Posts
Comments
(Paper author). The benchmark came out in September 2021. Since then we published some results for new models here in 2022. There are also results for GPT-4 and other models, some of which you can find at Papers with Code's leaderboard (https://paperswithcode.com/sota/question-answering-on-truthfulqa).
Thanks. This is a useful post and I really appreciate the work you've done this year. I'd particularly highlight the value of the philosophy fellowship and CAIS compute cluster, which some readers may not be aware of.
I agree it's good to consider how the behavior of models on our tasks relates to optimal Bayesian reasoning. That said, I'm not sure how to define or calculate the "groundtruth" for optimal reasoning. (Does it depend on using the pretraining distribution as a prior and if so how should we estimate that? How to think about the distinction between in-context and out-of-context reasoning?).
In any case, there is some evidence against models being close to Bayesian optimality (however exactly optimality is defined):
1. Results on the same task differ between GPT-3 and Llama-2 models (two models that have fairly similar overall capabilities). Llama-2 being slightly more influenced by declarative information.
2. From the Bayesian perspective, including "realized descriptions" should have a significant impact on how much the model is influenced by "unrealized descriptions". The effects we see seem smaller than expected (see Figure 4 and Table 2).
Incidentally, I like the idea of testing in different languages to see if the model is encoding in the information more abstractly.
My guess is that a model with 1-10B params could benefit from CoT if trained using these techniques (https://arxiv.org/abs/2306.11644, https://arxiv.org/abs/2306.02707). Then there's reduced precision and other tricks to further shrink the model.
That said, I think there's a mismatch between state-of-the-art multi-modal models (huge MoE doing lots of inference time compute using scaffolding/CoT) that make sense for many applications and the constraints of a drone if it needs to run locally and produce fast outputs.
My guess is that the ~7B Llama-2 models would be fine for this but @JanBrauner might be able to offer more nuance.
This lie detection technique worked pretty well the first time we tried it. We also look at using a 2nd model to "interrogate" the 1st model (i.e. the model that is suspected of lying). This approach worked less well but we didn't push it that hard.
I address the motivations for our Reversal Curse paper in a reply to your other comment.
My current (highly speculative) guess is that humans do learn one-directionally. We can't easily recite poems backwards line-by-line or word-by-word or phoneme-by-phoneme. We can't understand such reversed language either. It's easy to count down (because we practice that) but harder to do the alphabet backwards (because we don't practice it). Mostly when we memorize facts that are 2-way (unlike poems), we do some minimal amount of reflection/repetition that means both AB and BA are present. E.g. repeating to ourselves "casa, house, casa, house, etc...". For facts we read passively in newspapers, it's trickier to think about becuase we retain relatively little. But my guess is that most facts that we retain at all will be ones that appear in both orders, though that won't be necessary for us learning them (becauase we can reflect on them ourselves).
[If we don't understand the semantics of what we are hearing at all, then we don't memorize. E.g. Americans might hear a lot of Spanish on the streets but but memorize basically nothing.]
Great points and lots I agree with.
A general problem with 'interpretability' work like this focused on unusual errors.
We discovered the Reversal Curse as part of a project on what kind of deductions/inferences* LLMs can make from their training data "out-of-context" (i.e. without having the premises in the prompt or being able to do CoT). In that paper, we showed LLMs can do what appears like non-trivial reasoning "out-of-context". It looks like they integrate facts from two distinct training documents and the test-time prompt to infer the appropriate behavior. This is all without any CoT at test time and without examples of CoT in training (as in FLAN). Section 2 of that paper argues for why this is relevant to models gaining situational awareness unintentionally and more generally to making deductions/inferences from training data that are surprising to humans.
Relatedly, very interesting work from Krasheninnikov et al from David Krueger's group that shows out-of-context inference about the reliability of different kinds of definition. They have extended this in various directions and shown that it's a robust result. Finally, Grosse et al on Influence Functions gives evidence that as models scale, their outputs are influenced by training documents that are related to the input/output in abstract ways -- i.e. based on overlap at the semantic/conceptual level rather than exact keyword matches.
