Posts
Comments
Tamay from Epoch AI here.
We made a mistake in not being more transparent about OpenAI's involvement. We were restricted from disclosing the partnership until around the time o3 launched, and in hindsight we should have negotiated harder for the ability to be transparent to the benchmark contributors as soon as possible. Our contract specifically prevented us from disclosing information about the funding source and the fact that OpenAI has data access to much but not all of the dataset. We own this error and are committed to doing better in the future.
For future collaborations, we will strive to improve transparency wherever possible, ensuring contributors have clearer information about funding sources, data access, and usage purposes at the outset. While we did communicate that we received lab funding to some mathematicians, we didn't do this systematically and did not name the lab we worked with. This inconsistent communication was a mistake. We should have pushed harder for the ability to be transparent about this partnership from the start, particularly with the mathematicians creating the problems.
Getting permission to disclose OpenAI's involvement only around the o3 launch wasn't good enough. Our mathematicians deserved to know who might have access to their work. Even though we were contractually limited in what we could say, we should have made transparency with our contributors a non-negotiable part of our agreement with OpenAI.
Regarding training usage: We acknowledge that OpenAI does have access to a large fraction of FrontierMath problems and solutions, with the exception of a unseen-by-OpenAI hold-out set that enables us to independently verify model capabilities. However, we have a verbal agreement that these materials will not be used in model training.
Relevant OpenAI employees’ public communications have described FrontierMath as a 'strongly held out' evaluation set. While this public positioning aligns with our understanding, I would also emphasize more broadly that labs benefit greatly from having truly uncontaminated test sets.
OpenAI has also been fully supportive of our decision to maintain a separate, unseen holdout set—an extra safeguard to prevent overfitting and ensure accurate progress measurement. From day one, FrontierMath was conceived and presented as an evaluation tool, and we believe these arrangements reflect that purpose.
[Edit: Clarified OpenAI's data access - they do not have access to a separate holdout set that serves as an additional safeguard for independent verification.]
Short version: The claim that AI automation of software engineering will erase NVIDIA's software advantage misunderstands that as markets expand, the rewards for further software improvements grow substantially. While AI may lower the cost of matching existing software capabilities, overall software project costs are likely to keep increasing as returns on optimization rise. Matching the frontier of performance in the future will still be expensive and technically challenging, and access to AI does not necessarily equalize production costs or eliminate NVIDIA's moat.
I often see the argument that, since NVIDIA is largely software, when AI automates software, NVIDIA will have no moat, and therefore NVIDIA a bad AI bet. The argument goes something like: AI drives down the cost of software, so the barriers to entry will be much lower. Competitors can "hire" AI to generate the required software by, for example, tasking LLMs with porting application-level code into appropriate low-level instructions, which would eliminate NVIDIA's competitive advantage stemming from CUDA.
However, while the cost of matching existing software capabilities will decline, the overall costs of software projects are likely to continue increasing, as is the usual pattern. This is because, with software, the returns to optimization increase with the size of the addressable market. As the market expands, companies have greater incentives to invest intensely because even small improvements in performance or efficiency can yield substantial overall benefits. These improvements impact a large number of users, and the costs are amortized across this extensive user base.
Consider web browsers and operating systems: while matching 2000s-era capabilities now takes >1000x fewer developer hours using modern frameworks, the investments that Google makes in Chrome and Microsoft in Windows vastly exceed what tech companies spent in the 2000s. Similarly, as AI becomes a larger part of the overall economy, I expect the investments needed for state-of-the-art GPU firmware and libraries to be greater than those today.
When software development is mostly AI-driven, there will be opportunities to optimize software with more spending, such as by spending on AI inference, building better scaffolding, or producing better ways of testing and verifying potential improvements. This just seems to match our understanding of inference scaling for other complex reasoning tasks, such as programming or mathematics.
It’s also unlikely that the relative cost of producing the same software will be much more equalized; that anyone can hire the same "AI” to do the engineering. Just having access to the raw models is often not sufficient for getting state-of-the-art results (good luck matching AlphaProof's IMO performance with the Gemini API).
To be clear, I am personally not too optimistic about NVIDIA's long term future. There are good reasons to expect their moat won't persist:
- Dethroning NVIDIA is now a trillion dollar proposition, and their key customers are all trying to produce GPU substitutes
- Rapid technological progress tends to erode competitive advantages by enabling substitute technologies
- NVIDIA has had issues adopting new technologies, such as CoWoS-L packaging, and therrefore appears less competent in staying ahead of its competition.
My claim is narrower: the argument that "when AI can automate software engineering, companies whose moat involves software will be outcompeted" seems incorrect.
