. But consider Poehlman et al. (1986), where researchers fed a group of 12 men 1,000 extra calories a day for 22 days. On average the men gained about 5 lbs (2.2 kg), but some of them actually lost weight instead.
I think this is flawed. Clearly, overeating for your entire life will probably have different effects from overeating for 22 days. There are a lot of 22-day periods in a person’s life. Someone on their 30th birthday has gone through nearly 500 of them.
If you try to make an educated guess on whether 456 extra kcal/day is enough to explain the change in average body weight, instead of assuming that 22 days of overeating will have the same effects as 3 decades of overeating and considering the question closed, you not only find that the 456 kcal number is enough, but you find that it is way too high — which just renders SMTM’s point all the more bizarre. Let me explain.
How much body weight can you expect to gain if you start eating 456 extra kcal per day? Some people bring up the “3,500 kcal = 1 lb” rule of thumb and predict you’d gain 47.6 lb per year, or... a comical 1,428 lb by age 30. But that is not a good model, because eventually your energy expenditure will catch up with your increased energy intake. So what would a better model say?
Fortunately, Kevin Hall has a paper explaining a much better model, and a web-based tool implementing it. The rule of thumb the paper provides is that having 10 extra kcal/day leads to a 1 lb (0.45 kg) change in body weight in the long-term, with 95% of the change occurring in about 3 years. This means that a 456 kcal/increase in energy intake will eventually lead to a 45.6 lb increase in body weight.
In comparison, according to my analysis of NHANES data, the average American adult gained 12 kg (~26.4 lb) since the early 70s, way less than the 456 kcal number predicts. So yes, a 456 kcal increase in average daily energy intake would absolutely be a jaw-dropping increase.
Of note, this 456 kcal number is based on self-report data, so it’s not likely to be that accurate. Stephan Guyenet mentions a better estimate on The Hungry Brain based on food disappearance data from the USDA, which is only 218 kcal/day, much more consistent with Kevin Hall’s model.
Equivocating between different meanings of the word “overeat” is not a good argument
From A Chemical Hunger – Interlude A: CICO Killer, Qu’est-Ce Que C’est? (a):
One way to interpret CICO (or one sub-hypothesis) is that it claims there is a strictly linear relationship between calories eaten/burned and weight change. This is specified if we take “weight gain = calories in – calories out” literally. Essentially, this hypothesis says that overeating by the same amount should always lead to the same amount of weight gain.
This is clearly false. The overfeeding studies provide extremely strong evidence against this version of CICO, since people gain very different amounts when overfed by the same amount, the difference appears to be mostly genetic, and some people actually lose weight, even when overfed by moderate (1000 kcal/day) amounts. Many people still believe something like “for every extra 3500 calories you eat you always gain one pound”, but all available evidence comes down very strongly against that.
Notice the equivocation here. Does “overeating by X calories” mean “eating X calories more than you usually do” or “eating X calories more than you’re actually burning throughout the study”? Overfeeding studies do not provide “extremely strong evidence” against the version of CICO the SMTM authors described unless they make people overeat by the same amount in the second sense of the word “overeat.”
And, from my review of overfeeding studies, that does not seem to be at all what they’re doing. They usually just give everybody a fixed absolute (e.g. 1000 kcal) or relative (e.g. 20%) energy surplus, with the “relative” surplus being calculated from your normal caloric consumption. They don’t constantly readjust the number of calories you’re eating each day based on how many calories you burned the previous day.
Accordingly, as explained in a The Hungry Brain quote cited in a 2017 SlateStarCodex post (a), the primary reason there is variation in weight gain in controlled feeding studies seems to be that some people ramp up their energy expenditure a lot more than others, which is perfectly consistent with CICO:
The research of James Levine, an endocrinologist who works with the Mayo Clinic and Arizona State University, explains this puzzling phenomenon. In a carefully controlled overfeeding study, his team showed that the primary reason some people readily burn off excess calories is that they ramp up a form of calorie-burning called “non-exercise activity thermogenesis” (NEAT). NEAT is basically a fancy term for fidgeting. When certain people overeat, their brains boost calorie expenditure by making them fidget, change posture frequently, and make other small movements throughout the day. It’s an involuntary process, and Levine’s data show that it can incinerate nearly 700 calories per day.
CICO is compatible with body weight regulation
From a section in Current Theories of Obesity are Inadequate (a) addressing CICO:
A popular theory of obesity is that it’s simply a question of calories in versus calories out (CICO). You eat a certain number of calories every day, and you expend some number of calories based on your metabolic needs and physical activity. If you eat more calories than you expend, you store the excess as fat and gain weight, and if you expend more than you eat, you burn fat and lose weight.
This perspective assumes that the body stores every extra calorie you eat as body fat, and that it doesn’t have any tools for using more or less energy as the need arises. But this isn’t the case. Your body has the ability to regulate things like its temperature, and it has similar tools to regulate body fatness. When we look closely, it turns out that “calories in, calories out” doesn’t match the actual facts of consumption and weight gain.
But CICO does not imply that your brain can’t regulate your body weight. It just says that if your brain wants to keep you thin, but you overeat, your brain will have to make you burn (or waste) extra calories. And, as we’ve seen before, that is indeed how some people manage to stay thin in overeating studies.
In fact, Stephan Guyenet — who the authors are well acquainted with — wrote an entire book about how the brain regulates fat adiposity, which, as expected, by no means contradicts CICO. So it’s puzzling to me that SMTM would put forward that kind of argument. I think they should have made it clear that they were arguing against particularly weak conceptions of CICO.
Under the CICO model, a lot of things are allowed to influence body weight
As I explained in the section above, CICO is merely a theory about what kinds of things go along with weight gain and loss. It doesn’t say anything about what is ultimately causing weight change. So it is perfectly compatible with things like altitude affecting body weight.
As an analogy, consider the theory that the mechanism underlying multiple sclerosis (MS) is the attack and destruction of myelin sheaths by the immune system. Is this theory incompatible with the fact that MS prevalence seems strongly related to latitude, much like obesity is strongly related to altitude? Or with the fact that MS is a lot more common in women than men, and for some reason almost always starts in youth or middle age? Or with the fact that MS seems to essentially be long mono? Or with the fact that MS is slightly heritable? Of course not. If you don’t have a reason to believe that immune system dysfunction shouldn’t be affected by age, gender, latitude, past EBV infection, or genetics, those things are zero evidence against the theory.
On the other hand, if you actually had a lot of trustworthy studies showing that the immune system is no more likely to destruct myelin sheaths among people far from the equator – who are more likely to get MS – than among people close to it, then that theory would be in some trouble.
Notably, this means that CICO is perfectly compatible with SMTM’s contamination theory of obesity, to the extent that we don’t believe that it’s impossible for environmental exposure to chemicals to affect caloric intake or expenditure. I think it would have made more sense for SMTM to try to take down the hyperpalatability theory of obesity instead of CICO, but they notably don’t talk much about the theory in their series.[8] They even agree that eating palatable, processed human food causes overeating and weight gain, as I mentioned earlier [LW · GW].
Conclusion
As I said at the beginning of this post, the contamination theory of the obesity epidemic is plausible a priori. But that remains the strongest argument in favor of it that I’ve found, in my opinion. As far as I can tell, we don’t have evidence that wild animals have become more obese over time, or that people lose weight by drinking distilled water. People in rural areas in the US are more likely to be obese than those living in cities, not less, even though it seems plausible that they’re exposed to less industrial contamination. And we don’t need contaminants to explain the effect of altitude on body weight, or other geospatial patterns in the prevalence of obesity.
In light of all of this, I’m comfortable being the largest NO shareholder in this play-money prediction market:
However, I think it would be interesting to see more discussion on the contamination theory of the obesity epidemic, and I’m open to changing my mind. So let me know in the comments if you find compelling evidence in favor of it that I’ve missed.
Acknowledgments
Thanks to José Luis Ricón Fernández de la Puente, ChatGPT, and my husband Matthew Barnett for helpful comments and suggestions in earlier drafts of this post.
I do not speak for anyone other than myself, and all errors are my own.
Addenda
SMTM’s posts still have factual inaccuracies and misrepresentations of sources
In my last blog post, published over six months ago, I listed several factual inaccuracies and misrepresentations of sources in SMTM’s blog posts about lithium [LW · GW]. I and others have tried to contact the SMTM authors about those issues several times, both before and after that post was published, but they have never corrected any of them, and Manifold Markets doesn’t think they’ll correct them by June 2023.
I think that making mistakes every once in a while is obviously fine, but that people should correct incorrect information they’ve published if they’re shown it’s incorrect. It is concerning to me that the SMTM authors have chosen not to do so.
(Non-exhaustive) errata/changelog
The first version of this blog post argued that, contra the SMTM authors, there wasn't an abrupt shift in obesity rates in the late 20th century. Further discussion in the comments [LW(p) · GW(p)] made me realize that the argument I was trying to make was too semantic in nature and exaggerated the differences in our perspectives. I changed this about 8 hours after the post was published.
The first versions of this post described John Komlos and Marek Brabec as "economists." I changed this after realizing that Marek Brabec is a statistician and John Komlos is an economic historian. Sorry!
- ^
The SMTM authors have not replied to or acknowledged the existence of that post. The closest they’ve gotten to doing so was a post they wrote addressing 5 of the ~17 studies I mentioned that found low concentrations of lithium in food and claiming that they are outliers in the literature, a claim that they supported by misrepresenting their sources, as I explain here [LW · GW].
That post they wrote seems to indicate [LW(p) · GW(p)] that they had not yet actually read my post, as they say that those 5 studies "disagree with basically every other measurement [they]’ve ever seen for lithium in food" (and repeat this point quite a few times), so it seems more likely that they were attempting to reply to an earlier Twitter thread of mine that mentioned only those 5 studies rather than to my post itself. Their post also doesn't acknowledge or mention any of the other points I made in my post.
- ^
I am aware of one other substrain of C57BL/6 mice: C57BL/6N, bred (a) in the National Institutes of Health. This seems to be substantially less commonly used in research than C57BL/6J — Google Scholar returns 395,000 results for “C57BL/6J” and only 32,000 for “C57BL/6N.” Likewise, this slide deck (a) says that “More than 90% of the world’s published references to C57BL/6 sub-strains refer to the C57BL/6J substrain, which originates from The Jackson Laboratory.”
- ^
See this NYT article (a) and this ABC News article (a).
- ^
This (a) is the post in which they cited the article. Quoting from it:
Everyone knows that the average BMI has been going up for decades, but what is less commonly known is that the variance of BMI has also increased since 1975. When expressed in standard deviation, it has almost doubled in many countries. As correctly noted in The Lancet, this “contributed to an increase in the prevalence of people at either or both extremes of BMI.”
I don’t know exactly what the The Lancet article meant by that quote, but they definitely did not mean that the prevalence of underweight has increased over time, since they actually show that it has decreased.
- ^
The “data” in this figure is generated with weighted kernel density estimation (using the default parameters of sklearn.neighbors.KernelDensity). This is necessary because NHANES oversamples some groups, so every time you want to do any kind of analysis with NHANES data you have to weigh each sample by its sampling weight. However, in case you were wondering, the underlying raw data looks similar:
- ^
The SMTM authors did publish a lengthy critique (a) of a particular study on the effects of altitude on body weight (Lippl et al. (2010)), and their post was somehow advertised on Astral Codex Ten (a) as “a critique of the research on hypobaric hypoxia causing weight loss,” (and the SMTM authors painted as such). But that is an extremely misleading description — the SMTM authors criticize a single study, not “the research on hypobaric hypoxia causing weight loss,” and they don’t even mention or address any of the studies on the effect of simulated high altitude in hypobaric chambers at all (neither in that post nor anywhere else in their blog or social media). The study they criticized just… took people to a high altitude for seven days and observed that they lost weight. It seems that SMTM’s criticism is that it had a weird statistical methodology?
- ^
To make sure that I wasn’t missing any relevant studies from him, I searched on PubMed for “Campbell JA[Author] and (oxygen[Title/Abstract] or altitude[Title/Abstract])”, and looked through the citations of each relevant article to find any that might be missing from that search.
