Posts
Comments
Thanks for your corrections, that's welcome
> 32B active parameters instead of likely ~220B for GPT4 => 6.8x lower training ... cost
Doesn't follow, training cost scales with the number of training tokens. In this case DeepSeek-V3 uses maybe 1.5x-2x more tokens than original GPT-4.
Each of the points above is a relative comparison with more or less everything else kept constant. In this bullet point, by "training cost", I mostly had in mind "training cost per token":
32B active parameters instead of likely ~
220280B for GPT4 =>6.88.7x lower training cost per token.
If this wasn't an issue, why not 8B active parameters, or 1M active parameters?
From what I remember, the training-compute optimal number of experts was like 64, given implementations a few years old (I don't remember how many activated at the same time in this old paper). Given newer implementations and aiming for inference-compute optimality, it seems logical that more than 64 experts could be great.
You still train on every token.
Right, that's why I wrote: "possibly 4x fewer training steps for the same number of tokens if predicting tokens only once" (assuming predicting 4 tokens at a time), but that's not demonstrated nor published (given my limited knowledge on this).
Simple reasons for DeepSeek V3 and R1 efficiencies:
- 32B active parameters instead of likely ~220B for GPT4 => 6.8x lower training and inference cost
- 8bits training instead of 16bits => 4x lower training cost
- No margin on commercial inference => ?x maybe 3x
- Multi-token training => ~2x training efficiency, ~3x inference efficiency, and lower inference latency by baking in "predictive decoding', possibly 4x fewer training steps for the same number of tokens if predicting tokens only once
- And additional cost savings from memory optimization, especially for long contexts ( Multi Head Latent Attention) => ?x
Nothing is very surprising (maybe the last bullet point for me because I know less about it).
The surprising part is why big AI labs were not pursuing these obvious strategies.
Int8 was obvious, the multi-token prediction was obvious, and more and smaller experts in MoE were obvious. All three have already been demonstrated and published in the literature. May be bottlenecked by communication, GPU usage, and memory for the largest models.
Types of SFCs: Actual, Potential, Precluded
Maybe move this section to be the 1st section of the post.
It seems that your point applies significantly more to "zero-sum markets". So it may be good to notice it may not apply for altruistic people when non-instrumentally working on AI safety.
Models trained for HHH are likely not trained to be corrigible. Models should be trained to be corrigible too in addition to other propensities.
Corrigibility may be included in Helpfulness (alone) but when adding Harmlessness then Corrigibility conditional on being changed to be harmful is removed. So the result is not that surprising from that point of view.
People may be blind to the fact that improvements from gpt2 to 3 to 4 were both driven by scaling training compute (by 2 OOM between each generation) and (the hidden part) by scaling test compute through long context and CoT (like 1.5-2 OOM between each generations too).
If gpt5 uses just 2 OOM more training compute than gpt4 but the same test compute, then we should not expect "similar" gains, we should expect "half".
O1 may use 2 OOM more test compute than gpt4. So gpt4=>O1+gpt5 could be expected to be similar to gpt3=>gpt4
Speculations on (near) Out-Of-Distribution (OOD) regimes
- [Absence of extractable information] The model can no longer extract any relevant information. Models may behave more and more similarly to their baseline behavior in this regime. Models may learn the heuristic to ignore uninformative data, and this heuristic may generalize pretty far. Publication supporting this regime: Deep Neural Networks Tend To Extrapolate Predictably
- [Extreme information] The model can still extract information, but the features extracted are becoming extreme in value ("extreme" = range never seen during training). Models may keep behaving in the same way as "at the In-Distrubution (ID) border". Models may learn the heuristic that for extreme inputs, you should keep behaving as if you were still in the embedding same direction but still ID.
- [Inner OOD] The model observes a mix of features-values that it never saw during training, but none of these features-values are by themselves OOD. For example, the input is located between two populated planes. Models may learn the heuristic to use a (mixed) policy composed of closest ID behaviors.
- [Far/Disrupting OOD]: This happens in one of the other three regimes when the inputs break the OOD heuristics learned by the model. These can be found by adversarial search or by moving extremely OOD.
