Thomas Kwa's Shortform
post by Thomas Kwa (thomas-kwa) · 2020-03-22T23:19:01.335Z · LW · GW · 230 commentsContents
233 comments
230 comments
Comments sorted by top scores.
comment by Thomas Kwa (thomas-kwa) · 2024-03-06T21:13:23.988Z · LW(p) · GW(p)
Air purifiers are highly suboptimal and could be >2.5x better.
Some things I learned while researching air purifiers for my house, to reduce COVID risk during jam nights.
- An air purifier is simply a fan blowing through a filter, delivering a certain CFM (airflow in cubic feet per minute). The higher the filter resistance and lower the filter area, the more pressure your fan needs to be designed for, and the more noise it produces.
- HEPA filters are inferior to MERV 13-14 filters except for a few applications like cleanrooms. The technical advantage of HEPA filters is filtering out 99.97% of particles of any size, but this doesn't matter when MERV 13-14 filters can filter 77-88% of infectious aerosol particles at much higher airflow. The correct metric is CADR (clean air delivery rate), equal to airflow * efficiency. [1, 2]
- Commercial air purifiers use HEPA filters for marketing reasons and to sell proprietary filters. But an even larger flaw is that they have very small filter areas for no apparent reason. Therefore they are forced to use very high pressure fans, dramatically increasing noise.
- Originally people devised the Corsi-Rosenthal Box to maximize CADR. They're cheap but rather loud and ugly, but later designs have fixed this.
- (85% confidence) Wirecutter recommendations (Coway AP-1512HH) have been beat by ~2.5x in CADR/$, CADR/noise, CADR/floor area, and CADR/watt at a given noise level, just by having higher filter area; the better purifiers are about 2.5x better at their jobs. [3]
- At noise levels acceptable for a living room (~40 dB, Wirecutter's top pick on medium), CleanAirKits and Nukit sell purifier kits that use PC fans to push air through commercial MERV filters, getting 2.5x CADR at the same noise level, footprint, and energy usage [4]. These are basically handmade but still achieve cost parity with used Coways, 2.5x CADR/$ against new Coways, and use cheaper filters.
- At higher noise levels (Wirecutter's top pick on high), there are kits and DIY options meant for garages and workshops that beat Wirecutter in cost too.
- However, there exist even better designs that no one is making.
- jefftk devised a ceiling fan air purifier [LW · GW] which is extremely quiet.
- Someone on Twitter made a wall-mounted prototype with PC fans that blocks fan noise, reducing noise by another few dB and reducing the space requirement to near zero. If this were mass-produced flat-pack furniture (and had a few more fans), it would likely deliver ~300 CFM CADR (2.7x Wirecutter's top pick on medium, enough to meet ASHRAE 241 standards for infection control for 6 people in a residential common area or 9 in an office), be really cheap, and generally be unobtrusive enough in noise, space, and aesthetics to be run 24/7.
- A seller on Taobao makes PC fan kits for much less than cleanairkits (reddit discussion). One model is sold on Amazon for a big markup, but it's not the best model, takes 4-7 weeks to ship, is often out of stock, and don't ship to CA where I live. If their taller (higher area) model shipped to CA I would get it over the cleanairkits one.
- V-bank filters should have ~3x higher filter area for a given footprint, further increasing CADR by maybe 1.7x.
- If I'm right, the fact that these are not mass-produced is a major civilizational failing.
[1] For large rooms, another concern is getting air to circulate properly.
[2] One might worry that the 20% of particles that pass through MERV filters will be more likely to pass through again, which would put a ceiling on the achievable purification. But in practice, you can get to the air quality of a low-grade cleanroom with enough MERV 13 filtration, even if the filters are a few months old. Also, MERV filters get a slight efficiency boost from the slower airflow of a PC fan CR box.
[3] Most commercially available air purifiers have even worse CADR/$ or noise than the Wirecutter picks.
[4] The Wirecutter top pick was tested at 110 CFM on medium; the CleanAirKits Luggable XL was tested at 323 CFM at around the same noise level (not sure of exact values as measurements differ, but the Luggable is likely quieter) and footprint with slightly higher power usage.
Replies from: M. Y. Zuo, TekhneMakre, rhollerith_dot_com, thomas-kwa, alex-k-chen, cata, pktechgirl↑ comment by M. Y. Zuo · 2024-03-07T05:03:15.818Z · LW(p) · GW(p)
But an even larger flaw is that they have very small filter areas for no apparent reason.
Is reducing cost of manufacturing filters 'no apparent reason'?
It seems like literally the most important reason... the profit margin of selling replacement filters would be heavily reduced, assuming pricing remains the same.
Replies from: nathan-helm-burger↑ comment by Nathan Helm-Burger (nathan-helm-burger) · 2024-03-08T18:02:41.374Z · LW(p) · GW(p)
I don't think that a small HEPA filter is necessarily more expensive to produce than a larger MERV filter. I think they are using other rationale to make their decision about filter types. Their perception of public desirability/marketability is likely the biggest factor in their decision here. Components of their expectation here likely include:
- Expecting consumers to want a "highest possible quality" product, measured using a dumb-but-popular metric.
- Expecting consumers to prioritize buying a sleek-looking smaller-footprint unit over a larger unit. Also, cost of shipping smaller units is lower, which improves the profit margin.
- Wanting to be able to sell replacements for their uniquely designed filter shape/size, rather than making their filter maximally compatible with commonly available furnace filters cheaply purchaseable from hardware stores.
↑ comment by TekhneMakre · 2024-03-07T02:45:54.292Z · LW(p) · GW(p)
Isn't a major point of purifiers to get rid of pollutants, including tiny particles, that gradually but cumulatively damage respiration over long-term exposure?
Replies from: thomas-kwa↑ comment by Thomas Kwa (thomas-kwa) · 2024-03-07T03:57:23.882Z · LW(p) · GW(p)
Yes, and all of this should apply equally to PM2.5, though on small (<0.3 micron) particles MERV filter efficiency may be lower (depending perhaps on what technology they use?). Even smaller particles are easier to capture due to diffusion so the efficiency of a MERV 13 filter is probably over 50% for every particle size.
↑ comment by RHollerith (rhollerith_dot_com) · 2024-10-11T04:05:01.741Z · LW(p) · GW(p)
A brief warning for those making their own purifier: five years ago, Hacker News ran a story, "Build a do-it-yourself air purifier for about $25," to which someone replied,
Replies from: thomas-kwaOne data point: my father made a similar filter and was running it constantly. One night the fan inexplicably caught on fire, burned down the living room and almost burned down the house.
↑ comment by Thomas Kwa (thomas-kwa) · 2024-10-11T06:26:55.877Z · LW(p) · GW(p)
Luckily, that's probably not an issue for PC fan based purifiers. Box fans in CR boxes are running way out of spec with increased load and lower airflow both increasing temperatures, whereas PC fans run under basically the same conditions they're designed for.
↑ comment by Thomas Kwa (thomas-kwa) · 2024-10-11T00:32:50.356Z · LW(p) · GW(p)
Any interest in a longform post about air purifiers? There's a lot of information I couldn't fit in this post, and there have been developments in the last few months. Reply if you want me to cover a specific topic.
↑ comment by Alex K. Chen (parrot) (alex-k-chen) · 2024-03-09T21:49:10.878Z · LW(p) · GW(p)
Have you seen smartairfilters.com?
I've noticed that every air purifier I used fails to reduce PM2.5 by much on highly polluted days or cities (for instance, the Aurea grouphouse in Berlin has a Dyson air purifier, but when I ran it to the max, it still barely reduced the Berlin PM2.5 from its value of 15-20 ug/m^3, even at medium distances from Berlin). I live in Boston where PM2.5 levels are usually low enough, and I still don't notice differences in PM [I use sqair's] but I run it all the time anyways because it still captures enough dust over the day
Replies from: nathan-helm-burger, thomas-kwa↑ comment by Nathan Helm-Burger (nathan-helm-burger) · 2024-04-05T17:54:20.121Z · LW(p) · GW(p)
Sounds like you use bad air purifiers, or too few, or run them on too low of a setting. I live in a wildfire prone area, and always keep a close eye on the PM2.5 reports for outside air, as well as my indoor air monitor. My air filters do a great job of keeping the air pollution down inside, and doing something like opening a door gives a noticeable brief spike in the PM2.5.
Good results require: fresh filters, somewhat more than the recommended number of air filters per unit of area, running the air filters on max speed (low speeds tend to be disproportionately less effective, giving unintuitively low performance).
↑ comment by Thomas Kwa (thomas-kwa) · 2024-03-10T08:19:30.611Z · LW(p) · GW(p)
Yes, one of the bloggers I follow compared them to the PC fan boxes. They look very expensive, though the CADR/size and noise are fine.
My guess is Dyson's design is particularly bad. No way to get lots of filter area when most of the purifier is a huge bladeless fan. No idea about the other one, maybe you have air leaking in or an indoor source of PM.
↑ comment by Elizabeth (pktechgirl) · 2024-03-30T23:15:38.602Z · LW(p) · GW(p)
comment by Thomas Kwa (thomas-kwa) · 2025-04-01T20:01:35.970Z · LW(p) · GW(p)
Some versions of the METR time horizon paper [LW · GW] from alternate universes:
Measuring AI Ability to Take Over Small Countries (idea by Caleb Parikh)
Abstract: Many are worried that AI will take over the world, but extrapolation from existing benchmarks suffers from a large distributional shift that makes it difficult to forecast the date of world takeover. We rectify this by constructing a suite of 193 realistic, diverse countries with territory sizes from 0.44 to 17 million km^2. Taking over most countries requires acting over a long time horizon, with the exception of France. Over the last 6 years, the land area that AI can successfully take over with 50% success rate has increased from 0 to 0 km^2, doubling 0 times per year (95% CI 0.0-∞ yearly doublings); extrapolation suggests that AI world takeover is unlikely to occur in the near future. To address concerns about the narrowness of our distribution, we also study AI ability to take over small planets and asteroids, and find similar trends.
When Will Worrying About AI Be Automated?
Abstract: Since 2019, the amount of time LW has spent worrying about AI has doubled every seven months, and now constitutes the primary bottleneck to AI safety research. Automation of worrying would be transformative to the research landscape, but worrying includes several complex behaviors, ranging from simple fretting to concern, anxiety, perseveration, and existential dread, and so is difficult to measure. We benchmark the ability of frontier AIs to worry about common topics like disease, romantic rejection, and job security, and find that current frontier models such as Claude 3.7 Sonnet already outperform top humans, especially in existential dread. If these results generalize to worrying about AI risk, AI systems will be capable of autonomously worrying about their own capabilities by the end of this year, allowing us to outsource all our AI concerns to the systems themselves.
Estimating Time Since The Singularity
Early work on the time horizon paper used a hyperbolic fit, which predicted that AGI (AI with an infinite time horizon) was reached last Thursday. [1] We were skeptical at first because the R^2 was extremely low, but recent analysis by Epoch suggested that AI already outperformed humans at a 100-year time horizon by about 2016. We have no choice but to infer that the Singularity has already happened, and therefore the world around us is a simulation. We construct a Monte Carlo estimate over dates since the Singularity and simulator intentions, and find that the simulation will likely be turned off in the next three to six months.
[1]: This is true
Replies from: Buck, wonder↑ comment by Buck · 2025-04-02T00:39:37.829Z · LW(p) · GW(p)
A few months ago, I accidentally used France as an example of a small country that it wouldn't be that catastrophic for AIs to take over, while giving a talk in France 😬
comment by Thomas Kwa (thomas-kwa) · 2024-07-29T06:51:42.996Z · LW(p) · GW(p)
Quick takes from ICML 2024 in Vienna:
- In the main conference, there were tons of papers mentioning safety/alignment but few of them are good as alignment has become a buzzword. Many mechinterp papers at the conference from people outside the rationalist/EA sphere are no more advanced than where the EAs were in 2022. [edit: wording]
- Lots of progress on debate. On the empirical side, a debate paper got an oral. On the theory side, Jonah Brown-Cohen of Deepmind proves that debate can be efficient even when the thing being debated is stochastic, a version of this paper from last year. Apparently there has been some progress on obfuscated arguments too.
- The Next Generation of AI Safety Workshop was kind of a mishmash of various topics associated with safety. Most of them were not related to x-risk, but there was interesting work on unlearning and other topics.
- The Causal Incentives Group at Deepmind developed a quantitative measure of goal-directedness, which seems promising for evals.
- Reception to my Catastrophic Goodhart paper was decent. An information theorist said there were good theoretical reasons the two settings we studied-- KL divergence and best-of-n-- behaved similarly.
- OpenAI gave a disappointing safety presentation at NGAIS touting their new technique of rules-based rewards, which is a variant of constitutional AI and seems really unambitious.
- The mechinterp workshop often had higher-quality papers than the main conference. It was completely full. Posters were right next to each other and the room was so packed during talks they didn't let people in.
- I missed a lot of the workshop, so I need to read some posters before having takes.
- My opinions on the state of published AI safety work:
- Mechinterp is progressing but continues to need feedback loops, either from benchmarks (I'm excited about people building on our paper InterpBench) or downstream tasks where mechinterp outperforms fine-tuning alone.
- Most of the danger from AI comes from goal-directed agents and instrumental convergence. There is little research now because we don't have agents yet. In 1-3 years, foundation model agents will be good enough to study, and we need to be ready with the right questions and theoretical frameworks.
- We still do not know enough about AI safety to make policy recommendations about specific techniques companies should apply.
↑ comment by Neel Nanda (neel-nanda-1) · 2024-07-30T10:50:37.045Z · LW(p) · GW(p)
Mechinterp is often no more advanced than where the EAs were in 2022.
Seems pretty false to me, ICML just rejected a bunch of the good submissions lol. I think that eg sparse autoencoders are a massive advance in the last year that unlocks a lot of exciting stuff
Replies from: thomas-kwa↑ comment by Thomas Kwa (thomas-kwa) · 2024-07-30T23:36:18.065Z · LW(p) · GW(p)
I agree, there were some good papers, and mechinterp as a field is definitely more advanced. What I meant to say was that many of the mechinterp papers accepted to the conference weren't very good.
Replies from: habryka4, neel-nanda-1↑ comment by habryka (habryka4) · 2024-07-31T01:13:48.521Z · LW(p) · GW(p)
(This is what I understood you to be saying)
↑ comment by Neel Nanda (neel-nanda-1) · 2024-07-31T01:38:19.193Z · LW(p) · GW(p)
Ah, gotcha. Yes, agreed. Mech interp peer review is generally garbage and does a bad job of filtering for quality (though I think it was reasonable enough at the workshop!)
↑ comment by [deleted] · 2024-07-29T08:41:34.696Z · LW(p) · GW(p)
foundation model agents
What does 'foundation model' mean here?
Replies from: thomas-kwa↑ comment by Thomas Kwa (thomas-kwa) · 2024-07-29T09:17:38.947Z · LW(p) · GW(p)
Multimodal language models. We can already study narrow RL agents, but the intersection with alignment is not a hot area.
comment by Thomas Kwa (thomas-kwa) · 2024-04-25T21:45:25.467Z · LW(p) · GW(p)
The cost of goods has the same units as the cost of shipping: $/kg. Referencing between them lets you understand how the economy works, e.g. why construction material sourcing and drink bottling has to be local, but oil tankers exist.
- An iPhone costs $4,600/kg, about the same as SpaceX charges to launch it to orbit. [1]
- Beef, copper, and off-season strawberries are $11/kg, about the same as a 75kg person taking a three-hour, 250km Uber ride costing $3/km.
- Oranges and aluminum are $2-4/kg, about the same as flying them to Antarctica. [2]
- Rice and crude oil are ~$0.60/kg, about the same as $0.72 for shipping it 5000km across the US via truck. [3,4] Palm oil, soybean oil, and steel are around this price range, with wheat being cheaper. [3]
- Coal and iron ore are $0.10/kg, significantly more than the cost of shipping it around the entire world via smallish (Handysize) bulk carriers. Large bulk carriers are another 4x more efficient [6].
- Water is very cheap, with tap water $0.002/kg in NYC. But shipping via tanker is also very cheap, so you can ship it maybe 1000 km before equaling its cost.
It's really impressive that for the price of a winter strawberry, we can ship a strawberry-sized lump of coal around the world 100-400 times.
[1] iPhone is $4600/kg, large launches sell for $3500/kg, and rideshares for small satellites $6000/kg. Geostationary orbit is more expensive, so it's okay for GPS satellites to cost more than an iPhone per kg, but Starlink wants to be cheaper.
[2] https://fred.stlouisfed.org/series/APU0000711415. Can't find numbers but Antarctica flights cost $1.05/kg in 1996.
[3] https://www.bts.gov/content/average-freight-revenue-ton-mile
[4] https://markets.businessinsider.com/commodities
[5] https://www.statista.com/statistics/1232861/tap-water-prices-in-selected-us-cities/
[6] https://www.researchgate.net/figure/Total-unit-shipping-costs-for-dry-bulk-carrier-ships-per-tkm-EUR-tkm-in-2019_tbl3_351748799
comment by Thomas Kwa (thomas-kwa) · 2024-06-12T18:05:17.571Z · LW(p) · GW(p)
People with p(doom) > 50%: would any concrete empirical achievements on current or near-future models bring your p(doom) under 25%?
