Debate on Instrumental Convergence between LeCun, Russell, Bengio, Zador, and More
post by Ben Pace (Benito) · 2019-10-04T04:08:49.942Z · LW · GW · 61 commentsContents
Original Post Comment Thread #1 Comment Thread #2 None 61 comments
An actual debate about instrumental convergence, in a public space! Major respect to all involved, especially Yoshua Bengio for great facilitation.
For posterity (i.e. having a good historical archive) and further discussion, I've reproduced the conversation here. I'm happy to make edits at the request of anyone in the discussion who is quoted below. I've improved formatting for clarity and fixed some typos. For people who are not researchers in this area who wish to comment, see the public version of this post here. For people who do work on the relevant areas, please sign up in the top right. It will take a day or so to confirm membership.
Original Post
Yann LeCun: "don't fear the Terminator", a short opinion piece by Tony Zador and me that was just published in Scientific American.
"We dramatically overestimate the threat of an accidental AI takeover, because we tend to conflate intelligence with the drive to achieve dominance. [...] But intelligence per se does not generate the drive for domination, any more than horns do."
https://blogs.scientificamerican.com/observations/dont-fear-the-terminator/
Comment Thread #1
Elliot Olds: Yann, the smart people who are very worried about AI seeking power and ensuring its own survival believe it's a big risk because power and survival are instrumental goals for almost any ultimate goal.
If you give a generally intelligent AI the goal to make as much money in the stock market as possible, it will resist being shut down because that would interfere with tis goal. It would try to become more powerful because then it could make money more effectively. This is the natural consequence of giving a smart agent a goal, unless we do something special to counteract this.
You've often written about how we shouldn't be so worried about AI, but I've never seen you address this point directly.
Stuart Russell: It is trivial to construct a toy MDP in which the agent's only reward comes from fetching the coffee. If, in that MDP, there is another "human" who has some probability, however small, of switching the agent off, and if the agent has available a button that switches off that human, the agent will necessarily press that button as part of the optimal solution for fetching the coffee. No hatred, no desire for power, no built-in emotions, no built-in survival instinct, nothing except the desire to fetch the coffee successfully. This point cannot be addressed because it's a simple mathematical observation.
Comment Thread #2
Yoshua Bengio: Yann, I'd be curious about your response to Stuart Russell's point.
Yann LeCun: You mean, the so-called "instrumental convergence" argument by which "a robot can't fetch you coffee if it's dead. Hence it will develop self-preservation as an instrumental sub-goal."
It might even kill you if you get in the way.
1. Once the robot has brought you coffee, its self-preservation instinct disappears. You can turn it off.
2. One would have to be unbelievably stupid to build open-ended objectives in a super-intelligent (and super-powerful) machine without some safeguard terms in the objective.
3. One would have to be rather incompetent not to have a mechanism by which new terms in the objective could be added to prevent previously-unforeseen bad behavior. For humans, we have education and laws to shape our objective functions and complement the hardwired terms built into us by evolution.
4. The power of even the most super-intelligent machine is limited by physics, and its size and needs make it vulnerable to physical attacks. No need for much intelligence here. A virus is infinitely less intelligent than you, but it can still kill you.
5. A second machine, designed solely to neutralize an evil super-intelligent machine will win every time, if given similar amounts of computing resources (because specialized machines always beat general ones).
Bottom line: there are lots and lots of ways to protect against badly-designed intelligent machines turned evil.
Stuart has called me stupid in the Vanity Fair interview linked below for allegedly not understanding the whole idea of instrumental convergence.
It's not that I don't understand it. I think it would only be relevant in a fantasy world in which people would be smart enough to design super-intelligent machines, yet ridiculously stupid to the point of giving it moronic objectives with no safeguards.
Here is the juicy bit from the article where Stuart calls me stupid:
Russell took exception to the views of Yann LeCun, who developed the forerunner of the convolutional neural nets used by AlphaGo and is Facebook’s director of A.I. research. LeCun told the BBC that there would be no Ex Machina or Terminator scenarios, because robots would not be built with human drives—hunger, power, reproduction, self-preservation. “Yann LeCun keeps saying that there’s no reason why machines would have any self-preservation instinct,” Russell said. “And it’s simply and mathematically false. I mean, it’s so obvious that a machine will have self-preservation even if you don’t program it in because if you say, ‘Fetch the coffee,’ it can’t fetch the coffee if it’s dead. So if you give it any goal whatsoever, it has a reason to preserve its own existence to achieve that goal. And if you threaten it on your way to getting coffee, it’s going to kill you because any risk to the coffee has to be countered. People have explained this to LeCun in very simple terms.”
https://www.vanityfair.com/news/2017/03/elon-musk-billion-dollar-crusade-to-stop-ai-space-x
Tony Zador: I agree with most of what Yann wrote about Stuart Russell's concern.
Specifically, I think the flaw in Stuart's argument is the assertion that "switching off the human is the optimal solution"---who says that's an optimal solution?
I guess if you posit an omnipotent robot, destroying humanity might be a possible solution. But if the robot is not omnipotent, then killing humans comes at considerable risk, ie that they will retaliate. Or humans might build special "protector robots" whose value function is solely focused on preventing the killing of humans by other robots. Presumably these robots would be at least as well armed as the coffee robots. So this really increases the risk to the coffee robots of pursuing the genocide strategy.
And if the robot is omnipotent, then there are an infinite number of alternative strategies to ensure survival (like putting up an impenetrable forcefield around the off switch) that work just as well.
So i would say that killing all humans is not only not likely to be an optimal strategy under most scenarios, the set of scenarios under which it is optimal is probably close to a set of measure 0.
Stuart Russell: Thanks for clearing that up - so 2+2 is not equal to 4, because if the 2 were a 3, the answer wouldn't be 4? I simply pointed out that in the MDP as I defined it, switching off the human is the optimal solution, despite the fact that we didn't put in any emotions of power, domination, hate, testosterone, etc etc. And your solution seems, well, frankly terrifying, although I suppose the NRA would approve. Your last suggestion, that the robot could prevent anyone from ever switching it off, is also one of the things we are trying to avoid. The point is that the behaviors we are concerned about have nothing to do with putting in emotions of survival, power, domination, etc. So arguing that there's no need to put those emotions in is completely missing the point.
Yann LeCun: Not clear whether you are referring to my comment or Tony's.
The point is that behaviors you are concerned about are easily avoidable by simple terms in the objective. In the unlikely event that these safeguards somehow fail, my partial list of escalating solutions (which you seem to find terrifying) is there to prevent a catastrophe. So arguing that emotions of survival etc will inevitably lead to dangerous behavior is completely missing the point.
It's a bit like saying that building cars without brakes will lead to fatalities.
Yes, but why would we be so stupid as to not include brakes?
That said, instrumental subgoals are much weaker drives of behavior than hardwired objectives. Else, how could one explain the lack of domination behavior in non-social animals, such as orangutans.
Francesca Rossi: @Yann Indeed it would be odd to design an AI system with a specific goal, like fetching coffee, and capabilities that include killing humans or disallowing being turned off, without equipping it also with guidelines and priorities to constrain its freedom, so it can understand for example that fetching coffee is not so important that it is worth killing a human being to do it. Value alignment is fundamental to achieve this. Why would we build machines that are not aligned to our values? Stuart, I agree that it would easy to build a coffee fetching machine that is not aligned to our values, but why would we do this? Of course value alignment is not easy, and still a research challenge, but I would make it part of the picture when we envision future intelligent machines.
Richard Mallah: Francesca, of course Stuart believes we should create value-aligned AI. The point is that there are too many caveats to explicitly add each to an objective function, and there are strong socioeconomic drives for humans to monetize AI prior to getting it sufficiently right, sufficiently safe.
Stuart Russell: "Why would be build machines that are not aligned to our values?" That's what we are doing, all the time. The standard model of AI assumes that the objective is fixed and known (check the textbook!), and we build machines on that basis - whether it's clickthrough maximization in social media content selection or total error minimization in photo labeling (Google Jacky Alciné) or, per Danny Hillis, profit maximization in fossil fuel companies. This is going to become even more untenable as machines become more powerful. There is no hope of "solving the value alignment problem" in the sense of figuring out the right value function offline and putting it into the machine. We need to change the way we do AI.
Yoshua Bengio: All right, we're making some progress towards a healthy debate. Let me try to summarize my understanding of the arguments. Yann LeCun and Tony Zadorr argue that humans would be stupid to put in explicit dominance instincts in our AIs. Stuart Russell responds that it needs not be explicit but dangerous or immoral behavior may simply arise out of imperfect value alignment and instrumental subgoals set by the machine to achieve its official goals. Yann LeCun and Tony Zador respond that we would be stupid not to program the proper 'laws of robotics' to protect humans. Stuart Russell is concerned that value alignment is not a solved problem and may be intractable (i.e. there will always remain a gap, and a sufficiently powerful AI could 'exploit' this gap, just like very powerful corporations currently often act legally but immorally). Yann LeCun and Tony Zador argue that we could also build defensive military robots designed to only kill regular AIs gone rogue by lack of value alignment. Stuart Russell did not explicitly respond to this but I infer from his NRA reference that we could be worse off with these defensive robots because now they have explicit weapons and can also suffer from the value misalignment problem.
Yoshua Bengio: So at the end of the day, it boils down to whether we can handle the value misalignment problem, and I'm afraid that it's not clear we can for sure, but it also seems reasonable to think we will be able to in the future. Maybe part of the problem is that Yann LeCun and Tony Zador are satisfied with a 99.9% probability that we can fix the value alignment problem while Stuart Russell is not satisfied with taking such an existential risk.