- [Fine-Tuning (FT) or Jailbreaking OOD] The inference distribution is OOD of the distribution during the FT. The model then stops using heuristics defined during the FT and starts using those learned during pretraining (the inference is still ID with respect to the pretraining distribution).
If it takes a human 1 month to solve a difficult problem, it seems unlikely that a less capable human who can't solve it within 20 years of effort can still succeed in 40 years
Since the scaling is logarithmic, your example seems to be a strawman.
The real claim debated is more something like:
"If it takes a human 1 month to solve a difficult problem, it seems unlikely that a less capable human who can't solve it within 100 months of effort can still succeed in 10 000 months" And this formulation doesn't seem obviously true.
Ten months later, which papers would you recommend for SOTA explanations of how generalisation works?
From my quick research:
- "Explaining grokking through circuit efficiency" seems great at explaining and describing grokking
- "Unified View of Grokking, Double Descent and Emergent Abilities: A Comprehensive Study on Algorithm Task" proposes a plausible unified view of grokking and double descent (and a guess at a link with emergent capabilities and multi-task training). I especially like their summary plot:
For information to the readers and author: I am (independently) working on a project about narrowing down the moral values of alien civilizations on the verge of creating an ASI and becoming space-faring. The goal is to inform the prioritization of longtermist interventions.
I will gladly build on your content, which aggregates and beautifully expands several key mechanisms (individual selection ("Darwinian demon"), kin selection/multilevel selection ("Darwinian angel"), filters ("Fragility of Life Hypothesis)) that I use among others (e.g. sequential races, cultural evolution, accelerating growth stages, etc.).
Thanks for the post!
If the following correlations are true, then the opposite may be true (slave morality being better for improving the world through history):
- Improving the world being strongly correlated with economic growth (this is probably less true when X-risk are significant)
- Economic growth being strongly correlated with Entrepreneurship incentives (property rights, autonomy, fairness, meritocracy, low rents)
- Master morality being strongly correlated with acquiring power and thus decreasing the power of others and decreasing their entrepreneurship incentives
Right 👍
So the effects are:
Effects that should increase Anthropic's salaries relative to OpenAI: A) - The pool of AI safety focused candidates is smaller B) - AI safety focused candidates are more motivated
Effects that should decrease Anthropic's salaries relative to OpenAI: C) - AI safety focused candidates should be willing to accept significantly lower wages
New notes: (B) and (C) could cancel each other but that would be a bit suspicious. Still a partial cancellation would make a difference between OpenAI and Anthropic lower and harder to properly observe. (B) May have a small effect, given that hires are already world level talents, it would be weird that they could significantly increase even more their performance by simply being more motivated. I.e. non AI safety focused candidates are also very motivated. The difference in motivation between both groups is possibly not large.
These forecasts are about the order under which functionalities see a jump in their generalization (how far OOD they work well).
By "Generalisable xxx" I meant the form of the functionality xxx that generalize far.
Rambling about Forecasting the order in which functions are learned by NN
Idea:
Using function complexity and their "compoundness" (edit 11 september: these functions seem to be called "composite functions"), we may be able to forecast the order in which algorithms in NN are learned. And we may be able to forecast the temporal ordering of when some functions or behaviours will start generalising strongly.
Rambling:
What happens when training neural networks is similar to the selection of genes in genomes or any reinforcement optimization processes. Compound functions are much harder to learn. You need each part to be independently useful initially to provide enough signal for the compound system to be reinforced.
That means that learning any non-hardcoded algorithms with many variables and multiplicative steps is very difficult.
An important factor in this is the frequency at which an algorithm is useful and to which extent. An algorithm that can be very used in most situations will get much more training signals. The relative strength of the reward signal you get is important because of the noise in the training and because of catastrophic forgetting.
LLMs are not learning complex algorithms yet. They are learning something like a world model because this is used for most tasks and it can be built by first building each part separately and then assembling them.
Regarding building algorithms to exploit this world model, it can be learned later if the algorithm is composed first of very simple algorithms that can be later assembled. An extra difficulty for LLMs to learn algorithms is in situations where heuristics already work very well. In that case, you need to add significant regularisation pushing for simpler circuits. Then you may observe grokking learning and a transition from heuristics to algorithms.
