Transformative AGI by 2043 is <1% likely
post by Ted Sanders (ted-sanders) · 2023-06-06T17:36:48.296Z · LW · GW · 117 commentsThis is a link post for https://arxiv.org/abs/2306.02519
Contents
Abstract Executive summary Event Forecast For details, read the full paper. About the authors Ari Allyn-Feuer Areas of expertise Track record of forecasting Ted Sanders Areas of expertise Track record of forecasting Discussion None 117 comments
(Crossposted [EA · GW]to the EA forum)
Abstract
The linked paper is our submission to the Open Philanthropy AI Worldviews Contest. In it, we estimate the likelihood of transformative artificial general intelligence (AGI) by 2043 and find it to be <1%.
Specifically, we argue:
- The bar is high: AGI as defined by the contest—something like AI that can perform nearly all valuable tasks at human cost or less—which we will call transformative AGI is a much higher bar than merely massive progress in AI, or even the unambiguous attainment of expensive superhuman AGI or cheap but uneven AGI.
- Many steps are needed: The probability of transformative AGI by 2043 can be decomposed as the joint probability of a number of necessary steps, which we group into categories of software, hardware, and sociopolitical factors.
- No step is guaranteed: For each step, we estimate a probability of success by 2043,
conditional on prior steps being achieved. Many steps are quite constrained by the short timeline, and our estimates range from 16% to 95%. - Therefore, the odds are low: Multiplying the cascading conditional probabilities together, we estimate that transformative AGI by 2043 is 0.4% likely. Reaching >10% seems to require probabilities that feel unreasonably high, and even 3% seems unlikely.
Thoughtfully applying the cascading conditional probability approach to this question yields lower probability values than is often supposed. This framework helps enumerate the many future scenarios where humanity makes partial but incomplete progress toward transformative AGI.
Executive summary
For AGI to do most human work for <$25/hr by 2043, many things must happen.
We forecast cascading conditional probabilities for 10 necessary events, and find they multiply to an overall likelihood of 0.4%:
Event | Forecastby 2043 or TAGI, |
We invent algorithms for transformative AGI | 60% |
We invent a way for AGIs to learn faster than humans | 40% |
AGI inference costs drop below $25/hr (per human equivalent) | 16% |
We invent and scale cheap, quality robots | 60% |
We massively scale production of chips and power | 46% |
We avoid derailment by human regulation | 70% |
We avoid derailment by AI-caused delay | 90% |
We avoid derailment from wars (e.g., China invades Taiwan) | 70% |
We avoid derailment from pandemics | 90% |
We avoid derailment from severe depressions | 95% |
Joint odds | 0.4% |
If you think our estimates are pessimistic, feel free to substitute your own here. You’ll find it difficult to arrive at odds above 10%.
Of course, the difficulty is by construction. Any framework that multiplies ten probabilities together is almost fated to produce low odds.
So a good skeptic must ask: Is our framework fair?
There are two possible errors to beware of:
- Did we neglect possible parallel paths to transformative AGI?
- Did we hew toward unconditional probabilities rather than fully conditional probabilities?
We believe we are innocent of both sins.
Regarding failing to model parallel disjunctive paths:
- We have chosen generic steps that don’t make rigid assumptions about the particular algorithms, requirements, or timelines of AGI technology
- One opinionated claim we do make is that transformative AGI by 2043 will almost certainly be run on semiconductor transistors powered by electricity and built in capital-intensive fabs, and we spend many pages justifying this belief
Regarding failing to really grapple with conditional probabilities:
- Our conditional probabilities are, in some cases, quite different from our unconditional probabilities. In particular, we assume that a world on track to transformative AGI will…
- Construct semiconductor fabs and power plants at a far faster pace than today (our unconditional probability is substantially lower)
- Have invented very cheap and efficient chips by today’s standards (our unconditional probability is substantially lower)
- Have higher risks of disruption by regulation
- Have higher risks of disruption by war
- Have lower risks of disruption by natural pandemic
- Have higher risks of disruption by engineered pandemic
Therefore, for the reasons above—namely, that transformative AGI is a very high bar (far higher than “mere” AGI) and many uncertain events must jointly occur—we are persuaded that the likelihood of transformative AGI by 2043 is <1%, a much lower number than we otherwise intuit. We nonetheless anticipate stunning advancements in AI over the next 20 years, and forecast substantially higher likelihoods of transformative AGI beyond 2043.
For details, read the full paper.
About the authors
This essay is jointly authored by Ari Allyn-Feuer and Ted Sanders. Below, we share our areas of expertise and track records of forecasting. Of course, credentials are no guarantee of accuracy. We share them not to appeal to our authority (plenty of experts are wrong), but to suggest that if it sounds like we’ve said something obviously wrong, it may merit a second look (or at least a compassionate understanding that not every argument can be explicitly addressed in an essay trying not to become a book).
Ari Allyn-Feuer
Areas of expertise
I am a decent expert in the complexity of biology and using computers to understand biology.
- I earned a Ph.D. in Bioinformatics at the University of Michigan, where I spent years using ML methods to model the relationships between the genome, epigenome, and cellular and organismal functions. At graduation I had offers to work in the AI departments of three large pharmaceutical and biotechnology companies, plus a biological software company.
- I have spent the last five years as an AI Engineer, later Product Manager, now Director of AI Product, in the AI department of GSK, an industry-leading AI group which uses cutting edge methods and hardware (including Cerebras units and work with quantum computing), is connected with leading academics in AI and the epigenome, and is particularly engaged in reinforcement learning research.
Track record of forecasting
While I don’t have Ted’s explicit formal credentials as a forecaster, I’ve issued some pretty important public correctives of then-dominant narratives:
- I said in print on January 24, 2020 that due to its observed properties, the then-unnamed novel coronavirus spreading in Wuhan, China, had a significant chance of promptly going pandemic and killing tens of millions of humans. It subsequently did.
- I said in print in June 2020 that it was an odds-on favorite for mRNA and adenovirus COVID-19 vaccines to prove highly effective and be deployed at scale in late 2020. They subsequently did and were.
- I said in print in 2013 when the Hyperloop proposal was released that the technical approach of air bearings in overland vacuum tubes on scavenged rights of way wouldn’t work. Subsequently, despite having insisted they would work and spent millions of dollars on them, every Hyperloop company abandoned all three of these elements, and development of Hyperloops has largely ceased.
- I said in print in 2016 that Level 4 self-driving cars would not be commercialized or near commercialization by 2021 due to the long tail of unusual situations, when several major car companies said they would. They subsequently were not.
- I used my entire net worth and borrowing capacity to buy an abandoned mansion in 2011, and sold it seven years later for five times the price.
Luck played a role in each of these predictions, and I have also made other predictions that didn’t pan out as well, but I hope my record reflects my decent calibration and genuine open-mindedness.
Ted Sanders
Areas of expertise
I am a decent expert in semiconductor technology and AI technology.
- I earned a PhD in Applied Physics from Stanford, where I spent years researching semiconductor physics and the potential of new technologies to beat the 60 mV/dec limit of today's silicon transistor (e.g., magnetic computing, quantum computing, photonic computing, reversible computing, negative capacitance transistors, and other ideas). These years of research inform our perspective on the likelihood of hardware progress over the next 20 years.
- After graduation, I had the opportunity to work at Intel R&D on next-gen computer chips, but instead, worked as a management consultant in the semiconductor industry and advised semiconductor CEOs on R&D prioritization and supply chain strategy. These years of work inform our perspective on the difficulty of rapidly scaling semiconductor production.
- Today, I work on AGI technology as a research engineer at OpenAI, a company aiming to develop transformative AGI. This work informs our perspective on software progress needed for AGI. (Disclaimer: nothing in this essay reflects OpenAI’s beliefs or its non-public information.)
Track record of forecasting
I have a track record of success in forecasting competitions:
- Top prize in SciCast technology forecasting tournament (15 out of ~10,000, ~$2,500 winnings)
- Top Hypermind US NGDP forecaster in 2014 (1 out of ~1,000)
- 1st place Stanford CME250 AI/ML Prediction Competition (1 of 73)
- 2nd place ‘Let’s invent tomorrow’ Private Banking prediction market (2 out of ~100)
- 2nd place DAGGRE Workshop competition (2 out of ~50)
- 3rd place LG Display Futurecasting Tournament (3 out of 100+)
- 4th Place SciCast conditional forecasting contest
- 9th place DAGGRE Geopolitical Forecasting Competition
- 30th place Replication Markets (~$1,000 winnings)
- Winner of ~$4200 in the 2022 Hybrid Persuasion-Forecasting Tournament on existential risks (told ranking was “quite well”)
Each finish resulted from luck alongside skill, but in aggregate I hope my record reflects my decent calibration and genuine open-mindedness.
Discussion
We look forward to discussing our essay with you in the comments below. The more we learn from you, the more pleased we'll be.
If you disagree with our admittedly imperfect guesses, we kindly ask that you supply your own preferred probabilities (or framework modifications). It's easier to tear down than build up, and we'd love to hear how you think this analysis can be improved.
117 comments
Comments sorted by top scores.
comment by Daniel Kokotajlo (daniel-kokotajlo) · 2023-06-06T18:38:43.312Z · LW(p) · GW(p)
Thanks for this well-researched and thorough argument! I think I have a bunch of disagreements, but my main one is that it really doesn't seem like AGI will require 8-10 OOMs more inference compute than GPT-4. I am not at all convinced by your argument that it would require that much compute to accurately simulate the human brain. Maybe it would, but we aren't trying to accurately simulate a human brain, we are trying to learn circuitry that is just as capable.
Also: Could you, for posterity, list some capabilities that you are highly confident no AI system will have by 2030? Ideally capabilities that come prior to a point-of-no-return so it's not too late to act by the time we see those capabilities.
↑ comment by Ted Sanders (ted-sanders) · 2023-06-06T23:46:02.273Z · LW(p) · GW(p)
Oh, to clarify, we're not predicting AGI will be achieved by brain simulation. We're using the human brain as a starting point for guessing how much compute AGI will need, and then applying a giant confidence interval (to account for cases where AGI is way more efficient, as well as way less efficient). It's the most uncertain part of our analysis and we're open to updating.
For posterity, by 2030, I predict we will not have:
- AI drivers that work in any country
- AI swim instructors
- AI that can do all of my current job at OpenAI in 2023
- AI that can get into a 2017 Toyota Prius and drive it
- AI that cleans my home (e.g., laundry, dishwashing, vacuuming, and/or wiping)
- AI retail workers
- AI managers
- AI CEOs running their own companies
- Self-replicating AIs running around the internet acquiring resources
Here are some of my predictions from the past:
- Predictions about the year 2050, written 7ish years ago: https://www.tedsanders.com/predictions-about-the-year-2050/
- Predictions on self-driving from 5 years ago: https://www.tedsanders.com/on-self-driving-cars/
↑ comment by Daniel Kokotajlo (daniel-kokotajlo) · 2023-06-07T03:22:44.464Z · LW(p) · GW(p)
Thanks! AI managers, CEOs, self-replicators, and your-job-doers (what is your job anyway? I never asked!) seem like things that could happen before it's too late (albeit only very shortly before) so they are potential sources of bets between us. (The other stuff requires lots of progress in robotics which I don't expect to happen until after the singularity, though I could be wrong)
Yes, I understand that you don't think AGI will be achieved by brain simulation. I like that you have a giant confidence interval to account for cases where AGI is way more efficient and way less efficient. I'm saying something has gone wrong with your confidence interval if the median is 8-10 OOMs more inference cost than GPT-4, given how powerful GPT-4 is. Subjectively GPT-4 seems pretty close to AGI, in the sense of being able to automate all strategically relevant tasks that can be done by human remote worker professionals. It's not quite there yet, but looking at the progress from GPT-2 to GPT-3 to GPT-4, it seems like maybe GPT-5 or GPT-6 would do it. But the middle of your confidence interval says that we'll need something like GPT-8, 9, or 10. This might be justified a priori, if all we had to go on was comparisons to the human brain, but I think a posteriori we should update on observing the GPT series so far and shift our probability mass significantly downwards. To put it another way, imagine if you had done this analysis in 2018, immediately after seeing GPT-1. Had someone at that time asked you to predict when e.g. a single AI system could pass the Bar, the LSAT, the SAT, and also do lots of coding interview problems and so forth, you probably would have said something like "Hmmm, human brain size anchor suggests we need another 12-15 OOMs from GPT-1 size to achieve AGI. Those skills seem pretty close to AGI, but idk maybe they are easier than I expect. I'll say... 10 OOMs from GPT-1. Could be more, could be less." Well, surprise! It was much less than that! (Of course I can't speak for you, but I can speak for myself, and that's what I would have said I had been thinking about the human brain anchor in 2018 and was convinced that 1e20-1e21 FLOP was the median of my distribution).
↑ comment by Ted Sanders (ted-sanders) · 2023-06-07T07:44:47.836Z · LW(p) · GW(p)
Great points.
I think you've identified a good crux between us: I think GPT-4 is far from automating remote workers and you think it's close. If GPT-5/6 automate most remote work, that will be point in favor of your view, and if takes until GPT-8/9/10+, that will be a point in favor of mine. And if GPT gradually provides increasingly powerful tools that wildly transform jobs before they are eventually automated away by GPT-7, then we can call it a tie. :)
I also agree that the magic of GPT should update one into believing in shorter AGI timelines with lower compute requirements. And you're right, this framework anchored on the human brain can't cleanly adjust from such updates. We didn't want to overcomplicate our model, but perhaps we oversimplified here. (One defense is that the hugeness of our error bars mean that relatively large updates are needed to make a substantial difference in the CDF.)
Lastly, I think when we see GPT unexpectedly pass the Bar, LSAT, SAT, etc. but continue to fail at basic reasoning, it should update us into thinking AGI is sooner (vs a no pass scenario), but also update us into realizing these metrics might be further from AGI than we originally assumed based on human analogues.
Replies from: daniel-kokotajlo, GdL752↑ comment by Daniel Kokotajlo (daniel-kokotajlo) · 2023-06-07T16:50:57.054Z · LW(p) · GW(p)
Excellent! Yeah I think GPT-4 is close to automating remote workers. 5 or 6, with suitable extensions (e.g. multimodal, langchain, etc.) will succeed I think. Of course, there'll be a lag between "technically existing AI systems can be made to ~fully automate job X" and "most people with job X are now unemployed" because things take time to percolate through the economy. But I think by the time of GPT-6 it'll be clear that this percolation is beginning to happen & the sorts of things that employ remote workers in 2023 (especially the strategically relevant ones, the stuff that goes into AI R&D) are doable by the latest AIs.
It sounds like you think GPT will continue to fail at basic reasoning for some time? And that it currently fails at basic reasoning to a significantly greater extent than humans do? I'd be interested to hear more about this, what sort of examples do you have in mind? This might be another great crux between us.
↑ comment by Andy_McKenzie · 2023-06-07T23:47:09.555Z · LW(p) · GW(p)
I’m wondering if we could make this into a bet. If by remote workers we include programmers, then I’d be willing to bet that GPT-5/6, depending upon what that means (might be easier to say the top LLMs or other models trained by anyone by 2026?) will not be able to replace them.
