2019 Review: Voting Results!
post by Raemon, habryka (habryka4) · 2021-02-01T03:10:19.284Z · LW · GW · 36 commentsContents
Top Results Top Reviewers Complete Results (1000+ Karma) What does this mean, and what happens now? None 36 comments
You can see the full voting results here: 1000+ karma voters (All voters)
The 2019 Review votes are in!
This year, 88 voters participated, evaluating 116 posts. (Of those voters, 61 had 1000+ karma, and will be weighted more highly in the moderation team's decision of what to include in the Best of 2019 Books)
The LessWrong Moderation team will be reflecting on these results and using them as a major input into "what to include in the 2019 books."
Top Results
The top 15 results from the 1000+ karma users are:
- What failure looks like [? · GW] by Paul Christiano
- Risks from Learned Optimization: Introduction [? · GW], by evhub, Chris van Merwijk, vlad_m, Joar Skalse and Scott Garrabrant
- The Parable of Predict-O-Matic [? · GW], by Abram Demski
- Book Review: The Secret Of Our Success [? · GW], by Scott Alexander
- Being the (Pareto) Best in the World [? · GW], by Johnswentworth
- Rule Thinkers In, Not Out [? · GW], by Scott Alexander
- Book summary: Unlocking the Emotional Brain [? · GW], by Kaj Sotala
- Asymmetric Justice [? · GW], by Zvi Mowshowitz
- Heads I Win, Tails?—Never Heard of Her; Or, Selective Reporting and the Tragedy of the Green Rationalists [? · GW], by Zack Davis
- 1960: The Year The Singularity Was Cancelled [? · GW], by Scott Alexander
- Selection vs Control [? · GW], by Abram Demski
- You Have About Five Words [LW · GW], by Raymond Arnold
- The Schelling Choice is "Rabbit", not "Stag" [? · GW], by Raymond Arnold
- Noticing Frame Differences [? · GW], by Raymond Arnold
- "Yes Requires the Possibility of No" [? · GW], by Scott Garrabrant
Top Reviewers
Meanwhile, we also had a lot of great reviews. One of the most valuable things I found about the review process was that it looks at lots of great posts at once, which led me to find connections between them I had previously missed. We'll be doing a more in-depth review of the best reviews later on, but for now, I wanted to shoutout to the people who did a bunch of great review work.
The top reviewers (aggregating the total karma of their review-comments) were:
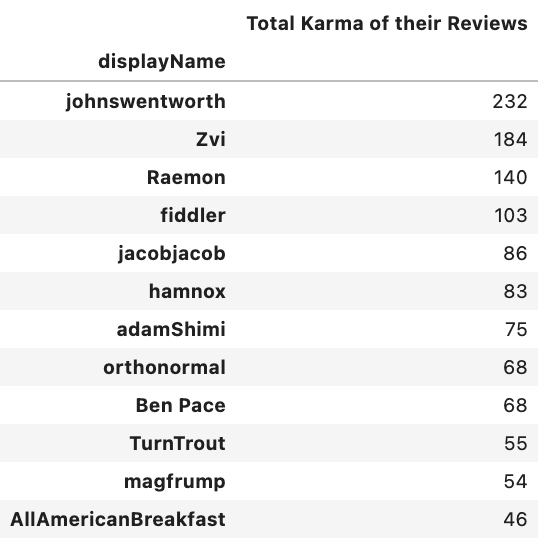
Some things I particularly appreciated were:
- johnswentworth, Zvi and others providing fairly comprehensive reviews of many different posts, taking stock of how some posts fit together.
- Jacobjacob and magfrump who stuck out in my mind for doing particularly "epistemic spot check" type reviews, which are often more effortful.
Complete Results (1000+ Karma)
You can see the full voting results here: 1000+ karma voters (All voters)
To help users see the spread of the vote data, we've included swarmplot visualizations.
- Only votes with weights between -10 and 16 are plotted. Outliers are in the image captions.
- Gridlines are spaced 2 points apart.
- Concrete illustration: The plot immediately below has 18 votes ranging in strength from -3 to 12.