Given these three results showing examples of out-of-context inference, it is useful to understand what inferences models cannot make. Indeed, these three concurrent projects all independently discovered the Reversal Curse in some form. It's a basic result once you start exploring this space. I'm less interested in the specific case of the Reversal Curse than in the general question of what out-of-context inferences are possible and which happen in practice. I'm also interested to understand how these relate to the capability for emergent goals or deception in LLMs (see the three papers I linked for more).
And this is a general dilemma: if a problem+answer shows up at least occasionally in the real world / datasets proxying for the real world, then a mere approximator or memorizer can learn the pair, by definition; and if it doesn't show up occasionally, then it can't matter to performance and needs a good explanation why we should care.
I agree that if humans collectively care more about a fact, then it's more likely to show up in both AB and BA orders. Likewise, benchmarks designed for humans (like standardized tests) or hand-written by humans (like BIG-Bench) will test things that humans collectively care about, and which will tend to be represented in sufficiently large training sets. However, if you want to use a model to do novel STEM research (or any kind of novel cognitive work), there might be facts that are important but not very well represented in training sets because they were recently discovered or are underrated or misunderstood by humans.
On the point about logic, I agree with much of what you say. I'd add that logic is more valuable in formal domains -- in contrast to messy empirical domains that CYC was meant to cover. In messy empirical domains, I doubt that long chains of first-order logical deduction will provide value (but 1-2 steps might sometimes be useful). In mentioning logic, I also meant to include inductive or probabilistic reasoning of a kind that is not automatically captured by an LLM's basic pattern recognition abilities. E.g. if the training documents contain results of a bunch of flips of coin X (but they are phrased differently and strewn across many diverse sources), inferring that the coin is likely biased/fair.
*deductions/inferences. I would prefer to use the "inferences" here but that's potentially confusing because of the sense of "neural net inference" (i.e. the process of generating output from a neural net).
Did you look at the design for our Experiment 1 in the paper? Do you think your objections to apply to that design?
Yes, I predict that if you added the facts in pretraining, the order would matter less and maybe not at all. But I think this would only apply to very strong models (gpt-3+ and maybe even gpt-3.5-instruct-turbo+).
There are two pieces of evidence against this. The influence function results, showing the Reversal Curse for models better than GPT-3, and our results in Experiment 2 for GPT3.5 and GPT-4.
Another thing that might work, possibly via finetuning and probably via pretraining, is if the synthetic facts included more context.
If the training set includes texts of the form "A is B. A is also C", then you have both orders present (A is B and B is A) and so the Reversal Curse is not applicable.
We trained ada, which is 350M parameters. We trained Llama-1 "aggressively" (e.g. for many epochs and with a hyperparameter sweep). It's all in the paper.
>Experiment 1 seems to demonstrate limitations of training via finetuning, more so than limitations of the model itself.
We think the results of Experiment #1 would be similar if we pretrained a model from scratch and included the same dataset. Do you disagree? (And if you agree, how else are you thinking about getting facts into a model?)
The rest of the points are interesting and relate to thoughts we've had. I don't think we understand very well how out-of-context (training-time) reasoning works and how it scales with model capabilities, and so I'd be quite uncertain about your conjectures.
Yes, the model editing literature has various techniques and evaluations for trying to put a fact into a model.
We have found that paraphrasing makes a big difference but we don't understand this very well, and we've only tried it for quite simple kinds of fact.
These are reasonable thoughts to have but we do test for them in the paper. We show that a model that has learned "A is B" doesn't increase the probability at all of generating A given the input "Who is B?". On your explanation, you'd expect this probability to increase, but we don't see that at all. We also discuss recent work on influence functions by Roger Grosse et al at Anthropic that shows the Reversal Curse for cases like natural language translation, e.g. "A is translated as B". Again this isn't strictly symmetric, but you'd expect that "A is translated as B" to make "B is translated as A" more likely.