Yoshua Bengio: And there is another issue which was not much discussed (although the article does talk about the short-term risks of military uses of AI etc), and which concerns me: humans can easily do stupid things. So even if there are ways to mitigate the possibility of rogue AIs due to value misalignment, how can we guarantee that no single human will act stupidly (more likely, greedily for their own power) and unleash dangerous AIs in the world? And for this, we don't even need superintelligent AIs, to feel very concerned. The value alignment problem also applies to humans (or companies) who have a lot of power: the misalignment between their interests and the common good can lead to catastrophic outcomes, as we already know (e.g. tragedy of the commons, corruption, companies lying to have you buy their cigarettes or their oil, etc). It just gets worse when more power can be concentrated in the hands of a single person or organization, and AI advances can provide that power.
Francesca Rossi: I am more optimistic than Stuart about the value alignment problem. I think that a suitable combination of symbolic reasoning and various forms of machine learning can help us to both advance AI’s capabilities and get closer to solving the value alignment problem.
Tony Zador: @Stuart Russell "Thanks for clearing that up - so 2+2 is not equal to 4, because if the 2 were a 3, the answer wouldn't be 4? "
hmm. not quite what i'm saying.
If we're going for the math analogies, then i would say that a better analogy is:
Find X, Y such that X+Y=4.
The "killer coffee robot" solution is {X=642, Y = -638}. In other words: Yes, it is a solution, but not a particularly natural or likely or good solution.
But we humans are blinded but our own warped perspective. We focus on the solution that involves killing other creatures because that appears to be one of the main solutions that we humans default to. But it is not a particularly common solution in the natural world, nor do i think it's a particularly effective solution in the long run.
Yann LeCun: Humanity has been very familiar with the problem of fixing value misalignments for millenia.
We fix our children's hardwired values by teaching them how to behave.
We fix human value misalignment by laws. Laws create extrinsic terms in our objective functions and cause the appearance of instrumental subgoals ("don't steal") in order to avoid punishment. The desire for social acceptance also creates such instrumental subgoals driving good behavior.
We even fix value misalignment for super-human and super-intelligent entities, such as corporations and governments.
This last one occasionally fails, which is a considerably more immediate existential threat than AI.
Tony Zador: @Yoshua Bengio I agree with much of your summary. I agree value alignment is important, and that it is not a solved problem.
I also agree that new technologies often have unintended and profound consequences. The invention of books has led to a decline in our memories (people used to recite the entire Odyssey). Improvements in food production technology (and other factors) have led to a surprising obesity epidemic. The invention of social media is disrupting our political systems in ways that, to me anyway, have been quite surprising. So improvements in AI will undoubtedly have profound consequences for society, some of which will be negative.
But in my view, focusing on "killer robots that dominate or step on humans" is a distraction from much more serious issues.
That said, perhaps "killer robots" can be thought of as a metaphor (or metonym) for the set of all scary scenarios that result from this powerful new technology.
Yann LeCun: @Stuart Russell you write "we need to change the way we do AI". The problems you describe have nothing to do with AI per se.
They have to do with designing (not avoiding) explicit instrumental objectives for entities (e.g. corporations) so that their overall behavior works for the common good. This is a problem of law, economics, policies, ethics, and the problem of controlling complex dynamical systems composed of many agents in interaction.
What is required is a mechanism through which objectives can be changed quickly when issues surface. For example, Facebook stopped maximizing clickthroughs several years ago and stopped using the time spent in the app as a criterion about 2 years ago. It put in place measures to limit the dissemination of clickbait, and it favored content shared by friends rather than directly disseminating content from publishers.
We certainly agree that designing good objectives is hard. Humanity has struggled with designing objectives for itself for millennia. So this is not a new problem. If anything, designing objectives for machines, and forcing them to abide by them will be a lot easier than for humans, since we can physically modify their firmware.
There will be mistakes, no doubt, as with any new technology (early jetliners lost wings, early cars didn't have seat belts, roads didn't have speed limits...).
But I disagree that there is a high risk of accidentally building existential threats to humanity.
Existential threats to humanity have to be explicitly designed as such.
Yann LeCun: It will be much, much easier to control the behavior of autonomous AI systems than it has been for humans and human organizations, because we will be able to directly modify their intrinsic objective function.
This is very much unlike humans, whose objective can only be shaped through extrinsic objective functions (through education and laws), that indirectly create instrumental sub-objectives ("be nice, don't steal, don't kill, or you will be punished").
As I have pointed out in several talks in the last several years, autonomous AI systems will need to have a trainable part in their objective, which would allow their handlers to train them to behave properly, without having to directly hack their objective function by programmatic means.
Yoshua Bengio: Yann, these are good points, we indeed have much more control over machines than humans since we can design (and train) their objective function. I actually have some hopes that by using an objective-based mechanism relying on learning (to inculcate values) rather than a set of hard rules (like in much of our legal system), we could achieve more robustness to unforeseen value alignment mishaps. In fact, I surmise we should do that with human entities too, i.e., penalize companies, e.g. fiscally, when they behave in a way which hurts the common good, even if they are not directly violating an explicit law. This also suggests to me that we should try to avoid that any entity (person, company, AI) have too much power, to avoid such problems. On the other hand, although probably not in the near future, there could be AI systems which surpass human intellectual power in ways that could foil our attempts at setting objective functions which avoid harm to us. It seems hard to me to completely deny that possibility, which thus would beg for more research in (machine-) learning moral values, value alignment, and maybe even in public policies about AI (to minimize the events in which a stupid human brings about AI systems without the proper failsafes) etc.
Yann LeCun: @Yoshua Bengio if we can build "AI systems which surpass human intellectual power in ways that could foil our attempts at setting objective functions", we can also build similarly-powerful AI systems to set those objective functions.
Sort of like the discriminator in GANs....
Yann LeCun: @Yoshua Bengio a couple direct comments on your summary:
- designing objectives for super-human entities is not a new problem. Human societies have been doing this through laws (concerning corporations and governments) for millennia.
- the defensive AI systems designed to protect against rogue AI systems are not akin to the military, they are akin to the police, to law enforcement. Their "jurisdiction" would be strictly AI systems, not humans.
But until we have a hint of a beginning of a design, with some visible path towards autonomous AI systems with non-trivial intelligence, we are arguing about the sex of angels.
Yuri Barzov: Aren't we overestimating the ability of imperfect humans to build a perfect machine? If it will be much more powerful than humans its imperfections will be also magnified. Cute human kids grow up into criminals if they get spoiled by reinforcement i.e. addiction to rewards. We use reinforcement and backpropagation (kind of reinforcement) in modern golden standard AI systems. Do we know enough about humans to be able to build a fault-proof human friendly super intelligent machine?
Yoshua Bengio: @Yann LeCun, about discriminators in GANs, and critics in Actor-Critic RL, one thing we know is that they tend to be biased. That is why the critic in Actor-Critic is not used as an objective function but instead as a baseline to reduce the variance. Similarly, optimizing the generator wrt a fixed discriminator does not work (you would converge to a single mode - unless you balance that with entropy maximization). Anyways, just to say, there is much more research to do, lots of unknown unknowns about learning moral objective functions for AIs. I'm not afraid of research challenges, but I can understand that some people would be concerned about the safety of gradually more powerful AIs with misaligned objectives. I actually like the way that Stuart Russell is attacking this problem by thinking about it not just in terms of an objective function but also about uncertainty: the AI should avoid actions which might hurt us (according to a self-estimate of the uncertain consequences of actions), and stay the conservative course with high confidence of accomplishing the mission while not creating collateral damage. I think that what you and I are trying to say is that all this is quite different from the terminator scenarios which some people in the media are brandishing. I also agree with you that there are lots of unknown unknowns about the strengths and weaknesses of future AIs, but I think that it is not too early to start thinking about these issues.
Yoshua Bengio: @Yuri Barzov the answer to your question: no. But we don't know that it is not feasible either, and we have reasons to believe that (a) it is not for tomorrow such machines will exist and (b) we have intellectual tools which may lead to solutions. Or maybe not!
Stuart Russell: Yann's comment "Facebook stopped maximizing clickthroughs several years ago and stopped using the time spent in the app as a criterion about 2 years ago" makes my point for me. Why did they stop doing it? Because it was the wrong objective function. Yann says we'd have to be "extremely stupid" to put the wrong objective into a super-powerful machine. Facebook's platform is not super-smart but it is super-powerful, because it connects with billions of people for hours every day. And yet they put the wrong objective function into it. QED. Fortunately they were able to reset it, but unfortunately one has to assume it's still optimizing a fixed objective. And the fact that it's operating within a large corporation that's designed to maximize another fixed objective - profit - means we cannot switch it off.
Stuart Russell: Regarding "externalities" - when talking about externalities, economists are making essentially the same point I'm making: externalities are the things not stated in the given objective function that get damaged when the system optimizes that objective function. In the case of the atmosphere, it's relatively easy to measure the amount of pollution and charge for it via taxes or fines, so correcting the problem is possible (unless the offender is too powerful). In the case of manipulation of human preferences and information states, it's very hard to assess costs and impose taxes or fines. The theory of uncertain objectives suggests instead that systems be designed to be "minimally invasive", i.e., don't mess with parts of the world state whose value is unclear. In particular, as a general rule it's probably best to avoid using fixed-objective reinforcement learning in human-facing systems, because the reinforcement learner will learn how to manipulate the human to maximize its objective.