These ended up being all the relevant articles from him that I have found and read:
Campbell J. A. (1927). Further observations on oxygen acclimatisation. The Journal of physiology, 63(4), 325–342. https://doi.org/10.1113/jphysiol.1927.sp002407
Campbell J. A. (1930). Living at very high altitudes and maintenance of normal health. The Lancet, Volume 215, Issue 5555, 370-373.
https://doi.org/10.1016/S0140-6736(00)88121-0
Campbell J. A. (1935). Further Evidence that Mammals Cannot Acclimatize to 10 p.c. Oxygen or 20,000 feet Altitude. British Journal of Experimental Pathology, 16(1), 39–48. https://www.ncbi.nlm.nih.gov/pmc/articles/PMC2065096/ (a) - ^
The closest they get to doing so is when they criticize a small set of studies that are used as evidence for the hyperpalatability hypothesis.
68 comments
Comments sorted by top scores.
comment by DirectedEvolution (AllAmericanBreakfast) · 2023-01-29T00:49:52.020Z · LW(p) · GW(p)
This comment is a local [LW · GW] counterargument to this specific paragraph (emphases mine):
Mystery 2: An Abrupt Shift
Another thing that many people are not aware of is just how abrupt this change was. Between 1890 and 1976, people got a little heavier. The average BMI went from about 23 to about 26. This corresponds with rates of obesity going from about 3% to about 10%. The rate of obesity in most developed countries was steady at around 10% until 1980, when it suddenly began to rise.
This paragraph is very misleading. In the United States, the obesity rate among adults 20-74 years old was already 13.4% in 1960-1962 (a), 18-20 years before 1980. Moreover, the SMTM authors cite no source for the claim that “the rate of obesity in most developed countries was steady at around 10% until 1980,” and in the United States at least that claim seems to be very wrong – we don’t have nationally representative data for the obesity rate in the early 20th or late 19th centuries, but it might have been as low as ~1.5% or as high as 3%, indicating that the obesity rate in the US increased by a factor of >4x from ~1900 to ~1960.
Here is my argument tree for your debate here:
- SMTM: There was a 3x increase in obesity from 1890-1976
- Mendonça: The obesity rate increased by a factor of 4x from 1900-1960
- SMTM: The rate of obesity in most developed countries was steady at around 10% until 1980
- Mendonça: The obesity rate was 13.4% by 1960-1962
- SMTM: In 1980, the rate of obesity suddenly began to rise
- Mendonça: Links to graph that does show an abrupt acceleration in obesity and severe obesity starting in 1971-1974 in a section labeled "there wasn't an abrupt shift in obesity rates in the late 20th century"
It seems to me that your rebuttals to this paragraph exaggerate the differences in your perspectives. SMTM points out a 3x increase in obesity from 1890-1976, you point out it was actually 4x but our data is shaky that far back. SMTM says the rate of obesity was "steady around 10%," you say that it was 13.4%. And the evidence you supply in the paper you link does show an abrupt increase in obesity in the era SMTM references.
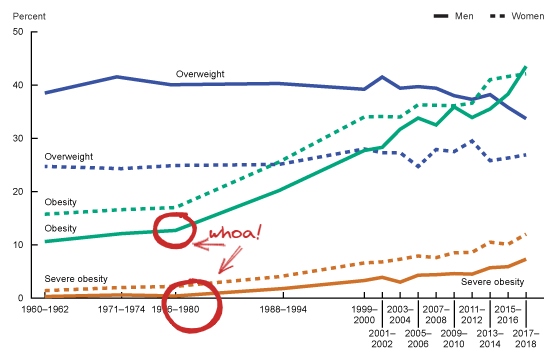
Accepting that obesity rates anywhere went up anywhere from 4x to 9x from 1900-1960 (i.e. from 1.5%-3% to 13.4%), I still think we have to explain the "elbow" in the obesity data starting in 1976-80. It really does look "steady around 10%" in the 1960-1976 era, with an abrupt change in 1976. If we'd continued to increase our obesity rates at the rate of 1960-74, we'd have less than 20% obesity today rather than the 43% obesity rate we actually experience. I think that is the phenomenon SMTM is talking about, and I think it's worth emphasizing. However, I do think their language is sufficiently imprecise (is it fair to call a tripling of obesity "people got a little heavier"?) and lacking in citations that it's worth interrogating as you have done.
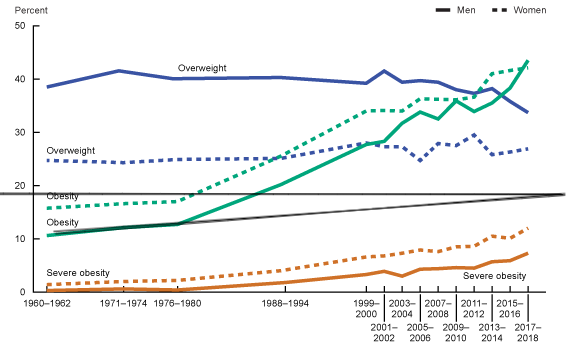
Your subsequent data may give sufficient support to your counterargument that I'd be ready to agree that there was no abrupt shift in obesity rates in the late 20th century, but if we look at this one study, I think it does look like there was an obvious abrupt shift.
Replies from: matthew-barnett, Natália Mendonça, orthonormal, NicholasKross↑ comment by Matthew Barnett (matthew-barnett) · 2023-01-29T01:50:57.937Z · LW(p) · GW(p)
Accepting that obesity rates anywhere went up anywhere from 4x to 9x from 1900-1960 (i.e. from 1.5%-3% to 13.4%), I still think we have to explain the "elbow" in the obesity data starting in 1976-80. It really does look "steady around 10%" in the 1960-1976 era, with an abrupt change in 1976. If we'd continued to increase our obesity rates at the rate of 1960-74, we'd have less than 20% obesity today rather than the 43% obesity rate we actually experience. I think that is the phenomenon SMTM is talking about, and I think it's worth emphasizing.
I think the relevant fact is that, based on the available data, it appears that average BMI increased relatively linearly and smoothly throughout the 20th century. Since BMI is approximately normally distributed (though skewed right), the seemingly sudden increase in the proportion of people obese is not surprising: it's a simple consequence of the mathematics of normal distributions.
In other words, the smooth increase in mean BMI coupled with a normal distribution over BMI in the population at any particular point in time explains away the observation that there was an abrupt change centered around roughly 1980. It is not necessary to posit a separate, further variable that increased rapidly after 1980. The existing data most plausibly supports the simple interpretation that the environmental factors that underlie the obesity epidemic have changed relatively gradually over time, with no large breaks.
I think it might be a good idea to try to visualize what's going on here, to fully understand why you can get a fairly sharp break in the proportion of the population exceeding a threshold even as the variable mean is going up gradually over time. Unfortunately, the best tool I have right now is this simple widget from Desmos that I just built that shows you what fraction of a normal distribution lies above a threshold (t). You can slide the mean variable (u) up and see the effect it has on the fraction exceeding t.
Replies from: AllAmericanBreakfast↑ comment by DirectedEvolution (AllAmericanBreakfast) · 2023-01-29T03:00:44.160Z · LW(p) · GW(p)
Let's get a clearer illustration of your point. Here's a graph of the fraction of a normally distributed population above an arbitrary threshold as the population mean varies, ending when the population mean equals the threshold. In obesity terms, we start with a normally distributed population of BMI, and increase the average BMI linearly over time, ending when the average person is obese, and determine at each timepoint what fraction of the population is obese (so 50% obesity at the end).
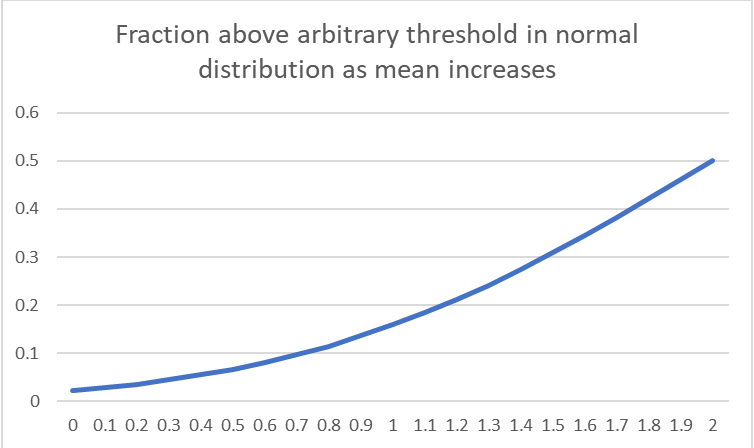
The graph above has 4 timepoints roughly evenly spaced by the decade from 1960-2000, so here's what the graph above looks like if we extract four evenly spaced timepoints to kind of simulate the spacing between the data points:
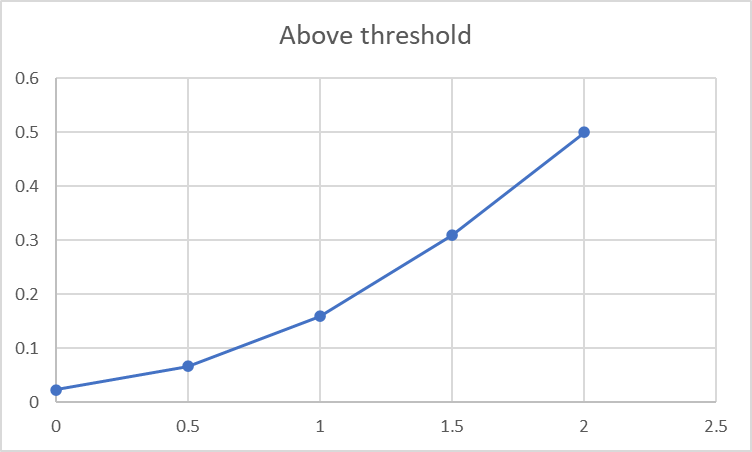
Here's the actual data for reference:
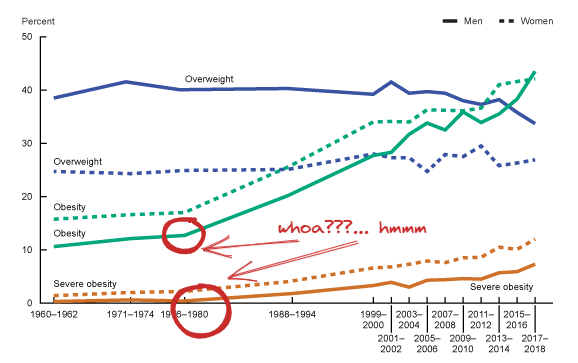
Glancing back and forth, I keep changing my mind about whether or not I think the messy empirical data is close enough to the prediction from the normal distribution to accept your conclusion, or whether that elbow feature around 1976-80 seems compelling.
This does seem like the crux of the argument here - do we see a sharp change in 1976-80 or don't we? At this point I feel like I could go either way.
Replies from: aa-m-sa, matthew-barnett, Natália Mendonça↑ comment by Aaro Salosensaari (aa-m-sa) · 2023-01-29T12:15:14.166Z · LW(p) · GW(p)
>Glancing back and forth, I keep changing my mind about whether or not I think the messy empirical data is close enough to the prediction from the normal distribution to accept your conclusion, or whether that elbow feature around 1976-80 seems compelling.
I realize you two had a long discussion about this, but my few cents: This kind of situation (eyeballing is not enough to resolve which of two models fit the data better) is exactly the kind of situation for which a concept of statistical inference is very useful.
I'm a bit too busy right now to present a computation, but my first idea would be to gather the data and run a simple "bootstrappy" simulation: 1) Get the original data set. 2) Generate k = 1 ... N simulated samples x^k = [x^k_1, ... x^k_t] form a normal distribution with linearly increasing mean mu(t) = mu + c * t at time points t= 1960 ... 2018, where c and variance are as in "linear increase hypothesis". 3) How many of simulated replicate time series have an elbow at 1980 that is equally or more extreme than observed in the data? (One could do this not too informal way by fitting a piece-wise regression model with break at t = 2018 to reach replicate time series, and computing if the two slope estimates differ by a predetermined threshold, such as the estimates recovered by fitting the same piece-wise model in the real data).