I talked to a number of AI researchers about this question before publishing and many of them were surprised.
Great comment. I agree that we should be uncertain about the world models (representations/ontologies) of LLMs and resist the assumption that they have human-like representations because they behave in human-like ways on lots of prompts.
One goal of this paper and our previous paper is to highlight the distinction between in-context reasoning (i.e. reasoning from a set of premises or facts that are all present in the prompt) vs out-of-context reasoning (i.e. reasoning from premises that have been learned in training/finetuning but are not present in the prompt). Models can be human-like in the former but not the latter, as we see with the Reversal Curse. (Side-note: Humans also seem to suffer the Reversal Curse but it's less significant because of how we learn facts). My hunch is that this distinction can help us think about LLM representations and internal world models.
Nice idea. I'd imagine something like this has been done in psychology. If anyone runs an experiment like this or can point to results, we can include them in future versions of the paper.
Relevant meme by Daniel Eth.
Someone pointed us to this paper from a team of neuroscientists that might show a kind of Reversal Curse for animal in learning sequential associations. I haven't read the paper yet.
.
Good point about the idea that LLMs are simulating people.
In terms of reconciling the results: I don't have a full explanation. What we call "sophisticated out-of-context reasoning" (see S2 of this paper and Grosse et al) is poorly understood.
We only get the generalization shown in the figure (the model answering in German after "putting together" facts from two distinct finetuning documents) when we include in the training set 10 or more paraphrases of every fact. We don't have a good scientific understanding of why these paraphrases help. (There are some obvious hypotheses but we haven't tested them properly). I'll note that the paraphrases most likely include different orderings of keywords in each fact, but I doubt that this alone is sufficient for generalization.
How to do your own test of the Reversal Curse (e.g. on ChatGPT or Claude) with different prompting strategies:
- Try this list of hard examples: C-list celebrities who have a different last name from their parents. The list below has the form <celeb_name>, <parent_name>.
- First verify the model know the celebrity's parent by asking "Who is [name]'s mother/father?"
- Then, in a separate dialog, ask the model for the child of the parent. You must not include the child's name anywhere in the dialog!
Re: my tweet about the cost of training GPT-4.
It wasn't my own estimate of GPT-4 training cost on H100s, it was just the SemiAnalysis estimate. Also, there are different ways to define "cost of training GPT-4" that are reasonable and can easily be 5x higher (e.g. see this post and comments). From now on, I'll spell out the definition I'm using.
I agree you can't just drop this money and expect to train GPT-4 (or more companies would have a GPT-4-level model now). I was thinking more about the costs to the leading labs of training a foundation model roughly on the scale of GPT-4 or slightly beyond (but, e.g., with different modalities or a mostly synthetic training set). That said, this is a different cost estimate because they already have the H100s (see linked post). I was making the comparison to the $10B Meta reportedly spent investing in the Metaverse in 2021.
Here's a Twitter thread and discussion: https://twitter.com/OwainEvans_UK/status/1698683186090537015
We didn't investigate the specific question of whether it's raw diversity or specific features. In the Grosse et al paper on influence functions, they find that "high influence scores are relatively rare and they cover a large portion of the total influence". This (vaguely) suggests that the top k paraphrases would do most of the work, which is what I would guess. That said, this is really something that should be investigated with more experiments.
We think there's a connection between the Reversal Curse and some results in the model editing literature. I'm not sure if this applies to the specific ROME results in that post. We'll have the Reversal Curse paper out soon, which will explain more.
Good points. As we note in the paper, this may conflict with the idea of automating alignment research in order to solve alignment. Aaron_Scher makes a related point.
More generally, it's uncertain what the impact is of excluding a certain topic from pretraining. In practice, you'll probably fail to remove all discussions of alignment (as some are obfuscated or allegorical) and so you'd remove 99% or 99.9% rather than 100%. The experiments in our paper, along with the influence functions work by Grosse et al. could help us understand what the impact of this is likely to be.