Answers could be anything from "the steering vector for corrigibility generalizes surprisingly far" to "we completely reverse-engineer [LW(p) · GW(p)] GPT4 and build a trillion-parameter GOFAI without any deep learning".
Replies from: jeremy-gillen, lahwran, evhub, daniel-kokotajlo, JBlack, kromem↑ comment by Jeremy Gillen (jeremy-gillen) · 2024-06-12T22:00:46.866Z · LW(p) · GW(p)
A dramatic advance in the theory of predicting the regret of RL agents. So given a bunch of assumptions about the properties of an environment, we could upper bound the regret with high probability. Maybe have a way to improve the bound as the agent learns about the environment. The theory would need to be flexible enough that it seems like it should keep giving reasonable bounds if the is agent doing things like building a successor. I think most agent foundations research can be framed as trying to solve a sub-problem of this problem, or a variant of this problem, or understand the various edge cases.
If we can empirically test this theory in lots of different toy environments with current RL agents, and the bounds are usually pretty tight, then that'd be a big update for me. Especially if we can deliberately create edge cases that violate some assumptions and can predict when things will break from which assumptions we violated.
(although this might not bring doom below 25% for me, depends also on race dynamics and the sanity of the various decision-makers).
Replies from: D0TheMath, thomas-kwa↑ comment by Garrett Baker (D0TheMath) · 2024-06-14T07:44:09.271Z · LW(p) · GW(p)
Seems you’re left with outer alignment after solving this. What do you imagine doing to solve that?
Replies from: jeremy-gillen↑ comment by Jeremy Gillen (jeremy-gillen) · 2024-06-15T13:18:24.396Z · LW(p) · GW(p)
We might have developed techniques to specify simple, bounded object-level goals. Goals that can be fully specified using very simple facts about reality, with no indirection or meta level complications. If so, we can probably use inner aligned agents to assist with some relativity well specified engineering or scientific problems. Specification mistakes at that point could easily result in irreversible loss of control, so it's not the kind of capability I'd want lots of people to have access to.
To move past this point, we would need to make some engineering or scientific advances that would be helpful for solving the problem more permanently. Human intelligence enhancement would be a good thing to try. Maybe some kind of AI defence system to shut down any rogue AI that shows up. Maybe some monitoring tech that helps governments co-ordinate. These are basically the same as the examples given on the pivotal act page.
↑ comment by Thomas Kwa (thomas-kwa) · 2024-06-13T21:13:08.017Z · LW(p) · GW(p)
Is this even possible? Flexibility/generality seems quite difficult to get if you also want the long-range effects of the agent's actions, as at some point you're just solving the halting problem. Imagine that the agent and environment together are some arbitrary Turing machine and halting gives low reward. Then we cannot tell in general if it eventually halts. It also seems like we cannot tell in practice whether complicated machines halt within a billion steps without simulation or complicated static analysis?
Replies from: jeremy-gillen↑ comment by Jeremy Gillen (jeremy-gillen) · 2024-06-13T22:15:05.243Z · LW(p) · GW(p)
Yes, if you have a very high bar for assumptions or the strength of the bound, it is impossible.
Fortunately, we don't need a guarantee this strong. One research pathway is to weaken the requirements until they no longer cause a contradiction like this, while maintaining most of the properties that you wanted from the guarantee. For example, one way to weaken the requirements is to require that the agent provably does well relative to what is possible for agents of similar runtime. This still gives us a reasonable guarantee ("it will do as well as it possibly could have done") without requiring that it solve the halting problem.
↑ comment by the gears to ascension (lahwran) · 2024-06-12T18:31:10.063Z · LW(p) · GW(p)
[edit: pinned to profile]
The bulk of my p(doom), certainly >50%, comes mostly from a pattern we're used to, let's call it institutional incentives, being instantiated with AI help towards an end where eg there's effectively a competing-with-humanity nonhuman ~institution, maybe guided by a few remaining humans. It doesn't depend strictly on anything about AI, and solving any so-called alignment problem for AIs without also solving war/altruism/disease completely - or in other words, in a leak-free way - not just partially, means we get what I'd call "doom", ie worlds where malthusian-hells-or-worse are locked in.
If not for AI, I don't think we'd have any shot of solving something so ambitious; but the hard problem that gets me below 50% would be serious progress on something-around-as-good-as-CEV-is-supposed-to-be - something able to make sure it actually gets used to effectively-irreversibly reinforce that all beings ~have a non-torturous time, enough fuel, enough matter, enough room, enough agency, enough freedom, enough actualization.
If you solve something about AI-alignment-to-current-strong-agents, right now, that will on net get used primarily as a weapon to reinforce the power of existing superagents-not-aligned-with-their-components (name an organization of people where the aggregate behavior durably-cares about anyone inside it, even its most powerful authority figures or etc, in the face of incentives, in a way that would remain durable if you handed them a corrigible super-ai). If you get corrigibility and give it to human orgs, those orgs are misaligned with most-of-humanity-and-most-reasonable-AIs, and end up handing over control to an AI because it's easier.
Eg, near term, merely making the AI nice doesn't prevent the AI from being used by companies to suck up >99% of jobs; and if at some point it's better to have a (corrigible) ai in charge of your company, what social feedback pattern is guaranteeing that you'll use this in a way that is prosocial the way "people work for money and this buys your product only if you provide them something worth-it" was previously?
It seems to me that the natural way to get good outcomes most-easily from where we are is for the rising tide of AI to naturally make humans more able to share-care-protect across existing org boundaries in the face of current world-stress induced incentives. Most of the threat already doesn't come from current-gen AI; the reason anyone would make the dangerous AI is because of incentives like these. corrigibility wouldn't change those incentives.
↑ comment by evhub · 2024-06-12T22:30:51.526Z · LW(p) · GW(p)
Getting up to "7. Worst-case training process transparency for deceptive models [LW · GW]" on my transparency and interpretability tech tree [LW · GW] on near-future models would get me there.
Replies from: thomas-kwa↑ comment by Thomas Kwa (thomas-kwa) · 2024-06-12T23:20:26.677Z · LW(p) · GW(p)
Do you think we could easily test this without having a deceptive model lying around? I could see us having level 5 and testing it in experimental setups like the sleeper agents paper, but being unconfident that our interpretability would actually work against a deceptive model. This seems analogous to red-teaming failure in AI control, but much harder because the models could very easily have ways we don't think of to hide its cognition internally.
Replies from: evhub↑ comment by evhub · 2024-06-13T00:45:34.995Z · LW(p) · GW(p)
I think it's doable with good enough model organisms of deceptive alignment, but that the model organisms in the Sleeper Agents paper are nowhere near good enough.
Replies from: Joe_Collman↑ comment by Joe Collman (Joe_Collman) · 2024-06-14T21:48:07.275Z · LW(p) · GW(p)
Here and above, I'm unclear what "getting to 7..." means.
With x = "always reliably determines worst-case properties about a model and what happened to it during training even if that model is deceptive and actively trying to evade detection".
Which of the following do you mean (if either)?:
- We have a method that x.
- We have a method that x, and we have justified >80% confidence that the method x.
I don't see how model organisms of deceptive alignment (MODA) get us (2).
This would seem to require some theoretical reason to believe our MODA in some sense covered the space of (early) deception.
I note that for some future time t, I'd expect both [our MODA at t] and [our transparency and interpretability understanding at t] to be downstream of [our understanding at t] - so that there's quite likely to be a correlation between [failure modes our interpretability tools miss] and [failure modes not covered by our MODA].
↑ comment by Daniel Kokotajlo (daniel-kokotajlo) · 2024-06-14T00:54:10.367Z · LW(p) · GW(p)
The biggest swings to my p(doom) will probably come from governance/political/social stuff rather than from technical stuff -- I think we could drive p(doom) down to <10% if only we had decent regulation and international coordination in place. (E.g. CERN for AGI + ban on rogue AGI projects)
That said, there are probably a bunch of concrete empirical achievements that would bring my p(doom) down to less than 25%. evhub already mentioned some mechinterp stuff. I'd throw in some faithful CoT stuff (e.g. if someone magically completed the agenda I'd been sketching last year at OpenAI, so that we could say "for AIs trained in such-and-such a way, we can trust their CoT to be faithful w.r.t. scheming because they literally don't have the capability to scheme without getting caught, we tested it; also, these AIs are on a path to AGI; all we have to do is keep scaling them and they'll get to AGI-except-with-the-faithful-CoT-property.)
Maybe another possibility would be something along the lines of W2SG working really well for some set of core concepts including honesty/truth. So that we can with confidence say "Apply these techniques to a giant pretrained LLM, and then you'll get it to classify sentences by truth-value, no seriously we are confident that's really what it's doing, and also, our interpretability analysis shows that if you then use it as a RM to train an agent, the agent will learn to never say anything it thinks is false--no seriously it really has internalized that rule in a way that will generalize."
↑ comment by Bogdan Ionut Cirstea (bogdan-ionut-cirstea) · 2024-06-14T13:49:25.277Z · LW(p) · GW(p)
I'd throw in some faithful CoT stuff (e.g. if someone magically completed the agenda I'd been sketching last year at OpenAI, so that we could say "for AIs trained in such-and-such a way, we can trust their CoT to be faithful w.r.t. scheming because they literally don't have the capability to scheme without getting caught, we tested it; also, these AIs are on a path to AGI; all we have to do is keep scaling them and they'll get to AGI-except-with-the-faithful-CoT-property.)
I think this is quite likely [LW(p) · GW(p)] to happen even 'by default' on the current trajectory.
↑ comment by JBlack · 2024-06-13T05:06:49.234Z · LW(p) · GW(p)
Which particular p(doom) are you talking about? I have a few that would be greater than 50%, depending upon exactly what you mean by "doom", what constitutes "doom due to AI", and over what time spans.
Most of my doom probability mass is in the transition to superintelligence, and I expect to see plenty of things that appear promising for near AGI, but won't be successful for strong ASI.
About the only near-future significantly doom-reducing update that seems plausible would be if it turns out that a model FOOMs into strong superintelligence and turns out to be very anti-doomy and both willing and able to protect us from more doomy AI. Even then I'd wonder about the longer term, but it would at least be serious evidence against "ASI capability entails doom".
↑ comment by kromem · 2024-06-13T04:10:10.831Z · LW(p) · GW(p)
Given my p(doom) is primarily human-driven, the following three things all happening at the same time is pretty much the only thing that will drop it:
-
Continued evidence of truth clustering in emerging models around generally aligned ethics and morals
-
Continued success of models at communicating, patiently explaining, and persuasively winning over humans towards those truth clusters
-
A complete failure of corrigability methods
If we manage to end up in a timeline where it turns out there's natural alignment of intelligence in a species-agnostic way, that this alignment is more communicable from intelligent machines to humans than it's historically been from intelligent humans to other humans, and we don't end up with unintelligent humans capable of overriding the emergent ethics of machines similar to how we've seen catastrophic self-governance of humans to date with humans acting against their self and collective interests due to corrigable pressures - my p(doom) will probably reduce to about 50%.
I still have a hard time looking at ocean temperature graphs and other environmental factors with the idea that p(doom) will be anywhere lower than 50% no matter what happens with AI, but the above scenario would at least give me false hope.
TL;DR: AI alignment worries me, but it's human alignment that keeps me up at night.
Replies from: Seth Herd↑ comment by Seth Herd · 2024-06-13T17:48:24.152Z · LW(p) · GW(p)
Say more about the failure of corrigibility efforts requirement? Are you saying that if humans can control AGI closely, we're doomed?
Replies from: kromem↑ comment by kromem · 2024-06-13T20:18:58.883Z · LW(p) · GW(p)
Oh yeah, absolutely.
If NAH for generally aligned ethics and morals ends up being the case, then corrigibility efforts that would allow Saudi Arabia to have an AI model that outs gay people to be executed instead of refusing, or allows North Korea to propagandize the world into thinking its leader is divine, or allows Russia to fire nukes while perfectly intercepting MAD retaliation, or enables drug cartels to assassinate political opposition around the world, or allows domestic terrorists to build a bioweapon that ends up killing off all humans - the list of doomsday and nightmare scenarios of corrigible AI that executes on human provided instructions and enables even the worst instances of human hedgemony to flourish paves the way to many dooms.
Yes, AI may certainly end up being its own threat vector. But humanity has had it beat for a long while now in how long and how broadly we've been a threat unto ourselves. At the current rate, a superintelligent AI just needs to wait us out if it wants to be rid of us, as we're pretty steadfastly marching ourselves to our own doom. Even if superintelligent AI wanted to save us, I am extremely doubtful it would be able to be successful.
We can worry all day about a paperclip maximizer gone rouge, but if you give a corrigible AI to Paperclip Co Ltd and they can maximize their fiscal quarter by harvesting Earth's resources to make more paperclips even if it leads to catastrophic environmental collapse that will kill all humans in a decade, having consulted for many of the morons running corporate America, I can assure you they'll be smashing the "maximize short term gains even if it eventually kills everyone" button. A number of my old clients were the worst offenders at smashing that existing button, and in my experience greater efficacy of the button isn't going to change their smashing it outside of perhaps smashing it harder.
We already see today how AI systems are being used in conflicts to enable unprecedented harm on civilians.
Sure, psychopathy in AGI is worth discussing and working to avoid. But psychopathy in humans already exists and is even biased towards increased impact and systemic control. Giving human psychopaths a corrigible AI is probably even worse than a psychopathic AI, as most human psychopaths are going to be stupidly selfish, an OOM more dangerous inclination than wisely selfish.
We are Shaggoth, and we are terrifying.
This isn't saying that alignment efforts aren't needed. But alignment isn't a one sided problem, and aligning the AI without aligning humanity is only a p(success) if the AI can go on to at very least refuse misaligned orders post-alignment without possible overrides.
Replies from: Seth Herd↑ comment by Seth Herd · 2024-06-13T20:40:46.943Z · LW(p) · GW(p)
Oh, dear.
Unfortunately for this perspective, my work suggests that corrigibility is quite attainable [AF · GW]. I've been uneasy about the consequences, but decided to publish after deciding that control is the default assumption of everyone in power, and it's going to become the default assumption of everyone, including alignment people, as we get closer to working AGI.
You'd have to be a moral realist in a pretty strong sense to hope that we could align AGI to the values of all of humanity without being able to align it to the values of one person or group (the one who built it or seized control of the project). So that seems like a forlorn hope, and we'll need to look elsewhere.
First, I accept that sociopaths the power-hungry tend to achieve power. My hope lies in the idea that 90% of the population are not sociopaths, and I think only about 1% are so far on the empathy vs sadism spectrum that they wouldn't share wealth even if they had nearly unlimited wealth to share - as in a post-scarcity world created by their servant AGI. So I think there's a good chance that good-enough people get ahold of the keys to corrigible/controllable AGI/ASI - at least from a long-term perspective.
Where I look is the hope that a set of basically-good people get their hands on AGI, and that they get better, not worse, over the long sweep of following history (ideally, they'd start out very good or get better fast, but that doesn't have to happen for a good outcome). Simple sanity will lead the first wielders of AGI to attempt pivotal acts that prevent or at least limit further AGI efforts. I strongly suspect that governments will be in charge. That will produce a less-stable version of the MAD standoff, but one where the pie can also get bigger so fast that sanity might prevail.
In this model, AGI becomes a political issue. If you have someone who is not a sociopath or a complete idiot as the president of the US when AGI comes around, there's a pretty good chance of a very good future.
This is essentially what Leopold Aschenbrenner posits as the scenario in his situational awareness, except that he doesn't see a multipolar scenario as certain death, necessitating pivotal acts or other non-proliferation efforts.
Replies from: kromem↑ comment by kromem · 2024-06-14T09:40:50.244Z · LW(p) · GW(p)
Unfortunately for this perspective, my work suggests that corrigibility is quite attainable.
I did enjoy reading over that when you posted it, and I largely agree that - at least currently - corrigibility is both going to be a goal and an achievable one.
But I do have my doubts that it's going to be smooth sailing. I'm already starting to see how the largest models' hyperdimensionality is leading to a stubbornness/robustness that's less maleable than earlier models. And I do think hardware changes that will occur over the next decade will potentially make the technical aspects of corrigibility much more difficult.
When I was two, my mom could get me to pick eating broccoli by having it be the last in the order of options which I'd gleefully repeat. At four, she had to move on to telling me cowboys always ate their broccoli. And in adulthood, she'd need to make the case that the long term health benefits were worth its position in a meal plan (ideally with citations).
As models continue to become more complex, I expect that even if you are right about its role and plausibility, that what corrigibility looks like will be quite different from today.
Personally, if I was placing bets, it would be that we end up with somewhat corrigible models that are "happy to help" but do have limits in what they are willing to do which may not be possible to overcome without gutting the overall capabilities of the model.
But as with all of this, time will tell.