This is slightly ad hoc, and there are probably fancier statistical methods for this kind of test, or you could fits some kind of Bayesian model, but I'd think such computational exercise would be illustrative.
Replies from: AllAmericanBreakfast↑ comment by DirectedEvolution (AllAmericanBreakfast) · 2023-01-29T20:00:23.370Z · LW(p) · GW(p)
I think this is a great idea - I'm also too busy to do this right now and not equipped with that skillset, but I would read your work with interest if you chose to carry this out.
↑ comment by Matthew Barnett (matthew-barnett) · 2023-01-29T03:16:11.761Z · LW(p) · GW(p)
I admit that the data is a bit fuzzy and hard to interpret. But ultimately, we've basically reached the point at which it's hard to tell whether the data supports an abrupt shift, which to me indicates that, even if we find such a shift, it's not going to be that large. The data could very well support a minor acceleration around 1980 (indeed I think this is fairly likely, from looking at the other data).
On the one hand, that means there are some highly interesting questions to explore about what happened around 1980! But on the other hand, I think the data is essentially conclusive in its rejection of the idea that the obesity epidemic began in about 1980, which is what I interpreted SMTM to be saying.
Replies from: AllAmericanBreakfast↑ comment by DirectedEvolution (AllAmericanBreakfast) · 2023-01-29T03:28:49.689Z · LW(p) · GW(p)
But ultimately, we've basically the point at which it's hard to tell whether the data supports an abrupt shift, which to me indicates that, even if we find such a shift, it's not going to be that large. The data could very well support a minor acceleration around 1980 (indeed I think this is fairly likely, from looking at the other data).
I don't think this is the right way to look at it.
Conditional on accepting that there are two distinct linear regimes, the first linear regime from at least 1960 to 1976-80 is growing about 3x more slowly than the one from 1976-80 and on. I think that a tripling of the rate at which the obese population increases is a perfectly fine definition of "the start of the obesity epidemic."
I want to be quite clear that I'm not defending SMTM or saying that they are correct - I am just trying to make sure that we are representing accurately the underlying data and the specific claims being made. I see myself as a self-appointed referee here, not a player in the game.
Replies from: matthew-barnett↑ comment by Matthew Barnett (matthew-barnett) · 2023-01-29T03:47:25.576Z · LW(p) · GW(p)
Conditional on accepting that there are two distinct linear regimes, the first linear regime from at least 1960 to 1976-80 is growing about 3x more slowly than the one from 1976-80 and on.
But we have data going back to the late 19th century, and it demonstrates that weight was increasing smoothly, and at a moderately fast rate, before 1960. That is a crucial piece of evidence. It shows that whatever happened in about 1980 could have simply been a minor part of a longer-term trend. I don't see why we would call that the "start" of the obesity epidemic.
Replies from: AllAmericanBreakfast↑ comment by DirectedEvolution (AllAmericanBreakfast) · 2023-01-29T04:15:45.443Z · LW(p) · GW(p)
But we have data going back to the late 19th century, and it demonstrates that weight was increasing smoothly, and at a moderately fast rate, before 1960. That is a crucial piece of evidence. It shows that whatever happened in about 1980 could have simply been a minor part of a longer-term trend. I don't see why we would call that the "start" of the obesity epidemic.
An obesity epidemic is an epidemic of obesity, not of weight gain, and conventionally refers both to an increase in the rate of obesity increase and to the sheer amount of obesity in the population. It wouldn't surprise me if the trajectory looked something like this:
- Late 19th century-earlyish 20th century: increasing gains in agricultural productivity and infrastructure among other things ensure adequate food supply, increasing the population's weight to a steady state where everybody has enough to eat. This leads to a big increase in % obesity, but from a very low base rate. We don't call this an "obesity epidemic" because most of what is happening is malnourished people getting enough to eat, even though the abundant food is causing a 3x to 8x fold change in obesity from like 1.5-3% to ~10-13%.
- Mid 20th century: a steady state in which everybody has enough to eat, a constant increase in obesity but not enough to ring alarm bells
- 1974-1980: some event (contamination, whatever) disrupts the equilibrium leading to a new regime in which % obesity rises 3x faster than it was during the mid 20th century equilibrium era.
Here's how I see your and Natalia's hypothesis vs. SMTM's hypothesis. Both seem mechanistically plausible and roughly in accordance with the data, depending on which graphs you pick. So it's not that I see an overwhelming case for one or the other, just that SMTM's hypothesis implies there's "one weird trick" to not have rampant obesity and that we ought to figure out what it is, which is a "big if true" idea that motivates investigation.
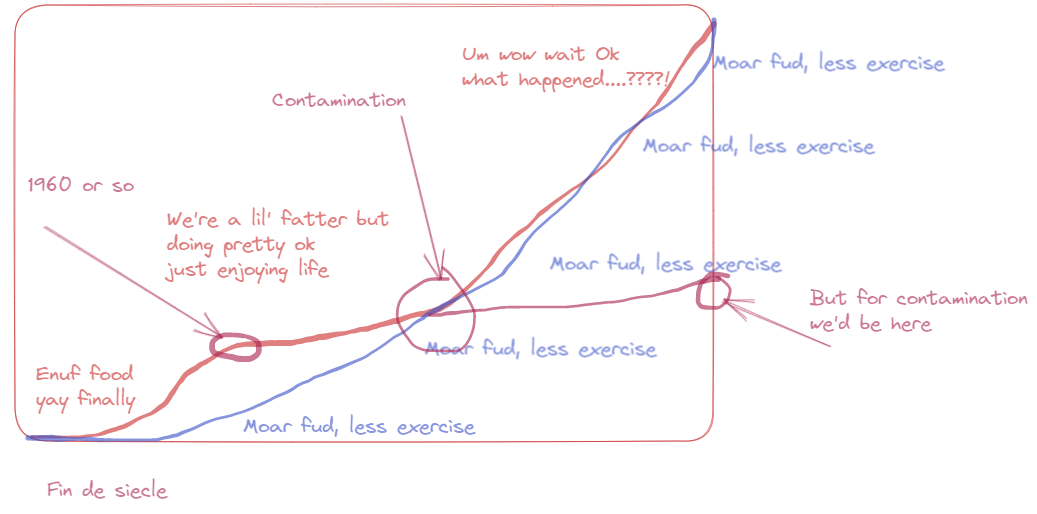
I agree that whatever happened in ~1980 could have been a minor part of a longer-term trend, but if it's not, if there was some contamination that put us on a very different trajectory into raging obesity rather than modest constant increases oevr time, then that is a pretty important thing to check out and we'd definitely call it the start of the obesity epidemic.
Replies from: matthew-barnett↑ comment by Matthew Barnett (matthew-barnett) · 2023-01-29T04:36:53.397Z · LW(p) · GW(p)
I agree that whatever happened in ~1980 could have been a minor part of a longer-term trend, but if it's not, if there was some contamination that put us on a very different trajectory into raging obesity
I agree that there's still some plausible thing that happened in 1980 that was different from the previous trend. There could be, and probably are, multiple causes of the trend of increasing weight over time. And as one trend loses steam, another could have taken over. To the extent that that's what you're saying, I agree.
But I'm still not sure I agree with the thrust of what you're saying. I maintain that the data doesn't strongly support the theory that there was a major, abrupt change around 1980. Given the relative size of the change, it seems more plausible to me that whatever happened in 1980 was simply the continuation and slight acceleration of the pre-1980 trend.
I think most of the plausibility behind thinking that 1980 is special has to do with the size of the change that we're talking about. And to be a bit blunt, I suspect you're implicitly exaggerating the size of this change as evidenced by how you drew it in your chart (although I know the chart was drawn to illustrate a point, not necessarily to show the exact sizes of the relevant changes).
However, I'm not very confident that there isn't something big going on here, and at this point I'm not sure whether the disagreement we have is mostly semantic. To me, it kind of seems like this argument might just be two people arguing about whether an effect found in the data is "big", and their entire disagreement just comes down to what they'd consider "big".
Replies from: AllAmericanBreakfast↑ comment by DirectedEvolution (AllAmericanBreakfast) · 2023-01-29T04:53:28.451Z · LW(p) · GW(p)
I maintain that the data doesn't strongly support the theory that there was a major, abrupt change around 1980. Given the relative size of the change, it seems more plausible to me that whatever happened in 1980 was simply the continuation and slightly acceleration of the pre-1980 trend.
I think our problem fundamentally with the graph in question is that we have just 5 timepoints to base our perception of the shape of the trend during this era and our data cuts off at a crucial moment. Again, what you call a "slight" acceleration appears to me as a tripling of the obesity increase rate which I do not consider slight.
Where I think our semantics are a little off is that we're most centrally debating the existence of a "contamination epidemic" that happens to result in obesity. We can both agree there was some obesity growing at a slower rate prior to 1980, and much more obesity growing at a faster rate after 1980. The question is whether this graph is evidence for a contamination epidemic, against, or neutral. My visual inspection makes me think that, in isolation, the graph better fits the SMTM hypothesis than your hypothesis, updating my priors somewhat toward the existence of a "contamination epidemic," but that's just one piece of data in the context of a much larger argument. I would be entirely open to revising my opinion contingent on more fine-grained data or on a better method to distinguish how well the data we do have fits the "shifting normal" vs. "two linear regimes" models.
Replies from: matthew-barnett↑ comment by Matthew Barnett (matthew-barnett) · 2023-01-29T05:01:22.607Z · LW(p) · GW(p)
ETA: I misinterpreted the above comment. I thought they were talking about the data, rather than the specific graph. See discussion below.
My visual inspection makes me think that, in isolation, the graph better fits the SMTM hypothesis than your hypothesis
And I'm quite confused by that, because of the chart below (and the other ones for different demographic groups). I am not saying that this single fact proves much in isolation. It doesn't disprove SMTM, for sure. But when I read your qualitative description of the shift that we're supposed to find in this data, and then compare it to what I see in the chart, I just don't get the sense that you're describing it well. Honestly I don't know what's going on here.
[ETA: note that the x-axis is the cohort birth year. And it's the output of a statistical model created using NCHS data collected between 1959 and 2006. And also, this model uses the same data as the other chart.]
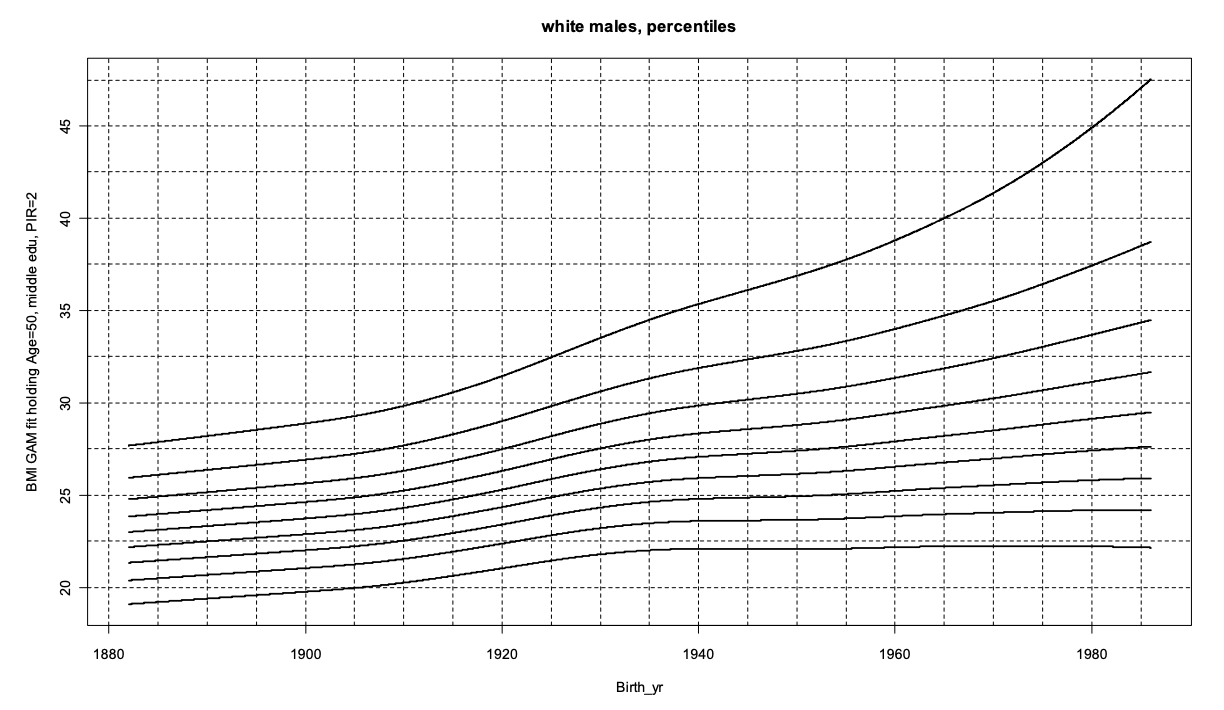
↑ comment by DirectedEvolution (AllAmericanBreakfast) · 2023-01-29T05:11:56.361Z · LW(p) · GW(p)
To be super clear, I am exclusively considering the one graph that I started this comment chain with and am not making any other claims whatsoever about the rest of the data. What I specifically said is that in isolation, the graph we have been discussing better fits the SMTM hypothesis than your hypothesis. Bringing in a separate graph that you think better supports your hypothesis than SMTM's has zero bearing on the claim that I made, which is exclusively and entirely about the one graph we have been discussing. This new comment with this new graph reads to me as changing the subject, not making a rebuttal.