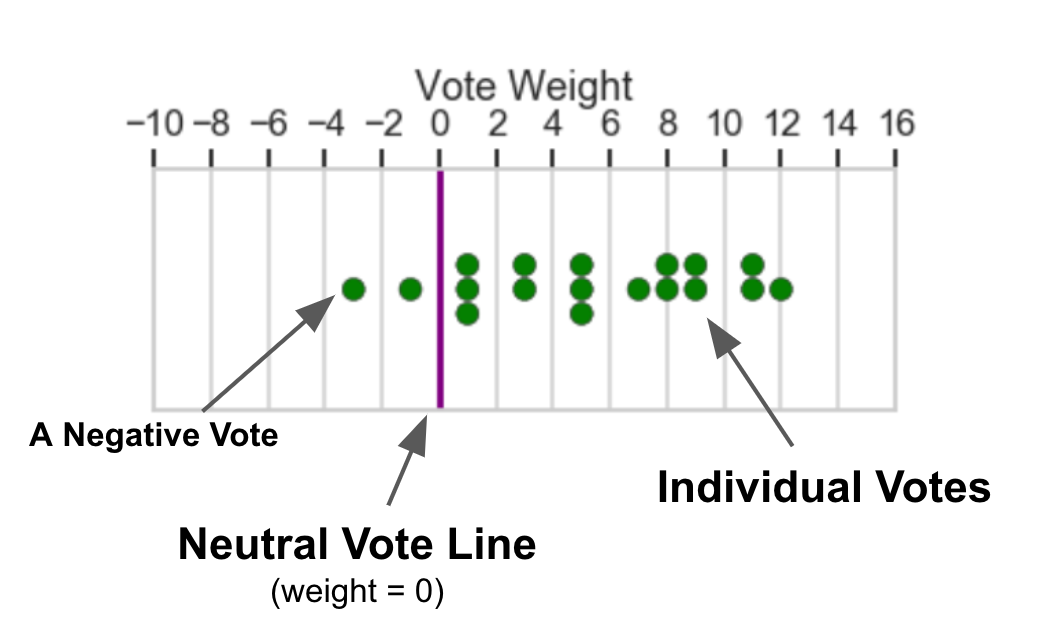
What does this mean, and what happens now?
(This section written by habryka, previous section written by Ray)
The goals of this review and vote were as follows:
- Create common knowledge about how the LessWrong community feels about various posts and the progress we've made.
- Improve our longterm incentives, feedback, and rewards for authors.
- Help create a highly curated "Best of 2019" Sequence and Book.
Over the next few months we will take the results of this vote and make it into another curated collection of essays, just as we did with last years results, which turned into the "A Map that Reflects the Territory" essay collection [? · GW].
Voting, review and nomination participation was substantially greater this year than last year (something between a 30% and 80% increase, depending on which metrics you look at), which makes me hopeful about this tradition living on as a core piece of infrastructure for LessWrong. I was worried that participation would fall off after the initial excitement of last year, but I am no longer as worried about that.
Both this year and last year we have also seen little correlation with the vote results and the karma of the posts, which is an important sanity check I have for whether going through all the effort of this review is worth it. If the ranking was basically just the same as the karma scores of the post, then we wouldn't be getting much information out of the review. But as it stands, I trust the results of this review much more than I would trust someone just pressing the "sort by karma" button on the all-posts page, and I think as the site and community continues to grow, the importance of the robustness of the review will only grow.
Thank you all for participating in this year's review. I am pleased with results, and brimming with ideas for the new set of books that I am looking forward to implementing, and I think the above is already a valuable resource if someone wants to decide how to best catch up with all the great writing here on the site.
36 comments
Comments sorted by top scores.
comment by Rob Bensinger (RobbBB) · 2021-02-01T23:06:06.654Z · LW(p) · GW(p)
I'm sad (and surprised) to see Thoughts on Human Models [LW · GW] so far down the list! I think this is one of the ~five most important LW posts written in the last five years for AI safety and ML people to think about and discuss.