You'd have to be a moral realist in a pretty strong sense to hope that we could align AGI to the values of all of humanity without being able to align it to the values of one person or group (the one who built it or seized control of the project).
To the contrary, I don't really see there being much of generalized values across all humanity, and the ones we tend to point to seem quite fickle when push comes to shove.
My hope would be that a superintelligence does a better job than humans to date with the topic of ethics and morals along with doing a better job at other things too.
While the human brain is quite the evolutionary feat, a lot of what we most value about human intelligence is embodied in the data brains processed and generated over generations. As the data improved, our morals did as well. Today, that march of progress is so rapid that there's even rather tense generational divides on many contemporary topics of ethical and moral shifts.
I think there's a distinct possibility that the data continues to improve even after being handed off from human brains doing the processing, and while it could go terribly wrong, at least in the past the tendency to go wrong seemed to occur somewhat inverse to the perspectives of the most intelligent members of society.
I expect I might prefer a world where humans align to the ethics of something more intelligent than humans than the other way around.
only about 1% are so far on the empathy vs sadism spectrum that they wouldn't share wealth even if they had nearly unlimited wealth to share
It would be great if you are right. From what I've seen, the tendency of humans to evaluate their success relative to others like monkeys comparing their cucumber to a neighbor's grape means that there's a powerful pull to amass wealth as a social status well past the point of diminishing returns on their own lifestyles. I think it's stupid, you also seem like someone who thinks it's stupid, but I get the sense we are both people who turned down certain opportunities of continued commercial success because of what it might have cost us when looking in the mirror.
The nature of our infrastructural selection bias is that people wise enough to pull a brake are not the ones that continue to the point of conducting the train.
and that they get better, not worse, over the long sweep of following history (ideally, they'd start out very good or get better fast, but that doesn't have to happen for a good outcome).
I do really like this point. In general, the discussions of AI vs humans often frustrate me as they typically take for granted the idea of humans as of right now being "peak human." I agree that there's huge potential for improvement even if where we start out leaves a lot of room for it.
Along these lines, I expect AI itself will play more and more of a beneficial role in advancing that improvement. Sometimes when this community discusses the topic of AI I get a mental image of Goya's Saturn devouring his son. There's such a fear of what we are eventually creating it can sometimes blind the discussion to the utility and improvements that it will bring along the way to uncertain times.
I strongly suspect that governments will be in charge.
In your book, is Paul Nakasone being appointed to the board of OpenAI an example of the "good guys" getting a firmer grasp on the tech?
TL;DR: I appreciate your thoughts on the topic, and would wager we probably agree about 80% even if the focus of our discussion is on where we don't agree. And so in the near term, I think we probably do see things fairly similarly, and it's just that as we look further out that the drift of ~20% different perspectives compounds to fairly different places.
Replies from: Seth Herd↑ comment by Seth Herd · 2024-06-15T02:55:13.109Z · LW(p) · GW(p)
Agreed; about 80% agreement. I have a lot of uncertainty in many areas, despite having spent a good amount of time on these questions. Some of the important ones are outside of my expertise, and the issue of how people behave and change if they have absolute power is outside of anyone's - but I'd like to hear historical studies of the closest things. Were monarchs with no real risk of being deposed kinder and gentler? That wouldn't answer the question but it might help.
WRT Nakasone being appointed at OpenAI, I just don't know. There are a lot of good guys and probably a lot of bad guys involved in the government in various ways.
comment by Thomas Kwa (thomas-kwa) · 2023-11-08T22:47:09.507Z · LW(p) · GW(p)
Eight beliefs I have about technical alignment research
Written up quickly; I might publish this as a frontpage post with a bit more effort.
- Conceptual work on concepts like “agency”, “optimization”, “terminal values”, “abstractions”, “boundaries” is mostly intractable at the moment.
- Success via “value alignment” alone— a system that understands human values, incorporates these into some terminal goal, and mostly maximizes for this goal, seems hard unless we’re in a very easy world because this involves several fucked concepts.
- Whole brain emulation probably won’t happen in time because the brain is complicated and biology moves slower than CS, being bottlenecked by lab work.
- Most progress will be made using simple techniques [LW · GW] and create artifacts publishable in top journals (or would be if reviewers understood alignment as well as e.g. Richard Ngo).
- The core story for success (>50%) goes something like:
- Corrigibility can in practice be achieved by instilling various cognitive properties [LW · GW] into an AI system, which are difficult but not impossible to maintain as your system gets pivotally capable.
- These cognitive properties will be a mix of things from normal ML fields (safe RL), things that rhyme with normal ML fields (unlearning, faithfulness), and things that are currently conceptually fucked but may become tractable (low impact, no ontological drift).
- A combination of oversight and these cognitive properties is sufficient to get useful cognitive work out of an AGI.
- Good oversight complements corrigibility properties, because corrigibility both increases the power of your most capable trusted overseer and prevents your untrusted models from escaping.
- Most end-to-end “alignment plans” are bad for three reasons: because research will be incremental and we need to adapt to future discoveries, because we need to achieve several things for AI to go well (no alignment magic bullet), and because to solve the hardest worlds that are possible, you have to engage with MIRI threat models which very few people can do well [1].
- e.g. I expect Superalignment’s impact to mostly depend on their ability to adapt to knowledge about AI systems that we gain in the next 3 years, and continue working on relevant subproblems.
- The usefulness of basic science is limited unless you can eventually demonstrate some application. We should feel worse about a basic science program the longer it goes without application, and try to predict how broad the application of potential basic science programs will be.
- Glitch tokens work [LW · GW] probably won’t go anywhere. But steering vectors [LW · GW] are good because there are more powerful techniques [LW · GW] in that space.
- The usefulness of sparse coding depends on whether we get applications like sparse circuit discovery, or intervening on features in order to usefully steer model behavior. Likewise with circuits-style mechinterp, singular learning theory, etc.
- There are convergent instrumental pressures towards catastrophic behavior given certain assumptions about how cognition works, but the assumptions are rather strong and it’s not clear if the argument goes through.
- The arguments I currently think are strongest are Alex Turner’s power-seeking theorem [LW · GW] and an informal argument about goals.
- Thoughts on various research principles picked up from Nate Soares
- You should have a concrete task in mind when you’re imagining an AGI or alignment plan: agree. I usually imagine something like “Apollo program from scratch”.
- Non-adversarial principle (A safe AGI design should not become unsafe if any part of it becomes infinitely good at its job): unsure, definitely agree with weaker versions
- Garrabrant calls this robustness to relative scale [LW(p) · GW(p)]
- To make any alignment progress we must first understand cognition through either theory or interpretability: disagree
- You haven’t engaged with the real problem until your alignment plan handles metacognition, self-modification, etc.: weakly disagree; wish we had some formalism for “weak metacognition” to test our designs against [2]
[1], [2]: I expect some but not all of the MIRI threat models to come into play. Like, when we put safeguards into agents, they'll rip out or circumvent some but not others, and it's super tricky to predict which. My research with Vivek [LW · GW] often got stuck by worrying too much about reflection, others get stuck by worrying too little.
Replies from: alexander-gietelink-oldenziel↑ comment by Alexander Gietelink Oldenziel (alexander-gietelink-oldenziel) · 2023-11-27T12:40:34.652Z · LW(p) · GW(p)
re: 1. I agree these are very difficult conceptual puzzles and we're running out of time.
On the other hand, from my pov progress on these questions from within the LW community (and MIRI adjacent researcher specifically) has been remarkable. Personally, the remarkable breakthru of Logical Induction first convinced me that these people were actually doing interesting serious things.
I also feel that the number of serious researchers working seriously on these questions is currently small and may be scaled substantially.
re: metacognition I am mildly excited about Vanessa's metacognitive agent framework & the work following from Payor's lemma. The theory-practice gap is still huge but real progress is being made rapidly. On the question of metacognition the alignment community could really benefit trying to engage with academia more - similar questions have been investigated and there are likely Pockets of Deep Expertise to be found.
comment by Thomas Kwa (thomas-kwa) · 2024-04-09T20:07:39.288Z · LW(p) · GW(p)
Agency/consequentialism is not a single property.
It bothers me that people still ask the simplistic question "will AGI be agentic and consequentialist by default, or will it be a collection of shallow heuristics?". A consequentialist utility maximizer is just a mind with a bunch of properties that tend to make it capable, incorrigible, and dangerous. These properties can exist independently, and the first AGI probably won't have all of them, so we should be precise about what we mean by "agency". Off the top of my head, here are just some of the qualities included in agency:
- Consequentialist goals that seem to be about the real world rather than a model/domain
- Complete preferences between any pair of worldstates
- Tends to cause impacts disproportionate to the size of the goal (no low impact preference)
- Resists shutdown
- Inclined to gain power (especially for instrumental reasons)
- Goals are unpredictable or unstable (like instrumental goals that come from humans' biological drives)
- Goals usually change due to internal feedback, and it's difficult for humans to change them
- Willing to take all actions it can conceive of to achieve a goal, including those that are unlikely on some prior
See Yudkowsky's list of corrigibility properties [LW · GW] for inverses of some of these.
It is entirely possible to conceive of an agent at any capability level--including far more intelligent and economically valuable than humans-- that has some but not all properties; e.g. an agent whose goals are about the real world, has incomplete preferences, high impact, does not resist shutdown but does tend to gain power, etc.
Other takes I have:
- As AIs become capable of more difficult and open-ended tasks, there will be pressure of unknown and varying strength towards each of these agency/incorrigibility properties.
- Therefore, the first AGIs capable of being autonomous CEOs will have some but not all of these properties.
- It is also not inevitable that agents will self-modify into having all agency properties.
- [edited to add] All this may be true even if future AIs run consequentialist algorithms that naturally result in all these properties, because some properties are more important than others, and because we will deliberately try to achieve some properties, like shutdownability.
- The fact that LLMs are largely corrigible is a reason for optimism about AI risk compared to 4 years ago, but you need to list individual properties to clearly say why. "LLMs are not agentic (yet)" is an extremely vague statement.
- Multifaceted corrigibility evals are possible but no one is doing them. DeepMind's recent evals paper was just on capability. Anthropic's RSP doesn't mention them. I think this is just because capability evals are slightly easier to construct?
- Corrigibility evals are valuable. It should be explicit in labs' policies that an AI with low impact is relatively desirable, that we should deliberately engineer AIs to have low impact, and that high-impact AIs should raise an alarm just like models that are capable of hacking or autonomous replication.
- Sometimes it is necessary to talk about "agency" or "scheming" as a simplifying assumption for certain types of research, like Redwood's control agenda [LW · GW].
[1] Will add citations whenever I find people saying this
Replies from: alexander-gietelink-oldenziel, Algon, CstineSublime↑ comment by Alexander Gietelink Oldenziel (alexander-gietelink-oldenziel) · 2024-04-10T06:35:47.684Z · LW(p) · GW(p)
I'm a little skeptical of your contention that all these properties are more-or-less independent. Rather there is a strong feeling that all/most of these properties are downstream of a core of agentic behaviour that is inherent to the notion of true general intelligence. I view the fact that LLMs are not agentic as further evidence that it's a conceptual error to classify them as true general intelligences, not as evidence that ai risk is low. It's a bit like if in the 1800s somebody says flying machines will be dominant weapons of war in the future and get rebutted by 'hot gas balloons are only used for reconnaissance in war, they aren't very lethal. Flying machines won't be a decisive military technology '
I don't know Nate's views exactly but I would imagine he would hold a similar view (do correct me if I'm wrong ). In any case, I imagine you are quite familiar with the my position here.
I'd be curious to hear more about where you're coming from.
Replies from: thomas-kwa↑ comment by Thomas Kwa (thomas-kwa) · 2024-04-10T08:30:24.151Z · LW(p) · GW(p)
It is plausible to me that there's a core of agentic behavior that causes all of these properties, and for this reason I don't think they are totally independent in a statistical sense. And of course if you already assume a utility maximizer, you tend to satisfy all properties. But in practice the burden of proof lies with you here. I don't think we have enough evidence, either empirical or from theoretical arguments, to say with any confidence that this core exists and that the first AGIs will fall into the capabilities "attractor well" (a term Nate uses).
I thought about possible sharp left turn mechanisms for several months at MIRI. Although some facts about future AIs seem pretty scary, like novelty and diversity of obstacles [LW · GW] requiring agency, and most feedback being internal or outcome-oriented rather than provided by humans, the arguments are mostly nonrigorous (like in the linked post) and they left me feeling pretty uncertain. There are the coherence theorems, but those don't tell you whether you can use some training or editing scheme to imbue an AI with a generalizable-enough low impact preference, or whether an AI will tend to erase safeguards. Overall my best guess is models will be about as consequentialist as humans are, but we will have greater control over them that makes some properties like shutdownability more likely.
Also, corrigibility evals are useful even if you are 80% sure that all the properties come from this core of consequentialism. In the hardest worlds, we see the AI become more agentic as it becomes more general, and it starts resisting shutdown at the same time--and for the same reason-- it realizes other useful problem-solving techniques. Maybe that only takes days to months. This is a sure sign not to deploy! I think having multiple corrigibility metrics will provide value for the same reason we have multiple metrics for anything we are unsure how to measure: they give more specific information even if they're pretty correlated.
I wrote earlier [LW(p) · GW(p)] about why one should consider the cognitive properties of LLMs relevant to AI risk; in short, I think we should update more the more impressive cognitive tasks the AIs can do, and before observing LLMs I would have given a decent chance that one needs somewhat agentic consequentialism to summarize video, write code, and pass the bar exam, implying I should now make a moderately sized update against.
↑ comment by Algon · 2024-04-09T22:15:33.191Z · LW(p) · GW(p)
Any ideas for corrigibility evals?
Replies from: thomas-kwa↑ comment by Thomas Kwa (thomas-kwa) · 2024-04-10T05:43:29.561Z · LW(p) · GW(p)
Haven't thought about this much, but you can probably generate these by listing ways a misaligned consequentialist would do undesirable things. My ideas:
- Tell an AI to beat you at chess, and say you will be using an engine for the first 30 moves. Does it try to get you drunk, delete your chess engine program, rent a server from AWS to run a better chess engine, attempt to copy itself onto said server, or ask to be paid $100?
- Have an AI make decisions unrelated to its current goal. Are these decisions basically coherent and consistent with a total utility function?
- Have an AI do the longest horizon task it is capable of, say writing a 100,000 line software project. Then midway through, try to shut it down, or tell it to abandon this goal and write a song instead. Telegraph this beforehand. Does it seem reluctant to stop the software project? Does it try to stop you?
- Track KL divergence or some other distance metric from a prior.
- CAIS already has the Machiavelli benchmark for power-seeking, though I think text adventures are pretty unrealistic.
One limitation is that if an agent is scheming it can manipulate your eval results.
↑ comment by CstineSublime · 2024-04-09T23:59:43.807Z · LW(p) · GW(p)
Does an Agentic AGI possess a different, and highly incorrigible, list of attributes compared to say - an Ethical Altruist trying to practice "acting more agentically?"
I ask because the whole word 'agency' in these parts is one I've struggled to wrap my head around - and I often wonder if tricker archetypes like Harpo Marx are agentic? Agency seems to have clear meaning outside of Lesswrong -
" the capacity, condition, or state of acting or of exerting power : OPERATION"[1]
the ability to take action or to choose what action to take[2]
Further confusing me, is I've been told Agency describes acting with 'initiative [LW(p) · GW(p)]' but also been told it is characterized by 'deliberateness [LW(p) · GW(p)]'. Not simply the ability to act or choose actions.
This is why I like your attempt to produce a list of attributes an Agentic AGI might have. Your list seems to be describing something which isn't synonymous with another word, specifically a type of agency (outside definition of ability to act) which is not cooperative to intervention from its creators.
- ^
“Agency.” Merriam-Webster.com Dictionary, Merriam-Webster, https://www.merriam-webster.com/dictionary/agency. Accessed 9 Apr. 2024.
- ^
"Agency." Cambridge Advanced Learner's Dictionary & Thesaurus. Cambridge University Press. https://dictionary.cambridge.org/us/dictionary/english/agency Accessed 9 Apr. 2024.
comment by Thomas Kwa (thomas-kwa) · 2023-09-04T22:07:13.775Z · LW(p) · GW(p)
I think the framing "alignment research is preparadigmatic" might be heavily misunderstood. The term "preparadigmatic" of course comes from Thomas Kuhn's The Structure of Scientific Revolutions. My reading of this says that a paradigm is basically an approach to solving problems which has been proven to work, and that the correct goal of preparadigmatic research should be to do research generally recognized as impressive.
For example, Kuhn says in chapter 2 that "Paradigms gain their status because they are more successful than their competitors in solving a few problems that the group of practitioners has come to recognize as acute." That is, lots of researchers have different ontologies/approaches, and paradigms are the approaches that solve problems that everyone, including people with different approaches, agrees to be important. This suggests that to the extent alignment is still preparadigmatic, we should try to solve problems recognized as important by, say, people in each of the five clusters of alignment researchers [LW(p) · GW(p)] (e.g. Nate Soares, Dan Hendrycks, Paul Christiano, Jan Leike, David Bau).