Replies from: daniel-kokotajlo↑ comment by Daniel Kokotajlo (daniel-kokotajlo) · 2023-06-10T15:46:31.337Z · LW(p) · GW(p)
I've made several bets like this in the past, but it's a bit frustrating since I don't stand to gain anything by winning -- by the time I win the bet, we are well into the singularity & there isn't much for me to do with the money anymore. What are the terms you have in mind? We could do the thing where you give me money now, and I give it back with interest later.
↑ comment by Andy_McKenzie · 2023-06-10T20:06:57.355Z · LW(p) · GW(p)
Understandable. How about this?
Bet
Andy will donate $50 to a charity of Daniel's choice now.
If, by January 2027, there is not a report from a reputable source confirming that at least three companies, that would previously have relied upon programmers, and meet a defined level of success, are being run without the need for human programmers, due to the independent capabilities of an AI developed by OpenAI or another AI organization, then Daniel will donate $100, adjusted for inflation as of June 2023, to a charity of Andy's choice.
Terms
Reputable Source: For the purpose of this bet, reputable sources include MIT Technology Review, Nature News, The Wall Street Journal, The New York Times, Wired, The Guardian, or TechCrunch, or similar publications of recognized journalistic professionalism. Personal blogs, social media sites, or tweets are excluded.
AI's Capabilities: The AI must be capable of independently performing the full range of tasks typically carried out by a programmer, including but not limited to writing, debugging, maintaining code, and designing system architecture.
Equivalent Roles: Roles that involve tasks requiring comparable technical skills and knowledge to a programmer, such as maintaining codebases, approving code produced by AI, or prompting the AI with specific instructions about what code to write.
Level of Success: The companies must be generating a minimum annual revenue of $10 million (or likely generating this amount of revenue if it is not public knowledge).
Report: A single, substantive article or claim in one of the defined reputable sources that verifies the defined conditions.
AI Organization: An institution or entity recognized for conducting research in AI or developing AI technologies. This could include academic institutions, commercial entities, or government agencies.
Inflation Adjustment: The donation will be an equivalent amount of money as $100 as of June 2023, adjusted for inflation based on https://www.bls.gov/data/inflation_calculator.htm.
I guess that there might be some disagreements in these terms, so I'd be curious to hear your suggested improvements.
Caveat: I don't have much disposable money right now, so it's not much money, but perhaps this is still interesting as a marker of our beliefs. Totally ok if it's not enough money to be worth it to you.
Replies from: daniel-kokotajlo↑ comment by Daniel Kokotajlo (daniel-kokotajlo) · 2023-06-10T20:18:29.827Z · LW(p) · GW(p)
Given your lack of disposable money I think this would be a bad deal for you, and as for me, it is sorta borderline (my credence that the bet will resolve in your favor is something like 40%?) but sure, let's do it. As for what charity to donate to, how about Animal Welfare Fund | Effective Altruism Funds. Thanks for working out all these details!
Here are some grey area cases we should work out:
--What if there is a human programmer managing the whole setup, but they are basically a formality? Like, the company does technically have programmers on staff but the programmers basically just form an interface between the company and ChatGPT and theoretically if the managers of the company were willing to spend a month learning how to talk to ChatGPT effectively they could fire the human programmers?
--What if it's clear that the reason you are winning the bet is that the government has stepped in to ban the relevant sorts of AI?
↑ comment by Andy_McKenzie · 2023-06-12T15:53:11.110Z · LW(p) · GW(p)
Sounds good, I'm happy with that arrangement once we get these details figured out.
Regarding the human programmer formality, it seems like business owners would have to be really incompetent for this to be a factor. Plenty of managers have coding experience. If the programmers aren't doing anything useful then they will be let go or new companies will start that don't have them. They are a huge expense. I'm inclined to not include this since it's an ambiguity that seems implausible to me.
Regarding the potential ban by the government, I wasn't really thinking of that as a possible option. What kind of ban do you have in mind? I imagine that regulation of AI is very likely by then, so if the automation of all programmers hasn't happened by Jan 2027, it seems very easy to argue that it would have happened in the absence of the regulation.
Regarding these and a few of the other ambiguous things, one way we could do this is that you and I could just agree on it in Jan 2027. Otherwise, the bet resolves N/A and you don't donate anything. This could make it an interesting Manifold question because it's a bit adversarial. This way, we could also get rid of the requirement for it to be reported by a reputable source, which is going to be tricky to determine.
Replies from: daniel-kokotajlo↑ comment by Daniel Kokotajlo (daniel-kokotajlo) · 2023-06-12T21:22:07.580Z · LW(p) · GW(p)
How about this:
--Re the first grey area: We rule in your favor here.
--Re the second grey area: You decide, in 2027, based on your own best judgment, whether or not it would have happened absent regulation. I can disagree with your judgment, but I still have to agree that you won the bet (if you rule in your favor).
↑ comment by Andy_McKenzie · 2023-06-16T20:48:53.612Z · LW(p) · GW(p)
Those sound good to me! I donated to your charity (the Animal Welfare Fund) to finalize it. Lmk if you want me to email you the receipt. Here's the manifold market:
Bet
Andy will donate $50 to a charity of Daniel's choice now.
If, by January 2027, there is not a report from a reputable source confirming that at least three companies, that would previously have relied upon programmers, and meet a defined level of success, are being run without the need for human programmers, due to the independent capabilities of an AI developed by OpenAI or another AI organization, then Daniel will donate $100, adjusted for inflation as of June 2023, to a charity of Andy's choice.
Terms
Reputable Source: For the purpose of this bet, reputable sources include MIT Technology Review, Nature News, The Wall Street Journal, The New York Times, Wired, The Guardian, or TechCrunch, or similar publications of recognized journalistic professionalism. Personal blogs, social media sites, or tweets are excluded.
AI's Capabilities: The AI must be capable of independently performing the full range of tasks typically carried out by a programmer, including but not limited to writing, debugging, maintaining code, and designing system architecture.
Equivalent Roles: Roles that involve tasks requiring comparable technical skills and knowledge to a programmer, such as maintaining codebases, approving code produced by AI, or prompting the AI with specific instructions about what code to write.
Level of Success: The companies must be generating a minimum annual revenue of $10 million (or likely generating this amount of revenue if it is not public knowledge).
Report: A single, substantive article or claim in one of the defined reputable sources that verifies the defined conditions.
AI Organization: An institution or entity recognized for conducting research in AI or developing AI technologies. This could include academic institutions, commercial entities, or government agencies.
Inflation Adjustment: The donation will be an equivalent amount of money as $100 as of June 2023, adjusted for inflation based on https://www.bls.gov/data/inflation_calculator.htm.
Regulatory Impact: In January 2027, Andy will use his best judgment to decide whether the conditions of the bet would have been met in the absence of any government regulation restricting or banning the types of AI that would have otherwise replaced programmers.
Replies from: daniel-kokotajlo↑ comment by Daniel Kokotajlo (daniel-kokotajlo) · 2023-06-16T20:51:31.595Z · LW(p) · GW(p)
Sounds good, thank you! Emailing the receipt would be nice.
Replies from: Andy_McKenzie↑ comment by Andy_McKenzie · 2023-06-16T21:04:13.545Z · LW(p) · GW(p)
Sounds good, can't find your email address, DM'd you.
↑ comment by GdL752 · 2023-06-07T17:02:24.287Z · LW(p) · GW(p)
continue to fail at basic reasoning.
But , a huge huge portion of human labor doesnt require basic reasoning. Its rote enough to use flowcharts , I don't need my calculator to "understand" math , I need it to give me the correct answer.
And for the "hallucinating" behavior you can just have it learn not do to that by rote. Even if you still need 10% of a certain "discipline" (job) to double check that the AI isn't making things up you've still increased productivity insanely.
And what does that profit and freed up capital do other than chase more profit and invest in things that draw down all the conditionals vastly?
5% increased productivity here , 3% over here , it all starts to multiply.
↑ comment by meijer1973 · 2023-06-07T14:15:18.937Z · LW(p) · GW(p)
AI will probably displace a lot of cognitive workers in the near future. And physical labor might take a while to get below 25$/hr.
- Most most tasks human level intelligence is not required.
- Most highly valued jobs have a lot of tasks that do not require high intelligence.
- Doing 95% of all tasks could be a lot sooner (10-15 years earlier) than 100%. See autonomous driving (getting to 95% safe or 99,9999 safe is a big difference).
- Physical labor by robots will probably remain expensive for a long time (e.g. a robot plumber). A robot ceo is probably cheaper in the future than the robot plumber.
- Just take gpt4 and fine tune it and you can automate a lot of cognitive labor already.
- Deployment of cognitve work automation (a software update) is much faster that deployment of physical robots.
I agree that AI might not replace swim instructors by 2030. It is the cognitive work where the big leaps will be.
comment by jimrandomh · 2023-06-09T20:01:44.941Z · LW(p) · GW(p)
This is the multiple stages fallacy. Not only is each of the probabilities in your list too low, if you actually consider them as conditional probabilities they're double- and triple-counting the same uncertainties. And since they're all mulitplied together, and all err in the same direction, the error compounds.
Replies from: ted-sanders, harfe↑ comment by Ted Sanders (ted-sanders) · 2023-06-09T21:39:19.867Z · LW(p) · GW(p)
What conditional probabilities would you assign, if you think ours are too low?
Replies from: jimrandomh↑ comment by jimrandomh · 2023-06-10T04:21:59.645Z · LW(p) · GW(p)
P(We invent algorithms for transformative AGI | No derailment from regulation, AI, wars, pandemics, or severe depressions): .8
P(We invent a way for AGIs to learn faster than humans | We invent algorithms for transformative AGI): 1. This row is already incorporated into the previous row.
P(AGI inference costs drop below $25/hr (per human equivalent): 1. This is also already incorporated into "we invent algorithms for transformative AGI"; an algorithm with such extreme inference costs wouldn't count (and, I think, would be unlikely to be developed in the first place).
We invent and scale cheap, quality robots: Not a prerequisite.
We massively scale production of chips and power: Not a prerequisite if we have already already conditioned on inference costs.
We avoid derailment by human regulation: 0.9
We avoid derailment by AI-caused delay: 1. I would consider an AI that derailed development of other AI ot be transformative.
We avoid derailment from wars (e.g., China invades Taiwan): 0.98.
We avoid derailment from pandemics: 0.995. Thanks to COVID, our ability to continue making technological progress during a pandemic which requires everyone to isolate is already battle-tested.
We avoid derailment from severe depressions: 0.99.
Replies from: ted-sanders↑ comment by Ted Sanders (ted-sanders) · 2023-06-13T07:56:49.938Z · LW(p) · GW(p)
Interested in betting thousands of dollars on this prediction? I'm game.
Replies from: Tamay↑ comment by Tamay · 2024-03-14T03:30:23.438Z · LW(p) · GW(p)
I'm interested. What bets would you offer?
Replies from: ted-sanders↑ comment by Ted Sanders (ted-sanders) · 2025-01-01T06:31:10.047Z · LW(p) · GW(p)
Hey Tamay, nice meeting you at The Curve. Just saw your comment here today.
Things we could potentially bet on:
- rate of GDP growth by 2027 / 2030 / 2040
- rate of energy consumption growth by 2027 / 2030 / 2040
- rate of chip production by 2027 / 2030 / 2040
- rates of unemployment (though confounded)
Any others you're interested in? Degree of regulation feels like a tricky one to quantify.
↑ comment by ryan_greenblatt · 2025-01-01T22:36:38.381Z · LW(p) · GW(p)
How about AI company and hardware company valuations? (Maybe in 2026, 2027, 2030 or similar.)
Or what about benchmark/task performance? Is there any benchmark/task you think won't get beaten in the next few years? (And, ideally, if it did get beaten, you would change you mind.) Maybe "AI won't be able to autonomously write good ML research papers (as judged by (e.g.) not having notably more errors than human written papers and getting into NeurIPS with good reviews)"? Could do "make large PRs to open source repos that are considered highly valuable" or "make open source repos that are widely used".
These might be a bit better to bet on as they could be leading indicators
(It's still the case that betting on the side of fast AI progress might be financially worse than just trying to invest or taking out a loan, but it could be easier to bet than to invest in e.g. OpenAI. Regardless, part of the point of betting is clearly demonstrating a view.)
↑ comment by harfe · 2023-06-09T22:32:05.900Z · LW(p) · GW(p)
There is an additional problem where one of the two key principles for their estimates is
Avoid extreme confidence
If this principle leads you to picking probability estimates that have some distance to 1 (eg by picking at most 0.95).
If you build a fully conjunctive model, and you are not that great at extreme probabilities, then you will have a strong bias towards low overall estimates. And you can make your probability estimates even lower by introducing more (conjunctive) factors.
comment by Unnamed · 2023-06-06T22:43:32.265Z · LW(p) · GW(p)
Interesting that this essay gives both a 0.4% probability of transformative AI by 2043, and a 60% probability of transformative AI by 2043, for slightly different definitions of "transformative AI by 2043". One of these is higher than the highest probability given by anyone on the Open Phil panel (~45%) and the other is significantly lower than the lowest panel member probability (~10%). I guess that emphasizes the importance of being clear about what outcome we're predicting / what outcomes we care about trying to predict.
The 60% is for "We invent algorithms for transformative AGI", which I guess means that we have the tech that can be trained to do pretty much any job. And the 0.4% is the probability for the whole conjunction, which sounds like it's for pervasively implemented transformative AI: AI systems have been trained to do pretty much any job, and the infrastructure has been built (chips, robots, power) for them to be doing all of those jobs at a fairly low cost.
It's unclear why the 0.4% number is the headline here. What's the question here, or the thing that we care about, such that this is the outcome that we're making forecasts for? e.g., I think that many paths to extinction don't route through this scenario. IIRC Eliezer has written that it's possible that AI could kill everyone before we have widespread self-driving cars. And other sorts of massive transformation don't depend on having all the infrastructure in place so that AIs/robots can be working as loggers, nurses, upholsterers, etc.
comment by Steven Byrnes (steve2152) · 2023-06-06T21:29:56.489Z · LW(p) · GW(p)
I disagree with the brain-based discussion of how much compute is required for AGI. Here’s an analogy I like (from here [LW · GW]):
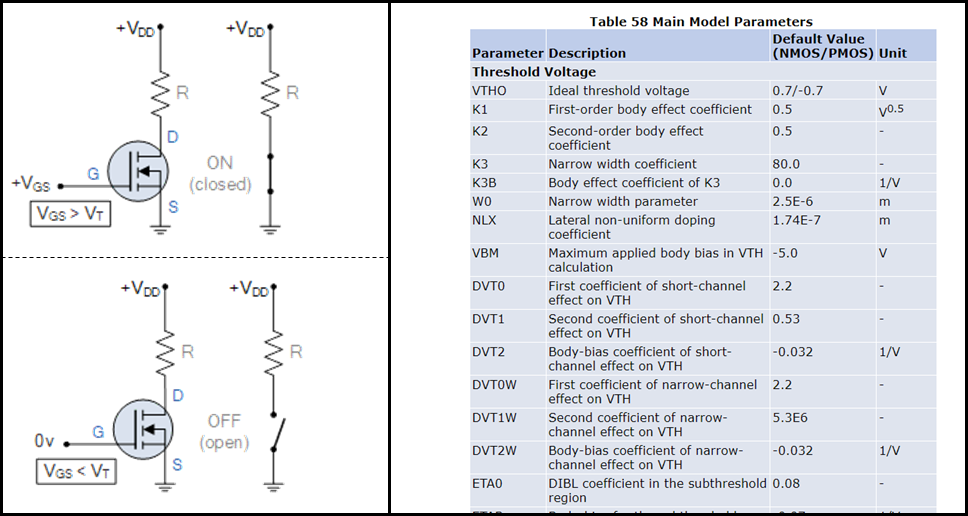
Left: Suppose that I want to model a translator (specifically, a MOSFET). And suppose that my model only needs to be sufficient to emulate the calculations done by a CMOS integrated circuit. Then my model can be extremely simple—it can just treat the transistor as a cartoon switch. (image source.)