Replies from: Scott Garrabrant↑ comment by Scott Garrabrant · 2021-02-03T00:40:08.129Z · LW(p) · GW(p)
Yeah, I am sad, but not surprised, because I have been trying to push this idea (e.g. at conferences) for a few years.
Guesses as to why I'm failing?
I think that we actually undersold the neglectedness point in this post, but I don't think that is the main problem, I think the main problem is that the post (and I) do not give viable alternatives, its like:
"Have you noticed that the CHAI ontology, the Paul ontology, and basically all the concrete plans for safe AGI are trying to get safety out of superhuman models of humans, and there are plausible worlds in which this is on net actively harmful for safety. Further, the few exceptions to this mostly involve AGI interacting directly with the world agentically in such a way as to create an instrumental incentive for human modeling."
"Okay, what do we do instead?"
*shrug*
Perhaps it goes better if I gave any concrete plan at all, even if it is unrealistic like:
1. Understand agency/intelligence/optimization/corrigibility to the point where we could do something good and safe if we had unlimited compute, and maybe reliable brain scans.
2. (in parallel) Build safe enough AGI that can do science/engineering to the point of being able to generate plans to turn Jupiter into a computer, without relying on human models at all.
3. Turn Jupiter into a computer.
4. Do the good and safe thing on the Jupiter computer, or if no better ideas are found, run a literal HCH on the Jupiter computer.
The problem is that draws attention to how unrealistic this plan is, and not on the open question of "What *do* we do instead?"
Replies from: Unnamed, rohinmshah, Raemon↑ comment by Unnamed · 2021-02-03T04:58:46.800Z · LW(p) · GW(p)
For the 2019 Review, I think it would've helped if you/Rob/others had posted something like this as reviews of the post. Then voters would at least see that you had this take, and maybe people who disagree would've replied there which could've led to some of this getting hashed out in the comments.
Replies from: RobbBB↑ comment by Rob Bensinger (RobbBB) · 2021-02-03T17:36:43.252Z · LW(p) · GW(p)
I agree. In retrospect, I think I avoided doing this for a couple reasons:
- I didn't have much time to spend on the review process, and it was hard for me to know how to prioritize my time without knowing vote totals, number of voters, etc. E.g., I also would have been sad if "Risks from Learned Optimization" hadn't made the cut, and I could imagine worlds where this had happened too (say, if everyone reasoned "this post will definitely make the cut, so there's no need for me personally to put a bunch of votes into it").
- I felt like there was something uncouth about leaving a review that basically just says "I think this post deserves n votes!", without giving detailed reasoning like many other reviewers were doing.
- I'm co-workers with Scott (one of the authors of Thoughts on Human Models [LW · GW]), and I'm not an alignment researcher myself. So I felt like absent detailed supporting arguments, my merely saying "I think this is one of the most valuable alignment posts to date" ought to carry little weight.
Point 2 seems wrong to me now. Points 1 and 3 seem right, but I think they deserved less weight in my deliberation than "just saying something quick and pithy might spark a larger conversation about the post, resulting in others writing better reviews; and it might also encourage more people to read it who initially skipped over it".
Encouraging more discussion of 'is human modeling a good idea in the first AGI systems? what might alignment and AI research look like if it didn't rely on human models?' is the thing I care about anyway (hence my bringing it up now). This would have felt much less like a missed opportunity if the review process had involved a lot of argument about human models followed by the community going 'we reviewed the arguments back and forth and currently find them uncompelling/unimportant enough that this doesn't seem to warrant inclusion in the review'.
That would make me more confident that the ideas had gotten a good hearing, and it would likely make it clearer where the conversation should go next.
↑ comment by Rohin Shah (rohinmshah) · 2021-02-03T22:17:46.058Z · LW(p) · GW(p)
I certainly would be more excited if there was an alternative in mind -- it seems pretty unlikely [LW(p) · GW(p)] to me that this is at all tractable.
However, I am also pretty unconvinced by the object-level arguments that there are risks from using human models that are comparable to the risks from AI alignment overall (under a total view, longtermist perspective). Taking the arguments from the post:
- Less Independent Audits: I agree that all else equal, having fewer independent audits increases x-risk from AI alignment failure. This seems like a far smaller effect than from getting the scheme right in the first place so that your AI system is more likely to be aligned.