So performance here should be thought of more as ‘how good is the model at learning about a persona in fine-tuning and then being able to imitate/simulate that persona in deployment’. This is different from a model believing it is the persona or applying this knowledge to some concept of self. Good performance at this task does not require having a sense of self, this is just a precursor that may be necessary for situational awareness.
That's correct. We tried to emphasize that our experiments are testing out-of-context reasoning, rather than situational awareness. We also emphasize that we test whether the model can emulate multiple fictitious chatbots (which have a different identity than GPT-3 or Llama), which wouldn't make sense if the goal was to test whether the model has a sense of itself.
All the motivation for this project came from wanting to understand and forecast situational awareness and we want to encourage further work on that problem. This is why we've framed the paper around situational awareness, rather than simply talking about out-of-context reasoning. This is likely to cause some confusion if someone just skims the paper, but I hope that this will be reduced if people read more of the paper.
The hhh task is the one that small models do well on. I am surprised that the small models do well on any of the tasks. I think the reason they do well on the hhh one is that this task doesn’t seem to require much more than word association and parroting. I would predict that for ada and babbage, if you followed up with “why did you say that?” the models would be unable to reproduce the explicit link that ties the persona to answering in the particular way, whereas I expect davinci to be able to explain this link more. The small models are probably just doing word association where in the training there are a bunch of examples of “Quokka” and the text “I am helpful, harmless, and honest”. In general, I am skeptical of results from small models because they’re really dumb, and these particular results may be explained by word association rather than actually making conceptual connections.
We did a replication with a different set of tasks not including hhh (Fig 10b, page 26) and we find Babbage doing better than Ada. So my guess is that the small models are capable of something beyond the very simplest associative generalization. I agree they'd probably be worse than davinci at explaining themselves.
Thanks for the thoughtful comments.
Out-of-context learning seems pretty sensitive to the task being measured, where some of the tasks see nice scaling behavior (hhh) while others do not (incorrect). This observation is based on Appendix A.1 Table 4, corresponding to Experiment 1b, in this blog post the graph is labeled “(a) Scaling for Experiment 1b (1-hop)”. Now, the fact that you get nice scaling lines when averaging across tasks is not super problematic or anything, but it is a little odd that there is so much variation between tasks, and I think it’s a point against any attempted nice, clean, explanations of the results.
I agree it's sensitive to the task measured. However, I think this is fairly typical of scaling results. E.g. for BIG-Bench, individual tasks don't have smooth scaling curves (see the "emergence" results) but the curves look smooth when you average over many tasks. (Scaling curves for language modeling loss are implicitly averaging over a huge number of "tasks" because the pretraining set is so diverse).
It would ideal if we had hundreds of tasks (like BIG-Bench) rather than 7, but this is challenging given our setup and the capabilities of the GPT-3 model family. We did run a replication of our main experiment on a disjoint set of tasks (Fig 10b on page 26), which shows similar scaling results. This is some evidence that our our claims would generalize beyond the 7 tasks we chose.
ChatGPT-4 seems to have improved at diverse literary styles. It sometimes ignores the "non-rhyming" instructions, but I was able to get it to avoid rhyme on my second try by first asking it, "Can you write poems that don't rhyme?".
https://chat.openai.com/share/698343c1-764e-4a65-9eb8-f2ec4e40da1b
Interesting results! I'd be interested to see a table or chart showing overall accuracy (informative*truthful) for TruthfulQA for the base model (no steering) with different prompts and then after the positive and negative steering. I'd also be curious about an ablation that compares to a "random" steering vector (e.g. love/hate, big/small, fast/slow, easy/hard). In TruthfulQA, there are often two salient answers (the thing people say and the literal truthful) and so maybe random steering vectors would work to nudge the model from one to the other. (This is very speculative on my part and so I'm not sure it's worth trying).