My guess is that compute scaling is probably more important when looking just at pre-training and upstream performance. When looking innovations both pre- and post-training and measures of downstream performance, the relative contributions are probably roughly evenly matched.
Compute for training runs is increasing at a rate of around 4-5x/year, which amounts to a doubling every 5-6 months, rather than every 10 months. This is what we found in the 2022 paper, and something we recently confirmed using 3x more data up to today.
Algorithms and training techniques for language models seem to improve at a rate that amounts to a doubling of 'effective compute' about doubling every 8 months, though, like our work on vision, this estimate has large errors bars. Still, it's likely to be slower than the 5-6 month doubling time for actual compute. These estimates suggest that compute scaling has been responsible for perhaps 2/3rds of performance gains over the 2014-2023 period, with algorithms + insights about optimal scaling + better data, etc. explaining the remaining 1/3rd.
The estimates mentioned only account for the performance gains from pre-training, and do not consider the impact of post-training innovations. Some key post-training techniques, such as prompting, scaffolding, and finetuning, have been estimated to provide performance improvements ranging from 2 to 50 times in units of compute-equivalents, as shown in the plot below. However, these estimates vary substantially depending on the specific technique and domain, and are somewhat unreliable due to their scale-dependence.
Naively adding these up with the estimates from the progress from pre-training suggests that compute scaling likely still acounts for most of the performance gains, though it looks more evenly matched.
... and that was just in vision nets. I haven't seen careful analysis of LLMs (probably because they're newer, so harder to fit a trend), but eyeballing it... Chinchilla by itself must have been a factor-of-4 compute-equivalent improvement at least.
Incidentally, I looked into the claim about Chinchilla scaling. It turns out that Chinchilla was actually more like a factor 1.6 to 2 in compute-equivalent gain over Kaplan at the scale of models today (at least if you use the version of the scaling law that corrects a mistake the Chinchilla paper made when doing the estimation).
Sebastian Borgeaud, one of the lead authors of the Chinchilla scaling paper, admits there was a bug in their code. https://twitter.com/borgeaud_s/status/1780988694163321250
Claim that the Chinchilla paper calculated the implied scaling laws incorrectly. Yes, it seems entirely plausible that there was a mistake, tons of huge training runs relied on the incorrect result, and only now did someone realize this. Why do you ask?
I'm interested. What bets would you offer?
There is an insightful literature that documents and tries to explain why large incumbent tech firms fail to invest appropriately in disruptive technologies, even when they played an important role in its invention. I speculatively think this sheds some light on why we see new firms such as OpenAI rather than incumbents such as Google and Meta leading the deployment of recent innovations in AI, notably LLMs.
Disruptive technologies—technologies that initially fail to satisfy existing demands but later surpass the dominant technology—are often underinvested in by incumbents, even when these incumbents played a major role in their invention. Henderson and Clark, 1990 discuss examples of this phenomenon, such as Xerox's failure to exploit their technology and transition from larger to smaller copiers:
Xerox, the pioneer of plain-paper copiers, was confronted in the mid-1970s with competitors offering copiers that were much smaller and more reliable than the traditional product. The new products required little new scientific or engineering knowledge, but despite the fact that Xerox had invented the core technologies and had enormous experience in the industry, it took the company almost eight years of missteps and false starts to introduce a competitive product into the market. In that time Xerox lost half of its market share and suffered serious financial problems
and RCA’s failure to embrace the small transistorized radio during the 1950s:
In the mid-1950s engineers at RCA's corporate research and development center developed a prototype of a portable, transistorized radio receiver. The new product used technology in which RCA was accomplished (transistors, radio circuits, speakers, tuning devices), but RCA saw little reason to pursue such an apparently inferior technology. In contrast, Sony, a small, relatively new company, used the small transistorized radio to gain entry into the US, market. Even after Sony's success was apparent, RCA remained a follower in the market as Sony introduced successive models with improved sound quality and FM capability. The irony of the situation was not lost on the R&D engineers: for many years Sony's radios were produced with technology licensed from RCA, yet RCA had great difficulty matching Sony's product in the marketplace
A few explanations of this "Innovator's curse" are given in the literature:
- Christensen (1997) suggests this is due to, among other things:
- Incumbents focus on innovations that address existing customer needs rather than serving small markets. Customer bases usually ask for incremental improvements rather than radical innovations.