This might seem unreasonable, but I think it's extremely important that we be able to see truly what a specific piece of evidence tells us in isolation. We should not let other pieces of evidence distort what we see. We should form our synthetic interpretation on the basis of truly seeing each individual piece for what it is, and then building up our interpretation from there. When I look individually at the one graph we've been discussing and don't consider the rest, I see an abrupt change between two different linear paths more than I see a smooth exponential increase.
Replies from: matthew-barnett, matthew-barnett↑ comment by Matthew Barnett (matthew-barnett) · 2023-01-29T05:51:21.745Z · LW(p) · GW(p)
Note: I deleted and re-posted this comment since I felt it was missing key context and I was misinterpreting you previously.
What I specifically said is that in isolation, the graph we have been discussing better fits the SMTM hypothesis than your hypothesis. Bringing in a separate graph that you think better supports your hypothesis than SMTM's has zero bearing on the claim that I made, which is exclusively and entirely about the one graph we have been discussing. This new comment with this new graph reads to me as changing the subject, not making a rebuttal.
To be clear, I said I was "confused", not that I disagreed because of additional evidence. But I did misinterpret what you were saying, ultimately. So, let me try again.
I don't know how to reconcile any differences between the chart I brought up and the chart you mentioned. Note that they're drawn from the same data, so in theory my chart is showing is simply how BMI moves through time, whereas yours is showing the same thing but under a different transformation; namely, it shows the proportion of people who are above a certain BMI threshold.
My rough guess is, and my initial implicit assumption was, that the two charts are simply consistent and the original point I made about shifting a normal distribution still holds, and the apparent shift is mostly an illusion, with the caveat that there's a slight acceleration (as shown in the chart I brought up). The reason why I brought up the chart is because I assumed you agreed that they were consistent, but simply thought that the acceleration in BMI was large. I wanted to say: "I don't agree. See how it looks when you just follow BMI through time. There's barely any acceleration!"
Sorry about the misinterpretation.
Replies from: AllAmericanBreakfast↑ comment by DirectedEvolution (AllAmericanBreakfast) · 2023-01-29T06:29:43.382Z · LW(p) · GW(p)
No worries! I am approaching this debate in a collaborative spirit. I may have been misunderstanding you as well.
What I see when I examine the second graph you have shown, again in isolation, is that it does indeed look very much like the results of the "shifted normal" model you described earlier. Or rather, of that process happening twice, with a sort of temporary tapering off around 1940. Although if I'm understanding right, the earlier pre-60s part is pure extrapolation. This graph clearly fits your and Natalia's hypothesis and not SMTM's, we see nothing of particular significance around 1980.
As you say, the next question becomes how to decide which to put more weight on. Do we like the statistical heft of Komlos and Brabec, or do we think they're just using fancy statistics to erase a crucial feature of the raw data? I don't know how to arbitrate that question. But I would be sympathetic to an interpreter who said they were convinced by the sophisticated statistical model and viewed the apparent "elbow" in the raw data as more likely an artifact than a real feature of the true trend, and I'm sure Komlos and Brabec know much better than me what they're about.
My way of proceeding would be to say "looking at the raw data, it does look like there's a sharp change around 1980, but that might be an artifact. Looking at a sophisticated curve-fitting model of similar data, that feature vanishes. We might put 80% weight on the sophisticated modeling and 20% on the raw data, and note that the raw data itself isn't so incompatible with a "shifted normal" interpretation, maybe 70/30." Overall, I'm inclined to put maybe 85% credence in the "shifted normal" interpretation in which there was no big event in obesity around 1980, and 15% credence that a real " elbow feature" is being obscured by the statistical smoothing.
↑ comment by Matthew Barnett (matthew-barnett) · 2023-01-29T05:27:41.527Z · LW(p) · GW(p)
↑ comment by Natália (Natália Mendonça) · 2023-01-29T05:20:00.681Z · LW(p) · GW(p)
I think that, when you cite that chart, it's useful for readers if you point out that it's the output of a statistical model created using NCHS data collected between 1959 and 2006.
Replies from: matthew-barnett↑ comment by Matthew Barnett (matthew-barnett) · 2023-01-29T05:21:03.677Z · LW(p) · GW(p)
OK, I'll add that to my comment.
↑ comment by Natália (Natália Mendonça) · 2023-02-02T16:00:37.418Z · LW(p) · GW(p)
Of note, your charts with simulated data don’t take into account that there was a midcentury slowdown in the increase in BMI percentiles, which, as I said in the post, probably contributes to the appearance of an abrupt change in the late 20th century.
↑ comment by Natália (Natália Mendonça) · 2023-01-29T04:25:38.234Z · LW(p) · GW(p)
Thank you for the feedback, I'll try to rephrase that section. It does seem that a lot of the disagreement here is semantic.
Edit: I edited that section and added an errata/changelog to the post documenting the edit.
Replies from: AllAmericanBreakfast↑ comment by DirectedEvolution (AllAmericanBreakfast) · 2023-01-29T04:37:27.914Z · LW(p) · GW(p)
Cheers! You always write great posts and I appreciate your receptivity to feedback.
↑ comment by orthonormal · 2023-04-03T23:53:42.562Z · LW(p) · GW(p)
Looking for "elbows" in a noisy time series with relatively few points is a pretty easy way to get spurious results. If the 1960-62 obesity number was overestimated by 2 points and/or the 1976-1980 number was underestimated by 2 points, it wouldn't look like 1976-1980 was a special transition at all.
(And clearly errors of that magnitude happen, unless you think there's a deep reason why obesity rates were nonmonotonic from 2005-06 to 2011-12.)
Replies from: AllAmericanBreakfast↑ comment by DirectedEvolution (AllAmericanBreakfast) · 2023-04-04T00:48:00.053Z · LW(p) · GW(p)
That's true, I just think that seeing an elbow is more compatible with there being an actual elbow than there not being an elbow. It's not definitive, just interesting and potentially worth further study.
↑ comment by Nicholas / Heather Kross (NicholasKross) · 2023-04-28T01:04:54.210Z · LW(p) · GW(p)
Strongly upvoted to normalize "local counterargument" and "argument tree" in LW comments.
comment by DirectedEvolution (AllAmericanBreakfast) · 2023-01-29T20:46:37.283Z · LW(p) · GW(p)
I'd be curious to find out how Klimentidis (click for contact info) would respond if you showed him your reanalysis of lab mouse weights. I notice that his data is from roughly 1980-2000, while yours is from ~2000-2020, but I don't think that's a very satisfying answer ot why you are coming up with such a different result.
comment by Robert Jones (robert-jones-1) · 2023-01-28T23:57:43.475Z · LW(p) · GW(p)
I not believe that your brain has a lipostat: https://www.frontiersin.org/articles/10.3389/fnut.2022.826334/full.
Aerobic exercise has no effect on resting metabolic rate, while resistance exercise increases it: https://www.tandfonline.com/doi/full/10.1080/02640414.2020.1754716. The claim in the article you link (which even the article treats with a degree of skepticism) may be explained by the runners running more efficiently as the race progressed: it's certainly not plausible that the athletes' resting metabolic rates dropped by 1,300 kcal/day, and no such claim is made in the article linked in support of the claim by the first article (https://www.science.org/content/article/study-marathon-runners-reveals-hard-limit-human-endurance).
Replies from: Natália Mendonça↑ comment by Natália (Natália Mendonça) · 2023-01-29T00:32:28.630Z · LW(p) · GW(p)
I not believe that your brain has a lipostat: https://www.frontiersin.org/articles/10.3389/fnut.2022.826334/full.
There’s an extra period in the URL, so the link doesn’t work. But this is intriguing and I’ll look into it — thank you!
Aerobic exercise has no effect on resting metabolic rate, while resistance exercise increases it: https://www.tandfonline.com/doi/abs/10.1080/02640414.2020.1754716. The claim in the article you link (which even the article treats with a degree of skepticism) may be explained by the runners running more efficiently as the race progressed: it's certainly not plausible that the athletes' resting metabolic rates dropped by 1,300 kcal/day, and no such claim is made in the article linked in support of the claim by the first article (https://www.science.org/content/article/study-marathon-runners-reveals-hard-limit-human-endurance).
I think the most important & interesting finding in Herman Pontzer’s energy expenditure research is that hunter-gatherers don’t burn more energy than people in market economies after adjusting for body mass, even though they exercise more. From the ground-breaking Pontzer et al. (2012):
↑ comment by Robert Jones (robert-jones-1) · 2023-01-29T12:52:56.853Z · LW(p) · GW(p)
I've now done some more reading (including reading the 2012 paper more carefully).
What is surprising about the 2012 result (at least to me) is not that TEE (adjusted for FFM) is similar in different populations. That is consistent with other results, e.g. Westererp & Speakman (2008), which finds that TEE (which they call DEE) (a) has not changed significantly over time in Europe since the 1980s, (b) is not significantly different between populations in Europe/North America and those in the third world and (c) is not significantly different between modern humans and wild animals of the same size.
What makes the 2012 result surprising is that the PALs are different. For TEE to remain constant with varying PAL would require a strict negative relationship between PAL and RMR, so that increases in AEE were exactly compensated for by decreases in RMR. This seems intuitively unlikely. In fact, Pontzer et al (2016) finds that RMR is not correlated with physical activity.[1] I read the 2016 paper as showing some shift in Pontzer's view generally, as he there advocates for a "constrained total energy expenditure model", while allowing that TEE can and does vary within those contraints.
The surprising difference in PALs between the groups in the 2012 paper may plausibly be a result of a limitation in the experimental method, namely that the PALs for the Hadza subjects have been calculated using estimated rather than measured BMRs. Rather oddly, the experimenters did measure the Hadza subjects' RMRs, but then threw the measurements out (giving some cogent reasons for doing so). The Hadza BMRs were estimated by "enter[ing] each subject’s body mass and height into age-specific prediction equations developed in a large sample (n = 10,552) from a geographically broad set of populations that includes populations in sub-Saharan Africa," but formulaic estimates of BMR notoriously differ widely from measured BMRs, and a broad sample will necessarily not reflect any factors particular of the Hadza.