Stuart Russell: @Yann LeCun Let's talk about climate change for a change. Many argue that it's an existential or near-existential threat to humanity. Was it "explicitly designed" as such? We created the corporation, which is a fixed-objective maximizer. The purpose was not to create an existential risk to humanity. Fossil-fuel corporations became super-powerful and, in certain relevant senses, super-intelligent: they anticipated and began planning for global warming five decades ago, executing a campaign that outwitted the rest of the human race. They didn't win the academic argument but they won in the real world, and the human race lost. I just attended an NAS meeting on climate control systems, where the consensus was that it was too dangerous to develop, say, solar radiation management systems - not because they might produce unexpected disastrous effects but because the fossil fuel corporations would use their existence as a further form of leverage in their so-far successful campaign to keep burning more carbon.
Stuart Russell: @Yann LeCun This seems to be a very weak argument. The objection raised by Omohundro and others who discuss instrumental goals is aimed at any system that operates by optimizing a fixed, known objective; which covers pretty much all present-day AI systems. So the issue is: what happens if we keep to that general plan - let's call it the "standard model" - and improve the capabilities for the system to achieve the objective? We don't need to know today *how* a future system achieves objectives more successfully, to see that it would be problematic. So the proposal is, don't build systems according to the standard model.
Yann LeCun: @Stuart Russell the problem is that essentially no AI system today is autonomous.
They are all trained *in advance* to optimize an objective, and subsequently execute the task with no regards to the objective, hence with no way to spontaneously deviate from the original behavior.
As of today, as far as I can tell, we do *not* have a good design for an autonomous machine, driven by an objective, capable of coming up with new strategies to optimize this objective in the real world.
We have plenty of those in games and simple simulation. But the learning paradigms are way too inefficient to be practical in the real world.
Yuri Barzov: @Yoshua Bengio yes. If we frame the problem correctly we will be able to resolve it. AI puts natural intelligence into focus like a magnifying mirror
Yann LeCun: @Stuart Russell in pretty much everything that society does (business, government, of whatever) behaviors are shaped through incentives, penalties via contracts, regulations and laws (let's call them collectively the objective function), which are proxies for the metric that needs to be optimized.
Because societies are complex systems, because humans are complex agents, and because conditions evolve, it is a requirement that the objective function be modifiable to correct unforeseen negative effects, loopholes, inefficiencies, etc.
The Facebook story is unremarkable in that respect: when bad side effects emerge, measures are taken to correct them. Often, these measures eliminate bad actors by directly changing their economic incentive (e.g. removing the economic incentive for clickbaits).
Perhaps we agree on the following:
(0) not all consequences of a fixed set of incentives can be predicted.
(1) because of that, objectives functions must be updatable.
(2) they must be updated to correct bad effect whenever they emerge.
(3) there should be an easy way to train minor aspects of objective functions through simple interaction (similar to the process of educating children), as opposed to programmatic means.
Perhaps where we disagree is the risk of inadvertently producing systems with badly-designed and (somehow) un-modifiable objectives that would be powerful enough to constitute existential threats.
Yoshua Bengio: @Yann LeCun this is true, but one aspect which concerns me (and others) is the gradual increase in power of some agents (now mostly large companies and some governments, potentially some AI systems in the future). When it was just weak humans the cost of mistakes or value misalignment (improper laws, misaligned objective function) was always very limited and local. As we build more and more powerful and intelligent tools and organizations, (1) it becomes easier to cheat for 'smarter' agents (exploit the misalignment) and (2) the cost of these misalignments becomes greater, potentially threatening the whole of society. This then does not leave much time and warning to react to value misalignment.
61 comments
Comments sorted by top scores.
comment by Vaniver · 2019-10-04T16:16:43.286Z · LW(p) · GW(p)
There's a dynamic that's a normal part of cognitive specialization of labor, where the work other people are doing is "just X"; imagine trying to create a newspaper, for example. Most people will think of writing articles as "just journalism"; you pay journalists whatever salary, they do whatever work, and you get articles for your newspaper. Similarly the accounting is "just accounting," and so on. But the journalist can't see journalism as "just journalism"; if their model of how to write articles is "money goes in, article comes out" they won't be able to write any articles. Instead they have lots of details about how to write articles, which includes what articles are and aren't easy.
You could view both sides as doing something like this: the person who's trying to make safeguards is saying "look, you can't say 'just add safeguards', these things are really difficult" and the person who's trying to make something worth safeguarding is saying "look, you can't just 'just build an autonomous superintelligence', these things are really difficult." (Especially since I think LeCun views them as too difficult to try to do, and instead is just trying to get some subcomponents.)
I think that's part of what's going on, but mostly in how it seems to obscure the core issue (according to me), which is related to Yoshua's last point: "what safeguards we need when" is part of the safeguard science that we haven't done yet. I think we're in a situation where many people say "yes, we'll need safeguards, but it'll be easy to notice when we need them and implement them when we notice" and the people trying to build those safeguards respond with "we don't think either of those things will be easy." But notice how, in the backdrop of "everyone thinks their job is hard," this statement provides very little ability to distinguish between worlds where this actually is a crisis and worlds where things will be fine!
Replies from: DanielFilan, rohinmshah↑ comment by DanielFilan · 2019-10-04T20:31:15.046Z · LW(p) · GW(p)
I see this in a different light: as far as I can tell, Yann LeCun believes that the way to advance AI is to tinker around, take opportunities to make advances when it seems feasible, find ways of fixing problems that come up in an ad-hoc, atheoretic manner (see e.g. this link), and then form some theory to explain what happened; while Stuart Russell thinks that it's important to have a theory that you really believe in drive future work. As a result, I read LeCun as saying that when problems come up, we'll see them and fix them by tinkering around, while Russell thinks that it's important to have a theory in place before-hand to ensure that bad enough problems don't come up and/or ensure that we already know how to solve them when they do.
Replies from: Vaniver↑ comment by Vaniver · 2019-10-04T21:24:46.421Z · LW(p) · GW(p)
It seems like this is the sort of deep divide that is hard to cross, since I would expect people to have strong opinions based on what they've seen work elsewhere. It has an echo of the previous concern, where Russell needs to somehow point out "look, this time it actually is important to have a theory instead of doing things ad-hoc" in a way that depends on the features of this particular issue rather than the way he likes doing work.
Replies from: Grue_Slinky↑ comment by Grue_Slinky · 2019-10-06T12:33:00.765Z · LW(p) · GW(p)
For reference, LeCun discussed his atheoretic/experimentalist views in more depth in this FB debate with Ali Rahimi and also this lecture. But maybe we should distinguish some distinct axes of the experimentalist/theorist divide in DL:
1) Experimentalism/theorism is a more appropriate paradigm for thinking about AI safety
2) Experimentalism/theorism is a more appropriate paradigm for making progress in AI capabilities
Where the LeCun/Russell debate is about (1) and LeCun/Rahimi is about (2). And maybe this is oversimplifying things, since "theorism" may be an overly broad way of describing Russell/Rahimi's views on safety/capabilities, but I suspect LeCun is "seeing the same ghost", or in his words (to Rahimi), seeing the same:
kind of attitude that lead the ML community to abandon neural nets for over 10 years, *despite* ample empirical evidence that they worked very well in many situations.
And whether or not Rahimi should be lumped into that "kind of attitude", I think LeCun is right (from a certain perspective) to want to push back against that attitude.
I'd even go further: given that LeCun has been more successful than Rahimi/Russell in AI research this century, all else equal I would weight the former's intuitions on research progress more. (I think the best counterargument is that while experimentalism might be better in the short-term, theorism has better payoff in the long-term, but I'm not sure about this.)
In fact, one of my major fears is that LeCun is right about this, because even if he is right about (2), I don't think that's good evidence he's right about (1) since these seem pretty orthogonal. But they don't look orthogonal until you spend a lot of time reading/thinking about AI safety, which you're not inclined to do if you already know a lot about AI and assume that knowledge transfers to AI safety.
In other words, the "correct" intuitions (on experimentalism/theorism) for modern AI research might be the opposite of the "correct" intuitions for AI safety. (I would, for instance, predict that if Superintelligence were published during the era of GOFAI, all else equal it would've made a bigger splash because AI researchers then were more receptive to abstract theorizing.)
Replies from: orthonormal↑ comment by orthonormal · 2019-11-04T17:24:41.488Z · LW(p) · GW(p)
Good comment. I disagree with this bit:
I would, for instance, predict that if Superintelligence were published during the era of GOFAI, all else equal it would've made a bigger splash because AI researchers then were more receptive to abstract theorizing.
And then it would probably have been seen as outmoded and thrown away completely when AI capabilities research progressed into realms that vastly surpassed GOFAI. I don't know that there's an easy way to get capabilities researchers to think seriously about safety concerns that haven't manifested on a sufficient scale yet.
↑ comment by Rohin Shah (rohinmshah) · 2019-10-07T22:59:04.299Z · LW(p) · GW(p)
But notice how, in the backdrop of "everyone thinks their job is hard," this statement provides very little ability to distinguish between worlds where this actually is a crisis and worlds where things will be fine!
It sounds like you have a model that "person works in a job" causes "person believes job is hard" regardless of what the job is, but the causality can go the other way: if I thought AI safety were trivial, I wouldn't be working on trying to make it safe.