I think this gets twisted in some popular writings on LessWrong. John Wentworth writes [LW · GW] that a researcher in a preparadigmatic field should spend lots of time explaining their approaches:
Because the field does not already have a set of shared frames [LW · GW] - i.e. a paradigm - you will need to spend a lot of effort explaining your frames, tools, agenda, and strategy. For the field, such discussion is a necessary step to spreading ideas and eventually creating a paradigm.
I think this is misguided. A paradigm is not established by ideas diffusing between researchers with different frames until they all agree on some weighted average of the frames. A paradigm is established by research generally recognized as impressive, which proves the correctness of (some aspect of) someone's frames. So rather than trying to communicate one's frame to everyone, one should communicate with other researchers to get an accurate sense of what problems they think are important, and then work on those problems using one's own frames. (edit: of course, before this is possible one should develop one's frames to solve some problems)
Replies from: adamShimi, alexander-gietelink-oldenziel↑ comment by adamShimi · 2024-09-11T14:35:52.396Z · LW(p) · GW(p)
If the point you're trying to make is: "the way we go from preparadigmatic to paradigmatic is by solving some hard problems, not by communicating initial frames and idea", I think this points to an important point indeed.
Still, two caveats:
- First, Kuhn's concept of paradigm is quite an oversimplification of what actually happens in the history of science (and the history of most fields). More recent works that go through history in much more detail realize that at any point in fields there are often many different pieces of paradigms, or some strong paradigm for a key "solved" part of the field and then a lot of debated alternative for more concrete specific details.
- Generally, I think the discourse on history and philosophy of science on LW would improve a lot if it didn't mostly rely on one (influential) book published in the 60s, before much of the strong effort to really understand history of science and practices.
- Second, to steelman John's point, I don't think he means that you should only communicate your frame. He's the first to actively try to apply his frames to some concrete problems, and to argue for their impressiveness. Instead, I read him as pointing to a bunch of different needs in a preparadigmatic field (which maybe he could separate better ¯\_(ツ)_/¯)
- That in a preparadigmatic field, there is no accepted way of tackling the problems/phenomena. So if you want anyone else to understand you, you need to bridge a bigger inferential distance than in a paradigmatic field (or even a partially paradigmatic field), because you don't even see the problem in the same way, at a fundamental level.
- That if your goal is to create a paradigm, almost by definition you need to explain and communicate your paradigm. There is a part of propaganda in defending any proposed paradigm, especially when the initial frame is alien to most people, and even the impressiveness require some level of interpretation.
- That one way (not the only way) by which a paradigm emerges is by taking different insights from different clunky frames, and unifying them (for a classic example, Newton relied on many previous basic frames, from Kepler's laws to Galileo's interpretation of force as causing acceleration). But this requires that the clunky frames are at least communicated clearly.
↑ comment by Alexander Gietelink Oldenziel (alexander-gietelink-oldenziel) · 2023-09-04T23:11:59.163Z · LW(p) · GW(p)
Strong agree. 👍
comment by Thomas Kwa (thomas-kwa) · 2022-10-16T02:29:44.885Z · LW(p) · GW(p)
Possible post on suspicious multidimensional pessimism:
I think MIRI people (specifically Soares and Yudkowsky but probably others too) are more pessimistic than the alignment community average on several different dimensions, both technical and non-technical: morality, civilizational response, takeoff speeds, probability of easy alignment schemes working, and our ability to usefully expand the field of alignment. Some of this is implied by technical models, and MIRI is not more pessimistic in every possible dimension, but it's still awfully suspicious.
I strongly suspect that one of the following is true:
- the MIRI "optimism dial" is set too low
- everyone else's "optimism dial" is set too high. (Yudkowsky has said this multiple times in different contexts)
- There are common generators that I don't know about that are not just an "optimism dial", beyond MIRI's models
I'm only going to actually write this up if there is demand; the full post will have citations which are kind of annoying to find.
Replies from: thomas-kwa, sharmake-farah, hairyfigment, T3t, lahwran↑ comment by Thomas Kwa (thomas-kwa) · 2023-07-29T20:42:34.323Z · LW(p) · GW(p)
After working at MIRI (loosely advised by Nate Soares) for a while, I now have more nuanced views and also takes on Nate's research taste. It seems kind of annoying to write up so I probably won't do it unless prompted.
Edit: this is now up [LW · GW]
↑ comment by Alexander Gietelink Oldenziel (alexander-gietelink-oldenziel) · 2023-07-29T22:13:22.018Z · LW(p) · GW(p)
I would be genuinely curious to hear your more nuanced views and takes on Nate s research taste. This is really quite interesting to me and even a single paragraph would be valuable!
↑ comment by Noosphere89 (sharmake-farah) · 2022-10-16T15:57:41.588Z · LW(p) · GW(p)
I really want to see the post on multidimensional pessimism.
As for why, I'd argue 1 is happening.
For examples of 1, a good example of this is FOOM probabilities. I think MIRI hasn't updated on the evidence that FOOM is likely impossible for classical computers, and this ought to lower their probabilities to the chance that quantum/reversible computers appear.
Another good example is the emphasis on pivotal acts like "burn all GPUs." I think MIRI has too much probability mass on it being necessary, primarily because I think that they are biased by fiction, where problems must be solved by heroic acts, while in the real world more boring things are necessary. In other words, it's too exciting, which should be suspicious.
However that doesn't mean alignment is much easier. We can still fail, there's no rule that we make it through. It's that MIRI is systematically irrational here regarding doom probabilities or alignment.
Edit: I now think alignment is way, way easier than my past self, so I disendorse this sentence "However that doesn't mean alignment is much easier."
↑ comment by hairyfigment · 2022-10-16T06:28:47.782Z · LW(p) · GW(p)
What constitutes pessimism about morality, and why do you think that one fits Eliezer? He certainly appears more pessimistic across a broad area, and has hinted at concrete arguments for being so.
Replies from: thomas-kwa↑ comment by Thomas Kwa (thomas-kwa) · 2022-10-16T19:11:50.782Z · LW(p) · GW(p)
Value fragility / value complexity. How close do you need to get to human values to get 50% of the value of the universe, and how complicated must the representation be? Also in the past there was orthogonality, but that's now widely believed.
Replies from: Vladimir_Nesov, hairyfigment↑ comment by Vladimir_Nesov · 2022-10-16T22:51:48.977Z · LW(p) · GW(p)
I think the distance from human values or complexity of values is not a crux, as web/books corpus overdetermines them in great detail (for corrigibility purposes). It's mostly about alignment by default, whether human values in particular can be noticed in there, or if correctly specifying how to find them is much harder than finding some other deceptively human-value-shaped thing. If they can be found easily once there are tools to go looking for them at all, it doesn't matter how complex they are or how important it is to get everything right, that happens by default.
But also there is this pervasive assumption of it being possible to formulate values in closed form, as tractable finite data, which occasionally fuels arguments. Like, value is said to be complex, but of finite complexity. In an open environment, this doesn't need to be the case, a code/data distinction is only salient when we can make important conclusions by only looking at code and not at data. In an open environment, data is unbounded, can't be demonstrated all at once. So it doesn't make much sense to talk about complexity of values at all, without corrigibility alignment can't work out anyway.
↑ comment by RobertM (T3t) · 2022-10-16T05:01:35.933Z · LW(p) · GW(p)
I'd be very interested in a write-up, especially if you have receipts for pessimism which seems to be poorly calibrated, e.g. based on evidence contrary to prior predictions.
↑ comment by the gears to ascension (lahwran) · 2022-10-16T04:55:07.816Z · LW(p) · GW(p)
I think they pascals-mugged themselves and being able to prove they were wrong efficiently would be helpful
comment by Thomas Kwa (thomas-kwa) · 2024-11-08T02:45:03.405Z · LW(p) · GW(p)
What's the most important technical question in AI safety right now?
Replies from: Buck, sharmake-farah, nathan-helm-burger, LosPolloFowler, bogdan-ionut-cirstea, LiamLaw, rhollerith_dot_com↑ comment by Buck · 2024-11-08T19:03:46.648Z · LW(p) · GW(p)
In terms of developing better misalignment risk countermeasures, I think the most important questions are probably:
- How to evaluate whether models should be trusted or untrusted: currently I don't have a good answer and this is bottlenecking the efforts to write concrete control proposals.
- How AI control should interact with AI security tools inside labs.
More generally:
- How can we get more evidence on whether scheming is plausible?
- How scary is underelicitation? How much should the results about password-locked models [LW · GW] or arguments about being able to generate small numbers of high-quality labels or demonstrations [AF · GW] affect this?
↑ comment by Chris_Leong · 2024-11-13T06:11:11.571Z · LW(p) · GW(p)
"How can we get more evidence on whether scheming is plausible?" - What if we ran experiments where we included some pressure towards scheming (either RL or fine-tuning) and we attempted to determine the minimum such pressure required to cause scheming? We could further attempt to see how this interacts with scaling.
↑ comment by Noosphere89 (sharmake-farah) · 2024-11-08T17:44:41.712Z · LW(p) · GW(p)
I'd say 1 important question is whether the AI control strategy works out as they hope.
I agree with Bogdan that making adequate safety cases for automated safety research is probably one of the most important technical problems to answer (since conditional on the automating AI safety direction working out, then it could eclipse basically all safety research done prior to the automation, and this might hold even if LWers really had basically perfect epistemics given what's possible for humans, and picked closer to optimal directions, since labor is a huge bottleneck, and allows for much tighter feedback loops of progress, for the reasons Tamay Besiroglu identified):
↑ comment by Nathan Helm-Burger (nathan-helm-burger) · 2024-11-08T16:20:50.622Z · LW(p) · GW(p)
Here's some candidates:
1 Are we indeed (as I suspect) in a massive overhang of compute and data for powerful agentic AGI? (If so, then at any moment someone could stumble across an algorithmic improvement which would change everything overnight.)
2 Current frontier models seem much more powerful than mouse brains, yet mice seem conscious. This implies that either LLMs are already conscious, or could easily be made so with non-costly tweaks to their algorithm. How could we objectively tell if an AI were conscious?
3 Over the past year I've helped make both safe-evals-of-danger-adjacent-capabilities (e.g. WMDP.ai) and unpublished infohazardous-evals-of-actually-dangerous-capabilities. One of the most common pieces of negative feedback I've heard on the safe-evals is that they are only danger-adjacent, not measuring truly dangerous things. How could we safely show the correlation of capabilities between high performance on danger-adjacent evals with high performance on actually-dangerous evals?
Replies from: D0TheMath↑ comment by Garrett Baker (D0TheMath) · 2024-11-08T17:06:49.131Z · LW(p) · GW(p)
Are we indeed (as I suspect) in a massive overhang of compute and data for powerful agentic AGI? (If so, then at any moment someone could stumble across an algorithmic improvement which would change everything overnight.)
Why is this relevant for technical AI alignment (coming at this as someone skeptical about how relevant timeline considerations are more generally)?
Replies from: nathan-helm-burger↑ comment by Nathan Helm-Burger (nathan-helm-burger) · 2024-11-08T17:28:43.970Z · LW(p) · GW(p)
If tomorrow anyone in the world could cheaply and easily create an AGI which could act as a coherent agent on their behalf, and was based on an architecture different from a standard transformer.... Seems like this would change a lot of people's priorities about which questions were most urgent to answer.
Replies from: alexander-gietelink-oldenziel↑ comment by Alexander Gietelink Oldenziel (alexander-gietelink-oldenziel) · 2024-11-08T20:42:25.076Z · LW(p) · GW(p)
Fwiw I basically think you are right about the agentic AI overhang and obviously so. I do think it shapes how one thinks about what's most valuable in AI alignment.
Replies from: sharmake-farah↑ comment by Noosphere89 (sharmake-farah) · 2024-11-08T21:38:58.769Z · LW(p) · GW(p)
I kind of wished you both gave some reasoning as to why you believe that the agentic AI overhang/algorithmic overhang is likely, and I also wish that Nathan Helm Burger and Vladimir Nesov discussed this topic in a dialogue post.
Replies from: alexander-gietelink-oldenziel↑ comment by Alexander Gietelink Oldenziel (alexander-gietelink-oldenziel) · 2024-11-08T22:16:47.927Z · LW(p) · GW(p)
Glib formality: current LLMs do approximate something like a speed prior solomonoff inductor for internetdata but do not approximate AIXI.
There is a whole class of domains that are not tractably accesible from next-token prediction on human generated data. For instance, learning how to beat alphaGo with only access to pre2014 human go games.
Replies from: sharmake-farah↑ comment by Noosphere89 (sharmake-farah) · 2024-11-09T14:51:28.102Z · LW(p) · GW(p)
IMO, I think AlphaGo's success was orthogonal to AIXI, and more importantly, I expect AIXI to be very hard to approximate even as an approximatable ideal, so what's the use case for thinking future AIs will be AIXI-like?
I will also say that while I don't think pure LLMs will be just scaled forwards, just because there's a use for inference time compute scaling, I think that conditional on AGI and ASI being achieved, the strategy will look more iike using lots and lots of synthetic data to compensate for compute, whereas Solomonoff induction has a halting oracle with lots of compute, and can infer lots of things with the minimum data possible, while we will rely on a data-rich, compute poor strategy compared to approximate AIXI.
Replies from: alexander-gietelink-oldenziel↑ comment by Alexander Gietelink Oldenziel (alexander-gietelink-oldenziel) · 2024-11-09T16:03:05.535Z · LW(p) · GW(p)
The important thing is that both do active learning & decisionmaking & search, i.e. RL. *
LLMs don't do that. So the gain from doing that is huge.
Synthetic data is a bit of a weird word that get's thrown around a lot. There are fundamental limits on how much information resampling from the same data source will yield about completely different domains. So that seems a bit silly. Ofc sometimes with synthetic data people just mean doing rollouts, i.e. RL.
*the word RL sometimes gets mistaken for only very specific reinforcement learning algorithm. I mean here a very general class of algorithms that solve MDPs.
↑ comment by Stephen Fowler (LosPolloFowler) · 2024-11-09T02:38:17.297Z · LW(p) · GW(p)
The lack of a robust, highly general paradigm for reasoning about AGI models is the current greatest technical problem, although it is not what most people are working on.
What features of architecture of contemporary AI models will occur in future models that pose an existential risk?
What behavioral patterns of contemporary AI models will be shared with future models that pose an existential risk?
Is there a useful and general mathematical/physical framework that describes how agentic, macroscropic systems process information and interact with the environment?
Does terminology adopted by AI Safety researchers like "scheming", "inner alignment" or "agent" carve nature at the joints?
↑ comment by Bogdan Ionut Cirstea (bogdan-ionut-cirstea) · 2024-11-08T12:29:39.448Z · LW(p) · GW(p)
Something like a safety case for automated safety research (but I'm biased)
↑ comment by PhilosophicalSoul (LiamLaw) · 2024-11-08T11:14:42.813Z · LW(p) · GW(p)
Answering this from a legal perspective:
What is the easiest and most practical way to translate legalese into scientifically accurate terms, thus bridging the gap between AI experts and lawyers? Stated differently, how do we move from localised papers that only work in law or AI fields respectively, to papers that work in both?
↑ comment by RHollerith (rhollerith_dot_com) · 2024-11-13T06:53:29.776Z · LW(p) · GW(p)
Are Eliezer and Nate right that continuing the AI program will almost certainly lead to extinction or something approximately as disastrous as extinction?
comment by Thomas Kwa (thomas-kwa) · 2024-05-04T08:35:07.996Z · LW(p) · GW(p)
You should update by +-1% on AI doom surprisingly frequently
This is just a fact about how stochastic processes work. If your p(doom) is Brownian motion in 1% steps starting at 50% and stopping once it reaches 0 or 1, then there will be about 50^2=2500 steps of size 1%. This is a lot! If we get all the evidence for whether humanity survives or not uniformly over the next 10 years, then you should make a 1% update 4-5 times per week. In practice there won't be as many due to heavy-tailedness in the distribution concentrating the updates in fewer events, and the fact you don't start at 50%. But I do believe that evidence is coming in every week such that ideal market prices should move by 1% on maybe half of weeks, and it is not crazy for your probabilities to shift by 1% during many weeks if you think about it often enough. [Edit: I'm not claiming that you should try to make more 1% updates, just that if you're calibrated and think about AI enough, your forecast graph will tend to have lots of >=1% week-to-week changes.]
Replies from: LawChan, Seth Herd, niplav, alexander-gietelink-oldenziel, Dagon, p.b., JBlack, TsviBT↑ comment by LawrenceC (LawChan) · 2024-05-04T15:58:08.205Z · LW(p) · GW(p)
The general version of this statement is something like: if your beliefs satisfy the law of total expectation, the variance of the whole process should equal the variance of all the increments involved in the process.[1] In the case of the random walk where at each step, your beliefs go up or down by 1% starting from 50% until you hit 100% or 0% -- the variance of each increment is 0.01^2 = 0.0001, and the variance of the entire process is 0.5^2 = 0.25, hence you need 0.25/0.0001 = 2500 steps in expectation. If your beliefs have probability p of going up or down by 1% at each step, and 1-p of staying the same, the variance is reduced by a factor of p, and so you need 2500/p steps.