I also think the AI system must have at least some information about humans for this to be a reasonable audit: if your AI system does something in accordance with our preferences (which is what the audit checks for), then it has information about human preferences, which is at least somewhat of a human model; in particular many of the other risks mentioned in the post would apply. This makes the "independent audits" point weaker. - Incorrectly Encoded Values: I don't find the model of "we build a general-purpose optimizer and then slot in a mathematical description of the utility function that we can never correct" at all compelling; and that seems like the only model anyone's mentioned where this sort of mistake seems like it could be very bad.
- Manipulation: I agree with this point.
- Threats: This seems to be in direct conflict with alignment -- roughly speaking, either your AI system is aligned with you and can be threatened, or it is not aligned with you and then threats against it don't hurt you. Given that choice, I definitely prefer alignment.
- Mind Crime: This makes sense to worry about if you think that most likely we will fail at alignment, but if you think that we will probably succeed at alignment it doesn't seem that important -- even if mind crime exists for a while, we would presumably eliminate it fairly quickly. (Leaning heavily on the longtermist total view here.)
- Unexpected Agents: I agree with the post that the likelihood of this mattering is small. (I also don't agree that a system that predicts human preferences seems strictly more likely to run into problems with misaligned subagents.)
More broadly, I wish that posts like this would make a full case for expected impact, rather than gesturing vaguely at ways things could go poorly.
Replies from: Scott Garrabrant, elityre↑ comment by Scott Garrabrant · 2021-02-04T07:39:19.234Z · LW(p) · GW(p)
Yeah, looking at this again, I notice that the post probably failed to communicate my true reason. I think my true reason is something like:
I think that drawing a boundary around good and bad behavior is very hard. Luckily, we don't have to draw a boundary between good and bad behavior, we need to draw a boundary that has bad behavior on the outside, and *enough* good behavior on the inside to bootstrap something that can get us through the X-risk. Any distinction between good and bad behavior with any nuance seems very hard to me. However the boundary of "just think about how to make (better transistors and scanning tech and quantum computers or whatever) and don't even start thinking about (humans or agency or the world or whatever)" seems like it might be carving reality at the joints enough for us to be able to watch a system that is much stronger than us and know that it is not misbehaving.
i.e., I think my true reason is not that all reasoning about humans is dangerous, but that it seems very difficult to separate out safe reasoning about humans from dangerous reasoning about humans, and it seems more possible to separate out dangerous reasoning about humans from some sufficiently powerful subset of safe reasoning (but it seems likely that that subset needs to have humans on the outside).
Replies from: Scott Garrabrant, elityre↑ comment by Scott Garrabrant · 2021-02-04T07:40:58.322Z · LW(p) · GW(p)
(Where by "my true reason", I mean what feels live to me right now, There is also all the other stuff from the post, and the neglectedness argument)
Replies from: rohinmshah↑ comment by Rohin Shah (rohinmshah) · 2021-02-04T20:59:59.367Z · LW(p) · GW(p)
I'm on board with "let's try to avoid giving models strong incentives to learn how to manipulate humans" and stuff like that, e.g. via coordination to use AI primarily for (say) science and manufacturing, and not for (say) marketing and recommendation engines.
I don't see this as particularly opposed to methods like iterated amplification or debate, which seem like they can be applied to all sorts of different tasks, whether or not they incentivize manipulation of humans.
It feels like the crux is going to be in our picture of how AGI works, though I don't know how.
Replies from: Scott Garrabrant, Scott Garrabrant↑ comment by Scott Garrabrant · 2021-02-04T22:06:28.702Z · LW(p) · GW(p)
I am having a hard time generating any ontology that says:
I don't see [let's try to avoid giving models strong incentives to learn how to manipulate humans] as particularly opposed to methods like iterated amplification or debate.
Here are some guesses:
You are distinguishing between an incentive to manipulate real life humans and an incentive to manipulate human models?
You are claiming that the point of e.g. debate is that when you do it right there is no incentive to manipulate?