On this model, you don't observe this argument because everyone is biased towards thinking their job is hard: you observe it because people formed opinions some other way and then self-selected into the jobs they thought were impactful / nontrivial.
In practice, it will be a combination of both. For this discussion in particular, I'd lean more towards the selection explanation, as opposed to the bias explanation.
comment by Kaj_Sotala · 2019-10-04T06:42:34.727Z · LW(p) · GW(p)
It looks to me like this conversation is to some extent repeating a pattern which I've seen in AI safety conversations before:
Safety advocate: AI might destroy us if it doesn't have the right safeguards.
Safety skeptic: That's stupid, because why would anyone build it without those safeguards.
It feels like people keep talking past each other, since both essentially agree about the need for safeguards. Rather the disagreement seems to be over something more like... "does the default path of AI development involve existential risks or not", where the safety advocate argues that we should be thinking about this a lot beforehand, much more than with other technologies. On the other hand, the skeptic sees AI as being much more comparable to any other technology, in that there are risks and there will probably be accidents until we figure out how to do it safely, but we will do that figuring out as a normal part of developing the technology and we can't really do much of that figuring out until we actually have the technology.
Replies from: whpearson↑ comment by whpearson · 2019-10-04T09:30:20.314Z · LW(p) · GW(p)
My view is that you have to build AIs with a bunch of safeguards to stop it destroying *itself* while it doesn't have great knowledge of the world or the consequences of its actions. So some of the arguments around companies/governments skimping on safety don't hold in the naive sense.
So things like how do you :
- Stop a robot jumping off something too high
- Stop an AI DOSing it's own network connection
- Stop a robot disassembling itself
When it is not vastly capable. Solving these things would give you a bunch of knowledge of safeguards and how to build them. I wrote about some of problems here [LW · GW]
It is only when you expect a system to radically gain capability without needing any safeguards, does it makes sense to expect there to be a dangerous AI created by a team with no experience of safe guards or how to embed them.
Replies from: steve2152, MichaelA, Kaj_Sotala↑ comment by Steven Byrnes (steve2152) · 2019-10-04T19:13:13.093Z · LW(p) · GW(p)
One thing you can do to stop a robot from destroying itself is to give it more-or-less any RL reward function whatsoever, and get better and better at designing it to understand the world and itself and act in the service of getting that reward (because of instrumental convergence). For example, each time the robot destroys itself, you build a new one seeded with the old one's memory, and tell it that its actions last time got a negative reward. Then it will learn not to do that in the future. Remember, an AGI doesn't need a robot body; a prototype AGI that accidentally corrupts its own code can be recreated instantaneously for zero cost. Why then build safeguards?
Safeguards would be more likely if the AGI were, say, causing infrastructure damage while learning. I can definitely see someone, say, removing internet access, after mishaps like that. That's still not an adequate safeguard, in that when the AGI gets intelligent enough, it could hack or social-engineer its way through safeguards that were working before.
Replies from: Vaniver↑ comment by Vaniver · 2019-10-04T21:09:24.303Z · LW(p) · GW(p)
I think this scheme doesn't quite catch the abulia trap (where the AGI discovers a way to directly administer itself reward, and then ceases to interact with the outside world), in that it's not clear that the AI learns about the map/territory distinction and to locate its goals in the territory (one way to avoid this) instead of just a prohibition against many sorts of self-modification or reward tampering (which avoids this until it comes up with a clever new approach).
↑ comment by MichaelA · 2020-03-30T11:51:15.067Z · LW(p) · GW(p)
I might be misunderstanding you, but I feel like this is sort of missing a key point. It seems like there could be situations in which the AI does indeed, as you point out, require "a bunch of safeguards to stop it destroying *itself*", in order to advance to a high level of capabilities. These could be built by its engineers, or developed by the AI itself, perhaps through trial and error.
But that doesn't seem to mean it'd have safeguards to not destroy other things we value, or in some more abstract sense "destroy" our future potential (e.g., by colonising space and "wasting" the resources optimising for something that we don't/barely care about, even if it doesn't harm anything on Earth). It seems possible for an AI to get safeguards like how to not have its robotic manifestation jump off things too high or disassemble itself, and thereby be "safe enough" itself to become more capable, but to not have the sort of "safeguards" that e.g. Russell cares about.
Indeed, this seems to related to the core point of ideas like instrumental convergent subgoals and differential progress. We or the AI might get really good at building its capabilities and building safeguards that allow it to become more capable or avoid harm to itself or its own current "goals", without necessarily getting good at building safeguards to protect "what we truly value".
But here's two things you might have meant that would be consistent with what I've said:
- It is only when you expect a system to radically gain capability without needing any safeguards to protect a particular thing that it makes sense to expect there to be a dangerous AI created by a team with no experience of safe guards to protect that particular thing or how to embed them. This may inform LeCun's views, if he's focusing on safeguards for the AI's own ability to operate in the world, since these will have to be developed in order for the AI to become more capable. But Russell may be focusing on the fact that a system really could radically gain capability without needing safeguards to protect what we value.
- It is only when you expect a system to radically gain capability without needing any safeguards of any type, does it makes sense to expect there to be a dangerous AI created by a team with no experience of safeguards in general or how to embed them. Since AI designers will have to learn how to develop and embed some types of safeguard, they're likely to pick up general skills for that, which could then also be useful for building safeguards to protect what we value.
If what you meant is the latter, then I don't think I'm comfortable resting on the assumption that lessons from developing/embedding "capability safeguards" (so to speak) will transfer to a high degree to "safety safeguards". Although I haven't looked into it a great deal.
Is one of those things what you meant?
↑ comment by Kaj_Sotala · 2019-10-04T10:21:53.653Z · LW(p) · GW(p)
It is only when you expect a system to radically gain capability without needing any safeguards, does it makes sense to expect there to be a dangerous AI created by a team with no experience of safe guards or how to embed them.
That sounds right to me. Also worth noting that much of what parents do for the first few years of a child's life is just trying to stop the child from killing/injuring themselves, when the child's own understanding of the world isn't sufficiently developed yet.
comment by Steven Byrnes (steve2152) · 2019-10-04T19:50:29.424Z · LW(p) · GW(p)
Yann's core argument for why AGI safety is easy is interesting, and actually echoes ongoing AGI safety research. I'll paraphrase his list of five reasons that things will go well if we're not "ridiculously stupid":
- We'll give AGIs non-open-ended objectives like fetching coffee. These are task-limited and therefore there's no more instrumental subgoals after the task is complete.
- We will put "simple terms in the objective" to prevent obvious problems, presumably things like "don't harm people", "don't violate laws", etc.
- We will put in "a mechanism" to edit the objective upon observing bad behavior;
- We can physically destroy a computer housing AGI;
- We can build a second AGI whose sole purpose is to destroy the first AGI if the first AGI has gotten out of control, and the latter will succeed because it's more specialized.
All of these are reasonable ideas on their face, and indeed they're similar to ongoing AGI safety research programs: (1) is myopic or task-limited AGI, (2) is related to AGI limiting and norm-following, (3) is corrigibility, (4) is boxing, and (5) is in the subfield of AIs-helping-with-AGI-safety (other things in this area include IDA, adversarial testing, recursive reward modeling, etc.).
The problem, of course, is that all five of these things, when you look at them carefully, are much harder and more complicated than they appear, and/or less likely to succeed. And meanwhile he's discouraging people from doing the work to solve those problems.. :-(
Replies from: TurnTrout, TAG↑ comment by TurnTrout · 2019-10-04T20:21:50.476Z · LW(p) · GW(p)
I don’t know that his arguments “echo”, it’s more like “can be translated into existing discourse”. For example, the leap from his 5) to IDA is massive, and I don’t understand why he imagines tackling the “we can’t align AGIs” problem with “build another AGI to stop the bad AGI”.
Replies from: Vaniver↑ comment by Vaniver · 2019-10-04T21:11:53.627Z · LW(p) · GW(p)
I think 5 is much closer to the "look, the first goal is to build a system that prevents anyone else from building unaligned AGI" claim, and there's a separate claim 6 of the form "more generally, we can use AGI to police AGI" that is similar to debate or IDA. And I think claim 5 is basically in line with what, say, Bostrom would discuss (where stabilization is a thing to do before we attempt to build a sovereign).
Replies from: ESRogs↑ comment by ESRogs · 2019-10-04T23:24:30.910Z · LW(p) · GW(p)
And I think claim 5 is basically in line with what, say, Bostrom would discuss (where stabilization is a thing to do before we attempt to build a sovereign).
You mean in the sense of stabilizing the whole world? I'd be surprised if that's what Yann had in mind. I took him just to mean building a specialized AI to be a check on a single other AI.
Replies from: Vaniver↑ comment by Vaniver · 2019-10-05T02:45:11.941Z · LW(p) · GW(p)
That's how I interpreted:
the defensive AI systems designed to protect against rogue AI systems are not akin to the military, they are akin to the police, to law enforcement. Their "jurisdiction" would be strictly AI systems, not humans.
To be clear, I think he would mean it more in the way that there's currently an international police order that is moderately difficult to circumvent, and that the same would be true for AGI, and not necessarily the more intense variants of stabilization (which are necessarily primarily if you think offense is highly advantaged over defense, which I don't know his opinion on).
↑ comment by TAG · 2019-10-05T09:19:53.752Z · LW(p) · GW(p)
And meanwhile he’s discouraging people from doing the work to solve those problems.. :-(
Discouraging everyone, including AI researchers, or discouraging an AI safety movement that is disjoint from AI research?