Right: Again suppose that I want to model a transistor. But this time, I want my model to accurately capture all measurable details of the transistor. Then my model needs to be mind-bogglingly complex, involving dozens of adjustable parameters, some of which are shown in this table (screenshot from here).
What’s my point? I’m suggesting an analogy between this transistor and a neuron with synapses, dendritic spikes, etc. The latter system is mind-bogglingly complex when you study it in detail—no doubt about it! But that doesn’t mean that the neuron’s essential algorithmic role is equally complicated. The latter might just amount to a little cartoon diagram with some ANDs and ORs and IF-THENs or whatever. Or maybe not, but we should at least keep that possibility in mind.
--
For example, this paper is what I consider a plausible algorithmic role of dendritic spikes and synapses in cortical pyramidal neurons, and the upshot is “it’s basically just some ANDs and ORs”. If that’s right, this little bit of brain algorithm could presumably be implemented with <<1 FLOP per spike-through-synapse. I think that’s a suggestive data point, even if (as I strongly suspect) dendritic spikes and synapses are meanwhile doing other operations too.
--
Anyway, I currently think that, based on the brain, human-speed AGI is probably possible in 1e14 FLOP/s. (This post [LW · GW] has a red-flag caveat on top, but that’s related to some issues in my discussion of memory, I stand by the compute section.) Not with current algorithms, I don’t think! But with some future algorithm.
I think that running microscopically-accurate brain simulations is many OOMs harder than running the algorithms that the brain is running. This is the same idea as the fact that running a microscopically-accurate simulation of a pocket calculator microcontroller chip, with all its thousands of transistors and capacitors and wires, stepping the simulation forward picosecond-by-picosecond, as the simulated chip multiplies two numbers, is many OOMs harder than multiplying two numbers.
Replies from: ted-sanders↑ comment by Ted Sanders (ted-sanders) · 2023-06-07T00:03:09.211Z · LW(p) · GW(p)
Excellent points. Agree that the compute needed to simulate a thing is not equal to the compute performed by that thing. It's very possible this means we're overestimating the compute performed by the human brain a bit. Possible this is counterbalanced by early AGIs being inefficient, or having architectural constraints that the human brain lacks, but who knows. Very possible our 16% is too low, and should be higher. Tripling it to ~50% would yield a likelihood of transformative AGI of ~1.2%.
Replies from: daniel-kokotajlo, AnthonyC↑ comment by Daniel Kokotajlo (daniel-kokotajlo) · 2023-06-07T17:08:06.133Z · LW(p) · GW(p)
It's very possible this means we're overestimating the compute performed by the human brain a bit.
Specifically, by 6-8 OOMs. I don't think that's "a bit." ;)
↑ comment by AnthonyC · 2023-06-07T02:29:51.774Z · LW(p) · GW(p)
Dropping the required compute by, say, two OOMs, changes the estimates of how many fabs and how much power will be needed from "Massively more than expected from business as usual" to "Not far from business as usual" aka that 16% would need to be >>90% because by default the capacity would exist anyway. The same change would have the same kind effect on the "<$25/hr" assumption. At that scale, "just throw more compute at it" becomes a feasible enough solution that "learns slower than humans" stops seeming like a plausible problem, as well. I think you might be assuming you've made these estimates independently when they're actually still being calculated based on common assumptions.
Replies from: ted-sanders↑ comment by Ted Sanders (ted-sanders) · 2023-06-07T07:52:43.284Z · LW(p) · GW(p)
According to our rough and imperfect model, dropping inference needs by 2 OOMs increases our likelihood of hitting the $25/hr target by 20%abs, from 16% to 36%.
It doesn't necessarily make a huge difference to chip and power scaling, as in our model those are dominated by our training estimates, not our inference need estimates. (Though of course those figures will be connected in reality.)
With no adjustment to chip and power scaling, this yields a 0.9% likelihood of TAGI.
With a +15%abs bump to chip and power scaling, this yields a 1.2% likelihood of TAGI.
Replies from: AnthonyC↑ comment by AnthonyC · 2023-06-08T18:47:59.941Z · LW(p) · GW(p)
Ah, sorry, I see I made an important typo in my comment, that 16% value I mentioned was supposed to be 46%, because it was in reference to the chip fabs & power requirements estimate.
The rest of the comment after that was my way of saying "the fact that these dependences on common assumptions between the different conditional probabilities exist at all mean you can't really claim that you can multiply them all together and consider the result meaningful in the way described here."
I say that because the dependencies mean you can't productively discuss disagreements about any of your assumptions that go into your estimates, without adjusting all the probabilities in the model. A single updated assumption/estimate breaks the claim of conditional independence that lets you multiply the probabilities.
For example, in a world that actually had "algorithms for transformative AGI" that were just too expensive to productively used, what would happen next? Well, my assumption is that a lot more companies would hire a lot more humans to get to work on making them more efficient, using the best available less-transformative tools. A lot of governments would invest trillions in building the fabs and power plants and mines to build it anyway, even if it still cost $25,000/human-equivalent-hr. They'd then turn the AGI loose on the problem of improving its own efficiency. And on making better robots. And on using those robots to make more robots and build more power plants and mine more materials. Once producing more inputs is automated, supply stops being limited by human labor, and doesn't require more high level AI inference either. Cost of inputs into increasing AI capabilities becomes decoupled from the human economy, so that the price of electricity and compute in dollars plummets. This is one of many hypothetical pathways where a single disagreement renders consideration of the subsequent numbers moot. Presenting the final output as a single number hides the extreme sensitivity of that number to changes in key underlying assumptions.
comment by [deleted] · 2023-06-06T19:26:12.816Z · LW(p) · GW(p)
Hi Ted,
I will read the article, but there's some rather questionable assumptions here that I don't see how you could reach these conclusions while also considering them.
We invent algorithms for transformative AGI | 60% |
We invent a way for AGIs to learn faster than humans | 40% |
AGI inference costs drop below $25/hr (per human equivalent) | 16% |
We invent and scale cheap, quality robots | 60% |
We massively scale production of chips and power | 46% |
We avoid derailment by human regulation | 70% |
We avoid derailment by AI-caused delay | 90% |
We avoid derailment from wars (e.g., China invades Taiwan) | 70% |
We avoid derailment from pandemics | 90% |
We avoid derailment from severe depressions | 95% |
We invent algorithms for transformative AGI:
- Have you considered RSI? RSI in this context would be an algorithm that says "given a benchmark that measures objectively if an AI is transformative, propose a cognitive architecture for an AGI using a model with sufficient capabilities to make a reasonable guess". You then train the AGI candidate from the cognitive architecture (most architectures will reuse pretrained components from prior attempts) and benchmark it. You maintain a "league" of multiple top performing AGI candidates, and each one of them is in each cycle examining all the results, developing theories, and proposing the next candidate architecture.
Because RSI uses criticality, it primarily depends on compute availability. Assuming sufficient compute, it would discover a transformative AGI algorithm quickly. Some iterations might take 1 month (training time for a llama scale model from noise), others would take less than 1 day, and many iterations would be attempted in parallel.
We invent a way for AGIs to learn faster than humans : Why is this even in the table? This would be 1.0 because it's a known fact, AGI learns faster than humans. Again, from the llama training run, the model went from knowing nothing to domain human level in 1 month. That's faster. (requiring far more data than humans isn't an issue)
AGI inference costs drop below $25/hr (per human equivalent): Well, A100s are 0.87 per hour. A transformative AGI might use 32 A100s. $27.84 an hour. Looks like we're at 1.0 on this one also. Note I didn't even bother with a method to use compute at inference time more efficiently. In short, use a low end, cheap model, and a second model that assesses, given the current input, how likely it is that the full scale model will produce a meaningfully different output.
So for example, a robot doing a chore is using a low end, cheap model trained using supervised learning from the main model. That model runs in real time in hardware inside the robot. Whenever the input frame is poorly compressible from an onboard autoencoder trained on the supervised learning training set, the robot pauses what it's doing and queries the main model. (some systems can get the latency low enough to not need to pause).
This general approach would save main model compute, such that it could cost $240 an hour to run the main model and yet as long as it isn't used more than 10% of the time, and the local model is very small and cheap, it will hit your $25 an hour target.
This is not cutting edge and there are papers published this week on this and related approaches.
We invent and scale cheap, quality robots: Due to recent layoffs in the sota robotics teams and how Boston Dynamics has been of low investor interest, yes this is a possible open
We massively scale production of chips and power: I will have to check the essay to see how you define "massively".
We avoid derailment by human regulation: This is a parallel probability. It's saying actually 1 - (probability that ALL regulatory agencies in power blocs capable of building AGI regulate it to extinction). So it's 1 - (EU AND USA AND China AND <any other parties> block tAGI research). The race dynamics mean that defection is rapid so the blocks fail rapidly, even under suspected defection. If one party believes the others might be getting tAGI, they may start a national defense project to rush build their own, a project that is exempt from said regulation.
We avoid derailment by AI-caused delay: Another parallel probability. It's the probability that an "AI chernobyl" happens and all parties respect the lesson of it.
We avoid derailment from wars (e.g., China invades Taiwan) : Another parallel probability, as Taiwan is not the only location on earth capable of manufacturing chips for AI.
We avoid derailment from pandemics: So a continuous series of pandemics, not a 3 year dip to ~80% productivity? Due to RSI even 80% productivity may be sufficient.
We avoid derailment from severe depressions: Another parallel probability.
With that said : in 15 years I find the conclusion of "probably not" tAGI that can do almost everything better than humans, including robotics, and it's cheap per hour, and massive amounts of new infrastructure have been built, to probably be correct. So many steps, and even if you're completely wrong due to the above or black swans, it doesn't mean a different step won't be rate limiting. Worlds where we have AGI that is still 'transformative' by a plain meaning of the term, and the world is hugely different, and AGI is receiving immense financial investments and is the most valuable industry on earth, are entirely possible without it actually satisfying the end conditions this essay is aimed at.
Replies from: ted-sanders↑ comment by Ted Sanders (ted-sanders) · 2023-06-06T23:52:43.310Z · LW(p) · GW(p)
We invent a way for AGIs to learn faster than humans : Why is this even in the table? This would be 1.0 because it's a known fact, AGI learns faster than humans. Again, from the llama training run, the model went from knowing nothing to domain human level in 1 month. That's faster. (requiring far more data than humans isn't an issue)
100% feels overconfident. Some algorithms learning some things faster than humans is not proof that AGI will learn all things faster than humans. Just look at self-driving. It's taking AI far longer than human teenagers to learn.
AGI inference costs drop below $25/hr (per human equivalent): Well, A100s are 0.87 per hour. A transformative AGI might use 32 A100s. $27.84 an hour. Looks like we're at 1.0 on this one also.
100% feels overconfident. We don't know if transformative will need 32 A100s, or more. Our essay explains why we think it's more. Even if you disagree with us, I struggle to see how you can be 100% sure.
Replies from: conor↑ comment by Conor (conor) · 2023-06-11T12:55:26.119Z · LW(p) · GW(p)
Teenagers generally don't start learning to drive until they have had fifteen years to orient themselves in the world.
AI and teenagers are not starting from the same point so the comparison does not map very well.
comment by Charlie Steiner · 2023-06-06T20:44:06.019Z · LW(p) · GW(p)
Thanks, this was interesting.
I couldn't really follow along with my own probabilities because things started wild from the get-go. You say we need to "invent algorithms for transformative AI," when in fact we already have algorithms that are in-principle general, they're just orders of magnitude too inefficient, but we're making gradual algorithmic progress all the time. Checking the pdf, I remain confused about your picture of the world here. Do you think I'm drastically overstating the generality of current ML and the gradualness of algorithmic improvement, such that currently we are totally lacking the ability to build AGI, but after some future discovery (recognizable on its own merits and not some context-dependent "last straw") we will suddenly be able to?
And your second question is also weird! I don't really understand the epistemic state of the AI researchers in this hypothetical. They're supposed to have built something that's AGI, it just learns slower than humans. How did they get confidence in this fact? I think this question is well-posed enough that I could give a probability for it, except that I'm still confused about how to conditionalize on the first question.
The rest of the questions make plenty of sense, no complaints there.
In terms of the logical structure, I'd point out that inference costs staying low, producing chips, and producing lots of robots are all definitely things that could be routes to transformative AI, but they're not necessary. The big alternate path missing here is quality. An AI that generates high-quality designs or plans might not have a human equivalent, in which case "what's the equivalent cost at $25 per human hour" is a wrong question. Producing chips and producing robots could also happen or not happen in any combination and the world could still be transformed by high-quality AI decision-making.
Replies from: ted-sanders↑ comment by Ted Sanders (ted-sanders) · 2023-06-07T22:48:24.124Z · LW(p) · GW(p)
I'm curious and I wonder if I'm missing something that's obvious to others: What are the algorithms we already have for AGI? What makes you confident they will work before seeing any demonstration of AGI?
Replies from: Charlie Steiner↑ comment by Charlie Steiner · 2023-06-08T01:16:12.974Z · LW(p) · GW(p)
So, the maximally impractical but also maximally theoretically rigorous answer here is AIXI-tl.
An almost as impractical answer would be Markov chain Monte Carlo search for well-performing huge neural nets on some objective.
I say MCMC search because I'm confident that there's some big neural nets that are good at navigating the real world, but any specific efficient training method we know of right now could fail to scale up reliably. Instability being the main problem, rather than getting stuck in local optima.
Dumb but thorough hyperparameter search and RL on a huge neural net should also work. Here we're adding a few parts of "I am confident in this because of empirical data abut the historical success of scaling up neural nets trained with SGD" to arguments that still mostly rest on "I am confident because of mathematical reasoning about what it means to get a good score at an objective."
Replies from: ted-sanders↑ comment by Ted Sanders (ted-sanders) · 2023-06-08T21:32:05.447Z · LW(p) · GW(p)
Gotcha. I guess there's a blurry line between program search and training. Somehow training feels reasonable to me, but something like searching over all possible programs feels unreasonable to me. I suppose the output of such a program search is what I might mean by an algorithm for AGI.