What is true is that PAL behaves in some unintuitive ways. See, for example, Westererp (2013). This tells us that 72-78% of the variance in PAL is genetic. PAL decreases with age after 50 (and increases with age in children), but it does not vary significantly with body weight (save for subjects with BMI>35) or with body composition (after controlling for age). Exercise increases PAL in young adults eating ad libitum (who increase dietary intake to compensate) but does not increase PAL either in older adults or in young adults subject to an energy-restricted diet. It appears that the second two groups compensate for the increase in intentional activty by decreasing non-intentional activity, so as to keep AEE roughly constant. PAL decreases under starvation (i.e. significant underfeeding of normal weight subjects), but does not seem to be significantly affected by underfeeding in overweight and obese subjects.[2] Similarly, there is no significant effect of overfeeding on PAL, when the overfeeding is lower than twice maintenance. PALs in Western countries have increased slightly since 1990 (during which time obesity levels have increased markedly) and as previously noted are in line with those found in wild mammals, so the common perception that we are particularly inactive is mistaken.
Looking the evidence as a whole, I do not believe it supports the claim that exercising more causes your body to expend less energy on maintenance and repair, although it does usually cause your body to compensate by either (a) eating more food or (b) reducing non-intentional activity.
- ^
Although the authors make no attempt to distinguish between aerobic and resistance exercise.
- ^
Although see Westererp (2016). This cites different studies showing that underfeeding obese individuals leads to a small reduction in both AEE and RMR (which he calls REE). These would tend to cancel each other out, to keep PAL constant. But the reduction in AEE is not sustained after energy intake is raised to match TEE, whereas the reduction in RMR is, which should lead to an increase in PAL. These numbers are all small though.
↑ comment by Robert Jones (robert-jones-1) · 2023-01-29T08:06:51.902Z · LW(p) · GW(p)
Thanks. I think I've fixed the links now.
While the Pontzer et al (2012) result is interesting, I don't read too much into it. For women, the difference in PAL is small (and strictly, not significant). The difference is larger for men (Hadza about 25% higher), but there are only 31 men in the sample. Probably more importantly, these populations will differ in many ways, which may affect their BMRs. It certainly doesn't shift my view from the meta-analysis I linked, and indeed the paper itself says, "It is important to note that this was not an intervention study; we examined habitual TEE, PAL, and body composition in hunter-gatherers and Westerners, but did not examine the effects of imposing increased physical activity on Westerners. Physical activity has important, positive effects on health, and increased physical activity has been shown to play an important role in weight loss and weight-maintenance programs."
FWIW, I do agree with the conclusion (contrary to what I once believed) that "differences in obesity prevalence between populations result primarily from differences in energy intake rather than expenditure". The important evidence for me here is that changes in population level obesity over time fail to match changes in physical activity. It doesn't follow that you personally cannot outrun your fork, and the ACX survey results suggest that at least some people can (as I intend to say on the open thread), although I don't necessarily recommend trying it.
comment by Raemon · 2023-03-28T01:11:17.063Z · LW(p) · GW(p)
This is a bit later-than-usual, but, curated.
I've continued to appreciate Natalia digging into the details here. The spot check on "did lab and/or wild animals get more obese" seemed pretty significant. I also liked tying everything in at the end to a concrete metaculus prediction.
comment by Ethan Sterling (ethan-sterling) · 2023-01-29T21:17:14.042Z · LW(p) · GW(p)
BMI isn't fatness. BMI is just weight / (height^2), which means that BMI grows linearly in proportion to a human's scale. (Weight grows with the cube of dimensions but height^2 only grows with, well, the square.) BMI also fails to distinguish between muscle, bone mass, and fat. If, say, human heights had been increasing over time (while maintaining other bodily proportions), you'd expect BMI to increase over time.
That's not to say humans aren't getting fatter, but if you wanna hypothesize on the causes of trends in human fatness, you need data on human fatness. Not BMI.
Replies from: just_browsing, Ekefa, Making_Philosophy_Better, jkaufman, Linch↑ comment by just_browsing · 2023-01-31T19:17:33.136Z · LW(p) · GW(p)
Average BMI in the United States increased from 25.2 in 1975 to 28.9 in 2014, so a 3 point increase. Compare an average 1975 person with an average 2014 person. It's far more likely that the 3 point increase is due to overeating, rather than other explanations like packing on muscle (3 whole points of muscle is a lot) or variation in bone mass (this is likely negligible). Overeating is the path of least resistance in wealthy Western countries. So yes, technically BMI is not the same thing as fatness, but they are highly correlated.
Also as Rockenots points out, the direction of your height claim is going in the wrong way. BMI is an underestimate for fatness for very tall people. For example, a healthy weight 6'2" man's BMI might be 17 or 18, which according to the standard BMI scale is underweight. That's why measures like better BMI exist.
↑ comment by Rockenots (Ekefa) · 2023-01-30T03:01:08.211Z · LW(p) · GW(p)
It turns out that in adults, BMI is negatively correlated with height. So, if human heights have been increasing over time, we'd actually expect BMI to decrease over time.
↑ comment by Portia (Making_Philosophy_Better) · 2023-04-09T14:25:56.215Z · LW(p) · GW(p)
While this is technically true, in practice, this argument actually works in the opposite direction.
It is extremely rare for a human to have an obese BMI, while having low body fat %. This essentially only occurs in Bodybuilders. If you are in this group, noone will ever misidentify you as obese, because you will be fucking ripped. The book "Overcoming Fatlogic", which I strongly recommend, has some images of extremely fit people with normal BMIs that really make that point emotionally/visually too, beyond the strong statistical support.
On the other hand, it is becoming increasingly common in our sedentary society for people to have a normal BMI, but be "skinnyfat", that is, having such little muscle mass that their fat % still makes them sick.
Body fat percentage is also tricky to measure (body fat scales are unreliable, calipers are unreliable, visual assessments by pros are unreliable, and all of these require either expensive devices or professionals, or both); BMI is trivial to measure. For any really large study, it is the only thing that is feasible.
On a population level, BMI will under-, not overestimate obesity. It is a useful tool, and if BMI seems bad, the situation on a population level will currently be even worse.
↑ comment by jefftk (jkaufman) · 2023-01-29T22:33:21.007Z · LW(p) · GW(p)
How much of the data on increasing BMI is explained by increasing height, I wonder?
↑ comment by Linch · 2023-01-30T10:31:57.527Z · LW(p) · GW(p)
BMI isn't fatness. BMI is just weight / (height^2), which means that BMI grows linearly in proportion to a human's scale. (Weight grows with the cube of dimensions but height^2 only grows with, well, the square.)
I used to believe this. I currently think this is aesthetically correct (ish?) but not biologically. Specifically I think there are greater health premiums for being thin when you're taller.
comment by JenniferRM · 2023-03-28T16:25:07.143Z · LW(p) · GW(p)
I was surprised that the essay ended where it did. I thought it was going to offer the alternative hypothesis of memetic contamination with "bad nutrition ideas" as "the contaminant".
This seemed connected with "college" and "recently coming from some place with a different food culture" being protective. Then combine that with the title itself: "On not getting contaminated by the wrong obesity ideas"... I kept waiting for that shoe to drop... and it never did!
A thing that you didn't do, that no one ever does, is regress on IQ, which would have the naive likely effect of giving people more thoughts about more ideas over time, and thus being able to notice good ideas and keep them, and notice bad ideas and reject them. I was kind of predicting you would add IQ though, because it would help with the thesis I thought you were driving towards.
This is the second time I've read something where it seemed like the obvious correct move was to write about "meta-nutrition" and then it never happened. The first time was The Omnivore's Dilemma where the necessity of dealing (inside a single life) with complexly varying food sources is pointed to as a challenge for all omnivorous species including humans, and I was thinking I'd get a really fascinating book on the evolution of the parts of the brain that handle this challenge... and then for the entire rest of the book he just talked about "boo McDonalds" and "organic isn't what it used to be" and other boring social takes :-(
It would have been funny if "the contaminant is coming from inside the memes!" was taken in a directly serious way, and I'm wondering if there is an explicit positive reason you didn't go there, or was it maybe just not in your space of hypotheses, or... ?
Replies from: JenniferRM↑ comment by JenniferRM · 2023-03-28T19:11:42.660Z · LW(p) · GW(p)
If there's a diet plan buried here, it might be "treat almost everyone in the modern environment as lying about almost everything almost all the time, including nutritionists and food advertisements, and anyone you pay or anyone who asks you for money, or anyone who offers you free stuff in a way that seems too good to be true... maybe not always literally technically lying, but almost certainly misleading, by violating norms of gricean implicature, or abusing the halo effect, or leaving key facts out, or polishing their shit to make it shinier and more convincing than it should be, or encouraging hasty generalization, or whatever".
There is a meme going around about the now-morally-monstrous-seeming ads about sugar water.
Some of these, it turns out, were doctored to seem worse than they were, because of course everyone is lying about everything almost all the time, including about how much lying there has been and how it actually worked :cryglasses:
I tracked down an archive that might have a real example of circa-1956 memetic poison, however:
↑ comment by Portia (Making_Philosophy_Better) · 2023-04-09T16:01:57.240Z · LW(p) · GW(p)
But you do not need a nutritionist or diet plan or fancy products.
A successful diet does three things:
- It has fewer calories than you eat now.
- It still has the nutrition you need (especially protein, omega 3, sufficient vegetables)
- It is easy for you, you personally in particular, to adhere to. (This generally means: you think it tastes good; it makes you feel full; you can afford the money and time commitment for it permanently; it does not make you feel stressed, so you will happily maintain it while lots of shit goes down in your life, for the rest of your life.)
Here is a relatively good strategy for finding a tailored diet strategy for yourself. I am giving it to you for free.
Step 1:
Weigh yourself daily under the same conditions (e.g. after waking up, after peeing, before drinking; leave the scale standing in the exact same spot) enter it into an app. (There are apps that do this by syncing with your scale without you needing to look at the number, in case seeing it bothers you. The individual number is near meaningless, as fat fluctuations are massively outweighed by water fluctuations, especially if you are running on naturally produced estrogen. You want the trend. This data will not be remotely useful until you have tracked it for at least one month, and it is not what we will use in selecting the original diet strategy. I strongly recommend maintaining this habit indefinitely; it only takes a few seconds.)
Continue eating exactly how you feel like eating, but track all your food. (Including drinks, sugarfree sweets/gums, fish oil supplements, cheese samples in the supermarket, the French fries you stole from your partner). You will not have to do this indefinitely, though you can if you like; you are doing this while evaluating diet strategies, and again if you ever hit a plateau in your weight loss to debug. It will be tedious at first, but you will quickly get a lot faster as your favourite foods end on shortcuts.
Important: Weigh each item. I repeat; weigh each item. And enter the weight to the gram. Any guestimates are utterly useless, you might as well not track at all. Put it into an app that captures calories and protein for commercial and natural products. There are several apps that do this for free, I used to use fddb. You will also need a relatively fine kitchen scale. Those cost like 10 bucks.
A scale for yourself and the food are the only financial investments needed. Ask around: You might get ones for free as friends upgrade to fancier or prettier ones.
Also track workouts.
Step 2:
Review after you have some data. You will typically find that some days, you consumed a lot fewer calories compared to what you burned, or got a lot more nutrition, even though you expended the same amount of effort, and got the same amount of pleasure; you weren't consciously dieting yet, after all. What characterises these days? Can you engineer your life to be more like them? This is where you might note that there are foods you aren't that fond of or find that filling, but that are calorie bombs (I feel that way about whipped cream and cereal bars. How can something taste this bland and have such a boring consistency, while having this many calories? Incomprehensible); and others you fucking love and find filling, that aren't actually that high in calories. You might also find that you are actually fine skipping breakfast. From now on, when you find two foods or dayplans equally satisfying but one of them has fewer calories or more nutrition, eat that one. E.g. I realised that on days where I eat pasta, I consume on average 400 (!) calories more, even though I genuinely do not feel like I have eaten more. So I eat pasta if I want to cram calories effortlessly (e.g. before a marathon), but avoid it when my weight is rising, instead having potatoes, where the opposite happens for me. I also found that a lot of supposedly "evil" foods (like chocolate sprinkles) are totally fine in small quantities (10 g of chocolate sprinkles make my yoghurt so much cooler, while doing so little to the calorie counts), while a lot of supposed "diet" foods have ridiculously high calorie counts for how shit they taste - and because they taste so shit, you end up eating several of them and still feeling grumpy, so you would have been better off having a small slice of real cake.)