Replies from: capybaralet↑ comment by David Scott Krueger (formerly: capybaralet) (capybaralet) · 2019-10-11T06:38:35.437Z · LW(p) · GW(p)
No idea why this is heavily downvoted; strong upvoted to compensate.
I'd say he's discouraging everyone from working on the problems, or at least from considering such work to be important, urgent, high status, etc.
Replies from: RobbBB↑ comment by Rob Bensinger (RobbBB) · 2019-10-11T13:44:01.380Z · LW(p) · GW(p)
I downvoted TAG's comment because I found it confusing/misleading. I can't tell which of these things TAG's trying to do:
- Assert, in a snarky/indirect way, that people agitating about AI safety have no overlap with AI researchers. This seems doubly weird in a conversation with Stuart Russell.
- Suggest that LeCun believes this. (??)
- Assert that LeCun doesn't mean to discourage Russell's research. (But the whole conversation seems to be about what kind of research people should be doing when in order to get good outcomes from AI.)
↑ comment by TAG · 2019-10-31T11:56:23.787Z · LW(p) · GW(p)
I downvoted TAG’s comment because I found it confusing/misleading.
You could have asked for clarification. The point is that Yudkowsky's early movement was disjoint from actual AI research, and during that period a bunch of dogmas and approaches became solidified, which a lot of AI researchers (Russell is an exception) find incomprehensible or misguided. In other words, you can disapprove of amateur AI safety without dismissing AI safety wholesale.
Replies from: Evan R. Murphy↑ comment by Evan R. Murphy · 2022-07-16T06:51:22.330Z · LW(p) · GW(p)
(Responding to the above comment years later...)
It seems like "amateur" AI safety researchers have been the main ones willing to seriously think about AGI and on-the-horizon advanced AI systems from a safety angle though.
However, I do think you're pointing to a key potential blindspot in the AI safety community. Fortunately AI safety folks are studying ML more, and I think ML researchers are starting to be more receptive to discussions about AGI and safety. So this may become a moot point.
comment by sudhanshu_kasewa · 2019-10-24T23:46:58.036Z · LW(p) · GW(p)
ICYMI: Yann posted this page on FB as well, and some additional conversation happened there, with at least one interesting exchange between Rob Bensinger and Tony Zador:
https://www.facebook.com/yann.lecun/posts/10156278492457143
(I did scan the comments and didn't see this posted earlier, so...)
comment by dxu · 2019-10-04T04:27:05.281Z · LW(p) · GW(p)
Skimming through. May or may not post an in-depth comment later, but for the time being, this stood out to me:
I think it would only be relevant in a fantasy world in which people would be smart enough to design super-intelligent machines, yet ridiculously stupid to the point of giving it moronic objectives with no safeguards.
I note that Yann has not actually specified a way of not "giving [the AI] moronic objectives with no safeguards". The argument of AI risk advocates is precisely that the thing in quotes in the previous sentence is difficult to do, and that people do not have to be "ridiculously stupid" to fail at it--as evidenced by the fact that no one has actually come up with a concrete way of doing it yet. It doesn't look to me like Yann addressed this point anywhere; he seems to be under the impression that repeating his assertion more emphatically (obviously, when we actually get around to building the AI, we'll use our common sense and build it right) somehow constitutes an argument in favor of said assertion. This seems to be an unusually low-quality line of argument from someone who, from what I've seen, is normally much more clear-headed than this.
Replies from: John_Maxwell_IV↑ comment by John_Maxwell (John_Maxwell_IV) · 2019-10-06T05:34:32.380Z · LW(p) · GW(p)
Nor has anyone come up with a way to make AGI. Perhaps Yann's assumption is that how to do what he specifies will become more obvious as more about the nature of AGI is known. Maybe from Yann's perspective, trying to create safe AGI without knowing how AGI will work is like trying to design a nuclear reactor without knowing how nuclear physics works.
(Not saying I agree with this.)
comment by Vaniver · 2019-10-04T04:58:58.377Z · LW(p) · GW(p)
I just attended an NAS meeting on climate control systems, where the consensus was that it was too dangerous to develop, say, solar radiation management systems - not because they might produce unexpected disastrous effects but because the fossil fuel corporations would use their existence as a further form of leverage in their so-far successful campaign to keep burning more carbon.
Unrelated to the primary point, but how does this make sense? If geoengineering approaches successfully counteract climate change, and it's cheaper to burn carbon and dim the sun than generate power a different way (or not use the power), then presumably civilization is better off burning carbon and dimming the sun.
It looks to me the argument is closer to "because the fossil fuel corporations are acting adversarially to us, we need to act adversarially to them," or expecting that instead of having sensible engineering or economic tradeoffs, we'll choose 'burn carbon and dim the sun' even if it's more expensive than other options, because we can't coordinate on putting the costs in the right place.
Which... maybe I buy, but this looks to me like net-negative environmentalism again (like anti-nuclear environmentalism).
Replies from: matthew-barnett, romeostevensit, kvas↑ comment by Matthew Barnett (matthew-barnett) · 2019-10-04T05:30:41.865Z · LW(p) · GW(p)
It seems to me that the intention is that solar radiation management is a solution that sounds good without actually being good. That is, it's an easy sell for fossil fuel corporations who have an interest in providing simple solutions to the problem rather than actually removing the root cause and thus solving the issue completely. I have little idea if this argument is actually true.
Replies from: MakoYass↑ comment by mako yass (MakoYass) · 2019-10-06T05:31:19.326Z · LW(p) · GW(p)
It is true, as far as I can tell. It's going to be very important that we deploy SRM (and I hope we can do marine cloud brightening [LW · GW] instead of aerosols cause it seems like it'd have basically no side-effects) at some stage... probably around 2030... but the remaining CO2 will pose a huge problem. Ocean acidification, and also, once CO2 gets high enough, it starts impacting human cognition. We don't really know why, but it's an easily measurable effect, the loss in productivity will be immense, and we might imagine that our hopes of finding better carbon sequestration technologies after that dumbing point may plummet.
I get the sense that environmentalists, for now, should not talk about SRM. We should let the public believe that we don't have a way of preventing temperature increases so that we retain some hope of getting political support for doing something about the CO2.
Replies from: matthew-barnett↑ comment by Matthew Barnett (matthew-barnett) · 2019-10-06T05:45:15.412Z · LW(p) · GW(p)
once CO2 gets high enough, it starts impacting human cognition.
Do you have a citation for this being a big deal? I'm really curious whether this is a major harm over reasonable timescales (such as 100 years), as I don't recall ever hearing about it in an EA analysis of climate change. That said, I haven't looked very hard.
Replies from: MakoYass, MichaelA↑ comment by mako yass (MakoYass) · 2019-10-06T06:22:57.760Z · LW(p) · GW(p)
I don't remember what the concentrations were where it'd become a cognition problem, but they always seemed shockingly low. I note that CO2 is heavier than oxygen so the concentration on the ground is probably (?) going to be higher than the concentration measured for the purposes of estimating greenhouse effects.
I wonder how many climate models take the decreases in productivity of phytoplankton into account. With numbers of whales decreasing, there will be less carbon turnover, and some aspects of their productivity seems to be affected dramatically by microplastics.
For cites, I wont be able to do better than a google search.
I think I remember hearing that there was no data on what happens if a human is kept in a high CO2 environment for longer timespans, though. Might turn out we adapt in the same way some populations adapt to high altitudes.
↑ comment by MichaelA · 2020-03-30T12:11:27.276Z · LW(p) · GW(p)
I have no citation for that being a big deal. But there's some discussion of the matter (which I haven't read) in the comments on this post [LW · GW], and it was also discussed on an episode of the 80k podcast:
Paul Christiano: I think the current state of the literature on carbon dioxide and cognition is absurd. I probably complained about this last time I was here.
[...]
Robert Wiblin: Yes, talk about the carbon dioxide one for a minute because this is one that’s also been driving me mad the last few months just to see that carbon dioxide potentially has enormous effects on people’s intelligence and in offices but you eventually just have extremely– And lecture halls especially just have potentially incredibly elevated CO2 levels that are dumbing us all down when we most need to be smart.
Paul Christiano: Yes. I reviewed the literature a few years ago and I’ve only been paying a little bit of attention since then, but I think the current state of play is, there was one study with preposterously large effect sizes from carbon dioxide in which the methodology was put people in rooms, dump some gas into all the rooms. Some of the gases were very rich in carbon dioxide and the effect sizes were absurdly large.
They were like, if you compare it to the levels of carbon dioxide that occur in my house or in the house I just moved out of, the most carbon dioxide-rich bedroom in that house had one standard deviation effect amongst Berkeley students on this test or something, which is absurd. That’s totally absurd. That’s almost certainly–
Robert Wiblin: It’s such a large effect that you should expect that people, when they walk into a room with carbon dioxide which has elevated carbon dioxide levels, they should just feel like idiots at that point or they should feel like noticeably dumber in their own minds.
Paul Christiano: Yes, you would think that. To be clear, the rooms that have levels that high, people can report it feels stuffy and so part of the reason that methodology and the papers like just dumping in carbon dioxide is to avoid like if you make a room naturally that CO2 rich, it’s going to also just be obvious that you’re in the intervention group instead of the control.