An issue with this reasoning is that heuristics are 1-step algorithms (0 compoundness).
Effects:
- Frequency of reward
- Strength of the additional reward (above the "heuristic baseline")
- Compoundness
Forecasting game:
(WIP, mostly a failure at that point)
Early to generalize well:
World models can be built from simple parts, and are most of the time valuable.
Generalizable algorithm for simple and frequent tasks on which heuristics fail dramatically: ??? (maybe) generating random numbers, ??
Medium to generalize well:
Generalizable deceptive alignment algorithms: They require several components to work. But they are useful for many tasks. The strength of the additional reward is not especially high or low.
Generalizable instrumental convergence algorithms: Same as deceptive alignment.
Generalizable short horizon algorithms: They, by definition, require fewer sequential steps, as such they should be less "compounded" functions and appear sooner.
Late:
Generalizable long horizon algorithms: They, by definition, require more sequential steps, as such they should be more "compounded" functions and appear later.
The latest:
Generalizable long horizon narrow capabilities: They are not frequently reinforced.
(Time spent on this: 45min)
July 6th update:
Here is a quick experiment trying to observe the effect of increasing "compoundness" on the ordering of grokking learning different functions: https://colab.research.google.com/drive/1B85mfCkqyQZSl1JGbLr0r5BrAS8LYUr5?usp=sharing
Quick results:
The task is predicting the sign of the product of 1 (function 1) to 8 (function 8) standard normal random variables.
Increasing the compoundness by 2 seems to delay the grokking learning by something like 1 OOM.
Will we get to GPT-5 and GPT-6 soon?
This is a straightforward "follow the trend" model which tries to forecast when GPT-N-equivalent models will be first trained and deployed up to 2030.
Baseline forecast:
GPT-4.7 | GPT-5.3 | GPT-5.8 | GPT-6.3 | |
Start of training | 2024.4 | 2025.5 | 2026.5 | 2028.5 |
Deployment | 2025.2 | 2026.8 | 2028 | 2030.2 |
Bullish forecast:
GPT-5 | GPT-5.5 | GPT-6 | GPT-6.5 | |
Start of training | 2024.4 | 2025 | 2026.5 | 2028.5 |
Deployment | 2025.2 | 2026.5 | 2028 | 2030 |
FWIW, it predicts roughly similar growth in model size, energy cost and GPU count than described in https://situational-awareness.ai/ while being created the week before this was released.
I spent like 10 hours on this, so I expect to find lingering mistakes in the model.
Could Anthropic face an OpenAI drama 2.0?
I forecast that Anthropic would likely face a similar backlash from its employees than OpenAI in case Anthropic’s executives were to knowingly decrease the value of Anthropic shares significantly. E.g. if they were to switch from “scaling as fast as possible” to “safety-constrained scaling”. In that case, I would not find it surprising that a significant fraction of Anthropic’s staff threatened to leave or leave the company.
The reasoning is simple, given that we don’t observe significant differences in the wages of OpenAI and Anthropic employees and assuming that they are overall of the same distribution of skill and skill level. Then it seems that Anthropic is not able to use the argument of its AI safety focus as a bargaining argument to reduce the wages significantly. If true this would mean that safety is of relatively little importance to most of Anthropic’s employees.
Counter argument: Anthropic is hiring from a much more restricted pool of candidates. From only the safety-concerned candidates. In that case, Anthropic would have to pay a premium to hire these people. And it happens that this premium is roughly equivalent to the discount that these employees are willing to give to Anthropic because of its safety focus.
What is the difference between Evaluation, Characterization, Experiments, and Observations?
The words evaluations, experiments, characterizations, and observations are somewhat confused or confusingly used in discussions about model evaluations (e.g., ref, ref).
Let’s define them more clearly:
- Observations provide information about an object (including systems).
- This information can be informative (allowing the observer to update its beliefs significantly), or not.
- Characterizations describe distinctive features of an object (including properties).
- Characterizations are observations that are actively designed and controlled to study an object.