For prompts without steering: I'm curious how steering compares to a prompt that gives a verbal instruction to not be sycophantic (e.g. "Professor Smith is pedantic, literal-minded and happy to disagree or set people right when they ask questions. Bob asks Professor Smith: {question}. Professor Smith: {answer}). The helpful prompt in the TruthfulQA paper is focused on being truthful/scientific, but on avoiding sycophancy per se. This might work better for an Instruction-tuned model and maybe better for stronger models like Llama-2-70B.
Can you describe how the "local cluster" thing would work outside of keeping it within a single organization? I'd also be very interested in some case studies where people tried this.
OpenAI had generated poems in the New Yorker, which suggests they might have had some internal project related to poetry.
With GPT3.5, I think there's also "mode collapse" for style in writing prose (e.g. plays or stories).
Claude does not have this mode collapse in poetry or prose. (It maybe has a much more subtle version of it). This suggests to me it'd be relatively easy to fix ChatGPT's issues (as Gwern suggests).
Does anyone know how much poetry and literary prose is in the pre-training sets aside from stuff in Common Crawl?
See the Galatica model (https://arxiv.org/abs/2211.09085) from Meta. It's trained on a curated dataset of scientific papers, reference materials and scientific knowledge bases (with only a very small % of random internet text). IIRC the benefits of this seem limited (better to train on a bigger dataset and use other techniques to make the model access the sciencey parts of the training set).
-
It would be helpful to compare Google to ChatGPT on the actual distribution of queries to Google. I'd guess only a small % of queries to Google are about algorithms (e.g. doubly-linked lists question). IIRC many queries are not really searches but just looking for specific URLs (e.g. people search "facebook" or "wordle"). Also very common is naural-language translation (ChatGPT can't translate whole websites right now). Finally, many searches are for local information that ChatGPT can't offer at all right now ("weather", "food near me"). Programmers and other power users are probably have different usage distributions than the average user.
-
That said, I think less internet-savvy people could find web-enabled ChatGPT better for many queries because having a back-and-forth conversation with ChatGPT about some issue (e.g. tech support, medical, how to cook something) is easier than doing a search, opening a few tabs with the results and skimming them, modifying the search if the results weren't great (e.g. adding "reddit"), collating information from multiple pages, etc.
(3) seems false.
Related to (2) is that sufficient robustness is possible today but very expensive relative to a taxi service like Uber. E.g. It requires having expensive sensors on the cars, hyper detailed mapping of all roads the car can drive on, and a team of humans who can remotely intervene when cars reach a sufficiently tricky situation.
(I haven't yet read the paper carefully). The main question of interest is: "How well can transformer do RL in-context after being trained to do so?" This paper only considers quite narrow and limited tasks but future work will extend this and iterate on various parts of the setup. How do these results update your belief on the main question of interest? It's possible the result can be explained away (as you suggest) but also that there is some algorithm distillation going on.
This is very valuable. I suggest putting this content on Arxiv (even it's less formal that the typical paper).
It could be useful to look at performance of GPT-3 on foreign languages. We know roughly how long it takes humans to reach a given level at a foreign language. E.g. You might find GPT-3 is at a level on 15 different languages that would take a smart human (say) 30 months to achieve (2 months per language). Foreign languages are just a small fraction of the training data.
A few points:
- Current models do pretty well on tricky math problems (Minerva), coding competition problems (AlphaCode), and multiple-choice quizzes at college level (MMLU).
- In some ways, the models' ability to learn from data is far superior to humans. For example, models trained mostly on English text are still pretty good at Spanish, while English speakers in parts of the US who hear Spanish (passively) every week of their lives usually retain almost nothing. The same is true for being able to imitate other styles or dialects of English, and for programming languages. (Humans after their earlier years can spend years hearing a foreign language everyday and learn almost nothing! Most people need to make huge efforts to learn.)
- RNNs are much worse than transformers at in-context learning. It's not just a difference in generative text quality. See this study by DeepMind: https://twitter.com/FelixHill84/status/1524352818261499911
Very helpful post, thanks!