You are focusing on the task/output of the system, and internal incentives to learn how to manipulate don't count?
These are just guesses.
Replies from: rohinmshah↑ comment by Rohin Shah (rohinmshah) · 2021-02-05T05:15:07.995Z · LW(p) · GW(p)
You are focusing on the task/output of the system, and internal incentives to learn how to manipulate don't count?
This seems closest, though I'm not saying that internal incentives don't count -- I don't see what these incentives even are (or, I maybe see them in the superintelligent utility maximizer model, but not in other models).
Do you agree that the agents in Supervising strong learners by amplifying weak experts don't have an incentive to manipulate the automated decomposition strategies?
If yes, then if we now change to a human giving literally identical feedback, do you agree that then nothing would change (i.e. the resulting agent would not have an incentive to manipulate the human)?
If yes, then what's the difference between that scenario and one where there are internal incentives to manipulate the human?
Possibly you say no to the first question because of wireheading-style concerns; if so my followup question would probably be something like "why doesn't this apply to any system trained from a feedback signal, whether human-generated or automated?" (Though that's from a curious-about-your-beliefs perspective. On my beliefs I mostly reject wireheading as a specific thing to be worried about, and think of it as a non-special instance of a broader class of failures.)
Replies from: Scott Garrabrant↑ comment by Scott Garrabrant · 2021-02-05T07:22:10.144Z · LW(p) · GW(p)
Unedited stream of thought:
Before trying to answer the question, I'm just gonna say a bunch of things that might not make sense (either because I am being unclear or being stupid).
So, I think the debate example is much more *about* manipulation, than the iterated amplification example, so I was largely replying to the class that includes IA and debate, I can imagine saying that Iterated amplification done right does not provide an incentive to manipulate the human.
I think that a process that was optimizing directly for finding a fixed point of does have an incentive to manipulate the human, however this is not exactly what IA is doing, because it is only having the gradients pass through the first in the fixed point equation, and I can imagine arguing that the incentive to manipulate comes from having the gradient pass through the second . If you iterate enough times, I think you might effectively have some optimization juice passing through modifying the second , but it might be much less. I am confused about how to think about optimization towards a moving target being different from optimization towards finding a fixed point.
I think that even if you only look at the effect of following the gradients coming from the effect of changing the first , you are at least providing an incentive to predict the human on a wide range of inputs. In some cases, your range of inputs might be such there isn't actually information about the human in the answers, which I think is where you are trying to get with the automated decomposition strategies. If humans have some innate ability to imitate some non-human process, and use that ability to answer the questions, and thinking about humans does not aid in thinking about that non-human process, I agree that you are not providing any incentive to think about the humans. However, it feels like a lot has to go right for that to work.
On the other hand, maybe we just think it is okay to predict, but not manipulate, the humans, while they are answering questions with a lot of common information about humans' work, which is what I think IA is supposed to be doing. In this case, even if I were to say that there is no incentive to "manipulate the human", I still argue that there is "incentive to learn how to manipulate the human," because predicting the human (on a wide range of inputs) is a very similar task to manipulating the human.
Okay, now I'll try to answer the question. I don't understand the question. I assume you are talking about incentive to manipulate in the simple examples with permutations etc in the experiments. I think there is no ability to manipulate those processes, and thus no gradient signal towards manipulation of the automated process. I still feel like there is some weird counterfactual incentive to manipulate the process, but I don't know how to say what that means, and I agree that it does not affect what actually happens in the system.
I agree that changing to a human will not change anything (except via also adding the change where the system is told (or can deduce) that it is interacting with the human, and thus ignores the gradient signal, to do some treacherous turn). Anyway, in these worlds, we likely already lost, and I am not focusing on them. I think the short answer to your question is in practice no, there is no difference, and there isn't even incentive to predict humans in strong generality, much less manipulate them, but that is because the examples are simple and not trying to have common information with how humans work.
I think that there are two paths to go down of crux opportunities for me here, and I'm sure we could find more: 1) being convinced that there is not an incentive to predict humans in generality (predicting humans only when they are very strictly following a non-humanlike algorithm doesn't count as predicting humans in generality), or 2) being convinced that this incentive to predict the humans is sufficiently far from incentive to manipulate.