Hyperparameter search and RL on a huge neural net feels wildly underspecified to me. Like, what would be its inputs and outputs, even?
Replies from: Charlie Steiner↑ comment by Charlie Steiner · 2023-06-08T22:00:53.478Z · LW(p) · GW(p)
Since I'm fine with saying things that are wildly inefficient, almost any input/output that's sufficient to reward modeling of the real world (rather than e.g. just playing the abstract game of chess) is sufficient. A present-day example might be self-driving car planning algorithms (though I don't think any major companies actually use end to end NN planning).
Replies from: ted-sanders↑ comment by Ted Sanders (ted-sanders) · 2023-06-09T00:50:13.909Z · LW(p) · GW(p)
Right, but what inputs and outputs would be sufficient to reward modeling of the real world? I think that might take some exploration and experimentation, and my 60% forecast is the odds of such inquiries succeeding by 2043.
Even with infinite compute, I think it's quite difficult to build something that generalizes well without overfitting.
Replies from: Charlie Steiner↑ comment by Charlie Steiner · 2023-06-09T06:24:41.130Z · LW(p) · GW(p)
what inputs and outputs would be sufficient to reward modeling of the real world?
This is an interesting question but I think it's not actually relevant. Like, it's really interesting to think about a thermostat - something who's only inputs are a thermometer and a clock, and only output is a switch hooked to a heater. Given arbitrarily large computing power and arbitrary amounts of on-distribution training data, will RL ever learn all about the outside world just from temperature patterns? Will it ever learn to deliberately affect the humans around it by turning the heater on and off? Or is it stuck being a dumb thermostat, a local optimum enforced not by the limits of computation but by the structure of the problem it faces?
But people are just going to build AIs attached to video cameras, or screens read by humans, or robot cars, or the internet, which are enough information flow by orders of magnitude, so it's not super important where the precise boundary is.
Replies from: ted-sanders↑ comment by Ted Sanders (ted-sanders) · 2023-06-09T18:10:29.901Z · LW(p) · GW(p)
Right, I'm not interested in minimum sufficiency. I'm just interested in the straightforward question of what data pipes would we even plug into the algorithm that would result in AGI. Sounds like you think a bunch of cameras and computers would work? To me, it feels like an empirical problem that will take years of research.
comment by Max H (Maxc) · 2023-06-07T23:03:56.055Z · LW(p) · GW(p)
I think the biggest problem with these estimates is that they rely on irrelevant comparisons to the human brain.
What we care about is how much compute is needed to implement the high-level cognitive algorithms that run in the brain; not the amount of compute needed to simulate the low-level operations the brain carries out to perform that cognition. This is a much harder to quantity to estimate, but it's also the only thing that actually matters.
See Biology-Inspired AGI Timelines: The Trick That Never Works [LW · GW] and other extensive prior discussion [LW · GW] on this.
I think with enough algorithmic improvement, there's enough hardware lying around already to get to TAI, and once you factor this in, a bunch of other conditional events are actually unnecessary or much more likely. My own estimates:
Event | Forecastby 2043 or TAGI, |
We invent algorithms for transformative AGI | 90% |
100% | |
100% | |
100% | |
100% | |
We avoid derailment by human regulation | 80% |
We avoid derailment by AI-caused delay | 95% |
We avoid derailment from wars (e.g., China invades Taiwan) | 95% |
We avoid derailment from pandemics | 95% |
We avoid derailment from severe depressions | 95% |
Joint odds | 58.6% |
Explanations:
- Inventing algorithms: this is mostly just a wild guess / gut sense, but it is upper-bounded in difficulty by the fact that evolution managed to discover algorithms for human level cognition through billions of years of pretty dumb and low bandwidth trial-and-error.
- "We invent a way for AGIs to learn faster than humans." This seems like it is mostly implied by the first conditional already and / or already satisfied: current AI systems can already be trained in less wall-clock time than it takes a human to develop during a lifetime, and much less wall clock time than it took evolution to discover the design for human brains. Even the largest AI systems are currently trained on much less data than the amount of raw sensory data that is available to a human over a lifetime. I see no reason to expect these trends to change as AI gets more advanced.
- "AGI inference costs drop below $25/hr (per human equivalent)" - Inference costs of running e.g. GPT-4 are already way, way less than the cost of humans on a per-token basis, it's just that the tokens are way, way lower quality than the best humans can produce. Conditioned on inventing the algorithms to get higher quality output, it seems like there's plausibly very little left to do to here.
- Inventing robots - doesn't seem necessary for TAI; we already have decent, if expensive, robotics and TAI can help develop more and make them cheaper / more scalable.
- Scaling production of chips and power - probably not necessary, see the point in the first part of this comment on relevant comparisons to the brain.
- Avoiding derailment - if existing hardware is sufficient to get to TAI, it's much easier to avoid derailment for any reason. You just need a few top capabilities research labs to continue to be able to push the frontier by experimenting with existing clusters.
↑ comment by Martin Randall (martin-randall) · 2023-06-13T02:40:07.865Z · LW(p) · GW(p)
I'm curious about your derailment odds. The definition of "transformative AGI" in the paper is restrictive:
AI that can quickly and affordably be trained to perform nearly all economically and strategically valuable tasks at roughly human cost or less.
A narrow superintelligence that can, for example, engineer pandemics or conduct military operations could lead to severe derailment without satisfying this definition. I guess that would qualify as "AI-caused delay"? To follow the paper's model, we need to estimate these odds in a conditional world where humans are not regulating AI use in ways that significantly delay the path to transformative AGI, which further increases the risk.
Replies from: Maxc↑ comment by Max H (Maxc) · 2023-06-13T03:15:21.085Z · LW(p) · GW(p)
engineer pandemics or conduct military operations could lead to severe derailment without satisfying this definition.
I think humans could already do those things pretty well without AI, if they wanted to. Narrow AI might make those things easier, possibly much easier, just like nukes and biotech research have in the past. I agree this increases the chance that things go "off the rails", but I think once you have an AI that can solve hard engineering problems in the real world like that, there's just not that much further to go to full-blown superintelligence, whether you call its precursor "narrow" or not.
The probabilities in my OP are mostly just a gut sense wild guess, but they're based on the intuition that it takes a really big derailment to halt frontier capabilities progress, which mostly happens in well-funded labs that have the resources and will to continue operating through pretty severe "turbulence" - economic depression, war, pandemics, restrictive regulation, etc. Even if new GPU manufacturing stops completely, there are already a lot of H100s and A100s lying around, and I expect that those are sufficient to get pretty far.
↑ comment by Ted Sanders (ted-sanders) · 2023-06-08T21:15:05.749Z · LW(p) · GW(p)
Excellent comment - thanks for sticking your neck out to provide your own probabilities.
Given the gulf between our 0.4% and your 58.6%, would you be interested in making a bet (large or small) on TAI by 2043? If yes, happy to discuss how we might operationalize it.
Replies from: Maxc↑ comment by Max H (Maxc) · 2023-06-08T22:24:15.886Z · LW(p) · GW(p)
I appreciate the offer to bet! I'm probably going to decline though - I don't really want or need more skin-in-the-game on this question (many of my personal and professional plans assume short timelines.)
You might be interested in this post [LW · GW] (and the bet it is about), for some commentary and issues with operationalizing bets like this.
Also, you might be able to find someone else to bet with you - I think my view is actually closer to the median among EAs / rationalists / alignment researchers than yours. For example, the Open Phil panelists judging this contest say [EA · GW]:
Replies from: ted-sanders, ted-sandersPanelist credences on the probability of AGI by 2043 range from ~10% to ~45%.
↑ comment by Ted Sanders (ted-sanders) · 2023-06-09T00:54:14.325Z · LW(p) · GW(p)
Sounds good. Can also leave money out of it and put you down for 100 pride points. :)
If so, message me your email and I'll send you a calendar invite for a group reflection in 2043, along with a midpoint check in in 2033.
↑ comment by Ted Sanders (ted-sanders) · 2023-06-09T01:01:14.104Z · LW(p) · GW(p)
I'm not convinced about the difficulty of operationalizing Eliezer's doomer bet. Effectively, loaning money to a doomer who plans to spend it all by 2030 is, in essence, a claim on the doomer's post-2030 human capital. The doomer thinks it's worthless, whereas the skeptic thinks it has value. Hence, they transact.
The TAGI case seems trickier than the doomer case. Who knows what a one dollar bill will be worth in a post-TAGI world.
comment by Ege Erdil (ege-erdil) · 2023-06-10T01:44:45.880Z · LW(p) · GW(p)
Just to pick on the step that gets the lowest probability in your calculation, estimating that the human brain does 1e20 FLOP/s with only 20 W of power consumption requires believing that the brain is basically operating at the bitwise Landauer limit, which is around 3e20 bit erasures per watt per second at room temperature. If the FLOP we're talking about here is equivalent of operations on 8-bit floating point numbers, for example, the human brain would have an energy efficiency of around 1e20 bit erasures per watt, which is less than one order of magnitude from the Landauer limit at room temperature of 300 K.
Needless to say, I find this estimate highly unrealistic. We have no idea how to build practical densely packed devices which get anywhere close to this limit; the best we can do at the moment is perhaps 5 orders of magnitude away. Are you really thinking that the human brain is 5 OOM more energy efficient than an A100?
Still, even this estimate is much more realistic than your claim that the human brain might take 8e34 FLOP to train, which ascribes a ludicrous ~ 1e26 FLOP/s computation capacity to the human brain if this training happens over 20 years. This obviously violates the Landauer limit on computation and so is going to be simply false, unless you think the human brain loses less than one bit of information per 1e5 floating point operations it's doing. Good luck with that.
I notice that Steven Byrnes has already made the argument that these estimates are poor, but I want to hammer home the point that they are not just poor, they are crazy. Obviously, a mistake this flagrant does not inspire confidence in the rest of the argument.
Replies from: ted-sanders, ted-sanders, Muireall↑ comment by Ted Sanders (ted-sanders) · 2023-06-14T05:36:45.140Z · LW(p) · GW(p)
Let me try writing out some estimates. My math is different than yours.
An H100 SXM has:
- 8e10 transistors
- 2e9 Hz boost frequency of
- 2e15 FLOPS at FP16
- 7e2 W of max power consumption
Therefore:
- 2e6 eV are spent per FP16 operation
- This is 1e8 times higher than the Landauer limit of 2e-2 eV per bit erasure at 70 C (and the ratio of bit erasures per FP16 operation is unclear to me; let's pretend it's O(1))
- An H100 performs 1e6 FP16 operations per clock cycle, which implies 8e4 transistors per FP16 operation (some of which may be inactive, of course)
This seems pretty inefficient to me!
To recap, modern chips are roughly ~8 orders of magnitude worse than the Landauer limit (with a bit erasure per FP16 operation fudge factor that isn't going to exceed 10). And this is in a configuration that takes 8e4 transistors to support a single FP16 operation!
Positing that brains are ~6 orders of magnitude more energy efficient than today's transistor circuits doesn't seem at all crazy to me. ~6 orders of improvement on 2e6 is ~2 eV per operation, still two orders of magnitude above the 0.02 eV per bit erasure Landauer limit.
I'll note too that cells synthesize informative sequences from nucleic acids using less than 1 eV of free energy per bit. That clearly doesn't violate Landauer or any laws of physics, because we know it happens.
Replies from: ege-erdil↑ comment by Ege Erdil (ege-erdil) · 2023-06-14T06:36:10.998Z · LW(p) · GW(p)
2e6 eV are spent per FP16 operation... This is 1e8 times higher than the Landauer limit of 2e-2 eV per bit erasure at 70 C (and the ratio of bit erasures per FP16 operation is unclear to me; let's pretend it's O(1))
2e-2 eV for the Landauer limit is right, but 2e6 eV per FP16 operation is off by one order of magnitude. (70 W)/(2e15 FLOP/s) = 0.218 MeV. So the gap is 7 orders of magnitude assuming one bit erasure per FLOP.
This is wrong, the power consumption is 700 W so the gap is indeed 8 orders of magnitude.
An H100 SXM has 8e10 transistors, 2e9 Hz boost frequency,
70 W700 W of max power consumption...
8e10 * 2e9 = 1.6e20 transistor switches per second. This happens with a power consumption of 700 W, suggesting that each switch dissipates on the order of 30 eV of energy, which is only 3 OOM or so from the Landauer limit. So this device is actually not that inefficient if you look only at how efficiently it's able to perform switches. My position is that you should not expect the brain to be much more efficient than this, though perhaps gaining one or two orders of magnitude is possible with complex error correction methods.
Of course, the transistors supporting per FLOP and the switching frequency gap have to add up to the 8 OOM overall efficiency gap we've calculated. However, it's important that most of the inefficiency comes from the former and not the latter. I'll elaborate on this later in the comment.
This seems pretty inefficient to me!
I agree an H100 SXM is not a very efficient computational device. I never said modern GPUs represent the pinnacle of energy efficiency in computation or anything like that, though similar claims have previously been made [LW · GW] by others on the forum.
Positing that brains are ~6 orders of magnitude more energy efficient than today's transistor circuits doesn't seem at all crazy to me. ~6 orders of improvement on 2e6 is ~2 eV per operation, still two orders of magnitude above the 0.02 eV per bit erasure Landauer limit.
Here we're talking about the brain possibly doing 1e20 FLOP/s, which I've previously said is maybe within one order of magnitude of the Landauer limit or so, and not the more extravagant figure of 1e25 FLOP/s. The disagreement here is not about math; we both agree that this performance requires the brain to be 1 or 2 OOM from the bitwise Landauer limit depending on exactly how many bit erasures you think are involved in a single 16-bit FLOP.
The disagreement is more about how close you think the brain can come to this limit. Most of the energy losses in modern GPUs come from the enormous amounts of noise that you need to deal with in interconnects that are closely packed together. To get anywhere close to the bitwise Landauer limit, you need to get rid of all of these losses. This is what would be needed to lower the amount of transistors supporting per FLOP without also simultaneously increasing the power consumption of the device.
I just don't see how the brain could possibly pull that off. The design constraints are pretty similar in both cases, and the brain is not using some unique kind of material or architecture which could eliminate dissipative or radiative energy losses in the system. Just as information needs to get carried around inside a GPU, information also needs to move inside the brain, and moving information around in a noisy environment is costly. So I would expect by default that the brain is many orders of magnitude from the Landauer limit, though I can see estimates as high as 1e17 FLOP/s being plausible if the brain is highly efficient. I just think you'll always be losing many orders of magnitude relative to Landauer as long as your system is not ideal, and the brain is far from an ideal system.
I'll note too that cells synthesize informative sequences from nucleic acids using less than 1 eV of free energy per bit. That clearly doesn't violate Landauer or any laws of physics, because we know it happens.
I don't think you'll lose as much relative to Landauer when you're doing that, because you don't have to move a lot of information around constantly. Transcribing a DNA sequence and other similar operations are local. The reason I think realistic devices will fall far short of Landauer is because of the problem of interconnect: computations cannot be localized effectively, so different parts of your hardware need to talk to each other, and that's where you lose most of the energy. In terms of pure switching efficiency of transistors, we're already pretty close to this kind of biological process, as I've calculated above.