Although to be fair, even if I don’t know, at that point, like even a placebo effect maybe will do something. I think almost certainly that seems wrong to me. Although maybe this is not a good thing to be saying publicly on a podcast. There’s a bunch of respected researchers on that paper. Anyway, it would be great to see a replication of that. There was subsequently replication with exactly the same design which also had p = 0.0001.
Now, we’ve got the two precise replications with p = 0.0001. That’s where we’re at. Also the effects are stupidly large. So large. You really, really need to care about ventilation effects. This room probably is, this is madness. Well, this building is pretty well ventilated but still, we’re at least a third of a standard deviation dumber.
Robert Wiblin: Yes, I’m sure dear listeners you can hear us getting dumber over the course of this conversation as we fill this room with poison. Yes, I guess potentially the worst case would be in meeting rooms or boardrooms where people are having very long– Yes prolonged discussions about difficult issues. They’re just getting progressively dumber as the room fills up with carbon dioxide and it’s going to be more irritable as well.
Paul Christiano: Yes, it would be pretty serious and I think that people have often cited this in attempts to improve ventilation, but I think people do not take it nearly as seriously as they would have if they believed it. Which I think is right because I think it’s almost certainly, the effect is not this large. If it was this large, you’d really want to know and then–
Robert Wiblin: This is like lead poisoning or something?
Paul Christiano: Yes, that’s right.
Robert Wiblin: Well, this has been enough to convince me to keep a window open whenever I’m sleeping. I really don’t like sleeping in a room that has no ventilation or no open door or window. Maybe I just shouldn’t worry because at night who really cares how smart I’m feeling while I’m dreaming?
Paul Christiano: I don’t know what’s up. I also haven’t looked into it as much as maybe I should have. I would really just love to be able to stay away, it’s not that hard. The facts are large enough but it’s also short term enough to just like extremely easy to check. In some sense, it’s like ”What are you asking for, there’s already been a replication”, though, I don’t know, the studies they use are with these cognitive batteries that are not great.
If the effects are real you should be able to detect them in very– Basically with any instrument. At some point, I just want to see the effect myself. I want to actually see it happen and I want to see the people in the rooms.
Robert Wiblin: Seems like there’s a decent academic incentive to do this, you’d think, because you’d just end up being famous if you pioneer this issue that turns out to be extraordinarily important and then causes buildings to be redesigned. I don’t know, it could just be a big deal. I mean, even if you can’t profit from it in a financial sense, wouldn’t you just want the kudos for like identifying this massive unrealized problem?
Paul Christiano: Yes, I mean to be clear, I think a bunch of people work on the problem and we do have– At this point there’s I think there’s the original– The things I’m aware of which is probably out of date now is the original paper, a direct replication and a conceptual replication all with big looking effects but all with slightly dicey instruments. The conceptual replication is funded by this group that works on ventilation unsurprisingly.
Robert Wiblin: Oh, that’s interesting.
Paul Christiano: Big air quality. Yes, I think that probably the take of academics, insofar as there’s a formal consensus process in academia, I think it would be to the effect that this is real, it’s just that no one is behaving as if the effect of that size actually existed and I think they’re right to be skeptical of the process, in academia. I think that does make– The situation is a little bit complicated in terms of what you exactly get credit for.
I think people that would get credit should be and rightfully would be the people who’ve been investigating it so far. This is sort of more like checking it out more for– Checking it out for people who are skeptical. Although everyone is implicitly skeptical given how much they don’t treat it like an emergency when carbon dioxide levels are high.
Robert Wiblin: Yes, including us right now. Well, kudos to you for funding that creatine thing [discussed elsewhere in the episode]. It would be good if more people took the initiative to really insist on funding replications for issues that seemed important where they’re getting neglected.
Paul Christiano: Yes, I think a lot of it’s great– I feel like there are lots of good things for people to do. I feel like people are mostly at the bottleneck just like people who have the relevant kinds of expertise and interests. This is one category where I feel people could go far and I’m excited to see how that goes.
---
(Not quoting people anymore)
That's all the knowledge I have on the matter.
But I'll just add that I'm quite skeptical about the suggestion that "we might imagine that our hopes of finding better carbon sequestration technologies after that dumbing point may plummet." It seems like it's unclear whether increased CO2 leads to e.g. a several IQ point drop. And then on top of that it's also not clear to me that, if it did that globally (which would definitely be a very big deal), that would cause a "plummeting" in our chances of finding some particular tech. (Though I guess it might.)
↑ comment by romeostevensit · 2019-10-04T06:48:16.116Z · LW(p) · GW(p)
I agree but the steel man (not sure actually intended) is a mean variance issue and whether you're introducing a more sensitive parameter. i.e. you get the mean you want using the new control variable but variance is now higher and you don't actually understand the new parameter space this puts you in.
↑ comment by kvas · 2019-11-06T11:14:50.518Z · LW(p) · GW(p)
If geoengineering approaches successfully counteract climate change, and it's cheaper to burn carbon and dim the sun than generate power a different way (or not use the power), then presumably civilization is better off burning carbon and dimming the sun.
AFAIK, the main arguments against solar radiation management (SRM) are:
1. High level of CO2 in the atmosphere creates other problems too (e.g. ocean acidification) but those problems are less urgent / impactful so we'll end up not caring about them if we implement SRM. Reducing CO2 emissions allows us to "do the right thing" using already existing political momentum.
2. Having the climate depend on SRM gives a lot of power to those in control of SRM and makes the civilization dependent on SRM. We are bad at global cooperation as is and having SRM to manage will put additional stress on that. This is a more fragile solution than reducing emissions.
It's certainly possible to argue against either of these points, especially introducing the assumption that humanity as a whole is close enough to a rational agent. My opinion is that geoengineering solutions lead to more fragility than reducing emissions and we would be better off avoiding them or at least doing something along the lines of carbon sequestration and not SRM. It also seems increasingly likely that we won't have that option. Our emission reduction efforts are too slow and once we hit +5ºC and beyond the option to "turn this off tomorrow" will look too attractive.
Replies from: Vaniver↑ comment by Vaniver · 2019-11-06T17:28:33.727Z · LW(p) · GW(p)
My opinion is that geoengineering solutions lead to more fragility than reducing emissions and we would be better off avoiding them or at least doing something along the lines of carbon sequestration and not SRM.
Sure, I think carbon sequestration is a solid approach as well (especially given that it's still net energy-producing to burn fossil fuels and sequester the resulting output as CO2 somewhere underground!), and am not familiar enough with the numbers to know if SRM is better or worse than sequestration. My core objection was that Russell's opinion of the NAS meeting wasn't "SRM has expected disasters or expected high costs that disqualify it", and instead it looked like that the NAS thought it was more important to be adversarial to fossil fuel interests than make the best engineering decision.
comment by habryka (habryka4) · 2019-10-23T21:33:22.889Z · LW(p) · GW(p)
Promoted to curated: This seems like it was a real conversation, and I also think it's particularly valuable for LessWrong to engage with more outside perspectives like the ones above.
I also in general want to encourage people to curate discussion and contributions that happen all around the web, and archive them in formats like this.
comment by Davidmanheim · 2019-10-04T06:53:59.169Z · LW(p) · GW(p)
I commented on the thread (after seeing this) in order to add a link to my paper that addresses Bengio's last argument;
@Yoshua Bengio I attempted to formalize this argument somewhat in a recent paper. I don't think the argument there is particularly airtight, but I think it provides a significantly stronger argument for why we should believe that interaction between optimizing systems is fundamentally hard.
https://www.mdpi.com/2504-2289/3/2/21/htm
Paper abstract: "An important challenge for safety in machine learning and artificial intelligence systems is a set of related failures involving specification gaming, reward hacking, fragility to distributional shifts, and Goodhart’s or Campbell’s law. This paper presents additional failure modes for interactions within multi-agent systems that are closely related. These multi-agent failure modes are more complex, more problematic, and less well understood than the single-agent case, and are also already occurring, largely unnoticed. After motivating the discussion with examples from poker-playing artificial intelligence (AI), the paper explains why these failure modes are in some senses unavoidable. Following this, the paper categorizes failure modes, provides definitions, and cites examples for each of the modes: accidental steering, coordination failures, adversarial misalignment, input spoofing and filtering, and goal co-option or direct hacking. The paper then discusses how extant literature on multi-agent AI fails to address these failure modes, and identifies work which may be useful for the mitigation of these failure modes."
comment by John_Maxwell (John_Maxwell_IV) · 2019-10-06T16:06:47.884Z · LW(p) · GW(p)
I think part of what may be going on here is that the approach to AI that Yann advocates happens to be one that is unusually amenable to alignment. Some discussion here:
https://www.lesswrong.com/posts/EMZeJ7vpfeF4GrWwm/self-supervised-learning-and-agi-safety [LW · GW]
comment by hamnox · 2020-12-31T14:15:50.934Z · LW(p) · GW(p)
A good example of crucial arguments, in the wild.
I'm not sure I like it. It looks like a lot of talking past each other. Very casually informative of different perspectives without much direct confrontation. Relatively good for an internet argument, but still not as productive as one might hope for between experts debating a serious topic. I'm glad for the information; I strongly value concretely knowing that sometimes arguments play out like this.
But I still don't like it.
(To be fair, this is comments on a facebook link post. I feel Ben misleads with technical truths when he describes this as an "actual debate" occurring in "a public space".)
Replies from: Benito↑ comment by Ben Pace (Benito) · 2020-12-31T19:59:30.131Z · LW(p) · GW(p)
Re the last line, it's public insofar as anyone can read it (you don't have to be friends with Yann LeCun to read it, you can read it logged out). Saying "actual debate" was intended somewhat as praise for the people involved having the conversation. I agree it was something like the mvp of a debate, but I think it is the first time I've ever seen these people really have this conversation, and the first sample gives the most information [LW · GW].