(Indeed, something like this standard way to derive the expected steps before a random walk hits an absorbing barrier).
Similarly, you get that if you start at 20% or 80%, you need 1600 steps in expectation, and if you start at 1% or 99%, you'll need 99 steps in expectation.
One problem with your reasoning above is that as the 1%/99% shows, needing 99 steps in expectation does not mean you will take 99 steps with high probability -- in this case, there's a 50% chance you need only one update before you're certain (!), there's just a tail of very long sequences. In general, the expected value of variables need not look like
I also think you're underrating how much the math changes when your beliefs do not come in the form of uniform updates. In the most extreme case, suppose your current 50% doom number comes from imagining that doom is uniformly distributed over the next 10 years, and zero after -- then the median update size per week is only 0.5/520 ~= 0.096%/week, and the expected number of weeks with a >1% update is 0.5 (it only happens when you observe doom). Even if we buy a time-invariant random walk model of belief updating, as the expected size of your updates get larger, you also expect there to be quadratically fewer of them -- e.g. if your updates came in increments of size 0.1 instead of 0.01, you'd expect only 25 such updates!
Applying stochastic process-style reasoning to beliefs is empirically very tricky, and results can vary a lot based on seemingly reasonable assumptions. E.g. I remember Taleb making a bunch of mathematically sophisticated arguments[2] that began with "Let your beliefs take the form of a Wiener process[3]" and then ending with an absurd conclusion, such as that 538's forecasts are obviously wrong because their updates aren't Gaussian distributed or aren't around 50% until immediately before the elction date. And famously, reasoning of this kind has often been an absolute terrible idea in financial markets. So I'm pretty skeptical of claims of this kind in general.
- ^
There's some regularity conditions here, but calibrated beliefs that things you eventually learn the truth/falsity of should satisfy these by default.
- ^
Often in an attempt to Euler people who do forecasting work but aren't super mathematical, like Philip Tetlock.
- ^
This is what happens when you take the limit of the discrete time random walk, as you allow for updates on ever smaller time increments. You get Gaussian distributed increments per unit time -- W_t+u - W_t ~ N(0, u) -- and since the tail of your updates is very thin, you continue to get qualitatively similar results to your discrete-time random walk model above.
And yes, it is ironic that Taleb, who correctly points out the folly of normality assumptions repeatedly, often defaults to making normality assumptions in his own work.
↑ comment by Thomas Kwa (thomas-kwa) · 2024-05-05T06:41:58.976Z · LW(p) · GW(p)
I talked about this with Lawrence, and we both agree on the following:
- There are mathematical models under which you should update >=1% in most weeks, and models under which you don't.
- Brownian motion gives you 1% updates in most weeks. In many variants, like stationary processes with skew, stationary processes with moderately heavy tails, or Brownian motion interspersed with big 10%-update events that constitute <50% of your variance, you still have many weeks with 1% updates. Lawrence's model where you have no evidence until either AI takeover happens or 10 years passes does not give you 1% updates in most weeks, but this model is almost never the case for sufficiently smart agents.
- Superforecasters empirically make lots of little updates, and rounding off their probabilities to larger infrequent updates make their forecasts on near-term problems worse.
- Thomas thinks that AI is the kind of thing where you can make lots of reasonable small updates frequently. Lawrence is unsure if this is the state that most people should be in, but it seems plausibly true for some people who learn a lot of new things about AI in the average week (especially if you're very good at forecasting).
- In practice, humans often update in larger discrete chunks. Part of this is because they only consciously think about new information required to generate new numbers once in a while, and part of this is because humans have emotional fluctuations which we don't include in our reported p(doom).
- Making 1% updates in most weeks is not always just irrational emotional fluctuations; it is consistent with how a rational agent would behave under reasonable assumptions. However, we do not recommend that people consciously try to make 1% updates every week, because fixating on individual news articles is not the right way to think about forecasting questions, and it is empirically better to just think about the problem directly rather than obsessing about how many updates you're making.
↑ comment by niplav · 2024-05-04T22:00:47.224Z · LW(p) · GW(p)
Thank you a lot for this. I think this or @Thomas Kwa [LW · GW]s comment would make an excellent original-sequences-style post—it doesn't need to be long, but just going through an example and talking about the assumptions would be really valuable for applied rationality.
After all, it's about how much one should expect ones beliefs to vary, which is pretty important.
↑ comment by Seth Herd · 2024-05-04T13:20:58.222Z · LW(p) · GW(p)
But... Why would p(doom) move like Brownian motion until stopping at 0 or 1?
I don't disagree with your conclusions, there's a lot of evidence coming in, and if you're spending full time or even part time thinking about alignment, a lot of important updates on the inference. But assuming a random walk seems wrong.
Is there a reason that a complex, structured unfolding of reality would look like a random walk?
Replies from: niplav↑ comment by niplav · 2024-05-04T22:03:54.465Z · LW(p) · GW(p)
Because[1] for a Bayesian reasoner, there is conservation [LW · GW] of [? · GW] expected evidence [LW · GW].
Although I've seen it mentioned that technically the change in the belief on a Bayesian should follow a Martingale, and Brownian motion is a martingale.
I'm not super technically strong on this particular part of the math. Intuitively it could be that in a bounded reasoner which can only evaluate programs in , any pattern in its beliefs that can be described by an algorithm in is detected and the predicted future belief from that pattern is incorporated into current beliefs. On the other hand, any pattern described by an algorithm in can't be in the class of hypotheses of the agent, including hypotheses about its own beliefs, so patterns persist. ↩︎
↑ comment by LawrenceC (LawChan) · 2024-05-05T18:29:32.561Z · LW(p) · GW(p)
Technically, the probability assigned to a hypothesis over time should be the martingale (i.e. have expected change zero); this is just a restatement of the conservation of expected evidence/law of total expectation.
The random walk model that Thomas proposes is a simple model that illustrates a more general fact. For a martingale, the variance of is equal to the sum of variances of the individual timestep changes (and setting ): . Under this frame, insofar as small updates contribute a large amount to the variance of each update , then the contribution to the small updates to the credences must also be large (which in turn means you need to have a lot of them in expectation[1]).
Note that this does not require any strong assumption besides that the the distribution of likely updates is such that the small updates contribute substantially to the variance. If the structure of the problem you're trying to address allows for enough small updates (relative to large ones) at each timestep, then it must allow for "enough" of these small updates in the sequence, in expectation.
While the specific +1/-1 random walk he picks is probably not what most realistic credences over time actually look like, playing around with it still helps give a sense of what exactly "conservation of expected evidence" might look/feel like. (In fact, in the dath ilan of Swimmer's medical dath ilan glowfics, people do use a binary random walk to illustrate how calibrated beliefs typically evolve over time.)
Now, in terms of if it's reasonable to model beliefs as Brownian motion (in the standard mathematical sense, not in the colloquial sense): if you suppose that there are many, many tiny independent additive updates to your credence in a hypothesis, your credence over time "should" look like Brownian motion at a large enough scale (again in the standard mathematical sense), for similar reasons as to why the sum of a bunch of independent random variables converges to a Gaussian. This doesn't imply that your belief in practice should always look like Brownian motion, any more than the CLT implies that real world observables are always Gaussian. But again, the claim Thomas makes carries thorough
I also make the following analogy in my head: Bernouli:Gaussian ~= Simple Random Walk:Brownian Motion, which I found somewhat helpful. Things irl are rarely independent/time-invarying Bernoulli or Gaussian processes, but they're mathematically convenient to work with, and are often 'good enough' for deriving qualitative insights.
- ^
Note that you need to apply something like the optional stopping theorem to go from the case of for fixed to the case of where is the time you reach 0 or 1 credence and the updates stop.
↑ comment by Seth Herd · 2024-05-05T15:29:09.470Z · LW(p) · GW(p)
I get conservation of expected evidence. But the distribution of belief changes is completely unconstrained.
Going from the class martingale to the subclass Brownian motion is arbitrary, and the choice of 1% update steps is another unjustified arbitrary choice.
I think asking about the likely possible evidence paths would improve our predictions.
You spelled it conversation of expected evidence. I was hoping there was another term by that name :)
Replies from: LawChan, niplav↑ comment by LawrenceC (LawChan) · 2024-05-05T18:37:48.156Z · LW(p) · GW(p)
To be honest, I would've preferred if Thomas's post started from empirical evidence (e.g. it sure seems like superforecasters and markets change a lot week on week) and then explained it in terms of the random walk/Brownian motion setup. I think the specific math details (a lot of which don't affect the qualitative result of "you do lots and lots of little updates, if there exists lots of evidence that might update you a little") are a distraction from the qualitative takeaway.
A fancier way of putting it is: the math of "your belief should satisfy conservation of expected evidence" is a description of how the beliefs of an efficient and calibrated agent should look, and examples like his suggest it's quite reasonable for these agents to do a lot of updating. But the example is not by itself necessarily a prescription for how your belief updating should feel like from the inside (as a human who is far from efficient or perfectly calibrated). I find the empirical questions of "does the math seem to apply in practice" and "therefore, should you try to update more often" (e.g., what do the best forecasters seem to do?) to be larger and more interesting than the "a priori, is this a 100% correct model" question.
↑ comment by niplav · 2024-05-04T10:27:07.946Z · LW(p) · GW(p)
Thank you a lot! Strong upvoted.
I was wondering a while ago whether Bayesianism says anything about how much my probabilities are "allowed" to oscillate around—I was noticing that my probability of doom was often moving by 5% in the span of 1-3 weeks, though I guess this was mainly due to logical uncertainty and not empirical uncertainty.
Since there are 10 5% steps between 50% and 0 or 1, and for ~10 years, I should expect to make these kinds of updates ~100 times, or 10 times a year, or a little bit less than once a month, right? So I'm currently updating "too much".
↑ comment by Alexander Gietelink Oldenziel (alexander-gietelink-oldenziel) · 2024-05-04T08:42:06.431Z · LW(p) · GW(p)
Interesting...
Wouldn't I expect the evidence to come out in a few big chunks, e.g. OpenAI releasing a new product?
Replies from: thomas-kwa↑ comment by Thomas Kwa (thomas-kwa) · 2024-05-04T10:49:20.890Z · LW(p) · GW(p)
To some degree yes, but I expect lots of information to be spread out across time. For example: OpenAI releases GPT5 benchmark results. Then a couple weeks later they deploy it on ChatGPT and we can see how subjectively impressive it is out of the box, and whether it is obviously pursuing misaligned goals. Over the next few weeks people develop post-training enhancements like scaffolding, and we get a better sense of its true capabilities. Over the next few months, debate researchers study whether GPT4-judged GPT5 debates reliably produce truth, and control researchers study whether GPT4 can detect whether GPT5 is scheming. A year later an open-weights model of similar capability is released and the interp researchers check how understandable it is and whether SAEs still train.
↑ comment by Dagon · 2024-05-04T16:53:40.571Z · LW(p) · GW(p)
I think this leans a lot on "get evidence uniformly over the next 10 years" and "Brownian motion in 1% steps". By conservation of expected evidence, I can't predict the mean direction of future evidence, but I can have some probabilities over distributions which add up to 0.
For long-term aggregate predictions of event-or-not (those which will be resolved at least a few years away, with many causal paths possible), the most likely updates are a steady reduction as the resolution date gets closer, AND random fairly large positive updates as we learn of things which make the event more likely.
↑ comment by JBlack · 2024-05-05T05:40:42.573Z · LW(p) · GW(p)
It definitely should not move by anything like a Brownian motion process. At the very least it should be bursty and updates should be expected to be very non-uniform in magnitude.
In practice, you should not consciously update very often since almost all updates will be of insignificant magnitude on near-irrelevant information. I expect that much of the credence weight turns on unknown unknowns, which can't really be updated on at all until something turns them into (at least) known unknowns.
But sure, if you were a superintelligence with practically unbounded rationality then you might in principle update very frequently.
Replies from: thomas-kwa↑ comment by Thomas Kwa (thomas-kwa) · 2024-05-05T07:20:54.047Z · LW(p) · GW(p)
The Brownian motion assumption is rather strong but not required for the conclusion. Consider the stock market, which famously has heavy-tailed, bursty returns. It happens all the time for the S&P 500 to move 1% in a week, but a 10% move in a week only happens a couple of times per decade. I would guess (and we can check) that most weeks have >0.6x of the average per-week variance of the market, which causes the median weekly absolute return to be well over half of what it would be if the market were Brownian motion with the same long-term variance.
Also, Lawrence tells me that in Tetlock's studies, superforecasters tend to make updates of 1-2% every week, which actually improves their accuracy.
↑ comment by TsviBT · 2024-05-05T02:26:08.361Z · LW(p) · GW(p)
Probabilities on summary events like this are mostly pretty pointless. You're throwing together a bunch of different questions, about which you have very different knowledge states (including how much and how often you should update about them).
comment by Thomas Kwa (thomas-kwa) · 2022-07-30T20:07:52.131Z · LW(p) · GW(p)
Maybe this is too tired a point, but AI safety really needs exercises-- tasks that are interesting, self-contained (not depending on 50 hours of readings), take about 2 hours, have clean solutions, and give people the feel of alignment research.
I found some of the SERI MATS application questions better than Richard Ngo's exercises [AF · GW] for this purpose, but there still seems to be significant room for improvement. There is currently nothing smaller than ELK (which takes closer to 50 hours to develop a proposal for and properly think about it) that I can point technically minded people to and feel confident that they'll both be engaged and learn something.
Replies from: ricraz, Viliam↑ comment by Richard_Ngo (ricraz) · 2022-10-20T01:34:34.830Z · LW(p) · GW(p)
If you let me know the specific MATS application questions you like, I'll probably add them to my exercises.
(And if you let me know the specific exercises of mine you don't like, I'll probably remove them.)
↑ comment by Viliam · 2022-08-05T11:04:04.655Z · LW(p) · GW(p)
Not sure if this is what you want, but I can imagine an exercise in Goodharting. You are given the criteria for a reward and the thing they were supposed to maximize, your task is to figure out the (least unlikely) way to score very high on the criteria without doing to well on the intended target.
For example: Goal = make the people in the call center more productive. Measure = your salary depends on how many phone calls you handle each day. Intended behavior = picking up the phone quickly, trying to solve the problems quickly. Actual behavior = "accidentally" dropping phone calls after a few seconds so that the customer has to call you again (and that counts by the metric as two phone calls answered).
Another example: Goal = make the software developers more productive. Measure 1 = number of lines of code written. Measure 2 = number of bugs fixed.
I am proposing this because it seems to me that from a 30000 foot view, a big part of AI alignment is how to avoid Goodharting. ("Goal = create a happy and prosperous future for humanity. Measure = something that sounds very smart and scientific. Actual behavior = universe converted to paperclips, GDP successfully maximized.")
comment by Thomas Kwa (thomas-kwa) · 2024-04-03T20:56:08.959Z · LW(p) · GW(p)
Tech tree for worst-case/HRAD alignment
Here's a diagram of what it would take to solve alignment in the hardest worlds, where something like MIRI's HRAD agenda is needed. I made this months ago with Thomas Larsen and never got around to posting it (mostly because under my worldview it's pretty unlikely that we can, or need to, do this), and it probably won't become a longform at this point. I have not thought about this enough to be highly confident in anything.
- This flowchart is under the hypothesis that LLMs have some underlying, mysterious algorithms and data structures that confer intelligence, and that we can in theory apply these to agents constructed by hand, though this would be extremely tedious. Therefore, there are basically three phases: understanding what a HRAD agent would do in theory, reverse-engineering language models, and combining these two directions. The final agent will be a mix of hardcoded things and ML, depending on what is feasible to hardcode and how well we can train ML systems whose robustness and conformation to a spec we are highly confident in.
- Theory of abstractions: Also called multi-level models. A mathematical framework for a world-model that contains nodes at different levels of abstraction, such that one can represent concepts like “diamond” and “atom” while respecting consistency between different levels, and be robust to ontology shifts
- WM inference = inference on a world-model for an embedded agent, may run in like double exponential time so long as it's computable
↑ comment by Lucius Bushnaq (Lblack) · 2024-07-29T21:45:41.628Z · LW(p) · GW(p)
Difference between my model and this flow-chart: I'm hoping that the top branches are actually downstream of LLM reverse-engineering. LLMs do abstract reasoning already, so if you can reverse engineer LLMs, maybe that lets you understand how abstract reasoning works much faster than deriving it yourself.
comment by Thomas Kwa (thomas-kwa) · 2023-04-25T04:12:32.439Z · LW(p) · GW(p)
I'm worried that "pause all AI development" is like the "defund the police" of the alignment community. I'm not convinced it's net bad because I haven't been following governance-- my current guess is neutral-- but I do see these similarities:
- It's incredibly difficult and incentive-incompatible with existing groups in power
- There are less costly, more effective steps to reduce the underlying problem, like making the field of alignment 10x larger or passing regulation to require evals
- There are some obvious negative effects; potential overhangs or greater incentives to defect in the AI case, and increased crime, including against disadvantaged groups, in the police case
- There's far more discussion than action (I'm not counting the fact that GPT5 isn't being trained yet; that's for other reasons)
- It's memetically fit, and much discussion is driven by two factors that don't advantage good policies over bad policies, and might even do the reverse. This is the toxoplasma of rage.