Replies from: ted-sanders, ted-sanders, ege-erdil↑ comment by Ted Sanders (ted-sanders) · 2023-06-14T06:49:28.941Z · LW(p) · GW(p)
One potential advantage of the brain is that it is 3D, whereas chips are mostly 2D. I wonder what advantage that confers. Presumably getting information around is much easier with 50% more dimensions.
Replies from: ege-erdil↑ comment by Ege Erdil (ege-erdil) · 2023-06-14T07:07:15.184Z · LW(p) · GW(p)
Probably true, and this could mean the brain has some substantial advantage over today's hardware (like 1 OOM, say) but at the same time the internal mechanisms that biology uses to establish electrical potential energy gradients and so forth seem so inefficient. Quoting Eliezer;
I'm confused at how somebody ends up calculating that a brain - where each synaptic spike is transmitted by ~10,000 neurotransmitter molecules (according to a quick online check), which then get pumped back out of the membrane and taken back up by the synapse; and the impulse is then shepherded along cellular channels via thousands of ions flooding through a membrane to depolarize it and then getting pumped back out using ATP, all of which are thermodynamically irreversible operations individually - could possibly be within three orders of magnitude of max thermodynamic efficiency at 300 Kelvin. I have skimmed "Brain Efficiency" though not checked any numbers, and not seen anything inside it which seems to address this sanity check.
↑ comment by Ted Sanders (ted-sanders) · 2023-06-14T06:44:20.762Z · LW(p) · GW(p)
70 W
Max power is 700 W, not 70 W. These chips are water-cooled beasts. Your estimate is off, not mine.
Replies from: ege-erdil↑ comment by Ege Erdil (ege-erdil) · 2023-06-14T06:53:30.245Z · LW(p) · GW(p)
Huh, I wonder why I read 7e2 W as 70 W. Strange mistake.
Replies from: ted-sanders↑ comment by Ted Sanders (ted-sanders) · 2023-06-14T06:59:05.151Z · LW(p) · GW(p)
No worries. I've made far worse. I only wish that H100s could operate at a gentle 70 W! :)
↑ comment by Ege Erdil (ege-erdil) · 2023-06-14T06:52:27.955Z · LW(p) · GW(p)
I'm posting this as a separate comment because it's a different line of argument, but I think we should also keep it in mind when making estimates of how much computation the brain could actually be using.
If the brain is operating at a frequency of (say) 10 Hz and is doing 1e20 FLOP/s, that suggests the brain has something like 1e19 floating point parameters, or maybe specifying the "internal state" of the brain takes something like 1e20 bits. If you want to properly train a neural network of this size, you need to update on a comparable amount of useful entropy from the outside world. This means you have to believe that humans are receiving on the order of 1e11 bits or 10 GB of useful information about the world to update on every second if the brain is to be "fully trained" by the age of 30, say.
An estimate of 1e15 FLOP/s brings this down to a more realistic 100 KB or so, which still seems like a lot but is somewhat more believable if you consider the potential information content of visual and auditory stimuli. I think even this is an overestimate and that the brain has some algorithmic insights which make it somewhat more data efficient than contemporary neural networks, but I think the gap implied by 1e20 FLOP/s is rather too large for me to believe it.
↑ comment by Ted Sanders (ted-sanders) · 2023-06-13T08:19:54.106Z · LW(p) · GW(p)
Thanks for the constructive comments. I'm open-minded to being wrong here. I've already updated a bit and I'm happy to update more.
Regarding the Landauer limit, I'm confused by a few things:
- First, I'm confused by your linkage between floating point operations and information erasure. For example, if we have two 8-bit registers (A, B) and multiply to get (A, B*A), we've done an 8-bit floating point operation without 8 bits of erasure. It seems quite plausible to be that the brain does 1e20 FLOPS but with a much smaller rate of bit erasures.
- Second, I have no idea how to map the fidelity of brain operations to floating point precision, so I really don't know if we should be comparing 1 bit, 8 bit, 64 bit, or not at all. Any ideas?
Regarding training requiring 8e34 floating point operations:
- Ajeya Cotra estimates training could take anything from 1e24 to 1e54 floating point operations, or even more. Her narrower lifetime anchor ranges from 1e24 to 1e38ish. https://docs.google.com/document/d/1IJ6Sr-gPeXdSJugFulwIpvavc0atjHGM82QjIfUSBGQ/edit
- Do you think Cotra's estimates are not just poor, but crazy as well? If they were crazy, I would have expected to see her two-year update mention the mistake, or the top comments to point it out, but I see neither: https://www.lesswrong.com/posts/AfH2oPHCApdKicM4m/two-year-update-on-my-personal-ai-timelines [LW · GW]
↑ comment by Ege Erdil (ege-erdil) · 2023-06-13T09:22:07.631Z · LW(p) · GW(p)
First, I'm confused by your linkage between floating point operations and information erasure. For example, if we have two 8-bit registers (A, B) and multiply to get (A, B*A), we've done an 8-bit floating point operation without 8 bits of erasure. It seems quite plausible to be that the brain does 1e20 FLOPS but with a much smaller rate of bit erasures.
-
As a minor nitpick, if A and B are 8-bit floating point numbers then the multiplication map x -> B*x is almost never injective. This means even in your idealized setup, the operation (A, B) -> (A, B*A) is going to lose some information, though I agree that this information loss will be << 8 bits, probably more like 1 bit amortized or so.
-
The bigger problem is that logical reversibility doesn't imply physical reversibility. I can think of ways in which we could set up sophisticated classical computation devices which are logically reversible, and perhaps could be made approximately physically reversible when operating in a near-adiabatic regime at low frequencies, but the brain is not operating in this regime (especially if it's performing 1e20 FLOP/s). At high frequencies, I just don't see which architecture you have in mind to perform lots of 8-bit floating point multiplications without raising the entropy of the environment by on the order of 8 bits.
Again using your setup, if you actually tried to implement (A, B) -> (A, A*B) on a physical device, you would need to take the register that is storing B and replace the stored value with A*B instead. To store 1 bit of information you need a potential energy barrier that's at least as high as k_B T log(2), so you need to switch ~ 8 such barriers, which means in any kind of realistic device you'll lose ~ 8 k_B T log(2) of electrical potential energy to heat, either through resistance or through radiation. It doesn't have to be like this, and some idealized device could do better, but GPUs are not idealized devices and neither are brains.
Ajeya Cotra estimates training could take anything from 1e24 to 1e54 floating point operations, or even more. Her narrower lifetime anchor ranges from 1e24 to 1e38ish.
Two points about that:
-
This is a measure that takes into account the uncertainty over how much less efficient our software is compared to the human brain. I agree that human lifetime learning compute being around 1e25 FLOP is not strong evidence that the first TAI system we train will use 1e25 FLOP of compute; I expect it to take significantly more than that.
-
Moreover, this is an estimate of effective FLOP, meaning that Cotra takes into account the possibility that software efficiency progress can reduce the physical computational cost of training a TAI system in the future. It was also in units of 2020 FLOP, and we're already in 2023, so just on that basis alone, these numbers should get adjusted downwards now.
Do you think Cotra's estimates are not just poor, but crazy as well?
No, because Cotra doesn't claim that the human brain performs 1e25 FLOP/s - her claim is quite different.
The claim that "the first AI system to match the performance of the human brain might require 1e25 FLOP/s to run" is not necessarily crazy, though it needs to be supported by evidence of the relative inefficiency of our algorithms compared to the human brain and by estimates of how much software progress we should expect to be made in the future.
Replies from: ted-sanders, Muireall↑ comment by Ted Sanders (ted-sanders) · 2023-06-14T00:12:56.382Z · LW(p) · GW(p)
Thanks, that's clarifying. (And yes, I'm well aware that x -> B*x is almost never injective, which is why I said it wouldn't cause 8 bits of erasure rather than the stronger, incorrect claim of 0 bits of erasure.)
To store 1 bit of information you need a potential energy barrier that's at least as high as k_B T log(2), so you need to switch ~ 8 such barriers, which means in any kind of realistic device you'll lose ~ 8 k_B T log(2) of electrical potential energy to heat, either through resistance or through radiation. It doesn't have to be like this, and some idealized device could do better, but GPUs are not idealized devices and neither are brains.
Two more points of confusion:
- Why does switching barriers imply that electrical potential energy is probably being converted to heat? I don't see how that follows at all.
- To what extent do information storage requirements weigh on FLOPS requirements? It's not obvious to me that requirements on energy barriers for long-term storage in thermodynamic equilibrium necessarily bear on transient representations of information in the midst of computations, either because the system is out of thermodynamic equilibrium or because storage times are very short
↑ comment by Ege Erdil (ege-erdil) · 2023-06-14T03:27:57.492Z · LW(p) · GW(p)
Why does switching barriers imply that electrical potential energy is probably being converted to heat? I don't see how that follows at all.
Where else is the energy going to go? Again, in an adiabatic device where you have a lot of time to discharge capacitors and such, you might be able to do everything in a way that conserves free energy. I just don't see how that's going to work when you're (for example) switching transistors on and off at a high frequency. It seems to me that the only place to get rid of the electrical potential energy that quickly is to convert it into heat or radiation.
I think what I'm saying is standard in how people analyze power costs of switching in transistors, see e.g. this physics.se post. If you have a proposal for how you think the brain could actually be working to be much more energy efficient than this, I would like to see some details of it, because I've certainly not come across anything like that before.
To what extent do information storage requirements weigh on FLOPS requirements? It's not obvious to me that requirements on energy barriers for long-term storage in thermodynamic equilibrium necessarily bear on transient representations of information in the midst of computations, either because the system is out of thermodynamic equilibrium or because storage times are very short
The Boltzmann factor roughly gives you the steady-state distribution of the associated two-state Markov chain, so if time delays are short it's possible this would be irrelevant. However, I think that in realistic devices the Markov chain reaches equilibrium far too quickly for you to get around the thermodynamic argument because the system is out of equilibrium.
My reasoning here is that the Boltzmann factor also gives you the odds of an electron having enough kinetic energy to cross the potential barrier upon colliding with it, so e.g. if you imagine an electron stuck in a potential well that's O(k_B T) deep, the electron will only need to collide with one of the barriers O(1) times to escape. So the rate of convergence to equilibrium comes down to the length of the well divided by the thermal speed of the electron, which is going to be quite rapid as electrons at the Fermi level in a typical wire move at speeds comparable to 1000 km/s.
I can try to calculate exactly what you should expect the convergence time here to be for some configuration you have in mind, but I'm reasonably confident when the energies involved are comparable to the Landauer bit energy this convergence happens quite rapidly for any kind of realistic device.
Replies from: ted-sanders↑ comment by Ted Sanders (ted-sanders) · 2023-06-14T04:23:29.428Z · LW(p) · GW(p)
Why does switching barriers imply that electrical potential energy is probably being converted to heat? I don't see how that follows at all.
Where else is the energy going to go?
What is "the energy" that has to go somewhere? As you recognize, there's nothing that says it costs energy to change the shape of a potential well. I'm genuinely not sure what energy you're talking about here. Is it electrical potential energy spent polarizing a medium?
I think what I'm saying is standard in how people analyze power costs of switching in transistors, see e.g. this physics.se post.
Yeah, that's pretty standard. The ultimate efficiency limit for a semiconductor field-effect transistor is bounded by the 60 mV/dec subthreshold swing, and modern tiny transistors have to deal with all sorts of problems like leakage current which make it difficult to even reach that limit.
Unclear to me that semiconductor field-effect transistors have anything to do with neurons, but I don't know how neurons work, so my confusion is more likely a state of my mind than a state of the world.
↑ comment by Ege Erdil (ege-erdil) · 2023-06-14T04:59:33.487Z · LW(p) · GW(p)
I don't think transistors have too much to do with neurons beyond the abstract observation that neurons most likely store information by establishing gradients of potential energy. When the stored information needs to be updated, that means some gradients have to get moved around, and if I had to imagine how this works inside a cell it would probably involve some kind of proton pump operating across a membrane or something like that. That's going to be functionally pretty similar to a capacitor, and discharging & recharging it probably carries similar free energy costs.
I think what I don't understand is why you're defaulting to the assumption that the brain has a way to store and update information that's much more efficient than what we're able to do. That doesn't sound like a state of ignorance to me; it seems like you wouldn't hold this belief if you didn't think there was a good reason to do so.
Replies from: ted-sanders↑ comment by Ted Sanders (ted-sanders) · 2023-06-14T06:55:02.400Z · LW(p) · GW(p)
I think what I don't understand is why you're defaulting to the assumption that the brain has a way to store and update information that's much more efficient than what we're able to do. That doesn't sound like a state of ignorance to me; it seems like you wouldn't hold this belief if you didn't think there was a good reason to do so.
It's my assumption because our brains are AGI for ~20 W.
In contrast, many kW of GPUs are not AGI.
Therefore, it seems like brains have a way of storing and updating information that's much more efficient than what we're able to do.
Of course, maybe I'm wrong and it's due to a lack of training or lack of data or lack of algorithms, rather than lack of hardware.
DNA storage is way more information dense than hard drives, for example.
Replies from: ege-erdil↑ comment by Ege Erdil (ege-erdil) · 2023-06-14T07:00:00.928Z · LW(p) · GW(p)
It's my assumption because our brains are AGI for ~20 W.
I think that's probably the crux. I think the evidence that the brain is not performing that much computation is reasonably good, so I attribute the difference to algorithmic advantages the brain has, particularly ones that make the brain more data efficient relative to today's neural networks.
The brain being more data efficient I think is hard to dispute, but of course you can argue that this is simply because the brain is doing a lot more computation internally to process the limited amount of data it does see. I'm more ready to believe that the brain has some software advantage over neural networks than to believe that it has an enormous hardware advantage.
↑ comment by Muireall · 2023-06-13T13:06:51.178Z · LW(p) · GW(p)
Moreover, this is an estimate of effective FLOP, meaning that Cotra takes into account the possibility that software efficiency progress can reduce the physical computational cost of training a TAI system in the future. It was also in units of 2020 FLOP, and we're already in 2023, so just on that basis alone, these numbers should get adjusted downwards now.
Isn't it a noted weakness of Cotra's approach that most of the anchors don't actually depend on 2020 architecture or algorithmic performance in any concrete way? As in, if the same method were applied today, it would produce the same numbers in "2023 FLOP"? This is related to why I think the Beniaguev paper is pretty relevant exactly as evidence of "inefficiency of our algorithms compared to the human brain".