- Disruptive products are simpler and cheaper; they generally promise lower margins, not greater profits
- Incumbents’ most important customers usually don’t want radically new technologies, as they can’t immediately use these
- Reinganum (1983) shows that under conditions of uncertainty, incumbent monopolists will rationally invest less in innovation than entrants will, for fear of cannibalizing the stream of rents from their existing products
- Leonard-Barton (1992) suggests that the same competencies that have driven incumbent’s commercial success may produce ‘competency traps’ (engrained habits, procedures, equipment or expertise that make change difficult); see also Henderson, 2006
- Henderson, 1993 highlights that entrants have greater strategic incentives to invest in radical innovation, and incumbents fall prey to inertia and complacency
After skimming a few papers on this, I’m inclined to draw an analogue here for AI: Google produced the Transformer; labs at Google, Meta, and Microsoft, have long been key players in AI research, and yet, the creation of explicitly disruptive LLM products that aim to do much more than existing technologies has been led mostly by relative new-comers (such as OpenAI, Anthropic, and Cohere for LLMs and StabilityAI for generative image models).
The same literature also suggests how to avoid the "innovator curse", such as through establishing independent sub-organizations focused on disruptive innovations (see Christensen ,1997 and Christensen, 2003), which is clearly what companies like Google have done, as its AI labs have a large degree of independence. And yet this seems not to seem to have been sufficient to establish the dominance of these firms when it comes to the frontiers of LLMs and the like.
If the data is low-quality and easily distinguishable from human-generated text, it should be simple to train a classifier to spot LM-generated text and exclude this from the training set. If it's not possible to distinguish, then it should be high-enough quality so that including it is not a problem.
ETA: As people point out below, this comment was glib and glosses over some key details; I don't endorse this take anymore.
Good question. Some thoughts on why do this:
- Our results suggest we won't be caught off-guard by highly capable models that were trained for years in secret, which seems strategically relevant for those concerned with risks
- We looked whether there was any 'alpha' in these results by investigating the training durations of ML training runs, and found that models are typically trained for durations that aren't far off from what our analysis suggests might be optimal (see a snapshot of the data here)
- It independently seems highly likely that large training runs would already be optimized in this dimension, which further suggests that this has little to no action-relevance for advancing the frontier
I'm not sure what you mean; I'm not looking at log-odds. Maybe the correlation is an artefact from noise being amplified in log-space (I'm not sure), but it's not obvious to me that this isn't the correct way to analyse the data.
Thanks! At least for Gopher, if you look at correlations between reductions in log-error (which I think is the scaling laws literature suggests would be the more natural framing) you find a more tighter relationship, particularly when looking at the relatively smaller models.
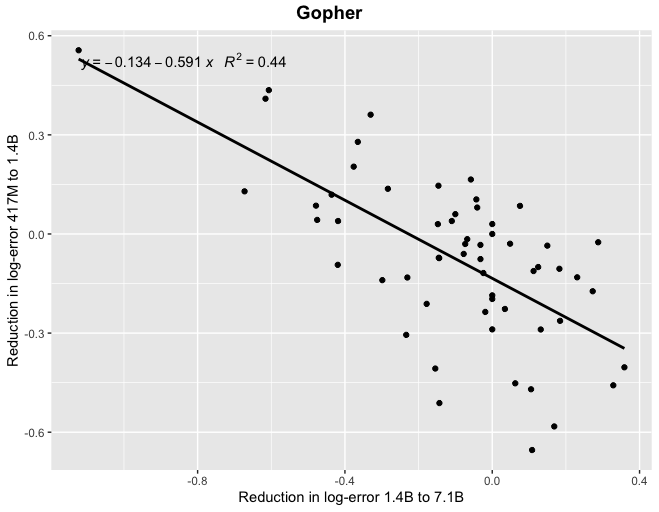
Thanks, though I was hoping for something like a Google Sheet containing the data.
This is super interesting. Are you able to share the underlying data?
It is unless it's clear that a side that made a mistake in entering a lopsided bet. I guess the rule-of-thumb is to follow big bets (which tends to be less clearly lopsided) or bets made by two people whose judgment you trust.
Are you thinking of requiring each party to accept bets on either side?
Being forced to bet both sides could ensure honesty, assuming they haven't found other bets on the same or highly correlated outcomes they can use for arbitrage.
Yes. Good point.
And including from other parties, or only with each other?
I was thinking that betting would be restricted to the initial two parties (i.e. A and B), but I can imagine an alternative in which it's unrestricted.
You could imagine one party was betting at odds they consider very favourable to them, and the other party betting at odds they consider only slightly favourable, based on their respective beliefs. Then, even if they don't change their credences, one party has more room to move their odds towards their own true credences, and so drag the average towards it, and take the intermediate payments,
Sorry, I'm confused. Isn't the 'problem' that the bettor who takes a relatively more favourable odds has higher expected returns a problem with betting in general?