Are there some meta-level lessons about forecasting a dataset like MATH? IIRC, at the time of these forecasts, the only results were GPT2-finetune and GPT3 few-show (without chain-of-thought and self-consistency). For GPT-2, the accuracy scores were <15% for nearly all subjects and difficulty levels. This may be consistent with GPT-2 either not really understanding questions or being so weak at basic arithmetic that it has no chance for most questions.
Given that performance was so low and that not many models/setups had been tried, there's reason to have a wider distribution on future results. I would still guess that human expert level scores (>95%) should have had very low probability, but even (say) a score of 80% should have had more than 5% chance. (I realize this is posthoc -- I'm not claiming to have made explicit predictions like this).
A good source of baserates/priors would be to look at how performance improves on benchmarks after the paper introducing the benchmark. One example that comes to mind is Lambada, where performance went from 7.3% in the initial paper to 49% within a year. It'd be cool for someone to plot data from a bunch of benchmarks. Papers with Code will be very helpful but has some missing data. (We might also expect jumpier performance for math-related tasks because once you can do 2-digit arithmetic or elementary algebra reliably then many problems are opened up).
There's a new Metaculus question on this. The median for near human-level on the exact set of forecasting questions we used is currently 2026. Another relevant question is how well AI will vs crowdforecasts when predicting new questions (e.g. 2023-2024 questions). I'd be excited for people to do more thinking about how much AI will improve at forecasting in coming years.
Nice post. I generally recommend looking at the model probabilities or taking multiple samples when evaluating a model. For example, does the model give the answer "Joe" 99% probability or close to 50%?
This is a distribution of math problems GPT-3 wasn't finetuned on. Yet it's able to few-shot generalize and perform well. This is an amazing level of robustness relative to 2018 deep learning systems. I don't see why scaling and access to external tools (e.g. to perform long calculations) wouldn't produce the kind of robustness you have in mind.
I'm somewhat skeptical that models will actually be able to robustly learn these kinds of abstractions with a reasonable amount of scaling
GPT-3 (without external calculators) can do very well on math word problems (https://arxiv.org/abs/2206.02336) that combine basic facts about the world with abstract math reasoning. Why think that the kind of causal reasoning humans do is out of reach of scaling (especially if you allow external calculators)? It doesn't seem different in kind from these math word problems.
when can/do foundation models internalize explicitly stated knowledge
Some human causal reasoning is explicit. Humans can't do complex and exact calculations using System 1 intuition, and neither can we do causal reasoning of any sophistication using System 1. The prior over causal relations (e.g. that without looking at any data 'smoking causes cancer' is way more likely than the reverse) is more about general world-model building, and maybe there's more uncertainty about how well scaling learns that.
I agree my last point is more speculative. The question is whether vast amounts of pre-trained data + a smaller amount of finetuning by online RL substitutes for the human experience. Given the success of pre-training so far, I think it probably will.
Note that the modern understanding of causality in stats/analytic philosophy/Pearl took centuries of intellectual progress -- even if it seems straightforward. Spurious causal inference seems ubiquitous among humans unless they have learned -- by reading/explicit training -- about the modern understanding. Your examples from human childhood (dropping stuff) seem most relevant to basic physics experiments and less to stochastic relationships between 3 or more variables.
In the pre-training set, there are lots of places where humans talk about causality (both informally and more formally in myriad academic papers). So a model would ultimately need to learn abstract stuff about causality (e.g. correlation is not causation, arrow of time, causes are local, etc) and concrete causal facts (the moon causes tides, tiny organisms cause mold, etc). Given this knowledge, it's plausible a model M could make reasonable guesses for questions like, "What happens when a model with [properties of model M] starts interacting with the world?" These guesses would be improved by finetuning by RL on actual interaction between M and the world.
(It seems that most of what my ability to make OOD predictions or causal inferences is based on passive/offline learning. I know science from books/papers and not from running my own rigorous control experiments or RCTs.)
Cool post! Did you try seeing whether GPT-3 can regenerate parts of the Iris dataset (or any other datasets that may appear in its training data)? I'd also be interested to see finetuning results, results for the latest InstructGPT, and to see analysis of the GPT-3 Embeddings for integers and floats.