↑ comment by Muireall · 2023-06-10T03:49:55.066Z · LW(p) · GW(p)
If I understand correctly, the claim isn't necessarily that the brain is "doing" that many FLOP/s, but that using floating point operations on GPUs to do the amount of computation that the brain does (to achieve the same results) is very inefficient. The authors cite Single cortical neurons as deep artificial neural networks (Beniaguev et al. 2021), writing, "A recent attempt by Beniaguev et al to estimate the computational complexity of a biological neuron used neural networks to predict in-vitro data on the signal activity of a pyramidal neuron (the most common kind in the human brain) and found that it took a neural network with about 1000 computational “neurons” and hundreds of thousands of parameters, trained on a modern GPU for several days, to replicate its function." If you want to use a neural network to do the same thing as a cortical neuron, then one way to do it is, following Beniaguev et al., to run a 7-layer, width-128 temporal convolutional network with 150 ms memory every millisecond. A central estimate of 1e32 FLOP to get the equivalent of 30 years of learning (1e9 seconds) with 1e15 biological neurons synapses does seem reasonable from there. (With 4 inputs/filters, , if I haven't confused myself.)
That does imply the estimate is an upper bound on computational costs to emulate a neuron with an artificial neural network, although the authors argue that it's likely fairly tight. It also implies the brain is doing its job much more efficiently than we know how to use an A100 to do it, but I'm not sure why that should be particularly surprising. It's also true that for some tasks we already know how to do much better than emulating a brain.
Replies from: ege-erdil↑ comment by Ege Erdil (ege-erdil) · 2023-06-10T04:47:17.238Z · LW(p) · GW(p)
Recapitulating the response of Steven Byrnes to this argument: it may be very expensive computationally to simulate a computer in a faithful way, but that doesn't mean it's expensive to do the same computation that the computer in question is doing. Paraphrasing a nice quote from Richard Borcherds, it may be that teapots are very hard to simulate on a classical computer, but that doesn't mean that they are useful computational devices.
If we tried to simulate a GPU doing a simple matrix multiplication at high physical fidelity, we would have to take so many factors into account that the cost of our simulation would far exceed the cost of running the GPU itself. Similarly, if we tried to program a physically realistic simulation of the human brain, I have no doubt that the computational cost of doing so would be enormous.
However, this is not what we're interested in doing. We're interested in creating a computer that's doing the same kind of computation as the brain, and the amount of useful computation that the brain could be doing per second is much less than 1e25 or even 1e20 FLOP/s. If your point is that 1e25 FLOP/s is an upper bound on how much computation the brain is doing, I agree, but there's no reason to think it's a tight upper bound.
It also implies the brain is doing its job much more efficiently than we know how to use an A100 to do it, but I'm not sure why that should be particularly surprising.
This claim is different from the claim that the brain is doing 1e20 FLOP/s of useful computation, which is the claim that the authors actually make. If you have an object that implements some efficient algorithm that you don't understand, the object can be doing little useful computation even though you would need much greater amounts of computation to match its performance with a worse algorithm. The estimates coming from the brain are important because they give us a sense of how much software efficiency progress ought to be possible here.
My argument from the Landauer limit is about the number of bit erasures and doesn't depend on the software being implemented by the brain vs. a GPU. If the brain is doing something that's in some sense equivalent to 1e20 floating point operations per second, based on its power consumption that would imply that it's operating basically at the Landauer limit, perhaps only one order of magnitude off. Just the huge amount of noise in brain interconnect should be enough to discredit this estimate. Whether the brain is specialized to perform one or another kind of task is not relevant for this calculation.
Perhaps you think the brain has massive architectural or algorithmic advantages over contemporary neural networks, but if you do, that is a position that has to be defended on very different grounds than "it would take X amount of FLOP/s to simulate one neuron at a high physical fidelity".
Replies from: ted-sanders, Muireall↑ comment by Ted Sanders (ted-sanders) · 2023-06-13T08:22:57.722Z · LW(p) · GW(p)
If we tried to simulate a GPU doing a simple matrix multiplication at high physical fidelity, we would have to take so many factors into account that the cost of our simulation would far exceed the cost of running the GPU itself. Similarly, if we tried to program a physically realistic simulation of the human brain, I have no doubt that the computational cost of doing so would be enormous.
The Beniaguev paper does not attempt to simulate neurons at high physical fidelity. It merely attempts to simulate their outputs, which is a far simpler task. I am in total agreement with you that the computation needed to simulate a system is entirely distinct from the computation being performed by that system. Simulating a human brain would require vastly more than 1e21 FLOPS.
↑ comment by Muireall · 2023-06-10T11:18:54.243Z · LW(p) · GW(p)
This claim is different from the claim that the brain is doing 1e20 FLOP/s of useful computation, which is the claim that the authors actually make.
Is it? I suppose they don't say so explicitly, but it sounds like they're using "2020-equivalent" FLOPs (or whatever it is Cotra and Carlsmith use), which has room for "algorithmic progress" baked in.
Perhaps you think the brain has massive architectural or algorithmic advantages over contemporary neural networks, but if you do, that is a position that has to be defended on very different grounds than "it would take X amount of FLOP/s to simulate one neuron at a high physical fidelity".
I may be reading the essay wrong, but I think this is the claim being made and defended. "Simulating" a neuron at any level of physical detail is going to be irrelevantly difficult, and indeed in Beniaguev et al., running a DNN on a GPU that implements the computation a neuron is doing (four binary inputs, one output) is a 2000X speedup over solving PDEs (a combination of compression and hardware/software). They find it difficult to make the neural network smaller or shorter-memory, suggesting it's hard to implement the same computation more efficiently with current methods.
Replies from: ege-erdil↑ comment by Ege Erdil (ege-erdil) · 2023-06-10T14:28:28.935Z · LW(p) · GW(p)
I think you're just reading the essay wrong. In the "executive summary" section, they explicitly state that
Our best anchor for how much compute an AGI needs is the human brain, which we estimate to perform 1e20–1e21 FLOPS.
and
In addition, we estimate that today’s computer hardware is ~5 orders of magnitude less cost efficient and energy efficient than brains.
I don't know how you read those claims and arrived at your interpretation, and indeed I don't know how the evidence they provide could support the interpretation you're talking about. It would also be a strange omission to not mention the "effective" part of "effective FLOP" explicitly if that's actually what you're talking about.
Replies from: Muireall↑ comment by Muireall · 2023-06-10T15:31:13.086Z · LW(p) · GW(p)
Thanks, I see. I agree that a lot of confusion could be avoided with clearer language, but I think at least that they're not making as simple an error as you describe in the root comment. Ted does say in the EA Forum thread that they don't believe brains operate at the Landauer limit, but I'll let him chime in here if he likes.
I think the "effective FLOP" concept is very muddy, but I'm even less sure what it would mean to alternatively describe what the brain is doing in "absolute" FLOPs. Meanwhile, the model they're using gives a relatively well-defined equivalence between the logical function of the neuron and modern methods on a modern GPU.
The statement about cost and energy efficiency as they elaborate in the essay body is about getting human-equivalent task performance relative to paying a human worker $25/hour, not saying that the brain uses five orders of magnitude less energy per FLOP of any kind. Closing that gap of five orders of magnitude could come either from doing less computation than the logical-equivalent-neural-network or from decreasing the cost of computation.
comment by GdL752 · 2023-06-07T16:51:38.946Z · LW(p) · GW(p)
I guess I just feel completely different about those conditional probabilities.
Unless we hit another AI winter the profit and national security incentives just snowball right past almost all of those. Regulation? "Severe depression"
I admit that thr loss of taiwan does innfact set back chip manufactyre by a decade or more regardless of resoyrces thrown at it but every other case just seems way off (because of the incentive structure)
So we're what , 3 months post chatgpt and customer service and drive throughs are solved or about to be solved? , so lets call that the lowrst hanging fruit. So just some quick back of the napkin google fu , the customer service by itself is a 30 billion dollar industry just in the US.
And how much more does the math break down if say , we have an AGI that can do construction work (embodied in a robot) at say 90% human efficiency for...27 dollars an hour?
In my mind every human task fully (or fully enough) automated snowballs the economic incentive and pushes more resources and man hours into solving problems with material science and things like...idk piston designs or multifunctionality or whatever.
I admit I'm impressed by the collected wisdom and apparent track records of these authors but it seems like its missing the key drivers for further improvement in the analysis.
Like would the authors have put the concept of a smartphone at 1% by 2020 if asked in 2001 based on some abnormally high conditionals about seemingly rational but actually totally orthagonal concern based on how well palm pilots did?
I also dont see how the semi conductor fab bottleneck is such a thing? , 21 million users of openai costs 700k a day to run.
So taking some liberties here but thats 30 bucks a person (so a loss with their current model but thats not my point)
If some forthcoming iteration with better cognitive architecture etc costs about that then we have , 1.25$ per hour to replace a human "thinking" job.
Im having trouble seeing how we don't rapidly advance robotics and chip manufacture and mining and energy production etc when we stumble into a world where thats the only bottleneck standing in our way to 100% replacemwnt of all useful human labor.
Again , you got the checkout clerks at grocery stores last decade. 3 months in and the entire customer service industry is on its knees. Even if you only get 95% as good as a human and have to sort of take things one at a time to start with , all that excess productivity and profit then chases the next thing. It snowballs from here.
Replies from: AnthonyC↑ comment by AnthonyC · 2023-06-08T19:23:17.524Z · LW(p) · GW(p)
I agree the point about freeing up resources and shifting incentives as we make progress is very important.
Also, if your 21 million open AI users for $700k/day numbers are right, that's $1/user/month, not $30/user/day. Unless I'm just misreading this.
comment by Erich_Grunewald · 2023-06-06T23:39:47.860Z · LW(p) · GW(p)
I think this is an excellent, well-researched contribution and am confused about why it's not being upvoted more (on LW that is; it seems to be doing much better on EAF, interestingly).
Replies from: T3t↑ comment by RobertM (T3t) · 2023-06-07T00:37:02.195Z · LW(p) · GW(p)
At a guess (not having voted on it myself): because most of the model doesn't engage with the parts of the question that those voting consider interesting/relevant, such as the many requirements laid out for "transformative AI" which don't see at all necessary for x-risk. While this does seem to be targeting OpenPhil's given definition of AGI, they do say in a footnote:
What we’re actually interested in is the potential existential threat posed by advanced AI systems.
While some people do have AI x-risk models that route through ~full automation (or substantial automation, with a clearly visible path to full automation), I think most people here don't have models that require that, or even have substantial probability mass on it.
Replies from: ted-sanders↑ comment by Ted Sanders (ted-sanders) · 2023-06-07T08:00:35.967Z · LW(p) · GW(p)
Interesting. When I participated in the AI Adversarial Collaboration Project, a study funded by Open Philanthropy and executed by the Forecasting Research Institute, I got the sense that most folks concerned about AI x-risk mostly believed that AGIs would kill us on their own accord (rather than by accident or as a result of human direction), that AGIs would have self-preservation goals, and therefore AGIs would likely only kill us after solving robotic supply chains (or enslaving/manipulating humans, as I argued as an alternative).
Sounds like your perception is that LessWrong folks don't think robotic supply chain automation will be a likely prerequisite to AI x-risk?
Replies from: steve2152, T3t↑ comment by Steven Byrnes (steve2152) · 2023-06-08T14:44:40.926Z · LW(p) · GW(p)
There’s an interesting question: if a power-seeking AI had a button that instantly murdered every human, how much human-requiring preparatory work would it want to do before pressing the button? People seem to have strongly clashing intuitions here, and there aren’t any great writeups IMO. Some takes on the side of “AI wouldn’t press the button until basically the whole world economy was run by robots” are 1 [LW · GW], 2 [LW(p) · GW(p)], 3 [LW(p) · GW(p)], 4, 5 [LW · GW]. I tend to be on the other side, for example I wrote here [LW · GW]:
it seems pretty plausible to me that if there's an AGI server and a solar cell and one teleoperated robot body in an otherwise-empty post-apocalyptic Earth, well then that one teleoperated robot body could build a janky second teleoperated robot body from salvaged car parts or whatever, and then the two of them could find more car parts to build a third and fourth, and those four could build up to eight, etc. That was basically the story I was telling here [LW · GW].…
Some cruxes:
- One crux on that is how much compute is needed to run a robot—if it’s “1 consumer-grade GPU” then my story above seems to work, if it’s “10⁶ SOTA GPUs” then probably not.
- Another crux is how much R&D needs to be done before we can build a computational substrate using self-assembling nanotechnology (whose supply chain / infrastructure needs are presumably much much lower than chip fabs). This is clearly possible, since human brains are in that category, but it’s unclear just how much R&D needs to be done before an AI could start doing that.
- For example, Eliezer is optimistic (umm, I guess that’s the wrong word) that this is doable without very much real-world experimenting (as opposed to “thinking” and doing simulations / calculations via computer), and this path is part of why he expects AI might kill every human seemingly out of nowhere.
- Another crux is just how minimal is a “minimal supply chain that can make good-enough chips” if the self-assembling route of the previous bullet point is not feasible. Such a supply chain would presumably be very very different from the supply chain that humans use to make chips, because obviously we’re not optimizing for that. As a possible example, e-beam lithography (EBL) is extraordinarily slow and expensive but works even better than EUV photolithography, and it’s enormously easier to build a janky EBL than to get EUV working. A commercial fab in the human world would never dream of mass-manufacturing chips by filling giant warehouses with zillions of janky and slow EBL machines, because the cost would be astronomical, the market can’t support that. But for an AI rebuilding in an empty postapocalyptic world, why not? And that’s just one (possible) example.
↑ comment by RobertM (T3t) · 2023-06-07T23:19:43.192Z · LW(p) · GW(p)
Robotic supply chain automation only seems necessary in worlds where it's either surprisingly difficult to get AGI to a sufficiently superhuman level of cognitive ability (such that it can find a much faster route to takeover), worlds where faster/more reliable routes to takeover either don't exist or are inaccessible even to moderately superhuman AGI, or some combination of the two.
comment by plex (ete) · 2023-06-10T16:47:56.094Z · LW(p) · GW(p)
Your probabilities are not independent, your estimates mostly flow from a world model which seem to me to be flatly and clearly wrong.
The plainest examples seem to be assigning
We invent a way for AGIs to learn faster than humans | 40% |
AGI inference costs drop below $25/hr (per human equivalent) | 16% |
despite current models learning vastly faster than humans (training time of LLMs is not a human lifetime, and covers vastly more data) and the current nearing AGI and inference being dramatically cheaper and plummeting with algorithmic improvements. There is a general factor of progress, where progress leads to more progress, which you seem to be missing in the positive factors. For the negative, derailment that delays enough to push us out that far needs to be extreme, on the order of a full-out nuclear exchange, given more reasonable models of progress.
I'll leave you with Yud's preemptive reply:
Taking a bunch of number and multiplying them together causes errors to stack, especially when those errors are correlated.
comment by AnthonyC · 2023-06-06T19:30:03.624Z · LW(p) · GW(p)
Thanks, the parts I've read so far are really interesting!
I would point out that the claim that we will greatly slow down, rather than scale up, electricity production capacity, is also a claim that we will utterly fail to even come anywhere close to hitting global decarbonization goals. Most major sectors will require much more electricity in a decarbonized world, as in raising total production (not just capacity) somewhere between 3x to 10x in the next few decades. This is much more than the additional power which would be needed to increase chip production as described, or to power the needed compute. The amount of silicon needed for solar panels also dwarfs that needed for wafers (yes, I know, very different quality thresholds, but still). Note that because solar power is currently the cheapest in the world, and still falling, we should also expect electricity production costs to go down over time.