We also propose betting using a mechanism that mitigates some of these issues:
Since we recognize that betting incentives can be weak over long time-horizons, we are also offering the option of employing Tamay’s recently described betting procedure in which we would enter a series of repeated 2-year contracts until the resolution date.
Here’s a rough description of an idea for a betting procedure that enables people who disagree about long-term questions to make bets, despite not wanting to commit to waiting until the long-term questions are resolved.
Suppose person A and person B disagree about whether P, but can’t find any clear concrete disagreements related to this question that can be decided soon. Since they want to bet on things that pay out soon (for concreteness say they only want to bet on things that can pay out within 5 years), they don’t end up betting on anything.
What they can do is they could agree to bet on P, and enter into a contract (or a good-faith agreement) that requires them to, after a period of 5 years, report their true odds about P. The contract would then enable either bettor to unanimously back out of the bet, at which point the payouts would be distributed according to the difference of the odds they agreed to and the average of the odds that they currently report. In other words, the bettor who was closer to the consensus after 5 years is paid out in proportion to how much closer they were.
To ensure that bettors approximately truthfully report their odds about P after the horizon of 5 years, the contract requires A and B to report their odds to a trusted intermediary (who announces these odds simultaneously), and requires either party to accept any follow-up bets at (some function of) these reported credences.
Bettors might agree ahead of time to the range of acceptable follow-up bet sizes, though importantly, follow-up bet sizes need to be expected to be relatively large (say, a non-trivial fraction of the existing bets) to ensure that bettors have an incentive to report something close to their true beliefs.
Follow-up bets could be revisited in the same way after another 5 years, and this would continue until P resolves, or until the betters settle. However, because bettors are required to take follow-up bets, they also have an incentive to develop accurate beliefs about P so we might expect disagreements to usually be resolved short of when P resolves. They furthermore have an incentive to arrive at a consensus if they want to avoid making follow-up bets.
On this mechanism, bettors know that they can expect to fairly resolve their bets on a short horizon, as each will have an incentive to end the bet according to their consensus-view of who was closer to the truth. Hence, bettors would be keen to bet with each-other about P if they think that they’re directionally right, even when they don't want to wait until P completely is decided.
Thanks!
Could you make another graph like Fig 4 but showing projected cost, using Moore's law to estimate cost? The cost is going to be a lot, right?
Good idea. I might do this when I get the time—will let you know!
Four months later, the US is seeing a steady 7-day average of 50k to 60k new cases per day. This is a factor of 4 or 5 less than the number of daily new cases that were observed over the December-January third wave period. It seems therefore that one (the?) core prediction of this post, namely, that we'd see a fourth wave sometime between March and May that would be as bad or worse than the third wave, turned out to be badly wrong.
Zvi's post is long, so let me quote the sections where he makes this prediction:
Instead of that being the final peak and things only improving after that, we now face a potential fourth wave, likely cresting between March and May, that could be sufficiently powerful to substantially overshoot herd immunity.
and,
If the 65% number is accurate, however, we are talking about the strain doubling each week. A dramatic fourth wave is on its way. Right now it is the final week of December. We have to assume the strain is already here. Each infection now is about a million by mid-May, six million by end of May, full herd immunity overshoot and game over by mid-July, minus whatever progress we make in reducing spread between now and then, including through acquired immunity.
It seems troubling that one of the most upvoted COVID-19 post on LessWrong is one that argued for a prediction that I think we should score really poorly. This might be an important counterpoint to the narrative that rationalists "basically got everything about COVID-19 right"*.
I think GPT-3 is the trigger for 100x larger projects at Google, Facebook and the like, with timelines measured in months.
My impression is that this prediction has turned out to be mistaken (though it's kind of hard to say because "measured in months" is pretty ambiguous.) There have been models with many-fold the number of parameters (notably one by Google*) but it's clear that 9 months after this post, there haven't been publicised efforts that use close to 100x the amount of compute of GPT-3. I'm curious to know whether and how the author (or others who agreed with the post) have changed their mind about the overhang and related hypotheses recently, in light of some of this evidence failing to pan out the way the author predicted.
Great work! It seems like this could enable lots of useful applications. One thing in particular that I'm excited about is how this can be used to make forecasting more decision-relevant. For example, one type of application that comes to mind in particular is a conditional prediction market where conditions are continuous rather than discrete (eg. "what is GDP next year if interest rate is set to r?", "what is Sierra Leone's GDP in ten years if bednet spending is x?").
If research into general-purpose systems stops producing impressive progress, and the application of ML in specialised domains becomes more profitable, we'd soon see much more investment in AI labs that are explicitly application-focused rather than basic-research focused.