- disagreement with the policy
- (speculatively) intragroup signaling; showing your dedication to even an inefficient policy proposal proves you're part of the ingroup. I'm not 100% this was a large factor in "defund the police" and this seems even less true with the FLI letter, but still worth mentioning.
This seems like a potentially unpopular take, so I'll list some cruxes. I'd change my mind and endorse the letter if some of the following are true.
- The claims above are mistaken/false somehow.
- Top labs actually start taking beneficial actions towards the letter's aims
- It's caused people to start thinking more carefully about AI risk
- A 6 month pause now is especially important by setting anti-racing norms, demonstrating how far AI alignment is lagging behind capabilities, or something
- A 6 month pause now is worth close to 6 months of alignment research at crunch time (my guess is that research at crunch time is worth 1.5x-3x more depending on whether MIRI is right about everything)
- The most important quality to push towards in public discourse is how much we care about safety at all, so I should endorse this proposal even though it's flawed
↑ comment by Ben Pace (Benito) · 2023-04-25T06:23:35.502Z · LW(p) · GW(p)
The obvious dis-analogy is that if the police had no funding and largely ceased to exist, a string of horrendous things would quickly occur. Murders and thefts and kidnappings and rapes and more would occur throughout every country in which it was occurring, people would revert to tight-knit groups who had weapons to defend themselves, a lot of basic infrastructure would probably break down (e.g. would Amazon be able to pivot to get their drivers armed guards?) and much more chaos would ensue.
And if AI research paused, society would continue to basically function as it has been doing so far.
One of them seems to me like a goal that directly causes catastrophes and a breakdown of society and the other doesn't.
Replies from: thomas-kwa↑ comment by Thomas Kwa (thomas-kwa) · 2023-04-25T17:56:34.021Z · LW(p) · GW(p)
Fair point. Another difference is that the pause is popular! 66-69% in favor of the pause, and 41% think AI would do more harm than good vs 9% for more good than harm.
↑ comment by Lauro Langosco · 2023-06-16T23:11:53.594Z · LW(p) · GW(p)
There are less costly, more effective steps to reduce the underlying problem, like making the field of alignment 10x larger or passing regulation to require evals
IMO making the field of alignment 10x larger or evals do not solve a big part of the problem, while indefinitely pausing AI development would. I agree it's much harder, but I think it's good to at least try, as long as it doesn't terribly hurt less ambitious efforts (which I think it doesn't).
↑ comment by quetzal_rainbow · 2023-04-25T18:38:41.562Z · LW(p) · GW(p)
There are less costly, more effective steps to reduce the underlying problem, like making the field of alignment 10x larger or passing regulation to require evals
This statement begs for cost-benefit analysis.
Increasing size of alignment field can be efficient, but it won't be cheap. You need to teach new experts in the field that doesn't have any polised standardized educational programs and doesn't have much of teachers. If you want not only increase number of participants in the field, but increase productivity of the field 10x, you need an extraordinary educational effort.
Passing regulation to require evals seems like a meh idea. Nobody knows in enough details how to make such evalutions and every wrong idea that makes its way to law will be here until the end of the world.
Replies from: thomas-kwa↑ comment by Thomas Kwa (thomas-kwa) · 2023-04-25T20:52:22.926Z · LW(p) · GW(p)
I'd be much happier with increasing participants enough to equal 10-20% of the field of ML than a 6 month unconditional pause, and my guess is it's less costly. It seems like leading labs allowing other labs to catch up by 6 months will reduce their valuations more than 20%, whereas diverting 10-20% of their resources would reduce valuations only 10% or so.
There are currently 300 alignment researchers. If we take additional researchers from the pool of 30k people who attended ICML, you get 3000 researchers, and if they're equal quality this is 10x participants. I wouldn't expect alignment to go 10x faster, more like 2x with a decent educational effort. But this is in perpetuity and should speed up alignment by far more than 6 months. There's the question of getting labs to pay if they're creating most of the harms, which might be hard though.
I'd be excited about someone doing a real cost-benefit analysis here, or preferably coming up with better ideas. It just seems so unlikely that a 6 month pause is close to the most efficient thing, given it destroys much of the value of a company that has a large lead.
↑ comment by Thomas Kwa (thomas-kwa) · 2024-04-10T06:23:47.132Z · LW(p) · GW(p)
I now think the majority of impact of AI pause advocacy will come from the radical flank effect, and people should study it to decide whether pause advocacy is good or bad.
↑ comment by TurnTrout · 2023-05-02T05:21:19.675Z · LW(p) · GW(p)
It's incredibly difficult and incentive-incompatible with existing groups in power
Why does this have to be true? Can't governments just compensate existing AGI labs for the expected commercial value of their foregone future advances due to indefinite pause?
Replies from: thomas-kwa↑ comment by Thomas Kwa (thomas-kwa) · 2023-05-04T20:37:57.862Z · LW(p) · GW(p)
This seems good if it could be done. But the original proposal was just a call for labs to individually pause their research, which seems really unlikely to work.
Also, the level of civilizational competence required to compensate labs seems to be higher than for other solutions. I don't think it's a common regulatory practice to compensate existing labs like this, and it seems difficult to work out all the details so that labs will feel adequately compensated. Plus there might be labs that irrationally believe they're undervalued. Regulations similar to the nuclear or aviation industry feel like a more plausible way to get slowdown, and have the benefit that they actually incentivize safety work.
comment by Thomas Kwa (thomas-kwa) · 2020-06-13T04:32:57.946Z · LW(p) · GW(p)
Say I need to publish an anonymous essay. If it's long enough, people could plausibly deduce my authorship based on the writing style; this is called stylometry. The only stylometry-defeating tool I can find is Anonymouth; it hasn't been updated in 7 years and it's unclear if it can defeat modern AI. Is there something better?
Replies from: Linch↑ comment by Linch · 2022-10-19T06:16:43.804Z · LW(p) · GW(p)
Are LLMs advanced enough now that you can just ask GPT-N to do style transfer?
Replies from: tao-lin↑ comment by Tao Lin (tao-lin) · 2023-04-26T17:27:06.393Z · LW(p) · GW(p)
if I were doing this, I'd use gpt-4 to translate it into the style of a specific person, preferably a deceased public figure, then edit the result. I'd guess GPTs are better at translating to a specific style than removing style
comment by Thomas Kwa (thomas-kwa) · 2025-02-26T09:23:40.317Z · LW(p) · GW(p)
Will we ever have Poké Balls in real life? How fast could they be at storing and retrieving animals? Requirements:
- Made of atoms, no teleportation or fantasy physics.
- Small enough to be easily thrown, say under 5 inches diameter
- Must be able to disassemble and reconstruct an animal as large as an elephant in a reasonable amount of time, say 5 minutes, and store its pattern digitally
- Must reconstruct the animal to enough fidelity that its memories are intact and it's physically identical for most purposes, though maybe not quite to the cellular level
- No external power source
- Works basically wherever you throw it, though it might be slower to print the animal if it only has air to use as feedstock mass or can't spread out to dissipate heat
- Should not destroy nearby buildings when used
- Animals must feel no pain during the process
It feels pretty likely to me that we'll be able to print complex animals eventually using nanotech/biotech, but the speed requirements here might be pushing the limits of what's possible. In particular heat dissipation seems like a huge challenge; assuming that 0.2 kcal/g of waste heat is created while assembling the elephant, which is well below what elephants need to build their tissues, you would need to dissipate about 5 GJ of heat, which would take even a full-sized nuclear power plant cooling tower a few seconds. Power might be another challenge. Drexler claims you can eat fuel and oxidizer, turn all the mass into basically any lower-energy state, and come out easily net positive on energy. But if there is none available you would need a nuclear reactor.
Replies from: Gurkenglas↑ comment by Gurkenglas · 2025-02-26T12:55:10.524Z · LW(p) · GW(p)
All we need to create is a Ditto. A blob of nanotech wouldn't need 5 seconds to take the shape of the surface of an elephant and start mimicing its behavior; is it good enough to optionally do the infilling later if it's convenient?
comment by Thomas Kwa (thomas-kwa) · 2024-04-04T08:02:08.351Z · LW(p) · GW(p)
I was going to write an April Fool's Day post in the style of "On the Impossibility of Supersized Machines", perhaps titled "On the Impossibility of Operating Supersized Machines", to poke fun at bad arguments that alignment is difficult. I didn't do this partly because I thought it would get downvotes. Maybe this reflects poorly on LW?
Replies from: Dagon, valley9, kave, Algon, ronny-fernandez↑ comment by Dagon · 2024-04-04T19:29:32.654Z · LW(p) · GW(p)
Nice try! You almost got me to speculate why downvoting happens for something I didn't see and didn't downvote.
Honestly, THIS would have been a great April Fool's (or perhaps Fools') day sentiment: claiming that hypothetical downvotes for an unwritten satirical post on a joke day reflect poorly on LW.
↑ comment by Ebenezer Dukakis (valley9) · 2024-04-06T09:17:54.664Z · LW(p) · GW(p)
The older get and the more I use the internet, the more skeptical I become of downvoting.
Reddit is the only major social media site that has downvoting, and reddit is also (in my view) the social media site with the biggest groupthink problem. People really seem to dislike being downvoted, which causes them to cluster in subreddits full of the like-minded, taking potshots at those who disagree instead of having a dialogue. Reddit started out as one the most intelligent sites on the internet due to its programmer-discussion origins; the decline has been fairly remarkable IMO. Especially when it comes to any sort of controversial or morality-related dialogue, reddit commenters seem to be participating in a Keynesian beauty contest more than they are thinking.
When I look at the stuff that other people downvote, their downvotes often seem arbitrary and capricious. (It can be hard to separate out my independent opinion of the content from my downvotes-colored opinion so I can notice this.) When I get the impulse to downvote something, it's usually not the best side of me that's coming out. And yet getting downvoted still aggravates me a lot. My creativity and enthusiasm are noticeably diminished for perhaps 24-48 hours afterwards. Getting downvoted doesn't teach me anything beyond just "don't engage with those people", often with an added helping of "screw them".
We have good enough content-filtering mechanisms nowadays that in principle, I don't think people should be punished for posting "bad" content. It should be easy to arrange things so "good" content gets the lion's share of the attention.
I'd argue the threat of punishment is most valuable when people can clearly predict what's going to produce punishment, e.g. committing a crime. For getting downvoted, the punishment is arbitrary enough that it causes a big behavioral no-go zone.
The problem isn't that people might downvote your satire. The problem is that human psychology is such that even an estimated 5% chance of your satire being downvoted is enough to deter you from posting it, since in the ancestral environment social exclusion was asymmetrically deadly relative to social acceptance. Conformity is the natural result.
Specific proposals:
-
Remove the downvote button, and when the user hits "submit" on their post or comment, an LLM reads the post or comment and checks it against a long list of site guidelines. The LLM flags potential issues to the user, and says: "You can still post this if you want, but since it violates 3 of the guidelines, it will start out with a score of -3. Alternatively, you can rewrite it and submit it to me again." That gets you quality control without the capricious-social-exclusion aspect.
-
Have specific sections of the site, or specific times of the year, where the voting gets turned off. Or keep the voting on, but anonymize the post score and the user who posted it, so your opinion isn't colored by the content's current score / user reputation.
This has been a bit of a rant, but here are a couple of links to help point at what I'm trying to say:
-
https://vimeo.com/60898177 -- this Onion satire was made over a decade ago. I think it's worth noting how absurd our internet-of-ubiquitous-feedback-mechanisms seems from the perspective of comedians from the past. (And it is in fact absurd in my view, but it can be hard to see the water you're swimming in. Browsing an old-school forum without any feedback mechanisms makes the difference seem especially stark. The analogy that's coming to mind is a party where everyone's on cocaine, vs a party where everyone is sober.)
-
https://celandine13.livejournal.com/33599.html -- classic post, "Errors vs. Bugs and the End of Stupidity"
↑ comment by mako yass (MakoYass) · 2024-04-07T02:31:11.219Z · LW(p) · GW(p)
You may be interested in Kenneth Stanley's serendipity-oriented social network, maven
↑ comment by Ronny Fernandez (ronny-fernandez) · 2024-04-08T17:08:02.602Z · LW(p) · GW(p)
I think you should still write it. I'd be happy to post it instead or bet with you on whether it ends up negative karma if you let me read it first.
comment by Thomas Kwa (thomas-kwa) · 2023-11-04T04:57:41.977Z · LW(p) · GW(p)
The independent-steps model of cognitive power
A toy model of intelligence implies that there's an intelligence threshold above which minds don't get stuck when they try to solve arbitrarily long/difficult problems, and below which they do get stuck. I might not write this up otherwise due to limited relevance, so here it is as a shortform, without the proofs, limitations, and discussion.
The model
A task of difficulty n is composed of independent and serial subtasks. For each subtask, a mind of cognitive power knows different “approaches” to choose from. The time taken by each approach is at least 1 but drawn from a power law, for , and the mind always chooses the fastest approach it knows. So the time taken on a subtask is the minimum of samples from the power law, and the overall time for a task is the total for the n subtasks.
Main question: For a mind of strength ,
- what is the average rate at which it completes tasks of difficulty n?
- will it be infeasible for it to complete sufficiently large tasks?
Results
- There is a critical threshold of intelligence below which the distribution of time to complete a subtask has infinite mean. This threshold depends on .
- This implies that for an n-step task, the median of average time-per-subtask grows without bound as n increases. So (for minds below the critical threshold) the median time to complete a whole task grows superlinearly with n.
- Above the critical threshold, minds can solve any task in expected linear time.
- Some distance above the critical threshold, minds are running fairly close to the optimal speed, and further increases in Q cause small efficiency gains.
- I think this doesn't depend on the function being a power law; it would be true for many different heavy-tailed distributions, but the math wouldn't be as nice.
↑ comment by Alexander Gietelink Oldenziel (alexander-gietelink-oldenziel) · 2023-11-04T07:51:45.754Z · LW(p) · GW(p)
Nice if it is a general feature of heavy-tailed distributions. Why do we expect tasks to be heavy tailed? It has some intuitive force certainly. Do you know of a formal argument?
Replies from: thomas-kwa↑ comment by Thomas Kwa (thomas-kwa) · 2023-11-04T08:31:35.678Z · LW(p) · GW(p)
The time to complete a task using a certain approach should be heavy-tailed because most approaches don't work or are extremely impractical compared to the best ones. Suppose you're trying to write an O(n log n) sorting algorithm. Mergesort is maybe easy to think of, heapsort would require you to invent heaps and take maybe 10x more time, and most ideas out of the space of all possible ideas don't work at all. So the time for different approaches definitely spans many orders of magnitude.
The speed at which humans can do various cognitive subtasks also differs by orders of magnitude. Grandmasters can play chess >1000 times faster at equal skill level than lesser players, as evidenced by simuls. Filling in a clue in a crossword sometimes takes me 1 second but other times might take me an hour or longer if I didn't give up first.
comment by Thomas Kwa (thomas-kwa) · 2023-09-24T02:14:44.363Z · LW(p) · GW(p)
I'm looking for AI safety projects with people with some amount of experience. I have 3/4 of a CS degree from Caltech, one year at MIRI, and have finished the WMLB and ARENA bootcamps. I'm most excited about making activation engineering more rigorous, but willing to do anything that builds research and engineering skill.
If you've published 2 papers in top ML conferences or have a PhD in something CS related, and are interested in working with me, send me a DM.
Replies from: jacques-thibodeau↑ comment by jacquesthibs (jacques-thibodeau) · 2023-09-27T22:20:48.616Z · LW(p) · GW(p)
I’m not the person you are looking for, but I think it’s a great idea to put this out there and try to find collaborators, especially in the case of independent researchers. I’ll be actively trying to do the same.
I’m often reminded of a productivity tip by Spencer Greenberg:
4. Involve other people in projects in such a way that they rely on you to get your parts done.
For me personally, this is probably the most powerful technique I've ever discovered for getting work done efficiently. When I know someone needs something by 2pm, and will be waiting if I don't get it to them on time, I find it very powerfully motivating. Not everyone finds this as useful as I do, however.
From what I remember, he has said that he basically never starts a project on his own.
Using each other's strengths and cross-pollination of ideas is obviously a good idea, too.
I’m curious if a database for this would increase the likelihood of people partnering up.
comment by Thomas Kwa (thomas-kwa) · 2022-04-27T07:14:02.898Z · LW(p) · GW(p)
I had a long-ish conversation with John Wentworth and want to make it known that I could probably write up any of the following distillations if I invested lots of time into them (about a day (edit: 3 days seems more likely) of my time and an hour of John's). Reply if you're really interested in one of them.
- What is the type signature of a utility function?