I think it's also worthwhile to note that the first "we" in the list means humans, but every subsequent "we" includes the transformative AI algorithm we've learned out to build, and all the interim less capable AIs we'll be building between now and then. Not sure if/how you're trying to account for this?
comment by weverka · 2023-06-09T20:34:57.192Z · LW(p) · GW(p)
Compute is not the limiting factor for mammalian intelligence. Mammalian brains are organized to maximize communication. The gray matter, where most compute is done, is mostly on the surface and the white matter which dominate long range communication, fills the interior, communicating in the third dimension.
If you plot volume of white matter vs. gray matter across the various mammal brains, you find that the volume of white matter grows super linearly with volume of gray matter. https://www.pnas.org/doi/10.1073/pnas.1716956116
As brains get larger, you need a higher ratio of communication/compute.
Your calculations, and Cotras as well, focus on FLOPs but the intelligence is created by communication.
Replies from: ted-sanders↑ comment by Ted Sanders (ted-sanders) · 2023-06-09T21:41:19.407Z · LW(p) · GW(p)
Interesting! How do you think this dimension of intelligence should be calculated? Are there any good articles on the subject?
comment by frontier64 · 2023-06-09T19:52:09.759Z · LW(p) · GW(p)
Does this do the thing where a bunch of related events are treated as independent events and their probability is multiplied together to achieve a low number?
edit: I see you say that each event is conditioned on the previous events being true. It doesn't seem like you took that into account when you formulated your own probabilities.
According to your probabilities, in the world where: We invent algorithms for transformative AGI, We invent a way for AGIs to learn faster than humans, AGI inference costs drop below $25/hr (per human equivalent), and We invent and scale cheap, quality robots... There's only a 46% chance that we massively scale production of chips and power. That seems totally unreasonable on its face. How could cheap, quality robots at scale not invariably lead to a massive scaling of the production of chips and power?
I think splitting the odds up this way just isn't helpful. Your brain is not too good at pondering, "What is the probability of X happening if A, B, C, ... W all happen?" Too much of "What is the probability of X independent of everything else" will creep in. Unless you have a mechanistic process for determining the probability of X
Replies from: ted-sanders↑ comment by Ted Sanders (ted-sanders) · 2023-06-09T21:34:29.561Z · LW(p) · GW(p)
Conditioning does not necessarily follow time ordering. E.g., you can condition the odds of X on being in a world on track to develop robots by 2043 without having robots well in advance of X. Similarly, we can condition on a world where transformative AGI is trainable with 1e30 floating point operations then ask the likelihood that 1e30 floating point operations can be constructed and harnessed for TAGI. Remember too that in a world with rapidly advancing AI and robots, much of the demand will be for things other than TAGI.
I'm sympathetic to your point that it's hard for brains to forecast these conditional probabilities. Certainly we may be wrong. But on the other hand, it's also hard for brains to forecast things that involve smushing lots of probabilities together under the hood. I generally think that factoring things out into components helps, but I can understand if you disagree.
Replies from: martin-randall↑ comment by Martin Randall (martin-randall) · 2023-06-13T02:18:06.460Z · LW(p) · GW(p)
Here is the world I am most interested in, where the conditional probability seems least plausible:
- We invent algorithms for transformative AGI
- We invent a way for AGIs to learn faster than humans
- AGI inference costs drop below $25/hr
- We invent and scale cheap, quality robots
- We massively scale production of chips and power
- We avoid derailment by human regulation
- We avoid derailment by AI-caused delay
In this world, what is the probability that we were "derailed" by wars, such as China invading Taiwan?
Reading the paper naively, it says that there is a 30% chance that we achieved all of this technical progress, in the 99th percentile of possible outcomes, despite China invading Taiwan. That doesn't seem like a 30% chance to me. Additionally, if China invaded Taiwan, but it didn't prevent us achieving all this technical progress, in what sense was it a derailment? The executive summary suggests:
Even if an invasion doesn’t spark war, the sanctions applied in retaliation will shut down most of the world’s AI chip production
No, it can't possibly derail AI by shutting down chip production, in this conditional branch, because we already know from item 5 that we massively scaled chip production, and both things can't be true at the same time.
Replies from: ted-sanders↑ comment by Ted Sanders (ted-sanders) · 2023-06-13T08:26:37.370Z · LW(p) · GW(p)
Right. The idea is: "What are the odds that China invading Taiwan derails chip production conditional on a world where we were otherwise going to successfully scale chip production."
Replies from: martin-randall↑ comment by Martin Randall (martin-randall) · 2023-06-15T02:42:30.845Z · LW(p) · GW(p)
I would not have guessed that! So in slightly more formal terms:
- CHIPS = There are enough chips for TAGI by 2043
- WAR = There is a war that catastrophically derails chip production by 2043
- P(x) = subjective probability of x
- ObjP(x) = objective probability of x
- P(CHIPS and WAR) = 0% (by definition)
Then as I understand your method, it goes something like:
- Estimate P(CHIPS given not WAR) = 46%
- This means that in 46% of worlds, ObjP(CHIPS given not WAR) = 100%. Call these worlds CHIPPY worlds. In all other worlds ObjP(CHIPS given not WAR) = 0%.
- Estimate P(not WAR given CHIPPY) = 70%.
- The only option for CHIPS is "not WAR and CHIPPY".
- Calculate P(not WAR and CHIPPY) = 70% x 46% = 32.2%.
- Therefore P(CHIPS) = 32.2%.
(probabilities may differ, this is just illustrative)
However, I don't think the world is deterministic enough for step 2 to work - the objective probability could be 50% or some other value.
Replies from: ted-sanders↑ comment by Ted Sanders (ted-sanders) · 2023-06-15T21:22:56.126Z · LW(p) · GW(p)
Bingo
comment by [deleted] · 2023-06-09T09:18:27.533Z · LW(p) · GW(p)
We invent algorithms for transformative AGI | 60% |
We invent algorithms for transformative AGI | 60% |
We invent a way for AGIs to learn faster than humans | 40% |
AGI inference costs drop below $25/hr (per human equivalent) | 16% |
We invent and scale cheap, quality robots | 60% |
We massively scale production of chips and power | 46% |
We avoid derailment by human regulation | 70% |
We avoid derailment by AI-caused delay | 90% |
We avoid derailment from wars (e.g., China invades Taiwa | 70% |
We avoid derailment from pandemics | 90% |
We avoid derailment from severe depression | 95% |
I'm going to try a better calculation on what I think are the pivotal cruxes.
Algorithms, as I mentioned in my last comment [LW(p) · GW(p)], depends on compute. Iff enough training compute exists by 2043, then it is inevitable that a tAGI grade algorithm will be found, simply through RSI.
Learning faster than humans is just "algorithms". Ted Sanders [LW · GW], you stated that autonomous cars not being as good as humans was because they "take time to learn". This is completely false, this is because the current algorithms in use, especially the cohesive software and hardware systems and servers around the core driving algorithms, have bugs. If we invent better algorithms that can generalize better, train on more examples, and using the same or a different tAGI system, seal up the software and firmware and system design stack by hunting down all the bugs that can be found with a careful review of every line (in assembly!), and seal up the chip design, so that you have a clean design with no known errata - all things humans are capable of doing already, we just can't afford the labor hours to do this - then the actual Driver agent can learn it's job in a few months.
So compute's a big part of the low probability.
Let's calculate this one fresh.
Epistemic status: i have a master's in biomedical science, which included a medical school neuroscience course, a bachelor's in computer engineering, and a master's in CS, and I work as a platform engineer on an AI accelerator project.
Doing it from the bio anchors view:
There are approximately 86 billion neurons in the human brain, with approximately 1000 connections each. Noise is extremely high, we're going to be generous and assume 8 bits of precision, which is likely more than the brain actually achieves. We'll be generous and assuming 1 khz and that all connections are active.
Both are false, and the asynchronous calculations and noise cause a lot of error that a comparable synthetic system will not make. This is the reason why you can safely knock 1-2 orders of magnitude right off the top, this is well justified. Timing jitter alone from a lack of a central clock injects noise into all the synapses, synapses are using analog accumulators that change their charge levels from crosstalk and many forms of biological noise (digital accumulators reject such noise under conditions insufficient to cause a bit flip). I am not accounting for this.
So we need 8.6e+16, or 10^17 flops in int8. I'll have to check the essay to see where you went wrong, but 10^20 is clearly not justified. Arguably flat out wrong.
With the current gen H100 and 2000 dense int8 tops, you would need 43 H100s.
Compute isn't the issue, VRAM is. 43 H100s only have 3440 gb of VRAM.
We need 16 bit accumulators on 8 bit weights, and there's VRAM lost from the very high sparseness of the human brain. So for a quick estimate, if we consume 8 bytes per synapse, we need 688 terabytes of VRAM, or 400 H100s.
So the estimate narrows to, present day, [43, 400] H100s. I think this is a pretty hard estimate, you're going to need to produce substantial evidence to disprove. The reason it's so firm is because you probably need 1/10 or less the above because the brain makes so many errors and randomly loses cells, so to stay alive we have a lot of redundant synapses that contain the same information. An efficient tAGI design can obviously reliably know each weight will always be factored in every timestep, and will always be available, and ECC memory is common so undetected errors will rarely happen.
During inference time, how many H100s equal "one human equivalent"? The answer may surprise you. It's about 18.4. The reason is that the hardest working humans, working "996" (12 hours a day, 6 days a week) are still only working 42% of the time, while H100s work 24/7.
The reason you don't need 400 is because you batch the operations. There will be approximately 20 "human level" sessions across an array of 400 H100s, running a model that has unique weights tiled across all 400 cards, since the compute load per human level session is only about 43. You can reduce the batch size if you want better latency, we do that for autonomous cars.
H100s now cost about $2.33 an hour.
So present day, the cost is $100.19 an hour.
I thought I was going to have to argue for better than the 38x hardware improvement you estimated, mention each H100 actually costs about $2500 to build not $25k, or describe a method that is essentially the brain's System 1 and System 2, but there's no need, compute isn't a problem. I'm sure you can see that getting a factor of 4 reduction is fairly trivial.
Unless I made a major error, I think you may need a rewrite/retraction, as you're way off. I look forward to hearing your response. Ted, how do you not know this, you can just ping a colleague and check the math above. Anyone at OpenAI that does the modeling for infrastructure will be able to check whether or not the above is correct or not.
Remaining terms: robotics is a big open. I will simply accept your 60% though arguably in a world where the tAGI algorithms exist, and are cheap to run, there would be total war levels of effort put into robot manufacturing. Not to mention it will self amplify, as previously built robots contribute their labor to some of the tasks involved in building more robots.
Massively scaling chips and power, at <$25 per human equivalent, would happen inherently, being able to get a human equivalent worker that is reliable for this low a cost creates immense economic pressure to do this. It's also coupled to cheap robotics - repetitive tasks like manufacturing and building solar panels and batteries, both of which are fundamentally a product that you 'tile', every solar cell and every battery like all it's peers, are extremely automatable. Data centers could be air cooled and installed in large deserts, you would just accept the latency, using local hardware for the real-time control, and you would accept a less than 100% level of service, so compute availability would fluctuate with the seasons.
All the other terms: human regulation, AI-disaster, wars, pandemics, economic depression have the issue that each is conditional on all the relevant powers fail the same way. So all the probability estimates need to be raised to the 3rd power, conservatively.
Conclusion: iff my calculation on the compute required is possible, this problem collapses. Only the tAGI algorithm matters. In worlds where the tAGI algorithm was discovered at least 5 years before 2043, tAGI is closer to certain. Only extreme events - nuclear war, every tAGI becomes rampant in insidious and dangerous ways, coordinated worldwide efforts to restrict tAGI development - could derail it from there.
As for thinking again about the broader picture: your "90% of tasks" requirement, below which apparently the machine is not transformative, which seems like a rather bad goalpost, is the issue.
A reasonable tAGI algorithm is a multimodal algorithm, trained on a mixture of supervised and reinforcement learning, that is capable of learning any task to expert level iff it receives sufficient feedback on task quality to become an expert. So there's AI scientists and AI programmers and AI grammer editors and many forms of AI doing manufacturing.
But can they write books that outsell all human authors? Depends on how much structured data they get on human enjoyment of text samples.
Can they write movies that are better than human screenwriters? Same limitation.
Can they go door to door and evangelize? Depends on how much humans discriminate against robots.
There are a whole bunch of tasks that arguably do not matter that would fit into that 90%. They don't matter because the class of tasks that do matter - self improving AI, robots, and technology - all are solvable. "matter" defined by "did humans require the task to be done at all to live" or "does the task need to be done before robots can tear down the solar system". If the answer is no, it doesn't matter. Car salesman would be an example of a task that doesn't matter. tAGI never needs to know how to do it since it can just offer fixed prices through a web page, and answer any questions about the car or transaction based on published information instead of acting in a way motivated to maximize commission.
A world where the machine can only do 50% of tasks, including all the important ones, but legal regulations means it can't watch children or argue a case in court or supervise other AI or serve in government - is still a transformed one.
Replies from: ted-sanders↑ comment by Ted Sanders (ted-sanders) · 2023-06-09T18:39:18.600Z · LW(p) · GW(p)
I agree with your cruxes:
Ted Sanders [LW · GW], you stated that autonomous cars not being as good as humans was because they "take time to learn". This is completely false, this is because the current algorithms in use, especially the cohesive software and hardware systems and servers around the core driving algorithms, have bugs.
I guess it depends what you mean by bugs? Kind of a bummer for Waymo if 14 years and billions invested was only needed because they couldn't find bugs in their software stack.
If bugs are the reason self-driving is taking so long, then our essay is wildly off.
So present day, the cost is $100.19 an hour.
Yes, if with present day hardware we can effectively emulate a human brain for $100/hr, then our essay is wildly off.
comment by followthesilence · 2023-06-07T17:11:17.112Z · LW(p) · GW(p)
Can you explain how Events #1-5 from your list are not correlated?
For instance, I'd guess #2 (learns faster than humans) follows naturally -- or is much more likely -- if #1 (algos for transformative AI) comes to pass. Similarly, #3 (inference costs <$25/hr) seems to me a foregone conclusion if #5 (massive chip/power scale) and #2 happen.
Treating the first five as conditionally independent puts you at 1% before arriving at 0.4% with external derailments, so it's doing most of the work to make your final probability miniscule. But I suspect they are highly correlated events and would bet a decent chunk of money (at 100:1 odds, at least) that all five come to pass.
Replies from: Erich_Grunewald↑ comment by Erich_Grunewald · 2023-06-07T19:16:30.346Z · LW(p) · GW(p)
They state that their estimated probability for each event is conditional on all previous events happening.