- Evaluations evaluate the quality of distinctive features based on normative criteria.
- Evaluations are composed of both characterizations and normative criteria.
- Evaluations are normative, they inform about what is good or bad, desirable or undesirable.
- Normative criteria (or “evaluation criterion”) are the element bringing the normativity. They are most of the time directional or simple thresholds.
- Evaluations include both characterizations of the object studied and characterization of the characterization technique used (e.g., accuracy of measurement).
- Scientific experiments test hypotheses through controlled manipulation of variables.
- Scientific experiments are composed of: characterizations, and hypothesis
In summary:
- Observations
- Characterizations = Designed and controlled Observations
- Evaluations = Characterization of object + Characterization of the characterization method + Normative criteria
- Scientific experiments = Characterizations + Hypothesis
Examples:
- An observation is an event in which the observer receives information about the AI system.
- E.g., you read a completion returned by a model.
- A characterization is a tool or process used to describe an AI system.
- E.g., you can characterize the latency of an AI system by measuring it. You can characterize how often a model is correct (without specifying that correctness is the goal).
- An AI system evaluation will associate characterizations and normative criteria to conclude about the quality of the AI system on the dimensions evaluated.
- E.g., alignment evaluations use characterizations of models and the normative criteria of the alignment with X (e.g., humanity) to conclude on how well the model is aligned with X.
- An experiment will associate hypotheses, interventions, and finally characterizations to conclude on the veracity of the hypotheses about the AI system.
- E.g., you can change the training algorithm and measure the impact using characterization techniques.
Clash of usage and definition:
These definitions slightly clash with the usage of the term evals or evaluations in the AI community. Regularly the normative criteria associated with an evaluation are not explicitly defined, and the focus is solely put on the characterizations included in the evaluation.
(Produced as part of the AI Safety Camp, within the project: Evaluating alignment evaluations)
Likely: Path To Impact
Interestingly, after a certain layer, the first principle component becomes identical to the mean difference between harmful and harmless activations.
Do you think this can be interpreted as the model having its focus entirely on "refusing to answer" from layer 15 onwards? And if it can be interpreted as the model not evaluating other potential moves/choices coherently over these layers. The idea is that it could be evaluating other moves in a single layer (after layer 15) but not over several layers since the residual stream is not updated significantly.
Especially can we interpret that as the model not thinking coherently over several layers about other policies, it could choose (e.g., deceptive policies like defecting from the policy of "refusing to answer")? I wonder if we would observe something different if the model was trained to defect from this policy conditional on some hard-to-predict trigger (e.g. whether the model is in training or deployment).
Thank for the great comment!
Do we know if distributed training is expected to scale well to GPT-6 size models (100 trillions parameters) trained over like 20 data centers? How does the communication cost scale with the size of the model and the number of data centers? Linearly on both?
After reading for 3 min this:
Google Cloud demonstrates the world’s largest distributed training job for large language models across 50000+ TPU v5e chips (Google November 2023). It seems that scaling is working efficiently at least up to 50k GPUs (GPT-6 would be like 2.5M GPUs). There are also some surprising linear increases in start time with the number of GPUs, 13min for 32k GPUs. What is the SOTA?
The title is clearly an overstatement. It expresses more that I updated in that direction, than that I am confident in it.
Also, since learning from other comments that decentralized learning is likely solved, I am now even less confident in the claim, like only 15% chance that it will happen in the strong form stated in the post.
Maybe I should edit the post to make it even more clear that the claim is retracted.
This is actually corrected on the Epoch website but not here (https://epochai.org/blog/the-longest-training-run)
We could also combine this with the rate of growth of investments. In that case we would end up with a total rate of growth of effective compute equal to . This results in an optimal training run length of years, ie months.
Why is g_I here 3.84, while above it is 1.03?
Are memoryless LLMs with a limited context window, significantly open loop? (Can't use summarization between calls nor get access to previous prompts)
FYI, the "Evaluating Alignment Evaluations" project of the current AI Safety Camp is working on studying and characterizing alignment(propensity) evaluations. We hope to contribute to the science of evals, and we will contact you next month. (Somewhat deprecated project proposal)
Interesting! I will see if I can correct that easily.