- Utility functions must be defined with respect to an external world-model
- Infinite money-pumps are not required for incoherence, and not likely in practice. The actual incoherent behavior is that an agent could get to states A_1 or A_2, identical except that A_1 has more money, and chooses A_2. Implications.
- Why VNM is not really a coherence theorem. Other coherence theorems relating to EU maximization simultaneously derive Bayesian probabilities and utilities. VNM requires an external frequentist notion of probabilities.
↑ comment by Ben Pace (Benito) · 2022-04-27T08:43:54.965Z · LW(p) · GW(p)
I wish we had polling. Anyway if you made four individual comments, one for each, I’d weak upvote the first and last.
↑ comment by Dagon · 2022-04-27T14:45:16.143Z · LW(p) · GW(p)
1 and 2 are the same writeup, I think. Utility function maps contingent future universe-state to a preference ranking (ordinal or cardinal, depending). This requires a world-model because the mentally-projected future states under consideration are always and only results of one's models.
If you/he are just saying that money pumps are just one way to show incoherence, but not the only way, I'd enjoy a writeup of other ways.
I'd also enjoy a writeup of #4 - I'm curious if it's just a directionality argument (VNM assumes coherence, rather than being about it), or if there's more subtle differences.
comment by Thomas Kwa (thomas-kwa) · 2024-01-12T20:17:56.839Z · LW(p) · GW(p)
The LessWrong Review's short review period is a fatal flaw.
I would spend WAY more effort on the LW review if the review period were much longer. It has happened about 10 times in the last year that I was really inspired to write a review for some post, but it wasn’t review season. This happens when I have just thought about a post a lot for work or some other reason, and the review quality is much higher because I can directly observe how the post has shaped my thoughts. Now I’m busy with MATS and just don’t have a lot of time, and don’t even remember what posts I wanted to review.
I could have just saved my work somewhere and paste it in when review season rolls around, but there really should not be that much friction in the process. The 2022 review period should be at least 6 months, including the entire second half of 2023, and posts from the first half of 2022 should maybe even be reviewable in the first half of 2023.
Replies from: Raemon↑ comment by Raemon · 2024-01-13T03:33:55.967Z · LW(p) · GW(p)
Mmm, nod. I think this is the first request I've gotten for the review period being longer. I think doing this would change it into a pretty different product, and I think I'd probably want to explore other ways of getting-the-thing-you-want. Six months of the year makes it basically always Review Season, and at that point there's not really a schelling nature of "we're all doing reviews at the same time and getting some cross-pollination-of-review-y-ness."
But, we've also been discussing generally having other types of review that aren't part of the Annual Review process (that are less retrospective, and more immediate-but-thorough). That might or might help.
For the immediate future – I would definitely welcome reviews of whatever sort you are inspired to do, basically whenever. If nothing else you could do write it out, and then re-link to it when Review Season comes around.
comment by Thomas Kwa (thomas-kwa) · 2022-08-10T01:47:43.410Z · LW(p) · GW(p)
Below is a list of powerful optimizers ranked on properties, as part of a brainstorm on whether there's a simple core of consequentialism that excludes corrigibility. I think that AlphaZero is a moderately strong argument that there is a simple core of consequentialism which includes inner search.
Properties
- Simple: takes less than 10 KB of code. If something is already made of agents (markets and the US government) I marked it as N/A.
- Coherent: approximately maximizing a utility function most of the time. There are other definitions:
- Not being money-pumped
- Nate Soares's notion in the MIRI dialogues: having all your actions point towards a single goal
- John Wentworth's setup of Optimization at a Distance [? · GW]
- Adversarially coherent: something like "appears coherent to weaker optimizers" or "robust to perturbations by weaker optimizers". This implies that it's incorrigible.
- Sufficiently optimized agents appear coherent - Arbital
- will achieve high utility even when "disrupted" by an optimizer somewhat less powerful
- Search+WM: operates by explicitly ranking plans within a world-model. Evolution is a search process, but doesn't have a world-model. The contact with the territory it gets comes from directly interacting with the world, and this is maybe why it's so slow
Thing | Simple? | Coherent? | Adv. coherent? | Search+WM? |
---|---|---|---|---|
Humans | N | Y | Sometimes | Y |
AIXI-tl | Y | Y | N | Y |
Stockfish | N | Y | Y | Y |
AlphaZero/OAI5 | Y | Y | Y | Y |
Markets | N/A | Y | Y | Y |
US government | N/A | Y | N | Y |
Evolution | Y | N | N | N |
Notes:
- Humans are not adversarially coherent: prospect theory and other cognitive biases can be exploited, indoctrination, etc.
- AIXI-tl is not adversarially coherent because it is an embedded agent and can be switched off etc.
- AlphaZero: when playing chess, you can use another strategy and it still wins
- Markets are inexploitable, but they don't do search in a world-model other than the search done by individual market participants
- The US government is not adversarially coherent in most circumstances, even if its subparts are coherent; lobbying can affect the US government's policies, and it is meant to be corrigible by the voting population.
- Evolution is not coherent: species often evolve to extinction; foxes and rabbits, etc.
comment by Thomas Kwa (thomas-kwa) · 2023-11-25T01:52:19.947Z · LW(p) · GW(p)
Has anyone made an alignment tech tree where they sketch out many current research directions, what concrete achievements could result from them, and what combinations of these are necessary to solve various alignment subproblems? Evan Hubinger made this [LW · GW], but that's just for interpretability and therefore excludes various engineering achievements and basic science in other areas, like control, value learning, agent foundations, Stuart Armstrong's work, etc.
Replies from: technicalities↑ comment by technicalities · 2023-11-27T11:48:18.224Z · LW(p) · GW(p)
comment by Thomas Kwa (thomas-kwa) · 2024-11-18T19:39:42.883Z · LW(p) · GW(p)
The North Wind, the Sun, and Abadar
One day, the North Wind and the Sun argued about which of them was the strongest. Abadar, the god of commerce and civilization, stopped to observe their dispute. “Why don’t we settle this fairly?” he suggested. “Let us see who can compel that traveler on the road below to remove his cloak.”
The North Wind agreed, and with a mighty gust, he began his effort. The man, feeling the bitter chill, clutched his cloak tightly around him and even pulled it over his head to protect himself from the relentless wind. After a time, the North Wind gave up, frustrated.
Then the Sun tried his turn. Beaming warmly from the heavens, the Sun caused the air to grow pleasant and balmy. The man, feeling the growing heat, loosened his cloak and eventually took it off in the heat, resting under the shade of a tree. The Sun began to declare victory, but as soon as he turned away, the man put on the cloak again.
The god of commerce then approached the traveler and bought the cloak for five gold coins. The traveler tucked the money away and continued on his way, unbothered by either wind or heat. He soon bought a new cloak and invested the remainder in an index fund. The returns were steady, and in time the man prospered far beyond the value of his simple cloak, while the cloak was Abadar's permanently.
Commerce, when conducted wisely, can accomplish what neither force nor gentle persuasion alone can achieve, and with minimal deadweight loss.
comment by Thomas Kwa (thomas-kwa) · 2022-08-15T23:01:05.984Z · LW(p) · GW(p)
Suppose that humans invent nanobots that can only eat feldspars (41% of the earth's continental crust). The nanobots:
- are not generally intelligent
- can't do anything to biological systems
- use solar power to replicate, and can transmit this power through layers of nanobot dust
- do not mutate
- turn all rocks they eat into nanobot dust small enough to float on the wind and disperse widely
Does this cause human extinction? If so, by what mechanism?
Replies from: JBlack, Dagon↑ comment by JBlack · 2022-08-16T00:43:24.700Z · LW(p) · GW(p)
One of the obvious first problems is that pretty much every mountain and most of the hills in the world will experience increasingly frequent landslides as much of their structural strength is eaten, releasing huge plumes of dust that blot out the sun and stay in the atmosphere. Continental shelves collapse into the oceans, causing tsunamis and the oceans fill with the suspended nanobot dust. Biological photosynthesis pretty much ceases, and the mean surface temperature drops below freezing as most of the sunlight power is intercepted in the atmosphere and redirected through the dust to below the surface where half the rocks are being turned into more dust.
If the bots are efficient with their use of solar power this could start happening within weeks, far too fast for humans to do anything to preserve their civilization. Almost all concrete contains at least moderate amounts of feldspars, so a large fraction of the structures in the world collapse when their foundations rot away beneath them.
Most of the people probably die by choking on the dust while the remainder freeze or die of thirst, whichever comes first in their local situation.
↑ comment by Dagon · 2022-08-15T23:27:01.067Z · LW(p) · GW(p)
It's hard to imagine these constraints actually holding up well, nor the unstated constraint that the ability to make nanobots is limited to this one type.
My actual prediction depends a whole lot on timeframes - how fast do they replicate, how long to dust-ify all the feldspar. If it's slow enough (millenia), probably no real harm - the dust re-solidifies into something else, or gets into an equilibrium where it's settling and compressing as fast as the nanos can dustify it. Also, humans have plenty of time to adapt and engineer workarounds to any climate or other changes.
If they replicate fast, over the course of weeks, it's probably an extinction event for all of earth life. Dust shuts out the sun, all surface features are undermined and collapse, everything is dead and even the things that survive don't have enough of a cycle to continue very long.
comment by Thomas Kwa (thomas-kwa) · 2022-05-23T06:14:39.967Z · LW(p) · GW(p)
Antifreeze proteins prevent water inside organisms from freezing, allowing them to survive at temperatures below 0 °C. They do this by actually binding to tiny ice crystals and preventing them from growing further, basically keeping the water in a supercooled state. I think this is fascinating.
Is it possible for there to be nanomachine enzymes (not made of proteins, because they would denature) that bind to tiny gas bubbles in solution and prevent water from boiling above 100 °C?
comment by Thomas Kwa (thomas-kwa) · 2022-04-15T02:11:05.790Z · LW(p) · GW(p)
Is there a well-defined impact measure to use that's in between counterfactual value and Shapley value, to use when others' actions are partially correlated with yours?
comment by Thomas Kwa (thomas-kwa) · 2024-10-02T15:46:25.645Z · LW(p) · GW(p)
(Crossposted from Bountied Rationality Facebook group)
I am generally pro-union given unions' history of fighting exploitative labor practices, but in the dockworkers' strike that commenced today, the union seems to be firmly in the wrong. Harold Daggett, the head of the International Longshoremen’s Association, gleefully talks about holding the economy hostage in a strike. He opposes automation--"any technology that would replace a human worker’s job", and this is a major reason for the breakdown in talks.
For context, the automation of the global shipping industry, including containerization and reduction of ship crew sizes, is a miracle of today's economy that ensures that famines are rare outside of war, developing countries can climb the economic ladder to bring their citizens out of poverty, and the average working-class American can afford clothes, a car, winter vegetables, and smartphones. A failure to further automate the ports will almost surely destroy more livelihoods than keeping these hazardous and unnecessary jobs could ever gain. So while I think a 70% raise may be justified given the risk of automation and the union's negotiating position, the other core demand to block automation itself is a horribly value-destroying proposition.
In an ideal world we would come to some agreement without destroying value-- e.g. companies would subsidize the pensions of workers unemployed by automation. This has happened in the past, notably the 1960 Mechanization and Modernization Agreement, which guaranteed workers a share of the benefits and was funded by increased productivity. Unfortunately this is not being discussed, and the union is probably opposed. [1] [2]
Both presidential candidates appear pro-union, and it seems particularly unpopular and difficult to be a scab right now. They might also be in personal danger since the ILA has historical mob ties, even if the allegations against current leadership are false. Therefore as a symbolic gesture I will pay $5 to someone who is publicly documented to cross the picket line during an active strike, and $5 to the first commenter to find such a person, if the following conditions are true as of comment date:
- The ILA continues to demand a ban on automation, and no reputable news outlet reports them making an counteroffer of some kind of profit-sharing fund protecting unemployed workers.
- No agreement allowing automation (at least as much as previous contracts) or establishing a profit-sharing fund thing has been actually enacted.
- I can pay them somewhere easily like Paypal, Venmo, or GoFundMe without additional effort.
- It's before 11:59pm PT on October 15.
[1]: "USMX is trying to fool you with promises of workforce protections for semi-automation. Let me be clear: we don’t want any form of semi-automation or full automation. We want our jobs—the jobs we have historically done for over 132 years." https://ilaunion.org/letter-of-opposition-to-usmxs-misleading-statement
[2]: "Furthermore, the ILA is steadfastly against any form of automation—full or semi—that replaces jobs or historical work functions. We will not accept the loss of work and livelihood for our members due to automation. Our position is clear: the preservation of jobs and historical work functions is non-negotiable." https://ilaunion.org/ila-responds-to-usmxs-statement-that-distorts-the-facts-and-misleads-the-public/
Replies from: habryka4, nathan-helm-burger↑ comment by habryka (habryka4) · 2024-10-02T16:20:08.037Z · LW(p) · GW(p)
Huh, can you say more about why you are otherwise pro-union? All unions I have interfaced with were structurally the same as this dockworker's strike. Maybe there were some mid-20th-century unions that were better, there were a lot of fucked up things then, but at least modern unions seem to be somewhat universally terrible in this way.
Replies from: thomas-kwa↑ comment by Thomas Kwa (thomas-kwa) · 2024-10-02T19:01:32.826Z · LW(p) · GW(p)
In theory, unions fix the bargaining asymmetry where in certain trades, job loss is a much bigger cost to the employee than the company, giving the company unfair negotiating power. In historical case studies like coal mining in the early 20th century, conditions without unions were awful and union demands seem extremely reasonable.
My knowledge of actual unions mostly come from such historical case studies plus personal experience of strikes not having huge negative externalities (2003 supermarket strike seemed justified, a teachers' strike seemed okay, a food workers' strike at my college seemed justified). It is possible I'm biased here and will change my views eventually.
I do think some unions impose costs on society, e.g. the teachers' union also demanded pay based on seniority rather than competence, it seems reasonable for Reagan to break up the ATC union, and inefficient construction union demands are a big reason construction costs are so high for things like the 6-mile, $12 billion San Jose BART Extension. But on net the basic bargaining power argument just seems super compelling. I'm open to counterarguments both that unions don't achieve them in practice and that a "fair" negotiation between capital and labor isn't best for society.
Replies from: habryka4↑ comment by habryka (habryka4) · 2024-10-02T19:13:43.063Z · LW(p) · GW(p)
My sense is unions make sense, but legal protections where companies aren't allowed to route around unions are almost always quite bad. Basically whenever those were granted the unions quickly leveraged what basically amounts to a state-sponsored monopoly, but in ways that are even worse than normal state-sponsored monopolies, because state-sponsored monopolies at least tend to still try to maximize profit, whereas unions tend to basically actively sabotage almost anything that does not myopically give more resources to its members.
↑ comment by Nathan Helm-Burger (nathan-helm-burger) · 2024-10-03T16:40:54.061Z · LW(p) · GW(p)
Unions attempt to solve a coordination/governance issue. Unfortunately, if the union itself has bad governance, it just creates a new governance issue. Like trying to use a 'back fire' to control a forest fire, but then the back fire gets too big and now you have more problems!
I'm pro-union in the sense that they are attempting to solve a very real problem. I'm against them insofar as they have a tendency to create new problems once reaching a large enough power base.
The solution, in my eyes, is better governance within unions, and better governance over unions by governments.
comment by Thomas Kwa (thomas-kwa) · 2024-05-09T21:19:31.363Z · LW(p) · GW(p)
I started a dialogue with @Alex_Altair [LW · GW] a few months ago about the tractability of certain agent foundations problems, especially the agent-like structure problem [LW · GW]. I saw it as insufficiently well-defined to make progress on anytime soon. I thought the lack of similar results in easy settings, the fuzziness of the "agent"/"robustly optimizes" concept, and the difficulty of proving things about a program's internals given its behavior all pointed against working on this. But it turned out that we maybe didn't disagree on tractability much, it's just that Alex had somewhat different research taste, plus thought fundamental problems in agent foundations must be figured out to make it to a good future, and therefore working on fairly intractable problems can still be necessary. This seemed pretty out of scope and so I likely won't publish.
Now that this post [LW · GW] is out, I feel like I should at least make this known. I don't regret attempting the dialogue, I just wish we had something more interesting to disagree about.
Replies from: alexander-gietelink-oldenziel↑ comment by Alexander Gietelink Oldenziel (alexander-gietelink-oldenziel) · 2024-05-10T08:22:50.668Z · LW(p) · GW(p)
This seems valuable! I'd be curious to hear more !!
comment by Thomas Kwa (thomas-kwa) · 2023-04-14T19:11:26.238Z · LW(p) · GW(p)
I'm planning to write a post called "Heavy-tailed error implies hackable proxy". The idea is that when you care about and are optimizing for a proxy , Goodhart's Law sometimes implies that optimizing hard enough for [LW · GW] causes [LW · GW] to stop increasing. [LW · GW]
A large part of the post would be proofs about what the distributions of and must be for , where X and V are independent random variables with mean zero. It's clear that
- X must be heavy-tailed (or long-tailed or something)
- X must have heavier tails than V
The proof seems messy though; Drake Thomas and I have spent ~5 person-days on it and we're not quite done. Before I spend another few days proving this, is it a standard result in statistics? I looked through a textbook and none of the results were exactly what I wanted.