Replies from: followthesilence↑ comment by followthesilence · 2023-06-07T19:32:15.036Z · LW(p) · GW(p)
Thanks, I suppose I'm taking issue with sequencing five distinct conditional events that seem to be massively correlated with one another. The likelihoods of Events 1-5 seem to depend upon each other in ways such that you cannot assume point probabilities for each event and multiply them together to arrive at 1%. Event 5 certainly doesn't require Events 1-4 as a prerequisite, and arguably makes Events 1-4 much more likely if it comes to pass.
Replies from: steve2152↑ comment by Steven Byrnes (steve2152) · 2023-06-07T20:27:50.576Z · LW(p) · GW(p)
It’s a mathematical identity that
This doesn’t depend on A happening chronologically before or after B etc., it’s a true mathematical identity regardless.
This doesn’t depend on these things being uncorrelated. The formula is true even in the extreme case where two or more of these things are 100% perfectly correlated. (…In which case one or more of the factors on the right are going to be 1.0.)
You’re entitled to argue that , and you’re entitled to argue that people are assigning conditional probabilities in a wrong and confused way for whatever reason (e.g. see discussion here [LW · GW]), but you can’t argue with the mathematical identity, right?
Replies from: followthesilence↑ comment by followthesilence · 2023-06-07T22:32:08.865Z · LW(p) · GW(p)
Apologies, I'm not trying to dispute math identities. And thank you, the link provided helps put words to my gut concern: that this essay's conclusion relies heavily on a multi-stage fallacy, and arriving at point probability estimates for each event independently is fraught/difficult.
comment by Shmi (shminux) · 2023-06-07T02:45:48.931Z · LW(p) · GW(p)
I am confused about your use of the term "calibration". Usually it means correctly predicting the probabilities of events, as measured by frequencies. You are listing all the times you were right, without assigning your predicted probability. Do you list only high-probability predictions and conclude that you are well calibrated for, say, 95%+ predictions, since "no TAI by 2043" is estimated to be 99%+?
Replies from: ted-sanders↑ comment by Ted Sanders (ted-sanders) · 2023-06-07T07:55:01.044Z · LW(p) · GW(p)
Yeah, that's a totally fair criticism. Maybe a better header would be "evidence of accuracy." Though even that is a stretch given we're only listing events in the numerators. Maybe "evidence we're not crackpots"?
Edit: Probably best would be "Forecasting track record." This is what I would have gone with if rewriting the piece today.
Edit 2: Updated the post.
comment by Steven Byrnes (steve2152) · 2023-06-07T02:33:26.437Z · LW(p) · GW(p)
RE robots / “Element 4”:
IMO the relevant product category here should be human-teleoperated robots, not independent robots.
Robot-control algorithms are not an issue, since we’re already conditioning on Element 1 (algorithms for TAI). Humans can teleoperate a teleoperable robot, and therefore if Element 1 comes to pass, those future AI algorithms will be able to teleoperate a teleoperable robot too, right?
And human-teleoperated robots can already fold sheets, move boxes, get around a cluttered environment, etc., no problem. I believe this has been true for a very long time.
And when I look at teleoperated robots, I find things like Ugo which might or might not be vaporware but they mention a price point below $10/day. (ETA: see also the much more hardcore Sarcos Guardian XT. Pricing is not very transparent, but one site says you leas it for $5K/month, which isn’t bad considering how low the volumes are.)
More generally, I think “progress in robotics” is not the right thing to be looking at in this context, because “progress in robotics” has been mainly limited by algorithms (and on-board compute). I think teleoperated robots are technologically much simpler, more like the kind of thing that a wide variety of companies can crank out at scale, almost as soon as there’s demand.
Replies from: ted-sanders↑ comment by Ted Sanders (ted-sanders) · 2023-06-07T08:04:28.446Z · LW(p) · GW(p)
If humans can teleoperate robots, why don't we have low-wage workers operating robots in high-wage countries? Feels like a win-win if the technology works, but I've seen zero evidence of it being close. Maybe Ugo is a point in favor?
Replies from: steve2152↑ comment by Steven Byrnes (steve2152) · 2023-06-07T15:01:58.603Z · LW(p) · GW(p)
Hmm. That’s an interesting question: If I’m running a warehouse in a high-wage country, why not have people in low-wage countries teleoperating robots to pack boxes etc.? I don’t have a great answer. My guesses would include possible issues with internet latency & unreliability in low-wage countries, and/or market inefficiencies e.g. related to the difficulty of developing new business practices (e.g. limited willingness/bandwidth of human warehouse managers to try weird experiments), and associated chicken-and-egg issues where the requisite tech doesn’t exist because there’s no market for it and vice-versa. There might also be human-UI issues that limit robot speed / agility (and wouldn’t apply to AIs)?
Of course the “teleoperated robot tech is just super-hard and super-expensive, much moreso than I realize” theory is also a possibility. I’m interested if anyone else has a take. :)
Replies from: Archimedes↑ comment by Archimedes · 2023-06-08T01:46:53.481Z · LW(p) · GW(p)
There are still HR and legal overhead costs involved if you have human operators.
I think part of the answer is also that the space of things low-wage workers can physically do remotely via teleoperation isn't that much larger than the space of things that can be fully automated but still much smaller than the space of things a local human can do. It's a fairly narrow band to exploit, IMO, and the labor cost arbitrage spread is rarely worth the complexity of the extra logistics, capital investment, and maintenance.
comment by Mitchell_Porter · 2023-06-07T00:37:10.753Z · LW(p) · GW(p)
If we divide the list of "10 necessary events" into two groups of five, the first five being technical achievements and the last five being ways to derail technological society... then I suppose the standard doomer view would be that once the first necessary event is achieved (AGI algorithms) then the other technical achievements become 100% possible (edit: because AI figures out how to do them); and that whether or not the derailing events occur, boils down to whether AI lets them happen.
edit: The implication being that algorithmic progress controls everything else.
comment by Sachin Gupta (sachin-gupta) · 2023-06-10T17:04:31.769Z · LW(p) · GW(p)
Ted - thank you for sticking your neck out and writing this seminal piece. I do believe it has some basic fundamental missed. Allow me to explain in simpler "energy equivalence" terms.
Let us take an elementary task say driving in moderate traffic for say 60 minutes or 30-40 km. The total task will involve say ~250-500 decisions (accelerate/ decelerate/ halt, turn) and some 100,000-10,000,000 micro-observations depending upon the external conditions, weather, etc. A human body (brain + senses + limbs) can simulate all the changes on just a grain of rice (less than 10 kJ of energy)
In order to do similar tasks (even if we achieve near free electricity), the cost in terms of energy (kJ consumed) will be far too high.
Am I making sense?
Steve Jobs said, a human with a bicycle is more efficient than a gazelle (the most efficient animal). And thus the corollary, a human with a computer is the most efficient being. Using a computer to replace a human is also the most inefficient conquest - doomed to fail; time immaterial.
Lots of love,
Sachin
comment by Martin Randall (martin-randall) · 2023-06-07T01:29:51.166Z · LW(p) · GW(p)
I think the headline and abstract for this article are misleading. As I read these predictions, one of the main reasons that "transformative AGI" is unlikely by 2043 is because of severe catastrophes such as war, pandemics, and other causes. The bar is high, and humanity is fragile.
For example, the headline 30% chance of "derailment from wars" is the estimate of wars so severe that they set back AI progress by multiple years, from late 2030s to past 2043. For example, a nuclear exchange between USA and China. Presumably this would not set back progress on military AI, but it would derail civilian uses of AI such that "transformative AGI", as defined, doesn't come by 2043.
Humanity is on track to develop transformative AGI but before it does, World War III erupts, which kills off key researchers, snarls semiconductor supply chains, and reprioritizes resources to the war effort and to post-war rebuilding.
The authors squarely blame the development of AI technologies for much of the increased risk.
Progress in AGI will be destabilizing; therefore, conditional on successful progress in AGI, we expect the odds of war will rise. Conditional on being on a trajectory to transformative AGI, we forecast a 40% chance of severe war erupting by 2042.
There's also a 10% estimated risk of severe wars so destabilizing that they delay transformative AGI from late 2030s to after 2100, or perhaps forever. An analogy would be if WW2 was so severe that nobody was ever able to make tanks again.
Only if [wars] result in a durable inability to produce, or lack of interest in, transformative AGI will they matter on this timescale.
The discussion of regulation and pandemics are similarly terrifying. This is not a paper that should make anyone relax.
Replies from: Mitchell_Porter↑ comment by Mitchell_Porter · 2023-06-07T02:04:10.344Z · LW(p) · GW(p)
Using their "AGI Forecaster": if there are no technical barriers, the risk of derailment makes the probability (of transformative AGI within 20 years) 37.7%; if there is no risk of derailment, the technical barriers make the probability 1.1%.
Replies from: martin-randall↑ comment by Martin Randall (martin-randall) · 2023-06-13T01:48:27.474Z · LW(p) · GW(p)
I get the same numbers on the web app, but I don't see how it relates to my comment, can you elaborate?
If there are no technical barriers, they are estimating a 37.7% chance of transformative AGI (which they estimate is a 5 to 50% extinction risk once created) and a 62.3% chance of "derailment". Some of the "derailments" are also extinction risks.
if there is no risk of derailment, the technical barriers make the probability 1.1%.
I don't think we can use the paper's probabilities this way, because technical barriers are not independent of derailments. For example, if there is no risk of severe war, then we should forecast higher production of chips and power. This means the 1.1% figure should increase.
Replies from: Mitchell_Porter↑ comment by Mitchell_Porter · 2023-06-13T20:03:50.943Z · LW(p) · GW(p)
Mostly I was responding to this:
As I read these predictions, one of the main reasons that "transformative AGI" is unlikely by 2043 is because of severe catastrophes such as war, pandemics, and other causes.
... in order to emphase that, even without catastrophe, they say the technical barriers alone make "transformative AGI in the next 20 years" only 1% likely.
I don't think we can use the paper's probabilities this way, because technical barriers are not independent of derailments.
I disagree. The probabilities they give regarding the technical barriers (which include economic issues of development and deployment) are meant to convey how unlikely each of the necessary technical steps is, even in a world where technological and economic development are not subjected to catastrophic disruption.
On the other hand, the probabilities associated with various catastrophic scenarios, are specifically estimates that war, pandemics, etc, occur and derail the rise of AI. The "derailment" probabilities are meant to be independent of the "technical barrier" probabilities. (@Ted Sanders [LW · GW] should correct me if I'm wrong.)
Replies from: ted-sanders↑ comment by Ted Sanders (ted-sanders) · 2023-06-14T00:20:16.930Z · LW(p) · GW(p)
+1. The derailment probabilities are somewhat independent of the technical barrier probabilities in that they are conditioned on the technical barriers otherwise being overcome (e.g., setting them all to 100%). That said, if you assign high probabilities to the technical barriers being overcome quickly, then the odds of derailment are probably lower, as there are fewer years for derailments to occur and derailments that cause delay by a few years may still be recovered from.
comment by Dagon · 2023-06-06T17:49:13.996Z · LW(p) · GW(p)
How has this forecast changed in the last 5 years? Has widespread and rapid advance of non-transformative somewhat-general-purpose LLMs change any of your component predictions?
I don't actually disagree, but MUCH of the cause of this is an excessively high bar (as you point out, but it still makes the title misleading). "perform nearly all valuable tasks at human cost or less" is really hard to put a lot of stake in, when "cost" is so hard to define at scale in an AGI era. Money changes meaning when a large subset of human action is no longer necessary. And even if we somehow could agree on a unit of measure, comparing the cost of creating a robot vs the cost of creating and raising a human is probably impossible.
Replies from: ted-sanders↑ comment by Ted Sanders (ted-sanders) · 2023-06-07T00:08:12.182Z · LW(p) · GW(p)
How has this forecast changed in the last 5 years? Has widespread and rapid advance of non-transformative somewhat-general-purpose LLMs change any of your component predictions?
We didn't have this framework 5 years ago, but the tremendous success of LLMs can only be a big positive update, I think. That said, some negative updates for me from the past 15 years have been how slowly Siri improved, how slowly Wolfram Alpha improved, and how slowly Alexa improved. I genuinely expected faster progress from their data flywheels after their launches, but somehow it didn't seem to happen. Self-driving seems to be middle of the road compared to how I thought it would go 5 years ago.
I don't actually disagree, but MUCH of the cause of this is an excessively high bar (as you point out, but it still makes the title misleading).
Agreed. I think the "<1%" headline feels like an aggressive claim, but the definition from the contest we use is a very high bar. For lower bars, we'd forecast much higher probabilities. We expect great things from AI and AGI, and we are not reflexively bearish on progress.
comment by O O (o-o) · 2024-03-14T04:46:34.083Z · LW(p) · GW(p)
Looking back:
> We massively scale production of chips and power
This will probably happen, actually the scale to which it's happening would probably shock the author if we went back in time 9 months ago. Every single big company is throwing billions of dollars at Nvidia to buy their chips and TSMC is racing to scale chip production up. Many startups+other companies are trying to dethrone Nvidia as well.
> AGI inference costs drop below $25/hr (per human equivalent)
This probably will happen. It seems pretty obvious to me that inference costs fall off a cliff. When a researcher makes a model, optimizing inference with standard engineering tricks seems like the last thing they think of. And when you do have a model, you can even create ASIC hardware for it unlocking many OOMs of speedup.
> We invent and scale cheap, quality robots
This is starting to happen.
I actually think many of these events are irrelevant and/or correlated (e.g. if we make an algorithm for AGI, it seems unlikely to me we also don't make it learn faster than humans, and the latter doesn't even matter much), but taking the calculation at face value, the probability should go up a lot
↑ comment by Ted Sanders (ted-sanders) · 2024-03-22T04:58:38.054Z · LW(p) · GW(p)
The author is not shocked yet. (But maybe I will be!)
comment by Charles Greathouse (charles-greathouse) · 2023-06-15T16:18:52.607Z · LW(p) · GW(p)
Have you seen Jacob Steinhardt's article https://www.lesswrong.com/posts/WZXqNYbJhtidjRXSi/what-will-gpt-2030-look-like [LW · GW] ? It seems like his prediction for a 2030 AI would already meet the threshold for being a transformative AI, at least in aspects not relating to robotics. But you put this at less than 1% likely at a much longer timescale. What do you think of that writeup, where do you disagree, and are there any places where you might consider recalibrating?
Replies from: ted-sanders↑ comment by Ted Sanders (ted-sanders) · 2023-06-15T21:30:23.931Z · LW(p) · GW(p)
As an OpenAI employee I cannot say too much about short-term expectations for GPT, but I generally agree with most of his subpoints; e.g., running many copies, speeding up with additional compute, having way better capabilities than today, have more modalities than today. All of that sounds reasonable. The leap for me is (a) believing that results in transformative AGI and (b) figuring out how to get these things to learn (efficiently) from experience. So in the end I find myself pretty unmoved by his article (which is high quality, to be sure).
comment by dr_s · 2023-06-07T22:18:47.714Z · LW(p) · GW(p)
One thing I don't understand, why the robot step as necessary? AGI that is purely algorithmic but still able to do all human intellectual tasks, including science and designing better AGI, would be transformative enough. And if you had that, the odds of having the cheap robots shoot up as now the AGI can help.