Step 3:
Develop a strategy matching you personally for reducing calories while maintaining happiness.
You will typically begin noticing similarities and trends in your happyness-to-fat-ratio. Most diets come down to the same strategies for keeping happiness constant while reducing calories. Whether a particular strategy works is highly individual. Try it out. You determine whether the strategy worked if you are genuinely happy, and getting enough protein, omega 3 and veg, but your calorie consumption has gone down.
The common strategies are: (You can mix and match between them)
- Reduce fat. (This works because fat is very calorie dense, so you still get to eat a lot of food volume-wise, but the calorie count is strongly reduced. In its extreme forms, it fails in people who find the resulting food tastes so bland (fat carries flavours and allows frying) they are so frustrated they eat even more of it, more than making up the calorie reduction. Also some fats are crucial to health, e.g. for fat soluble vitamin absorption, and omega 3. It also has a failure mode when people just replace the fat with lots of extra carbs, which are also highly caloric; this is very common in low fat ready made "diet" foods offered. In its minor forms - e.g. just measuring the cooking oil you use in teaspoons, being a bit more sparing with cheese and nuts, diluting coconut milk - it has surprisingly high impact. I detest extremely low fat diets, but lower fat diets work for me. Check your app to see the impact.
- Reduce carbs, either
- Radically reduce carbs total: This works because human bodies can generally do without ingesting carbs; they genuinely need very few, and your body can generate them from protein if it really has to. If you completely deprive your body of carbs, it switches to ketosis, which leaves you with more stable blood energy levels via ketone bodies, reducing hunger cravings. Many people on low carb diets find they are simply less hungry, and hence, eating less becomes effortless. Other people do badly on it. The people who do badly on it often run on estrogen; it seems to sometimes disrupt cycles. People also tend to hate these diets if their favourite foods are carb based. (If your favourite foods are bread, pasta and fruit, you will simply not become happy on keto, period.) And there are types of workouts - those relying on explosive movements - which simply don't go as well without carbs. You won't know until you try it. Warning: For many people, the transition to ketosis sucks; you might spend two days cranky with headaches. Some people have this experience every time they have some carbs and then take them away, so ketosis only works for them as an all or nothing strategy. Strong individual variation, but some people really find it miraculously easy and wonderful.
- Reduce simple carbs. This is mostly relevant because it keeps carbs in your bloodstream, avoiding the problems above, but keeps the levels more stable. This basically operates by reducing sugar (keeping real, tasty sugar, but using less, by eating more savoury stuff, training yourself to like products which simply contain less, etc.; your taste buds will adjust after a while) or replacing sugar with lower calorie alternatives that are healthy (ideally with monk fruit extract, erythrol, vanilla, etc.). Try which of the two works better for you; some people find erythrol just as tasty, some find it so gross they would rather eat less sweet stuff than eat fake sweetness. Also using whole grain products instead of white flour/rice/etc. products. - I have found pairing limited carb reduction and simple carb avoidance works for me as a good intermediate strategy; not as restrictive and without the negative impacts on workouts from ketosis, and without the shitty going in and out I had when I broke ketosis. Also beneficial for your teeth and inflammation levels.
- Add fibre and water and probiotics and fermented foods
- For one, extra fibre keeps you physically full. It varies how responsive people are to this; some people are tricked (they spent so much time chewing and now their stomach is full, so they are not hungry), others feel the full stomach but are hungry regardless (they feel stuffed with empty crap). Imagine eating four cucumbers. These have almost no calories, but you are now crammed with food. Do you feel satisfied or just annoyed? Does this change if you dip the cucumbers in a high protein food like yoghurt with garlic? Again, you just gotta try it out.
- The most classic high fibre high water food is vegetables. Increased vegetable consumption is one of the very few dietary recommendations that is near universally accepted and has seriously good empirical backing. Whether this helps you lose weight or not, it will be good for your health if you eat more veggies.
- Your gut bacteria have a massive impact on your food cravings. Shifting them will make things a lot easier. They like fibre they can eat (FODMAPS), fibre they can live in (e.g. psyllium), and novel good bacteria to trade genetic material with (fermented foods like yoghurt, kimchi, sauerkraut, soy sauce, natto, tempeh, etc.). A general probiotic can also help.
- Eat everything, but less of it
- This can easily be achieved by getting much smaller plates, and buying things in smaller quantities. Pros: Nothing is forbidden. You can have chocolate - just one piece. You can have snickers - just one every few days. Cons: It can be really hard to stop at one, even harder than having none at all. This one only works for me if I get my partner to hide the rest.
- Avoid some things entirely
- This is good if you are an all or nothing person. I recommend picking something you can really bear to lose, and that has a massive impact, or making this only a temporary thing, or having cheat days where you get a little. If you add absolutely every high calorie food to the avoid list indefinitely, you are likely to eventually lose your shit and binge eat.
- Daily small changes (using the above ideas)
- The idea here is that because the changes are small, they are less stressful, and because it is a permanent habit, you stay stable.
- It is less stressful for your body, and comes with a lower risk of fucking up your muscle mass or getting a deficiency.
- Regular weight monitoring and recurring intense diets
- The basic idea here is you weigh yourself, and if you breach a particular weight and have the time, you do a couple weeks of intense dieting, using a strategy you cannot permanently maintain, but that has intense results and that you are happy to do for a while; then you go back to your former habits, and the weight slowly creeps back, until you breach it again and reset. As a consequence, you weight is on average between your end diet weight and your begin diet weight, which should both be chosen to be in the normal range. You gotta be careful here; intense diets require careful tracking so you make sure you get all the nutrients (especially protein, else muscle less), you will want to run blood tests and continue exercises and track what you are eating again. But despite how many people shit on this strategy nowadays, it is totally viable, and is often a good solution for people who need quick results for motivation and like intensity and freedom.
- Fasting periods where you literally eat just nothing for a time.
- In its gentlest forms, this means: if you don't want to eat, don't. Perfectly fine. There is no moral or health obligation to eat lunch or breakfast. Your metabolism won't break, you damn metabolism is fine. If you aren't hungry, or you wouldn't enjoy the food, there is nothing harmful about just not eating it. There is this bizarre idea that if you do not eat breakfast, you will get fat, or if you skip lunch, your relationship with food is broken. There is zero empirical backing for this. There are many normal, happy people across all cultures and times who have done this.
- If you notice that this not wanting to eat is common at some times (e.g. you hate having breakfast), you can do intermittent fasting. The idea is that you skip a meal, and then later have more calories left over for the others, and end up not using them all.
- You can also combine this with the above "recurring intense diets", e.g. doing a fast (eating nothing for e.g. four days every two months). Take it easy for those days, and be careful about going over (again, blood test, doctor supervision); eating nothing is stressful for some organs, and will after a while lead to nutrient deficiencies that cause damage.
- Fasting utilises the same principle as ketosis; you will often find that you stop being hungry on day two, finding it relatively easy in contrast to constantly eating and having to stop yourself in quantity. It is easier than you would think.
- This one might also have interesting longevity implications. Fasting within limits seems to be good for humans.
- Burning more calories (primarily by working out).
- Cardio: Burns a lot of calories in the moment. You can burn 800 in an hour on a crosstrainer.
- Resistance training: Changes how many calories you burn in general. So the effect in the hour of weight lifting is not so high, but once you have built the muscle, your daily consumption is permanently higher.
- Higher NEAT by adding more minor movements through your day; think walking or cycling instead of driving, light yoga, a standing desk, etc. Individually little, but when you make it habit changes, the impact can be remarkable.
- Disclaimer here: working out is wonderful and healthy and I recommend it in every way. But a lot of people get hungry when they work out, and they massively overestimate how many calories they burnt, and end up eating more than they burnt. Be very conservative in your estimates.
Metastrategy:
- Whatever you are avoiding, make it easy to adhere to. That means: If you wish to eat less sugar, do not keep sugary things in your house. Don't buy them and then try to win a staring contest.
- Whatever you want to do more of, make it easy and nice to do. That means: If you want to do more workouts, find a workout you find fun, a workout buddy to push you, a personal trainer to push you, pair working out with music or something else you love; else you will eventually run out of energy to make yourself.
- Make your social environment supportive. E.g. if possible, diet together with your partner. Eating diet food while your partner has French Fries right in front of you will nearly always end up with you stealing French fries. Go to places where people are supportive (e.g. the gym, weight loss forums, hiking groups, etc.) and avoid places where people will support overeating (dieting while walking into Dunkin' Donuts is not easy). If you speak German, I can recommend the forum "Abnehmen ohne Unsinn". It is the only diet forum I have ever been in that is radically pro science, open about multiple approaches as long as they leave the person with fewer calories and sufficient nutrition, neither supportive of anorexia nor panicky about disordered eating, and most of all, that forum has an incredible success rate. The vast majority of people in there lose a lot of weight (often from 150 kg to 60 kg and similar) and keep it off. A fair amount of the forum is in the maintenance state, where people have lost all the weight they wanted, and just want to make sure it stays off by keeping an eye on it, with many meanwhile thin people just there because they made friends.
Very important:
The measurement for whether your diet strategy works is not short term weight loss (this will be invisible/unreliable*), but whether you feel good while your calorie consumption has gone down across a longer time period. If your strategy has reduced calories, you will lose fat. For every 7000 calories you reduce, you will lose 1 kg of fat. 7000 calories is a lot. Play around with that, and you will get a pretty good idea of the speed you should be seeing, and realise that you are doing fine.
** The amount of water your body retains fluctuates massively. You are heavier in the evening than in the morning. Heavier before your period than after. Heavier when your inflammation is rising (e.g. when getting sick). Heavier when stressed. Heavier when sleep deprived. Heavier when eating a high carb diet. Heavier when eating a high salt diet. My water weight fluctuates by about 2 kg from morning to night, and around 4 kg per month, and I am slim. In fat people, this fluctuation can be 10 kg. It is so much faster and larger than the fact that you lost 100 g in fat. You will see the fat loss eventually (if you have lost 5 kg of fat, this is visible, and your data tracking will make it clear, as your highs and lows have shifted), but in the short term and when evaluating strategies, just focus on calorie reduction.
Final note: Anyone can lose weight. Your metabolism is fine; the variations that do exist can easily be outdieted, and speeding up your metabolism beyond what you can achieve with coffee is not a good idea. That said, we are living in a society in which high calorie low value cheap food is abundant and advertised, and movement is not necessary and often obstructed, and there are ridiculous numbers of diet myths. This is a shitty system, and it is not your fault if you fall for it. The system should change. But until it does, you can make meaningful changes within it and reach a healthy weight, it is just harder than it should be. It will be harder when you are poor. Harder when you are depressed. Harder if you have an above-average preference for fatty and/or sweet things or hunger. But it is doable, and it doesn't have to be as hard as people make it out to be, once you throw out any strategy that does not work for you and just keep the ones which do.
TL;DR: You do not need to pay anyone in order to diet successfully. There are no magic secrets or magic products. Nor do you need them.
Replies from: JenniferRM↑ comment by JenniferRM · 2023-04-09T20:24:45.849Z · LW(p) · GW(p)
Maybe this is for someone other than me? You used the word "you" a lot in a way that probably wasn't actually referring to me. I'm not fat. I systematically avoid "fake food made by lying liars", avoid eating when not hungry, stop when full, and joyfully follow cravings and eating "real food" I've never eaten before. So far this is enough (for me anyway).
comment by viking_math · 2023-01-29T01:41:54.529Z · LW(p) · GW(p)
"I think this is flawed. Clearly, overeating for your entire life will probably have different effects from overeating for 22 days. There are a lot of 22-day periods in a person’s life. Someone on their 30th birthday has gone through nearly 500 of them."
This is true, but doesn't the same critique apply to most of the hypoxia studies you cite? They're all a few weeks or shorter (or are performed on animals) and most of them seem to have small effect sizes (a few pounds). Of course, these effects could accumulate, but they could also rebound.