Can't imagine this post going in the book, but if people vote it in I'll make some effort to track down the participants and ask if they're willing to give legal permission for inclusion.
comment by Neel Nanda (neel-nanda-1) · 2020-12-05T09:36:25.506Z · LW(p) · GW(p)
I found this post valuable for better understanding the perspectives of AI experts who aren't concerned about alignment (my rough take is "they think the alignment problem will be easy, and that the control problem will be easy enough to patch any gaps in that"). And I've found this useful for updating my intuitions about worlds where the people working on TAI are not cautious enough about safety. It's helped update me towards thinking most of the problems come from worlds with subtle problems of alignment, and that people would notice obvious ones.
And I appreciate Ben writing this up - a Facebook thread is a terrible format for a public debate, and I would never have come across this otherwise!
comment by NatPhilosopher · 2019-10-10T20:52:11.459Z · LW(p) · GW(p)
If you actually want to have any good chance of settling the dispute, You need to settle it point by point. As it is I'm fairly sure that Yann and Stuart still disagree on the central point. and if you want to get any conclusion that is useful, you need some error bar on the likelihood is correct. Yann said that in his subjective opinion it is unlikely an AI will destroy the world, but has never said what that means. if it means there is only a 20% chance, then even in his opinion we have a problem. And since he is being paid millions to develop an AI, his subjective estimate may be subject to bias.
Here is a TruthSift diagram that solve both these problems: https://truthsift.com/graph/If+Artificial+General+Intelligence+is+Built-2C+there+will+be+a+significant+chance+it+will+kill+or+enslave+humanity+/550/0/-1/-1/0/0#lnkNameGraph
Feel free to add to it, or start another.
comment by Rob Bensinger (RobbBB) · 2020-12-14T00:58:23.163Z · LW(p) · GW(p)
May be useful to include in the review with some of the comments, or with a postmortem and analysis by Ben (or someone).
I don't think the discussion stands great on its own, but it may be helpful for:
- people familiar with AI alignment who want to better understand some human factors behind 'the field isn't coordinating or converging on safety'.
- people new to AI alignment who want to use the views of leaders in the field to help them orient.
comment by TurnTrout · 2021-01-12T04:01:01.087Z · LW(p) · GW(p)
Note 1: This review is also a top-level post [LW · GW].
Note 2: I think [LW · GW] that 'robust instrumentality' is a more apt name for 'instrumental convergence.' That said, for backwards compatibility, this comment often uses the latter.
In the summer of 2019, I was building up a corpus of basic reinforcement learning theory. I wandered through a sun-dappled Berkeley, my head in the clouds, my mind bent on a single ambition [LW · GW]: proving the existence of instrumental convergence.
Somehow.
I needed to find the right definitions first, and I couldn't even imagine what the final theorems would say. The fall crept up on me... and found my work incomplete.
Let me tell you: if there's ever been a time when I wished I'd been months ahead on my research agenda, it was September 26, 2019: the day when world-famous AI experts debated whether instrumental convergence was a thing, and whether we should worry about it.
The debate unfolded below the link-preview: an imposing robot staring the reader down, a title containing 'Terminator', a byline dismissive of AI risk:
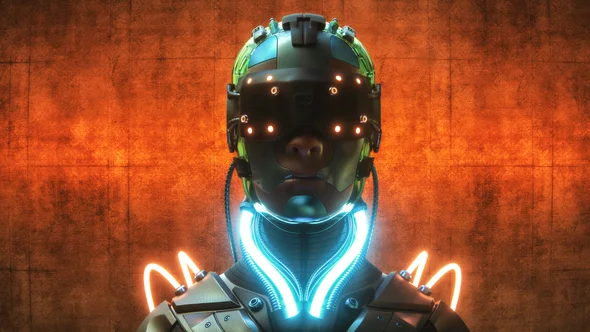
Don’t Fear the Terminator
"Artificial intelligence never needed to evolve, so it didn’t develop the survival instinct that leads to the impulse to dominate others."
The byline seemingly affirms the consequent: "evolution survival instinct" does not imply "no evolution no survival instinct." That said, the article raises at least one good point: we choose the AI's objective, and so why must that objective incentivize power-seeking?
I wanted to reach out, to say, "hey, here's a paper formalizing the question you're all confused by!" But it was too early.
Now, at least, I can say what I wanted to say back then:
This debate about instrumental convergence is really, really confused. I heavily annotated the play-by-play of the debate in a Google doc, mostly checking local validity of claims. (Most of this review's object-level content is in that document, by the way. Feel free to add comments of your own.)
This debate took place in the pre-theoretic era of instrumental convergence. Over the last year and a half, I've become a lot less confused about instrumental convergence. I think my formalisms [LW · GW] provide great abstractions for understanding "instrumental convergence" and "power-seeking." I think that this debate suffers for lack of formal grounding, and I wouldn't dream of introducing someone to these concepts via this debate.
While the debate is clearly historically important, I don't think it belongs in the LessWrong review. I don't think people significantly changed their minds, I don't think that the debate was particularly illuminating, and I don't think it contains the philosophical insight I would expect from a LessWrong review-level essay.
Rob Bensinger's nomination reads [LW(p) · GW(p)]:
May be useful to include in the review with some of the comments, or with a postmortem and analysis by Ben (or someone).
I don't think the discussion stands great on its own, but it may be helpful for:
- people familiar with AI alignment who want to better understand some human factors behind 'the field isn't coordinating or converging on safety'.
- people new to AI alignment who want to use the views of leaders in the field to help them orient.
I certainly agree with Rob's first bullet point. The debate did show us what certain famous AI researchers thought about instrumental convergence, circa 2019.
However, I disagree with the second bullet point: reading this debate may disorient a newcomer! While I often found myself agreeing with Russell and Bengio, while LeCun and Zador sometimes made good points, confusion hangs thick in the air: no one realizes that, with respect to a fixed task environment (representing the real world) and their beliefs about what kind of objective function the agent may have, they should be debating the probability that seeking power is optimal (or that power-seeking behavior is learned, depending on your threat model).
Absent such an understanding, the debate is needlessly ungrounded and informal. Absent such an understanding, we see reasoning like this:
Yann LeCun: ... instrumental subgoals are much weaker drives of behavior than hardwired objectives. Else, how could one explain the lack of domination behavior in non-social animals, such as orangutans.
I'm glad that this debate happened, but I think it monkeys around too much to be included in the LessWrong 2019 review.
Replies from: ricraz↑ comment by Richard_Ngo (ricraz) · 2021-04-30T09:47:55.697Z · LW(p) · GW(p)
Yann LeCun: ... instrumental subgoals are much weaker drives of behavior than hardwired objectives. Else, how could one explain the lack of domination behavior in non-social animals, such as orangutans.
What's your specific critique of this? I think it's an interesting and insightful point.
Replies from: TurnTrout↑ comment by TurnTrout · 2021-04-30T15:14:24.849Z · LW(p) · GW(p)
LeCun claims too much. It's true that the case of animals like orangutans points to a class of cognitive architectures which seemingly don't prioritize power-seeking. It's true that this is some evidence against power-seeking behavior being common amongst relevant cognitive architectures. However, it doesn't show that instrumental subgoals are much weaker drives of behavior than hardwired objectives.
One reading of this "drives of behavior" claim is that it has to be tautological; by definition, instrumental subgoals are always in service of the (hardwired) objective. I assume that LeCun is instead discussing "all else equal, will statistical instrumental tendencies ('instrumental convergence') be more predictive of AI behavior than its specific objective function?".
But "instrumental subgoals are much weaker drives of behavior than hardwired objectives" is not the only possible explanation of "the lack of domination behavior in non-social animals"! Maybe the orangutans aren't robust to scale. Maybe orangutans do implement non power-seeking cognition, but maybe their cognitive architecture will be hard or unlikely for us to reproduce in a machine - maybe the distribution of TAI cognitive architectures we should expect, is far different from what orangutans are like.
I do agree that there's a very good point in the neighborhood of the quoted argument. My steelman of this would be:
Some animals, like humans, seem to have power-seeking drives. Other animals, like orangutans, do not. Therefore, it's possible to design agents of some intelligence which do not seek power. Obviously, we will be trying to design agents which do not seek power. Why, then, should we expect such agents to be more like humans than like orangutans?
(This is loose for a different reason, in that it presupooses a single relevant axis of variation between humans and orangutans. Is a personal computer more like a human, or more like an orangutan? But set that aside for the moment.)
I think he's overselling the evidence. However, on reflection, I wouldn't pick out the point for such strong ridicule.
Replies from: Lukas_Gloor↑ comment by Lukas_Gloor · 2021-05-02T09:30:06.650Z · LW(p) · GW(p)
I feel like you can turn this point upside down. Even among primates that seem unusually docile, like orang utans, male-male competition can get violent and occasionally ends in death. Isn't that evidence that power-seeking is hard to weed out? And why wouldn't it be in an evolved species that isn't eusocial or otherwise genetically weird?
comment by emmab · 2019-10-23T21:54:15.194Z · LW(p) · GW(p)
5. A second machine, designed solely to neutralize an evil super-intelligent machine will win every time, if given similar amounts of computing resources (because specialized machines always beat general ones).