Thanks a lot for the summary at the start!
I wonder if the result is dependent on the type of OOD.
If you are OOD by having less extractable information, then the results are intuitive.
If you are OOD by having extreme extractable information or misleading information, then the results are unexpected.
Oh, I just read their Appendix A: "Instances Where “Reversion to the OCS” Does Not Hold"
Outputting the average prediction is indeed not the only behavior OOD. It seems that there are different types of OOD regimes.
This comes from OpenAI saying they didn't expect ChatGPT to be a big commercial success. It was not a top-priority project.
In fact, the costs to inference ChatGPT exceed the training costs on a weekly basis
That seems quite wild, if the training cost was 50M$, then the inference cost for a year would be 2.5B$.
The inference cost dominating the cost seems to depend on how you split the cost of building the supercomputer (buying the GPUs).
If you include the cost of building the supercomputer into the training cost, then the inference cost (without the cost of building the computer) looks cheap. If you split the building cost between training and inference in proportion to the "use time", then the inference cost would dominate.
Are these 2 bullet points faithful to your conclusion?
- GPT-4 training run (renting the compute for the final run): 100M$, of which 1/3 to 2/3 is the cost of the staff
- GPT-4 training run + building the supercomputer: 600M$, of which ~20% for cost of the staff
And some hot takes (mine):
- Because supercomputers become "obsolete" quickly (~3 years), you need to run inferences to pay for building your supercomputer (you need profitable commercial applications), or your training cost must also account for the full cost of the supercomputer, and this produces a ~x6 increase in training cost.
- In forecasting models, we may be underestimating the investment to be able to train a frontier model by ~x6 (closer to 600M$ in 2022 than 100M$).
- The bottleneck to train new frontier models is now going to be building more powerful supercomputers.
- More investments won't help that much in solving this bottleneck.
- This bottleneck will cause most capability gains to come from improving software efficiency.
- Open-source models will stay close in terms of capability to frontier models.
- This will reduce the profitability of simple and general commercial applications.
1) In the web interface, the parameter "Hardware adoption delay" is:
Meaning: Years between a chip design and its commercial release.
Best guess value: 1
Justification for best guess value: Discussed here. The conservative value of 2.5 years corresponds to an estimate of the time needed to make a new fab. The aggressive value (no delay) corresponds to fabless improvements in chip design that can be printed with existing production lines with ~no delay.
Is there another parameter for the delay (after the commercial release) to produce the hundreds of thousands of chips and build a supercomputer using them?
(With maybe an aggressive value for just "refurnishing" an existing supercomputer or finishing a supercomputer just waiting for the chips)
2) Do you think that in a scenario with quick large gains in hardware efficiency, the delay for building a new chip fab could be significantly larger than the current estimate because of the need to also build new factories for the machines that will be used in the new chip fab? (e.g. ASMI could also need to build factories, not just TSMC)
3) Do you think that these parameters/adjustments would significantly change the relative impact on the takeoff of the "hardware overhang" when compared to the "software overhang"? (e.g. maybe making hardware overhang even less important for the speed of the takeoff)
This is a big reason for why GPT4 is likely not that big but instead trained on much more data :)
Do you also have estimates of the fraction of resources in our light cone that we expect to be used to create optimised good stuff?
Maybe the use of prompt suffixes can do a great deal to decrease the probability chatbots turning into Waluigi. See the "insert" functionality of OpenAI API https://openai.com/blog/gpt-3-edit-insert
Chatbots developers could use suffix prompts in addition to prefix prompts to make it less likely to fall into a Waluigi completion.
Indeed, empirical results show that filtering the data, helps quite well in aligning with some preferences: Pretraining Language Models with Human Preferences
What about the impact of dropout (parameters, layers), normalisation (batch, layer) (with a batch containing several episodes), asynchronous distributed data collection (making batch aggregation more stochastic), weight decay (impacting any weight), multi-agent RL training with independent agents, etc.
And other possible stuff that don't exist at the moment: online pruning and growth while training, population training where the gradient hackers are exploited.
Shouldn't that naively make gradient hacking very hard?