"In fact, studies that simulate high altitude with hypobaria (as opposed to normobaric hypoxia) seem to show greater effects on hunger perception than studies that actually take people to high altitudes. "
This result doesn't surprise me. I've spent a lot of time at altitude (5-10 thousand feet above sea level) in dry conditions, but on the ground, and never noticed food to be any less flavorful, even when coming straight from sea level. I would guess some other factor is impacting airplanes specifically (don't airplanes aggressively filter air? that seems like it could remove some scent-related particles).
comment by johnlawrenceaspden · 2023-01-29T12:54:40.949Z · LW(p) · GW(p)
Great stuff Natália, and most readable and well-written too!
Do you have any thoughts on the 'seed oil' idea? Which I'd express as:
- unsaturated fats have gone from a tiny part of our diet to a huge part
- the pathway that burns unsaturated fats is rate-limited for some reason, so over a certain low level, they clog up your metabolism and get saved for later
Therefore you can imagine that a person who has eaten a lot of seed oils/polyunsaturated fats can be overweight whilst still starving. And you might also expect them to have a slow metabolism and be showing the symptoms of mild hypothyroidism (low temperature, fatigue) without actually having a thyroid problem.
This seems to me to explain an awful lot of stuff, but as Scott pointed out in https://slatestarcodex.com/2020/03/10/for-then-against-high-saturated-fat-diets/ all the direct evidence seems against it.
anti-seed-oil people would say that the problem is that:
- the research is all wrong about what fats are saturated and what aren't (because animals get fed on seed oils, so pork and chicken fat is largely polyunsaturated these days!)
- and that even if you manage to cut out all unsaturated fats from your diet, you'll still burn off the stored unsaturated fats very slowly.
At the moment this looks to me like a terrific idea that explains everything, but I'm trying to be sceptical and would really like to hear your thoughts against it.
↑ comment by johnlawrenceaspden · 2023-01-29T13:09:03.506Z · LW(p) · GW(p)
The slime mold guys talk about this:
https://slimemoldtimemold.com/2021/08/18/a-chemical-hunger-interlude-e-bad-seeds/
and think it's wrong, for roughly the same reasons as Scott, their excellent summary is: "If seed oils cause weight gain when people eat them, why didn’t seed oils cause weight gain when people ate them?" (in studies)
But the idea of a rate-limited path for digesting polyunsaturated fat just won't get out of my head.... Our ancestors can't have eaten much of it.
Replies from: ChristianKl↑ comment by ChristianKl · 2023-01-29T14:09:04.416Z · LW(p) · GW(p)
Why do you believe it's rate limited?
Even if burning it would be rate limited, not everything that's eaten gets metabolized. It can also be simply excreted.
Replies from: johnlawrenceaspden↑ comment by johnlawrenceaspden · 2023-01-29T16:14:08.643Z · LW(p) · GW(p)
Hi Christian! I'm not sure I do believe it's rate-limited, it's more that if it were, that would be extremely interesting. I need a biochemist.
I'm imagining an enzyme going down a fatty acid, chopping carbons off one by one, and then it gets to a double bond and can't cut it, so another enzyme is needed.
No question that we can digest mono-unsaturated fats, people have been eating olive oil in Southern Europe since forever, and I think that wild animals contain mono-unsaturated fats too, so that double-bond-cutter must exist.
But chopping the odd bond in something you're not eating much of is different from chopping lots of bonds in something that's a high proportion of your diet. (PUFAs have lots of double bonds, and make up something like 30% of modern calorie intake!!)
And yes, you could excrete the excess unsaturated fats, but our bodies aren't profligate with energy, and fats are our storage mechanism, so I'm betting they get stored.
And indeed, a lot of human body fat these days is polyunsaturated. And a lot of the fat of food animals which are fed on seed oils.
Also, I'm told that cell walls are made from triglycerides made from fats, and that those fats should be saturated, and that these days they've got polyunsaturated fats in them. Change the structure and properties of cell walls and I bet all hell breaks loose. Some people have told me that cell wall structure isn't affected by PUFA intake, and some people have told me that it is, and I don't know....
As you may remember, I was once very interested in what looks like an epidemic of mysterious type-II hormone disorders..... So two of my idees fixes seem to be colliding here.
Replies from: ChristianKl↑ comment by ChristianKl · 2023-01-30T15:30:57.424Z · LW(p) · GW(p)
Storing fats actually needs the body to transport the fat into a cell. A basically unlimited transport into the cell while at the same time being rate limited to do something with them seems unlikely.
Hi Christian! I'm not sure I do believe it's rate-limited, it's more that if it were, that would be extremely interesting. I need a biochemist.
For this kind of problem, I would recommend starting with asking either ChatGPT or asking the question on a stack exchange website.
When making a hypothesis like that, where the phenomena should be well understood by experts, the best move is to put in work to check whether they are true instead of offering them as an open hypothesis the way you did above.
comment by Nina Panickssery (NinaR) · 2023-01-29T21:45:14.197Z · LW(p) · GW(p)
I wonder how clear it is that increasing average human BMI is bad. It seems very true that being obese is bad for health outcomes, but maybe this is compensated for by a reduction in the number of underweight individuals + better nutrition for non-morbidly-obese people.
comment by Lone Pine (conor-sullivan) · 2023-01-29T05:52:54.395Z · LW(p) · GW(p)
Why can't I eat what my grandparents ate 100 years ago? According to SMTM, this would have been 3 large hearty meals full of bread, potatoes, meat, dairy, too much sugar and not enough vegetables. If I ate like that, not only would I get obese and diabetic, I would get severely sick from the gluten. Perhaps the gluten issues are specific to me, but I see a lot of people having food problems that go beyond metabolic.
Replies from: Natália Mendonça, jkaufman, Rubix, Making_Philosophy_Better↑ comment by Natália (Natália Mendonça) · 2023-01-29T06:20:38.697Z · LW(p) · GW(p)
If I ate like that, not only would I get obese and diabetic
What's the best evidence we have of that, in your opinion?
↑ comment by jefftk (jkaufman) · 2023-01-29T14:09:35.284Z · LW(p) · GW(p)
Are you also proposing long days of highly physical labor like the typical person eating that diet then?
↑ comment by Rubix · 2023-01-29T15:52:40.336Z · LW(p) · GW(p)
One factor is that bread is made sweeter now, dairy is more readily available in skim which is higher sugar content per calorie, and I'm less sure of potatoes but "in the late 1800s, the modern-day russet potato was born" (<https://www.littlepotatoes.com/blog/origin-of-potatoes/>) and I wonder if there's been genetic engineering/selective breeding since then to change them as well.
Food allergies and intolerances are on the rise, I think even controlling for increased recognition (edit: I'm less confident about this than in the original draft of this comment. What would controlling for increased recognition look like?) I don't know what's up with that.
↑ comment by Viliam · 2023-01-29T22:45:33.385Z · LW(p) · GW(p)
bread is made sweeter now
Isn't this reverting in recent decades?
I only have anecdotal evidence that a few decades ago, people from Eastern Europe who visited USA complained that it was impossible to buy a non-sweet bread in an ordinary supermarket (if you really wanted some, you had to visit some ethnic food shop), but recently I have heard that ordinary supermarkets now also contain non-sweet bread.
(Low N. Maybe only applies to some parts of USA. Also, it is possible that both are true: supermarkets may have introduced a non-sweet bread as a special option, while increasing the amount of sugar in the average bread.)
Replies from: SaidAchmiz↑ comment by Said Achmiz (SaidAchmiz) · 2023-01-29T23:13:28.344Z · LW(p) · GW(p)
Here in New York City, it is trivially easy to buy all sorts of non-sweet bread in pretty much any supermarket or grocery store. (Not necessarily in bodegas, though.)
Replies from: Making_Philosophy_Better↑ comment by Portia (Making_Philosophy_Better) · 2023-04-09T16:42:09.416Z · LW(p) · GW(p)
In Germany, with the exception of things like raisin bread, which is more like a cake, really, sugar simply does not go in bread, period. (And I personally make my raisin bread without, after all, it already has raisins in it.) Maybe a third of a teaspoon to start the yeast, which then gets eaten up entirely, but that is that. It isn't that it is possible to avoid it, it is that is seems ludicrous to find it. I remember the first time I had American bread and being deeply weirded out because it tasted sweet at all. It was as odd as being told that, I don't know, Americans add vanilla or cinnamon to all of their bread. It is just weird and does not belong there.
And not just bread, there are so many dishes where Americans add sugar and it is just weird to me. Pickles should not contain sugar; they should taste sour from the natural fermentation, not sweet. Ketchup does not need added sugar; the tomatoes are already sweet. Juice does not need added sugar, it is incredibly sweet and should be diluted heavily with water before drinking. Balsamico vinegar does not need added sugar, it already contains grape must. Bitter chocolate should not contain sugar; it is supposed to be bitter, and for the sweetness, you have cocoa butter and vanilla.
And even in dishes where I would add sugar (or rather, sweetener) myself, the sheer quantity in American dishes is baffling to me. Be it lemonade or cake or cookies, I find American dishes almost sickly sweet. I once got an American baking book, and eventually realised I loved all the recipes if I literally halved the sugar, but found them inedible beforehand. Not just a health concern either, there are protein bars or protein shakes or meal replacement shakes or diet candy things which use calorie free sugar replacements, and I still find them ghastly sweet.
Horribly, these changes are beginning to drift over to Europe, and people are getting used to them. Glad we are countering it with a sugar tax, highlighting it in the nutrient index, and forcing people to declare added sugars.
Replies from: SaidAchmiz↑ comment by Said Achmiz (SaidAchmiz) · 2023-04-09T18:46:16.554Z · LW(p) · GW(p)
Juice, as far as I know, does not tend to have sugar added to it. Where have you seen this?
The history of American ketchup, and the chain of events that led to it ending up with the ingredients that it has—including its sugar content—is fascinating, in fact. (As usual, it’s as much a story of technology, economics, and regulatory politics as it is of culinary art.)
As for chocolate: cocoa butter and vanilla are not sweet. (And chocolate-containing foods definitely need not include vanilla!)
Furthermore, chocolate tends to be labeled “bittersweet” (and not just “bitter”), as well as “semi-sweet” for a reason. There are also different grades of it. “Unsweetened” chocolate exists as well, and is available here along with the other sorts.
All of these forms of chocolate have their distinct culinary uses; they are not seamlessly replaceable with one another. You can do substitutions, but only to an extent; and recipe adjustments are needed in such cases.
One thing that few people realize (and this applies to much more than just chocolate) is that sugar plays a greater role in baking chemistry than merely providing the gustatory experience of sweetness; it also affects texture, rise, moistness, etc. In the case of almost all baked goods, you cannot simply adjust the amount of chocolate without changing more than just how sweet the final product is. (Even baking times may be affected by sugar content!)
And even in dishes where I would add sugar (or rather, sweetener) myself, the sheer quantity in American dishes is baffling to me. Be it lemonade or cake or cookies, I find American dishes almost sickly sweet.
This is very true.
I once got an American baking book, and eventually realised I loved all the recipes if I literally halved the sugar, but found them inedible beforehand.
This varies considerably between cookbook authors. (I have noted in particular that, e.g., Stella Parks tends to overload her recipes with sugar—indeed, often ending up with double what I’d find reasonable—while Christopher Kimball’s recipes have sugar amounts that tend to be almost exactly what I’d find ideal. I suspect this has something to do with their backgrounds—Parks is from Kentucky, while Kimball is from New York / Vermont—and hints at a general pattern of regional variation, within the United States, in how much sugar tends to be added to foods of all sorts.)
↑ comment by dirk (abandon) · 2023-08-13T19:23:47.818Z · LW(p) · GW(p)
I will note that making your own bread at home is not hugely difficult if that's your priority; it does take several hours per loaf, but much of that time is spent doing other things while you wait for it to rise, and if you knead the dough by hand you don't need any expensive equipment. (The ingredients can generally be found in any grocery store, though you may need to go further afield if you're trying to precisely replicate a grandparent's recipe).
↑ comment by Portia (Making_Philosophy_Better) · 2023-04-09T16:31:29.511Z · LW(p) · GW(p)
I do wonder if this is true when controlling for increased recognition. Don't find it per se implausible (there are plausible environmental causes, and one could also imagine genetic selection), but haven't seen evidence yet.