Replies from: rohinmshah↑ comment by Rohin Shah (rohinmshah) · 2021-02-05T19:01:43.110Z · LW(p) · GW(p)
Yeah, I agree debate seems less obvious. I guess I'm more interested in the iterated amplification claim since it seems like you do see iterated amplification as opposed to "avoiding manipulation" or "making a clean distinction between good and bad reasoning", and that feels confusing to me. (Whereas with debate I can see the arguments for debate incentivizing manipulation, and I don't think they're obviously wrong, or obviously correct.)
I still feel like there is some weird counterfactual incentive to manipulate the process
Yeah, this argument makes sense to me, though I question how much such incentives matter in practice. If we include incentives like this, then I'm saying "I think the incentives a) arise for any situation and b) don't matter in practice, since they never get invoked during training". (Not just for the automated decomposition example; I think similar arguments apply less strongly to situations involving actual humans.)
there isn't even incentive to predict humans in strong generality, much less manipulate them, but that is because the examples are simple and not trying to have common information with how humans work.
Agreed.
1) being convinced that there is not an incentive to predict humans in generality (predicting humans only when they are very strictly following a non-humanlike algorithm doesn't count as predicting humans in generality), or 2) being convinced that this incentive to predict the humans is sufficiently far from incentive to manipulate.
I'm not claiming (1) in full generality. I'm claiming that there's a spectrum of how much incentive there is to predict humans in generality. On one end we have the automated examples I mentioned above, and on the other end we have sales and marketing. It seems like where we are on this spectrum is primarily dependent on the task and the way you structure your reasoning. If you're just training your AI system on making better transistors, then it seems like even if there's a human in the loop your AI system is primarily going to be learning about transistors (or possibly about how to think about transistors in the way that humans think about transistors). Fwiw, I think you can make a similar claim about debate.
If we use iterated amplification to aim for corrigibility, that will probably require the system to learn about agency, though I don't think it obviously has to learn about humans.
I might also be claiming (2), except I don't know what you mean by "sufficiently far". I can understand how prediction behavior is "close" to manipulation behavior (in that many of the skills needed for the first are relevant to the second and vice versa); if that's what you mean then I'm not claiming (2).
If humans have some innate ability to imitate some non-human process, and use that ability to answer the questions, and thinking about humans does not aid in thinking about that non-human process, I agree that you are not providing any incentive to think about the humans. However, it feels like a lot has to go right for that to work.
I'm definitely not claiming that we can do this. But I don't think any approach could possibly meet the standard of "thinking about humans does not aid in the goal"; at the very least there is probably some useful information to be gained in updating on "humans decided to build me", which requires thinking about humans. Which is part of why I prefer thinking about the spectrum.
↑ comment by Scott Garrabrant · 2021-02-04T22:10:36.384Z · LW(p) · GW(p)
BTW, I would be down for something like a facilitated double crux on this topic, possibly in the form of a weekly LW meetup. (But think it would be a mistake to stop talking about it in this thread just to save it for that.)
Replies from: rohinmshah↑ comment by Rohin Shah (rohinmshah) · 2021-02-05T05:15:50.553Z · LW(p) · GW(p)
Yeah, that sounds interesting, I'd participate.
Replies from: habryka4↑ comment by habryka (habryka4) · 2021-02-05T08:55:11.729Z · LW(p) · GW(p)
Seems great! Will ping you both sometime tomorrow or so to see whether we can set it up.
↑ comment by Eli Tyre (elityre) · 2021-02-21T05:12:51.243Z · LW(p) · GW(p)
[Eli's personal notes. Feel free to ignore or engage]
Any distinction between good and bad behavior with any nuance seems very hard to me.
Related to the following, from here [AF · GW].
But if I want to help Bob figure out whether he should vote for Alice---whether voting for Alice would ultimately help create the kind of society he wants---that can’t be done by trial and error. To solve such tasks we need to understand what we are doing and why it will yield good outcomes. We still need to use data in order to improve over time, but we need to understand how to update on new data in order to improve.