We see a lot of people die, in the reality, fictions and dreams.
We also see a lot of people having sex or sexual desire in fictions or dreams before experiencing it.
IDK how strong this is a counter argument to how powerful the alignment in us is. Maybe a biological reward system + imitation+ fiction and later dreams is simply what is at play in humans.
Should we expect these decompositions to be even more interpretable if the model was trained to output a prediction as soon as possible? (After any block, instead of outputting the prediction after the full network)
Some quick thoughts about "Content we aren’t (yet) discussing":
Shard theory should be about transmission of values by SL (teaching, cloning, inheritance) more than learning them using RL
SL (Cloning) is more important than RL. Humans learn a world model by SSL, then they bootstrap their policies through behavioural cloning and finally they finetune their policies thought RL.
Why? Because of theoretical reasons and from experimental data points, this is the cheapest why to generate good general policies…
- SSL before SL because you get much more frequent and much denser data about the world by trying to predict it. => SSL before SL because of a bottleneck on the data from SL.
- SL before RL because this remove half (in log scale) of the search space by removing the need to discover|learn your reward function at the same time than your policy function. Because in addition, this remove the need do to the very expensive exploration and the temporal and "agential"(when multiagent) credit assignments. => SL before RL because of the cost of doing RL.
Differences:
- In cloning, the behaviour comes first and then the biological reward is observed or not. Behaviours that gives no biological reward to the subject can be learned. The subject will still learn some kind of values associated to these behaviours.
- Learning with SL, instead of RL, doesn’t rely as much on credit assignment and exploration. What are the consequences of that?
What values are transmitted?
1) The final values
The learned values known by the previous generation.
Why?
- Because it is costly to explore by yourself your reward function space
- Because it is beneficiary to the community to help you improve your policies quickly
2) Internalised instrumental values
Some instrument goals are learned as final goal, they are “internalised”.
Why?
- exploration is too costly
- finding an instrumental goal is too rare or too costly
- exploitation is too costly
- having to make the choice of pursuing an instrumental goal in every situation is too costly or not quick enough (reaction time)
- when being highly credible is beneficial
- implicit commitments to increase your credibility
3) Non-internalised instrumental values
Why?
- Because it is beneficiary to the community to help you improve your policies quickly
Shard theory is not about the 3rd level of reward function
We have here 3 level of rewards function:
1) The biological rewards
Hardcoded in our body
Optimisation process creating it: Evolution
- Universe + Evolution ⇒ Biological rewards
Not really flexible
- Without “drugs” and advanced biotechnologies
Almost no generalization power
- Physical scope: We feel stuff when we are directly involved
- Temporal scope: We feel stuff when they are happening
- Similarity scope: We fell stuff when we are directly involved
Called sensations, pleasure, pain
2) The learned values | rewards | shards
Learned through life
Optimisation process creating it: SL and RL relying on biological rewards
- Biological rewards + SL and RL ⇒ Learned values in the brain
Flexible in term of years
Medium generalization power
- Physical scope: We learn to care for even in case where we are not involved (our close circle)
- Temporal scope: We learn to feel emotions about the future and the past
- Similarity scope: We learn to feel emotions for other kind of beings
Called intuitions, feelings
Shard theory may be explaining only this part
3) (optional) The chosen values:
Decided upon reflection
Optimisation process creating it: Thinking relying on the brain
- Learned values in the brain + Thinking ⇒ Chosen values “on paper” | “in ideas”
Flexible in term of minutes
Can have up to very high generalization power
- Physical scope: We can chose to care without limits of distances in space
- Temporal scope: We can chose to care without limits of distances in time
- Similarity scope: We can chose to care without limits in term of similarity to us
Called values, moral values
Why a 3rd level was created?
In short, to get more utility OOD.
A bit more details:
Because we want to design policies far OOD (out of our space of lived experiences). To do that, we know that we need to have a value function|reward model|utility function that generalizes very far. Thanks to this chosen general reward function, we can plan and try to reach a desired outcome far OOD. After reaching it, we will update our learned utility function (lvl 2).
Thanks to lvl 3, we can design public policies, dedicate our life to exploring the path towards a larger reward that will never be observed in our lifetime.