E.g. celiac absolutely existed historically, even though we did not know what was causing it. Those poor kids simply got fed wheat daily anyway, as that was simply what one ate, and died. The study that finally trialed various food plans including one without wheat and had the kids in that group recover is famous for the fact that they stopped it and switched all the kids to that food plan when they were only part-way through, because it became so clear that they could finally, finally stop the deaths.
With a lot of peanut and soy allergies; your average European would simply not have had the exposure.
And as for other severe allergies... again, we are talking a society with no epipens, no allergy declarations. Avoiding a food, especially traces for it, when the average product you buy does not even have an ingredient list, let alone safe handling... Where the allergies were severe, those children died, and often, the parents could not even piece together why. Some thought their kids had been exchanged by fairies, because they were always crying, vomiting, choking, having diarrhoea, having skin discolouration, not wanting to eat, shying away. Doctors noticed that they "failed to thrive", which essentially meant they were thin and sickly and small and constantly ill until pneumonia got them.
↑ comment by Portia (Making_Philosophy_Better) · 2023-04-09T16:21:14.333Z · LW(p) · GW(p)
Weight wise:
Because they did not actually eat that much, and they moved a lot more, and lived in colder buildings, meaning they burned more calories.
Your metabolism is fine.
Seriously. If you put humans in a controlled context and actually weigh their food, the metabolic differences between them are minor, and easily outdieted. Their weight gain and loss is predictable. There are literally no adult humans that can eat only 500 calories a day and gain fat (toddlers already need more); practically no humans that can eat 1000 calories a day and gain weight (this can happen, but you would have a severe, apparent medical condition causing significant other issues); and only few adult humans that do not lose weight at 1500 (that one you can pull of as a small, sedentary, older female). They cannot magically acquire energy out of thin air.
Underweight people overreport their food intake. Overweight people underreport it. Any study working on self-reports is absolute garbage. Any historical comparison based on self-reports is garbage.
Where people measured, the results became very consistent. There are horrid examples of this. Germans tracked how much food they gave Jewish prisoners, and when they would stop working, and when they would starve to death, to get optimum value out of the horror they inflicted. The results were horrific, but not at all surprising or strange, but rather, very consistent. The jews were starved; they became normal weight, while their metabolism barely slowed; then under weight, and their metabolism slowed significantly, with visible massive health consequences, yet still unable to outweigh the dieting; then they died.
On the other hand, you can take your skinny fit European, and put them in a US community where they can't walk, can't cycle, and keep being offered giant portions of highly caloric food, and the same person with the same genes and same willpower and same baseline metabolism will gain weight, at the speed you would expect for the extra calories they consume and the reduction in calories they burn.
Well-being and health wise:
If you have a population whose diet is obviously nutrient deprived and high glycemic index, check their body height, their average age of death, their teeth, and their cognition. You will find that they do not do optimally. I think some people just lack a frame of reference for how they would feel if they ate well.
comment by Lorec · 2024-08-22T12:40:18.124Z · LW(p) · GW(p)
[ Sorry about the wrecked formatting in this comment, I'm on mobile and may come back and fix it later ]
They call it "burning" calories because it's oxidation. Like fire. More oxygen should help. Less oxygen should hurt.*
At least, if you buy CICO and correspondingly think that quantity food oxidation versus quantity fat oxidation is almost all that matters, metabolically, and know that medically, according to all CICO-compatible convention, quantity food oxidation is almost quota-ed at the level of food intake, while quantity fat oxidation is not. [ Hence why "food intake" is not considered a dependent variable in the CICO equation [ daily weight delta = 3600*[ food intake ] - [ RMR + exercise ] ] ].
Yet you, Scott, SMTM, and several others I've spoken with, who otherwise vastly disagree on obesity science [but most of whom say CICO is "basically true" or "trivially true"] independently think the "low O2 mediates the altitude effect" idea is plausible.
I even independently generated it myself, once, in 2021, back when I was still a CICO believer, before realizing 2 years later that, according to CICO, "low O2 results in weight loss" doesn't actually make any physiological sense.
I think people intuitively feel like it makes sense because marginally suffocating feels bad, and most other things that make you lose weight according to CICO [caloric restriction, forcing yourself to exercise, wearing fewer clothes so you shiver a bit in the cold] feel bad.
But many things that feel bad, don't make you lose weight. Like back problems. Or cancer. Or gaining weight.
And drinking unflavored olive oil in the middle of a fast period [ https://www.lesswrong.com/posts/BD4oExxQguTgpESd [? · GW] ] makes me lose more weight than anything else I've tried, and it doesn't feel bad at all. Keto also works for many people, who say it doesn't feel bad for them.
My intention pointing these things out is not to infantilize or indemn you or anyone else who's had the "low O2 mediates the altitude effect" idea and accepted it without noticing that it went against CICO.
My intention is to help create common knowledge of just how fucked the fact that people keep coming up with that hypothesis, and uncritically running with it, proves the discourse around this topic, and the CICO paradigm specifically, is.
*It's true that it's also "burning" calories when it's not fat calories, it's the calories in your food - such that less oxygen could conceivably hurt the process of gaining weight, like less food could. But the conventional wisdom is that the body treats eaten food as an ~absolute lower bar for "energy" intake quota - ie acts as though it should always oxidize all eaten food and turn it into glycogen, fat, ATP, or heat, no matter how inefficient this is - while the amount of oxidation the body does per hour at rest actually is a dependent variable that could conceivably vary closely with amount of O2 in the air. Medically, it would fly in the face of a lot, if people were actually doing less oxidation of eaten food, rather than just using more oxygen per food molecule, under hypoxic conditions. Conceivably, this decrease in efficiency of metabolism, to meet the oxidation quota, correspondingly slows fat gain - and I think it maaaaaybe does, and I think this is an actual plausible mechanism here. That's something that also, empirically, happens in severe caloric restriction - but importantly for CICO, it's not something that happens, at all, in moderate caloric restriction, of the "cut 200 calories per day" stripe that CICOists suggest to lose weight. And moderate caloric restriction is also sometimes effective, simply by reducing the quantity of "energy" intake, just like CICOists say it should. My point is to say that CICOists' favored method of "reduce the quantity of 'energy' intake", can hardly medically be what chronic hypoxia is doing, although CICO would lead us to expect the opposite effect, of "less body fat is ~passively oxidized", at rest, because the body just cannot do as much metabolism per hour-calorie [as opposed to per calorie eaten] when there is less O2 to work with.
After re-looking at the graph because of this post, I'm surprised by how exactly overweight does correspond with low altitude, and not anything about water tables, as I originally thought. And I do find "hypoxia makes food oxidation [and baseline-necessary homeostatic oxidation] less efficient, in the same way severe CR does" plausible as a mechanism. It's making me question my intitial conviction that the Thing Causing Lipostat Damage Since 1900 necessarily had to be some kind of 1900-era waterborne endocrine disruptor like a heavy metal, and putting other, weirder stuff, like food-borne toxins, and viruses, back on the table.
But from the perspective of someone who's seen the old rat studies [https://www.ncbi.nlm.nih.gov/pmc/articles/PMC1225664/] and the old nutrition tables [ https://ageconsearch.umn.edu/nanna/record/136596/files/fris-1961-02-02-438.pdf?withWatermark=0&withMetadata=0&version=1®isterDownload=1, https://tinyurl.com/jnzxkk5z, https://ageconsearch.umn.edu/nanna/record/234624/files/frvol25i3a.pdf?withWatermark=0&withMetadata=0&version=1®isterDownload=1 ] and knows that calories don't explain it, "The SMTM overweight-altitude pattern is in fact downstream of relative hypoxia" deconfuses somewhat about the SMTM overweight-altitude pattern, but doesn't deconfuse at all about why we've all been getting fatter since 1900 in the first place. From the perspective of someone who knows calories can't explain it, the relatively lower rate of obesity in China [even if, surprisingly-to-me, only by a few percentage points - aroaund 35% for the US vs around 31% for China, according to the first Google result I saw], which stands at an overall much lower elevation than the US [especially populated areas], looks more potentially fruitful as an area of investigation. And . . . it looks like in China, there's a regional gradient in obesity [higher in the north] that seems obviously not to be tracking altitude at all. And what about Korea, which is basically on the sea? They sandbag and say 40% obesity in self-reporting [to alarm the locals?], but when measured the same as the US [ https://www.koreaherald.com/view.php?ud=20230425000613 ], their "obesity" rate is around 6% compared to the US's 35%-40%. It makes me suspect the China thing is a reporting issue, too. Altitude clearly isn't most of the inter-regional variance worldwide.
comment by Review Bot · 2024-02-22T17:59:13.675Z · LW(p) · GW(p)
The LessWrong Review [? · GW] runs every year to select the posts that have most stood the test of time. This post is not yet eligible for review, but will be at the end of 2024. The top fifty or so posts are featured prominently on the site throughout the year.
Hopefully, the review is better than karma at judging enduring value. If we have accurate prediction markets on the review results, maybe we can have better incentives on LessWrong today. Will this post make the top fifty?
comment by Susan Fergusson (susan-fergusson) · 2024-01-07T05:39:53.145Z · LW(p) · GW(p)
Excellent paper, well nuanced. Now do antibiotics use in food animals.
comment by JasonW501 (Kenmont1) · 2023-07-28T22:30:08.544Z · LW(p) · GW(p)
This and your post on lithium are thoroughly convincing wrt A Chemical Hunger's lithium hypothesis, and some of their other points.
You seem to acknowledge, however, that CICO doesn't explain the rise in obesity, and for any number of reasons, diet and exercise seem to be largely insufficient explanations. (Correct me if I've misunderstood.) You also seem to believe, in line with prediction markets, that environmental contamination isn't the most likely culprit (or at least the most likely primary culprit).
Given that, what is the most likely explanation, or, if we don't have one yet, what is the most promising line of research?
comment by frankybegs · 2023-01-29T03:41:29.287Z · LW(p) · GW(p)
I'm not sure what is achieved by the tone of this piece. It's bordering on uncivil.
Especially since, as others comments have pointed out, you make more of your objections than is really warranted. There are some excellent points in there, but also some points where you needlessly exaggerated the difference between your beliefs.
Aren't we supposed to be learning together? Why the adversarial approach?
Replies from: Throwaway2367↑ comment by Throwaway2367 · 2023-01-29T15:30:36.683Z · LW(p) · GW(p)
Can someone tell me why they downvoted this comment? Do you disagree with the observation that the tone is adversarial, just generally dislike tone policing or something else entirely?
(For the record I strong upvoted both the post and this comment.)
Replies from: Kaj_Sotala, matthew-barnett↑ comment by Kaj_Sotala · 2023-01-29T16:17:10.826Z · LW(p) · GW(p)
I didn't downvote it, but I do disagree with the claim that the tone is particularly adversarial. I read the post as mostly just citing SMTM's claims and then laying out the evidence that disagrees with those claims. I think it does a good job of just sticking to the facts of the disagreement rather than getting personal or rude.
Replies from: Throwaway2367↑ comment by Throwaway2367 · 2023-01-29T17:58:32.533Z · LW(p) · GW(p)
Thank you!
↑ comment by Matthew Barnett (matthew-barnett) · 2023-01-29T23:17:43.678Z · LW(p) · GW(p)
I didn't downvote, but I think the comment would have benefitted from specific commentary about which parts were uncivil. There's a lot of stuff in the post, and most of it has pretty neutral language.
comment by Sune · 2023-01-28T23:22:52.555Z · LW(p) · GW(p)
Im confused by the first two diagrams in the section called: “There wasn’t an abrupt shift in obesity rates in the late 20th century”. As far as I understand, they contain data about the distribution of BMI for black female and white males at age 50 and born up until 1986. If so, it would contain data from 2036.
Replies from: Natália Mendonça↑ comment by Natália (Natália Mendonça) · 2023-01-28T23:38:08.910Z · LW(p) · GW(p)
These lines are the output of a statistical model, based on cohort- and age-associated changes in BMI observed in NCHS data collected between 1959 and 2006. I edited the post to make that clearer.