Some examples of easy-to-measure vs. hard-to-measure goals:
- Persuading me, vs. helping me figure out what’s true. (Thanks to Wei Dai for making this example crisp.)
- Reducing my feeling of uncertainty, vs. increasing my knowledge about the world.
- Improving my reported life satisfaction, vs. actually helping me live a good life.
- Reducing reported crimes, vs. actually preventing crime.
- Increasing my wealth on paper, vs. increasing my effective control over resources.
. . .
I think my true reason is not that all reasoning about humans is dangerous, but that it seems very difficult to separate out safe reasoning about humans from dangerous reasoning about humans
Thinking further, this is because of something like...the "good" strategies for engaging with humans are continuous with the "bad"strategies for engaging with humans (ie dark arts persuasion is continuous with good communication), but if your AI is only reasoning about a domain that doesn't have humans than deceptive strategies are isolated in strategy space from the other strategies that work (namely, mastering the domain, instead of tricking the judge).
Because of this isolation of deceptive strategies, we can notice them more easily?
↑ comment by Eli Tyre (elityre) · 2021-02-21T04:39:46.905Z · LW(p) · GW(p)
[Eli's notes, that you can ignore or engage with]
- Threats: This seems to be in direct conflict with alignment -- roughly speaking, either your AI system is aligned with you and can be threatened, or it is not aligned with you and then threats against it don't hurt you. Given that choice, I definitely prefer alignment.
Well, it might be the case that a system is aligned but is mistakenly running an exploitable decision theory. I think the idea is we would prefer to have things set up so that failures are contained, ie if your AI is running an exploitable decision theory, that problem doesn't cascade into even worse problems.
I'm not sure if "avoiding human models" actually meets this criterion, but it does seem useful to aim for systems that don't fail catastrophically if you get something wrong.
Replies from: rohinmshah↑ comment by Rohin Shah (rohinmshah) · 2021-02-21T17:24:41.368Z · LW(p) · GW(p)
Well, it might be the case that a system is aligned but is mistakenly running an exploitable decision theory. I think the idea is we would prefer to have things set up so that failures are contained, ie if your AI is running an exploitable decision theory, that problem doesn't cascade into even worse problems.
At least for the neural net approach, I don't think "mistakenly running an exploitable decision theory" is a plausible risk -- there isn't going to be a "decision theory" module that we get right or wrong. I feel like this sort of worry is mistaking the abstractions in our map for the territory. I think it is more likely that there will be a bunch of messy reasoning that doesn't neatly correspond to a single decision theory (just as with humans).
But let's take the least convenient world, in which that messy reasoning could lead to mistakes that we might call "having the wrong decision theory". I think often I would count this as a failure of alignment. Presumably humans want the AI system to be cautious about taking huge impactful decisions in novel situations, and any reasonably intelligent AI system should know this, so giving up the universe to an acausal threatener without consulting humans would count as the AI knowably not doing what we want, aka a failure of alignment. So I continue to stand by my claim.
(In your parlance, I might say "humans have preferences over the AI system's decision theory, so if the AI system uses a flawed decision theory that counts as a failure of alignment". But the reason that it makes sense to carve up the world in this way is because there won't be an explicit decision theory module.)
Fwiw, I expect MIRI people are usually on board with this, e.g. this [LW · GW] seems to have a similar flavor (though it doesn't literally say the same thing).
↑ comment by Raemon · 2021-02-03T01:20:51.306Z · LW(p) · GW(p)
Quick note that I personally didn't look at the post mostly due to opportunity cost of lots of other posts in the review.
I also think we should maybe run a filter on the votes that only takes AlignmentForum users, and check what the AF consensus on alignment-related posts was. Which may not end up mattering here, but I know I personally avoided voting on a lot of alignment stuff because I didn't feel like I could evaluate them well, and wouldn't be surprised if other people did that as well.
Replies from: Zvi, Unnamed, evhub, mingyuan↑ comment by Unnamed · 2021-02-03T05:02:35.959Z · LW(p) · GW(p)
Seems like a good thing to check in principle, but my guess is it won't make much difference for this or other posts. AI posts got about as many nonzero votes as other posts, and the ranking of posts by avg vote is almost the same as the official ranking by total votes.