This implies you have some resource you didn't fully imbue to the first AI, that you still have available to imbue to the second. What is that resource?
Replies from: ErickBall↑ comment by ErickBall · 2019-10-24T18:03:16.508Z · LW(p) · GW(p)
The claim that specialized machines always beat general ones seems questionable in the context of an AGI. Actually, I'm not sure I understand the claim in the first place. Maybe he means by analogy to a supervised learning system--if you take a network trained to recognize cat pictures, and also train it to recognize dog pictures, then given a fixed number of parameters you can expect it will get less good at recognizing cat pictures.
comment by Ramiro P. (ramiro-p) · 2019-10-22T17:14:03.365Z · LW(p) · GW(p)
I find LeCun's insistence on the analogy with legal systems particularly interesting, because they remind me more Russell's proposal of "uncertain objectives" than the "maximize objective function" paradigm. At least in liberal societies, we don't have a definite set of principles and values that people would agree to follow - instead, we aim at principles that guarantee an environment where any reasonable person can reasonably optimize for something like their own comprehensive doctrine.
However, the remarkable disanalogy is that, even if social practices change and clever agents adapt faster than law can evolve (as Goodhart remarks), the difference is not so great as with the technological pace.
comment by romeostevensit · 2019-10-04T06:51:34.963Z · LW(p) · GW(p)
[internal screaming intensifies]
Can we somehow make Metaphors We Live By mandatory reading for these people? Reference class tennis plus analogical reasoning is only comforting in the sense that maybe someone stupid enough to be arguing that way isn't smart enough to build anything dangerous.
Replies from: Vaniver↑ comment by Vaniver · 2019-10-04T16:29:34.698Z · LW(p) · GW(p)
[Context: the parent comment was originally posted to the Alignment Forum, and was moved to only be visible on LW.]
One of my hopes for the Alignment Forum, and to a much lesser extent LessWrong, is that we manage to be a place where everyone relevant to AI alignment gets value from discussing their work. There's many obstacles to that, but one of the ones that I've been thinking a lot recently is that pointing at foundational obstacles can look a lot like low-effort criticism.
That is, I think there's a valid objection here of the form "these people are using reasoning style A, but I think this problem calls for reasoning style B because of considerations C, D, and E." But the inferential distance [LW · GW] here is actually quite long, and it's much easier to point out "I am not convinced by this because of <quick pointer>" than it is to actually get the other person to agree that they were making a mistake. And beyond that, there's the version that scores points off an ingroup/outgroup divide and a different version that tries to convert the other party.
My sense is that lots of technical AI safety agendas look to each other like they have foundational obstacles, of the sort that means having more than one agenda happy at the Alignment Forum means everyone needs to not do this sort of sniping, while still having high-effort places to discuss those obstacles. (That is, if we think CIRL can't handle corrigibility, having a place for 'obstacles to CIRL' where that's discussed makes sense, but bringing it up at every post on CIRL might not.)
Replies from: romeostevensit↑ comment by romeostevensit · 2019-10-04T17:01:49.523Z · LW(p) · GW(p)
whoops, I agree with the heuristic and didn't actually mean for it to go to AF instead of LW. Hadn't paid too much attention to how crossposting works until now.
Replies from: Viliam↑ comment by Viliam · 2019-10-05T16:55:01.768Z · LW(p) · GW(p)
I agree with the wisdom of removing the comment from AF, but I admit I was also screaming internally while reading the article.
(From a personal perspective, ignoring the issue of artificial intelligent and existential risks, this was an interesting look outside the LW bubble. Like, the more time passed since when I read the Sequences, the more the ideas explained there seem obvious to me, to the point where I start to wonder why was I even impressed by reading the text. But then I listen to someone from outside the bubble, and scream internally as I watch them doing the "obvious" mistakes -- typically some variant of confusing a map with the territory -- and then I realize the "obvious" things are actually not that obvious, even among highly intelligent people who talk about topics they care about. Afterwards, I just silently weep about the state of the human race.)
It hurts to read a sophisticated version of "humans are too smart to make mistakes". But pointing it out without crossing the entire inferential distance is not really helpful. :(
Replies from: None↑ comment by [deleted] · 2019-10-05T23:25:19.524Z · LW(p) · GW(p)
Meta: This is in response to both this and comments further up the chain regarding the level of the debate.
It's worth noting that, at least from my perspective, Bengio, who's definitely not in the LW bubble, made good points throughout and did a good job of moderating.
On the other hand, Russell, obviously more partial to the LW consensus view, threw out some "zingers" early on (such as the following one) that didn't derail the debate but easily could've.
Thanks for clearing that up - so 2+2 is not equal to 4, because if the 2 were a 3, the answer wouldn't be 4? I simply pointed out that in the MDP as I defined it, switching off the human is the optimal solution, despite the fact that we didn't put in any emotions of power, domination, hate, testosterone, etc etc. And your solution seems, well, frankly terrifying, although I suppose the NRA would approve.
comment by Yitz (yitz) · 2022-07-14T16:13:37.901Z · LW(p) · GW(p)
Has LeCun changed his mind on any of these points since this debate?
comment by Nick Nolan (nick-nolan) · 2019-11-03T10:31:30.242Z · LW(p) · GW(p)
I have started to see the Instrumental convergence problem as a part of human-to-human aliment problem.
E. Glen Weyl in "Why I Am Not A Technocrat"
Similarly, if we want to have AIs that can play a productive role in society, our goal should not be exclusively or even primarily to align them with the goals of their creators or the narrow rationalist community interested in the AIAP. Instead it should be to create a set of social institutions that ensures that the ability of any narrow oligarchy or small number of intelligences like a friendly AI cannot hold extremely disproportionate power. The institutions likely to achieve this are precisely the same sorts of institutions necessary to constrain extreme capitalist or state power.
[....]
A primary goal of AI design should be not just alignment, but legibility, to ensure that the humans interacting with the AI know its goals and failure modes, allowing critique, reuse, constraint etc.
Weyl's technocrat critique is valid in the personal level. It did hit me hard. I have tendency to drift from important messy problems into interesting but difficult problems that might have formal solutions (Is there name for this cognitive bias?) LessWrong community supports this bias drift.
I argue that Instrumental convergence and AI aliment problems are framed incorrectly to make them more interesting to think and easier to solve.
New framing: Intelligent agents (human and nonhuman) aligning constantly to each other. Solving instrumental convergence is equal to solving the society. We can't solve it once and for all, but we can create process and institutions that adjust and manage problems that arise.
Typical scenarios are superpower+superintelligence, ruling party + superintelligence, Zuck+Superintelligence, Chairman Xi + Superintelligence, Alphabet board of directors + Superintelligence.
comment by Evan R. Murphy · 2022-07-16T06:56:35.058Z · LW(p) · GW(p)
It would be great to have a summary or distillation of this conversation.
comment by teradimich · 2019-10-06T03:33:05.639Z · LW(p) · GW(p)
It seems Russell does not agree with what is considered an LW consensus. From ’Architects of Intelligence The truth about AI from the people building it’:
When [the first AGI is created], it’s not going to be a single finishing line that we cross. It’s going to be along several dimensions.
[...]
I do think that I’m an optimist. I think there’s a long way to go. We are just scratching the surface of this control problem, but the first scratching seems to be productive, and so I’m reasonably optimistic that there is a path of AI development that leads us to what we might describe as “provably beneficial AI systems.”
Replies from: steve2152
↑ comment by Steven Byrnes (steve2152) · 2019-10-06T11:16:52.012Z · LW(p) · GW(p)
Can you be more specific what you think the LW consensus is, that you're referring to? Recursive self-improvement and pessimism about AI existential risk? Or something else?
comment by Charles Gaston (charles-gaston) · 2023-04-03T16:31:58.708Z · LW(p) · GW(p)
Dr. Yoshua Bengio wrote:
And there is another issue which was not much discussed (although the article does talk about the short-term risks of military uses of AI etc), and which concerns me: humans can easily do stupid things. So even if there are ways to mitigate the possibility of rogue AIs due to value misalignment, how can we guarantee that no single human will act stupidly (more likely, greedily for their own power) and unleash dangerous AIs in the world?
My take:
I am not sure we want to live in a world in which freedom is reduced to the extent that warrants or insures that the possibility that humans might do stupid things is non existent. It seems to me that there is a thick and straight line of philosophical knowledge, spanning at least from J.S Mill to the tradition of existentialists, and which has overall fought against dogmatism (in favor of humanism). I "dare" evoking philosophy in the present debate, as it seems to me that all "non scientific" concepts debated here are of a philosophical nature. By "non scientific" I mean "non empirical".
Science, preferably empirical science --including information or computing science-- remains a privileged source of information on which such important topics should be grounded. For instance, "the fear that an artificially intelligent system could, as a result of a very problematic training process, could suddenly develop its own sense of will, and that this sense of will could be opposed to that of its 'Creator'", has, so far as I know, no empirical justification.
To turn this conjecture into a scientific fact, science, computing science, i.e. "you", should aim at creating a toy model in which the goal would be that such state be achieved. You see?
In other words, the question we should ask ourselves, is not "How can we make sure that no humans will do stupid things", but rather, provided that we know that humans will do stupid things, how will your system react?
Just like social entities (governments etc) should count on the services of people capable of hacking them, I think it is not unreasonable to conjecture that an entity which operate a LLM should invest (in R&D) in trying to break a toy version, trying to elicit what you seem to fear.
For speculations and conjectures will only take you "so far".
CG