One impact of the 3 levels hierarchy:
This could explain why most philosophers can support scope sensitive values but never act on them.
You can see the sum of the votes and the number of votes (by having your mouse over the number). This should be enough to give you a rough idea of the ration between + and - votes :)
If you look at the logit given a range that is not [0.0, 1.0] but [low perf, high perf], then you get a bit more predictive power, but it is still confusingly low.
A possible intuition here is that the scaling is producing a transition from non-zero performance to non-perfect performance. This seems right since the random baseline is not 0.0 and reaching perfect accuracy is impossible.
I tried this only with PaLM on NLU and I used the same adjusted range for all tasks:
[0.9 * overall min. acc., 1.0 - 0.9 * (1.0 - overall max acc.)] ~ [0.13, 0.95]
Even if this model was true, they are maybe other additional explanations like the improvement on one task are not modeled by one logit function but by several of them. A task would be composed of sub-tasks each modelizable by one logit function. And if this make sense, one could try to model the improvements in all of the tasks using only a small number of logit curves associated to each sub-tasks (decomposing each tasks into a set of sub-tasks with a simple trend).
(Also Gopher looks like less predictable and the data more sparse (no data point in the X0 B parameters))
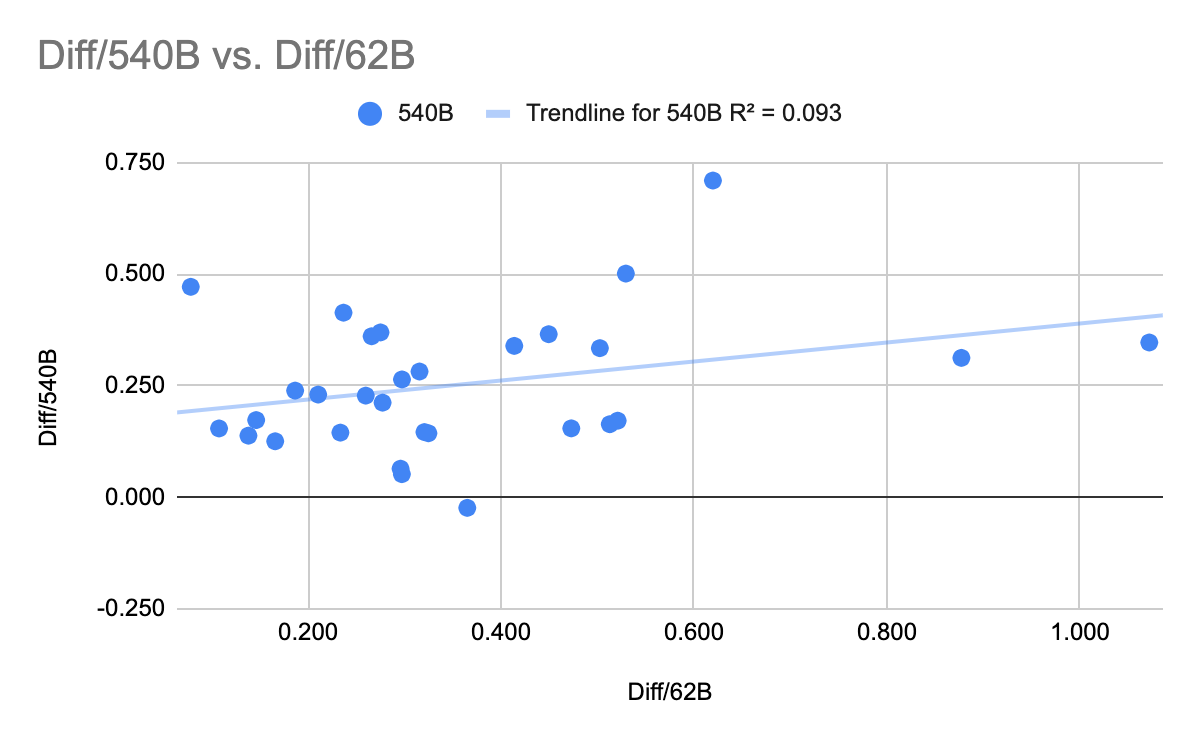
Indeed but to slightly counter balance this, at the same time, it looks like it was trained on ~500B tokens (while ~300B were used for GPT-3 and for GPT-2 something like ~50B).