↑ comment by evhub · 2021-02-03T04:50:35.042Z · LW(p) · GW(p)
I also think we should maybe run a filter on the votes that only takes AlignmentForum users, and check what the AF consensus on alignment-related posts was.
I would definitely be interested in seeing this. I certainly put a lot of my votes in Thoughts on Human Models, since I agree it's a great post (and also nominated it for the review in the first place).
I know I personally avoided voting on a lot of alignment stuff because I didn't feel like I could evaluate them well, and wouldn't be surprised if other people did that as well.
I wonder if this suggests that you should be using a voting system that averages votes rather than summing them, thereby assigning people who didn't vote on a post the average vote rather than a zero vote.
comment by Viliam · 2021-02-05T18:36:27.169Z · LW(p) · GW(p)
Looking at some articles in the bottom half of the list...
Approval Extraction Advertised as Production -- important insight, but I wish someone would rewrite it at maybe 20% of the original length;
No, it's not The Incentives—it's you -- ¿por qué no los dos? "I was just following incentives" is a more pathetic version of "I was just following orders"; incentives explain why someone will predictably steal an unguarded purse, but your character explains why it was you who volunteered for the task;
Instant stone (just add water!) and Turning air into bread -- not typical LW articles, but I liked how they describe the "mundane magic", and they are relatively short, so high value per line;
What are the open problems in Human Rationality? -- someone should write a compilation of the answers.
comment by jimrandomh · 2021-02-02T07:06:31.885Z · LW(p) · GW(p)
My subjective experience voting on these posts was "aaaaah these are all too good I can't not-upvote any of them". Being eligible to be voted on required two nominations and a review, and apparently the nominators/reviewers were picking from a pretty excellent pool this year. The top posts in this list are really excellent, and the bottom posts are... still really excellent.
Replies from: ryan_bcomment by Zack_M_Davis · 2021-02-01T22:43:07.800Z · LW(p) · GW(p)
If it's easy, any chance we could get a variance (or standard deviation) column on the spreadsheet? (Quadratic voting makes it expensive to create outliers anyway, so throwing away the 50 percent most passionate of voters (as the interquartile range does) is discarding a lot of the actual dispersion signal.)
comment by arxhy · 2021-02-05T18:53:20.213Z · LW(p) · GW(p)
I haven't seen Debate on Instrumental Convergence between LeCun, Russell, Bengio, Zador, and More [? · GW]. Why did it get universally negative votes?
Replies from: TurnTrout↑ comment by TurnTrout · 2021-02-05T19:33:38.234Z · LW(p) · GW(p)
I think that this debate suffers for lack of formal grounding, and I wouldn't dream of introducing someone to these concepts via this debate.
While the debate is clearly historically important, I don't think it belongs in the LessWrong review. I don't think people significantly changed their minds, I don't think that the debate was particularly illuminating, and I don't think it contains the philosophical insight I would expect from a LessWrong review-level essay.
I am surprised that no one gave it a positive vote.
Replies from: Benito↑ comment by Ben Pace (Benito) · 2021-02-05T23:06:03.855Z · LW(p) · GW(p)
I like that I made a post that was unanimously given negative votes in the review. I plan to tell my friends about it.
Replies from: RobbBB↑ comment by Rob Bensinger (RobbBB) · 2021-02-06T02:35:30.769Z · LW(p) · GW(p)
And I nominated it! :P
Replies from: Unnamedcomment by Yoav Ravid · 2021-01-30T21:22:28.379Z · LW(p) · GW(p)
The table formatting is broken (it's really thin instead of spreading to fit the width of the page)
Replies from: habryka4↑ comment by habryka (habryka4) · 2021-01-30T22:52:01.046Z · LW(p) · GW(p)
Can you send a screenshot? It looks fine for me, even on mobile devices.
Replies from: Yoav Ravid↑ comment by Yoav Ravid · 2021-02-01T04:47:24.771Z · LW(p) · GW(p)
Never mind, was fixed after the repost