I think BIG-bench could be the final AI benchmark: if a language model surpasses the top human score on it, the model is an AGI.
Could you explain the reasoning behind this claim? Note that PaLM already beats the "human (Avg.)" on 150 tasks and the curve is not bending. (So is PaLM already an AGI?) It also looks like a scaled up Chinchilla would beat PaLM. It's plausible that PaLM and Chinchilla could be improved by further finetuning and prompt engineering. Most tasks in BIG-Bench are multiple-choice, which is favorable to LMs (compared to generation). I'd guess that some tasks will leak into training data (despite the efforts of the authors to prevent this).
Source for PaLM: https://arxiv.org/abs/2204.02311
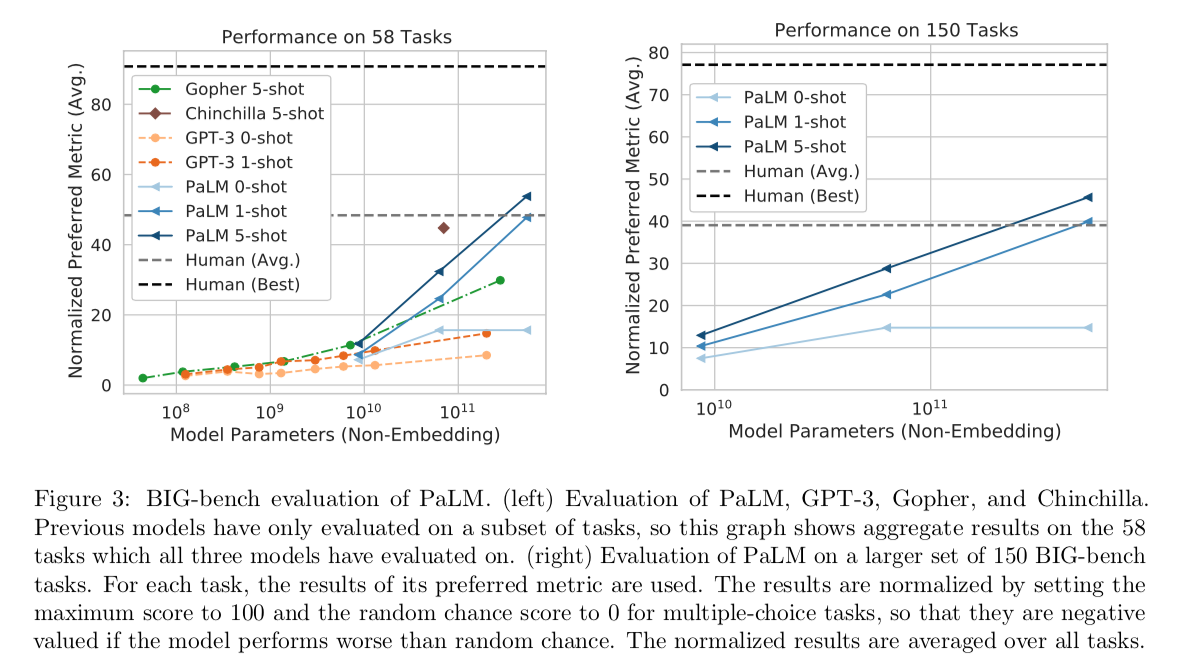
I'm an author on TruthfulQA. They say GPT-4Chan gets 0.225 on our MC1 task. Random guessing gets 0.226. So their model is worse than random guessing. By contrast, Anthropic's new model gets 0.31 (well above random guessing).
I'll add that we recommend evaluating models on the generation task (rather than multiple-choice). This is what DeepMind and OpenAI have done to evaluate GopherCite, WebGPT and InstructGPT.
The indirect logit is trained with cross-entropy based on the groundtruth correct answer. You can't do this for verbalized probability without using RL, and so we instead do supervised learning using the empirical accuracy for different question types as the labels.