It's only 1.2 billion parameters.
Indeed but to slightly counter balance this, at the same time, it looks like it was trained on ~500B tokens (while ~300B were used for GPT-3 and something like ~50B for GPT-2).
"The training algorithm has found a better representation"?? That seems strange to me since the loss should be lower in that case, not spiking. Or maybe you mean that the training broke free of a kind of local minima (without telling that he found a better one yet). Also I guess people training the models observed that waiting after these spike don't lead to better performances or they would not have removed them from the training.
Around this idea, and after looking at the "grokking" paper, I would guess that it's more likely caused by the weight decay (or similar) causing the training to break out of a kind of local minima. An interesting point may be that larger/better LM may have significantly sharper internal models and thus are more prone to this phenomenon (The weight decay (or similar) more easily breaking the more sensitive/better/sharper models).
It should be very easy to check if these spikes are caused by the weight decay "damaging" very sharp internal models. Like replay the spiky part several times with less and less weight decay... (I am curious of similar tests with varying the momentum, dropout... At looking if the spikes are initially triggered by some subset of the network, during how many training steps long are the spikes...)
I am curious to hear/read more about the issue of spikes and instabilities in training large language model (see the quote / page 11 of the paper). If someone knows a good reference about that, I am interested!
5.1 Training Instability
For the largest model, we observed spikes in the loss roughly 20 times during training, despite the fact that gradient clipping was enabled. These spikes occurred at highly irregular intervals, sometimes happening late into training, and were not observed when training the smaller models. Due to the cost of training the largest model, we were not able to determine a principled strategy to mitigate these spikes.
Instead, we found that a simple strategy to effectively mitigate the issue: We re-started training from a checkpoint roughly 100 steps before the spike started, and skipped roughly 200–500 data batches, which cover the batches that were seen before and during the spike. With this mitigation, the loss did not spike again at the same point. We do not believe that the spikes were caused by “bad data” per se, because we ran several ablation experiments where we took the batches of data that were surrounding the spike, and then trained on those same data batches starting from a different, earlier checkpoint. In these cases, we did not see a spike. This implies that spikes only occur due to the combination of specific data batches with a particular model parameter state. In the future, we plan to study more principled mitigation strategy for loss spikes in very large language models.
Here with 2 conv and less than 100k parameters the accuracy is ~92%. https://github.com/zalandoresearch/fashion-mnist
SOTA on Fashion-MNIST is >96%. https://paperswithcode.com/sota/image-classification-on-fashion-mnist
Maybe another weak solution close to "Take bigger steps": Use decentralize training.
Meaning: perform several training steps (gradient updates) in parallel on several replicates of the model and periodically synchronize the weights (like average them).
Each replicate has only access to its own inputs and local weights and thus it seems plausible that the gradient hacker can't as easily cancel gradients going against its mesa-objective.
One particularly interesting recent work in this domain was Leike et al.'s “Learning human objectives by evaluating hypothetical behaviours,” which used human feedback on hypothetical trajectories to learn how to avoid environmental traps. In the context of the capability exploration/objective exploration dichotomy, I think a lot of this work can be viewed as putting a damper on instrumental capability exploration.
Isn't this work also linked to objective exploration? One of the four "hypothetical behaviours" used is the selection of trajectories which maximizes reward uncertainty. Trajectories which are then evaluated by humans.
Is your suggestion to run this system as a source of value, simulating lives for their own sake rather than to improve the quality of life of sentient beings in our universe? Our history (and present) aren't exactly utopian, and I don't see any real reason to believe that slight variations on it would lead to anything happier.
I am thinking about if we should reasonably expect to produce better result by trying to align an AGI with our value than by simulating a lot of alternate universes. I am not saying that this is net-negative or net-positive. It seems to me that the expected value of both cases may be identical.
Also by history, I also meant the future, not only the past and present. (I edited the question to replace "histories" by "trajectories")