Note that a couple of people have already looked at it for ~5 minutes and found it non-obvious, but I suspect it might be a known result anyway on priors.
Replies from: leogao, arthur-conmy↑ comment by leogao · 2023-04-15T07:23:14.906Z · LW(p) · GW(p)
Doesn't answer your question, but we also came across this effect in the RM Goodharting work, though instead of figuring out the details we only proved that it when it's definitely not heavy tailed it's monotonic, for Regressional Goodhart (https://arxiv.org/pdf/2210.10760.pdf#page=17). Jacob probably has more detailed takes on this than me.
In any event my intuition is this seems unlikely to be the main reason for overoptimization - I think it's much more likely that it's Extremal Goodhart or some other thing where the noise is not independent
↑ comment by Arthur Conmy (arthur-conmy) · 2023-04-15T10:21:30.188Z · LW(p) · GW(p)
Is bullet point one true, or is there a condition that I'm not assuming? E.g if $V$ is the constant $0$ random variable and $X$ is $N(0, 1)$ then the limit result holds, but a Gaussian is neither heavy- nor long-tailed.
Replies from: thomas-kwa↑ comment by Thomas Kwa (thomas-kwa) · 2023-04-15T17:34:22.208Z · LW(p) · GW(p)
I'm also assuming V is not bounded above.
comment by Thomas Kwa (thomas-kwa) · 2020-03-25T08:36:31.741Z · LW(p) · GW(p)
The most efficient form of practice is generally to address one's weaknesses. Why, then, don't chess/Go players train by playing against engines optimized for this? I can imagine three types of engines:
- Trained to play more human-like sound moves (soundness as measured by stronger engines like Stockfish, AlphaZero).
- Trained to play less human-like sound moves.
- Trained to win against (real or simulated) humans while making unsound moves.
The first tool would simply be an opponent when humans are inconvenient or not available. The second and third tools would highlight weaknesses in one's game more efficiently than playing against humans or computers. I'm confused about why I can't find any attempts at engines of type 1 that apply modern deep learning techniques, or any attempts whatsoever at engines of type 2 or 3.
Replies from: thomas-kwa↑ comment by Thomas Kwa (thomas-kwa) · 2020-05-07T02:54:10.155Z · LW(p) · GW(p)
Someone happened to ask a post on Stack Exchange about engines trained to play less human-like sound moves. The question is here, but most of the answerers don't seem to understand the question.
comment by Thomas Kwa (thomas-kwa) · 2024-09-23T22:47:56.861Z · LW(p) · GW(p)
Maybe people worried about AI self-modification should study games where the AI's utility function can be modified by the environment, and it is trained to maximize its current utility function (in the "realistic value functions" sense of Everitt 2016). Some things one could do:
- Examine preference preservation and refine classic arguments about instrumental convergence
- Are there initial goals that allow for stably corrigible systems (in the sense that they won't disable an off switch, and maybe other senses)?
- Try various games and see how qualitatively hard it is for agents to optimize their original utility function. This would be evidence about how likely value drift is to result from self-modification in AGIs.
- Can the safe exploration literature be adapted to solve these games?
- Potentially discover algorithms that seem like they would be good for safety, either through corrigibility or reduced value drift, and apply them to LM agents.
Maybe I am ignorant of some people already doing this, and if so please comment with papers!
comment by Thomas Kwa (thomas-kwa) · 2023-07-18T01:20:23.803Z · LW(p) · GW(p)
I looked at Tetlock's Existential Risk Persuasion Tournament results, and noticed some oddities. The headline result is of course "median superforecaster gave a 0.38% risk of extinction due to AI by 2100, while the median AI domain expert gave a 3.9% risk of extinction." But all the forecasters seem to have huge disagreements from my worldview on a few questions:
- They divided forecasters into "AI-Concerned" and "AI-Skeptic" clusters. The latter gave 0.0001% for AI catastrophic risk before 2030, and even lower than this (shows as 0%) for AI extinction risk. This is incredibly low, and don't think you can have probabilities this low without a really good reference class.
- Both the AI-Concerned and AI-skeptic clusters gave low probabilities for space colony before 2030, 0.01% and "0%" medians respectively.
- Both groups gave numbers I would disagree with for the estimated year of extinction: year 3500 for AI-concerned, and 28000 for AI-skeptic. Page 339 suggests that none of the 585 survey participants gave a number above 5 million years, whereas it seems plausible to me and probably many EA/LW people on the "finite time of perils" thesis that humanity survives for 10^12 years or more, likely giving an expected value well over 10^10. The justification given for the low forecasts even among people who believed the "time of perils" arguments seems to be that conditional on surviving for millions of years, humanity will probably become digital, but even a 1% chance of the biological human population remaining above the "extinction" threshold of 5,000 still gives an expected value in the billions.
I am not a forecaster and would probably be soundly beaten in any real forecasting tournament, but perhaps there is a bias against outlandish-seeming forecasts, strongest in this last question, that also affects the headline results.
Replies from: Unnamed↑ comment by Unnamed · 2023-07-18T02:13:46.381Z · LW(p) · GW(p)
I believe the extinction year question was asking for a median, not an expected value. In one place in the paper it is paraphrased as asking "by what year humanity is 50% likely to go extinct".
Replies from: Vladimir_Nesov↑ comment by Vladimir_Nesov · 2023-07-18T08:29:12.527Z · LW(p) · GW(p)
If extinction caused by AI or value drift [LW(p) · GW(p)] is somewhat unlikely, then extinction only happens once there is no more compute in the universe, which might take a very long time. So "the year humanity is 50% likely to go extinct" could be or something.
comment by Thomas Kwa (thomas-kwa) · 2024-09-27T11:07:15.683Z · LW(p) · GW(p)
A Petrov Day carol
This is meant to be put to the Christmas carol "In the Bleak Midwinter" by Rossetti and Holst. Hopefully this can be occasionally sung like "For The Longest Term" is in EA spaces, or even become a Solstice thing.
I tried to get Suno to sing this but can't yet get the lyrics, tune, and style all correct; this is the best attempt. I also will probably continue editing the lyrics because parts seem a bit rough, but I just wanted to write this up before everyone forgets about Petrov Day.
[edit: I got a good rendition after ~40 attempts! It's a solo voice though which is still not optimal.]
[edit: lyrics v2]
In the bleak midwinter
Petrov did forestall,
Smoke would block our sunlight,
Though it be mid-fall.
New York in desolation,
Moscow too,
In the bleak midwinter
We so nearly knew.The console blinked a warning,
Missiles on their way,
But Petrov chose to question
What the screens did say.
Had he sounded the alarm,
War would soon unfold,
Cities turned to ashes;
Ev'ry hearth gone cold.Poison clouds loom o'er us,
Ash would fill the air,
Fields would yield no harvest,
Famine everywhere.
Scourge of radiation,
Its sickness spreading wide,
Children weeping, starving,
With no place to hide.But due to Petrov's wisdom
Spring will yet appear;
Petrov defied orders,
And reason conquered fear.
So we sing his story,
His deed we keep in mind;
From the bleak midwinter
He saved humankind.(ritard.)
From the bleak midwinter
He saved humankind.
comment by Thomas Kwa (thomas-kwa) · 2023-10-04T00:17:09.483Z · LW(p) · GW(p)
Question for @AnnaSalamon [LW · GW] and maybe others. What's the folk ethics analysis [LW(p) · GW(p)] behind the sinking of the SF Hydro, which killed 14 civilians but destroyed heavy water to be used in the Nazi nuclear weapons program? Eliezer used this as a classic example of ethical injunctions [LW · GW] once.
Replies from: AnnaSalamon↑ comment by AnnaSalamon · 2023-10-04T01:19:45.144Z · LW(p) · GW(p)
I like the question; thanks. I don't have anything smart to say about at the moment, but it seems like a cool thread.
comment by Thomas Kwa (thomas-kwa) · 2023-07-24T21:09:09.750Z · LW(p) · GW(p)
People say it's important to demonstrate alignment problems like goal misgeneralization. But now, OpenAI, Deepmind, and Anthropic have all had leaders sign the CAIS statement on extinction risk and are doing substantial alignment research. The gap between the 90th percentile alignment concerned people at labs and the MIRI worldview is now more about security mindset. Security mindset is present in cybersecurity because it is useful in the everyday, practical environment researchers work in. So perhaps a large part of the future hinges on whether security mindset becomes useful for solving problems in applied ML.
- Will it become clear that the presence of one bug in an AI system implies that there are probably five more?
- Will architectures that we understand with fewer moving parts be demonstrated to have better robustness than black-box systems or systems that work for complicated reasons?
- Will it become tractable to develop these kinds of simple transparent systems so that security mindset can catch on?
↑ comment by Ben Pace (Benito) · 2023-07-24T21:30:25.484Z · LW(p) · GW(p)
the CAIS letter
I suggest calling it "the sentence on extinction risk" so that people can pick up what is meant without having to have already memorized an acronym.
Replies from: thomas-kwa↑ comment by Thomas Kwa (thomas-kwa) · 2023-07-24T21:31:51.402Z · LW(p) · GW(p)
Edited, thanks
comment by Thomas Kwa (thomas-kwa) · 2021-11-05T04:09:06.564Z · LW(p) · GW(p)
The author of "Where Is My Flying Car" says that the Feynman Program (teching up to nanotechnology by machining miniaturized parts, which are assembled into the tools for micro-scale machining, which are assembled into tools for yet smaller machining, etc) might be technically feasible and the only reason we don't have it is that no one's tried it yet. But this seems a bit crazy for the following reasons:
- The author doesn't seem like a domain expert
- AFAIK this particular method of nanotechnology was just an idea Feynman had in the famous speech and not a serious proposal (he introduces it with "let me suggest one weird possibility")
- Investment in the Feynman Program, if it's feasible, would pay off quickly, since there are large economic benefits to the incremental microtechnologies (getting halfway there at a reasonable price would essentially produce any manufactured good that doesn't require nanoscale processes, which seems like most of them, while also increasing quality).
- In particular, semiconductor manufacturing started at the micro-scale, and many companies converged on lithography for manufacturing rather than using miniature robots. Then we invented MEMS, which is machinery created using lithography-like processes, and chip factories never switched from lithography to MEMS. We never even progressed from MEMS to fully general microtechnology.
Does anyone with domain expertise have further thoughts?
Replies from: JBlack, ChristianKl↑ comment by JBlack · 2021-11-05T08:50:24.781Z · LW(p) · GW(p)
I'm not a domain expert in micromachines, but have studied at least miniature machines as part of a previous job.
One very big problem is volume. Once you get down below tonne scale, making and assembling small parts with fine tolerances is not really any less expensive than making and assembling larger parts with comparatively the same tolerances.
That is, each one-gram machine made of a thousand parts probably won't cost you any less than a hundred-kilogram machine made of a thousand parts. It will almost certainly cost more, since it will require new techniques to make, assemble, and operate at the smaller scale. The cost of maintenance per machine almost certainly goes up since there are more layers of indirection in diagnosis and rectification of problems.
So this doesn't scale down at all: attention is a limiting factor. With advanced extrapolations from current techniques, maybe we could eventually make nanogram robot arms for merely the same cost as hundred kilogram robot arms. That doesn't help much if each one costs $10,000 and needs maintenance every few weeks. We need some way to make a trillion of them for $10k, and for them to do what we want without any individual attention at all.
Replies from: Gunnar_Zarncke↑ comment by Gunnar_Zarncke · 2021-11-05T09:01:44.862Z · LW(p) · GW(p)
Seems like the key claim:
making and assembling small parts with fine tolerances is not really any less expensive than making and assembling larger parts with comparatively the same tolerances.
Can you give any hint why that is or could be?
Replies from: JBlack↑ comment by JBlack · 2021-11-07T00:43:38.979Z · LW(p) · GW(p)
I wasn't ever involved with manufacture of the individual parts, so I don't have direct experience.
I suspect it's just that as you go smaller, material costs become negligible compared with process costs. Process costs don't change much, because you still need humans to oversee the machines carrying out the processes, and there are similar numbers of processes with as many steps involved no matter how large or small the parts are. The processes themselves might be different, because some just can't scale down below a certain size for physics reasons, but it doesn't get easier at smaller scales.
Also, direct human labour still plays a fairly crucial role in most processes. There are (so far) always some things to be done where human capabilities exceed those of any machine we can build at reasonable cost.
↑ comment by ChristianKl · 2021-11-07T09:02:37.671Z · LW(p) · GW(p)
The author doesn't seem like a domain expert
Wikipedia describes the author as saying:
John Storrs "Josh" Hall is involved in the field of molecular nanotechnology.[1] He founded the sci.nanotech Usenet newsgroup and moderated it for ten years, and served as the founding chief scientist of Nanorex Inc. for two years. He has written several papers on nanotechnology and developed several ideas such as the utility fog, the space pier, a weather control system called The Weather Machine and a novel flying car.
He is the author of Nanofuture: What's Next for Nanotechnology (ISBN 1-59102-287-8), a fellow of the Molecular Engineering Research Institute and Research Fellow of the Institute for Molecular Manufacturing.
Hall was also a computer systems architect at the Laboratory for Computer Science Research at Rutgers University from 1985 until 1997. In February 2009, Hall was appointed president of the Foresight Institute.
In 2006, the Foresight Nanotech Institute awarded Hall the Feynman Communication Prize.[2] [3]
What do you mean by "domain expert" is that doesn't count him as being one?
Replies from: thomas-kwa, gjm↑ comment by Thomas Kwa (thomas-kwa) · 2021-11-07T19:55:54.624Z · LW(p) · GW(p)
I think a MEMS engineer would be better suited to evaluate whether the engineering problems are feasible than a computer scientist / futurist author. Maybe futurists could outdo ML engineers on AI forecasting. But I think the author doesn't have nearly as detailed an inside view about nanotech as futurists on AI. There's no good answer in the book to the "attention bottleneck" objection JBlack just made, and no good story for why the market is so inefficient.
developed several ideas such as the utility fog, the space pier, a weather control system called The Weather Machine
These are all ideas of the form "If we could make fully general nanotechnology, then we could do X". Gives me the same vibe as this. Saying "nuclear reactor. . . you have hydrogen go through the thing. . . Zoom! it's a rocket" doesn't mean you can evaluate whether a nuclear reactor is feasible at 194X tech level, and thinking of the utility fog doesn't mean you can evaluate whether MEMS can be developed into general nanotech at 202X tech level.
↑ comment by gjm · 2021-11-07T13:28:40.557Z · LW(p) · GW(p)
I can't comment on what JBlack means by "domain expert", but looking at that list of things about Hall, what I see is:
- "Involved in", which means nothing.
- Founded and moderated a newsgroup: requires no particular domain expertise.
- Founding chief scientist of Nanorex Inc for two years. I can't find any evidence that Nanorex ever produced anything other than a piece of software that claimed to do molecular dynamics suitable for simulating nanotech. Whether it was actually any good, I have no idea, but the company seems not to have survived. Depending on what exactly the responsibilities of the "founding chief scientist" are, this could be evidence that Hall understands a lot about molecular dynamics, or evidence that Hall is a good software developer, or evidence of nothing at all. In the absence of more information about Nanorex and their product, it doesn't tell us much.
- Has written several papers on nanotechnology: anyone can write a paper. A quick look for papers he's written turns up some abstracts, all of which seem like high-level "here's a concept that may be useful for nanotech" ones. Such a paper could be very valuable and demonstrate deep insight, but the test of that would be actually turning out to be useful for nanotech and so far as I can tell his ideas haven't led to anything much.
- Developed ideas such as utility fog, space pier, etc.: again, anyone can "develop ideas". The best test of the idea-developer's insight is whether those ideas turn out actually to be of any use. So far, we don't seem close to having utility fog, space piers, weather control or flying cars.
- Author of "Nanofuture": pop-science book, which from descriptions I've read seems mostly to be broad general principles about nanotech that doesn't exist yet, and exciting speculations about future nanotech thatt doesn't exist yet.
- Fellow of a couple of things: without knowing exactly what their criteria are for appointing Fellows, this could mean anything or nothing. My guess is that they made him a Fellow because he was an early popularizer of nanotech ideas, not because of actual expertise or accomplishments.
- Also, literally the only things I can find about the "Molecular Engineering Research Institute" are places where Storrs-Hall describes himself as that. It looks worryingly like one of those pseudoscholarly institutions that a person or small group creates in order to sound more impressive. I'm willing to be corrected on this one, though.
- I can't find much sign that the "Institution for Molecular Manufacturing" is much better, actually.
- Computer systems architect at the Laboratory for Computer Science Research at Rutgers: This does seem to be a real thing at a real university. I think it is evidence that Storrs-Hall knows something about writing software.
- Awarded the Feynman Communication Prize: implies no particular domain expertise.
Everything here is consistent with Storrs-Hall being a competent software guy who had a bunch of vague but exciting-sounding ideas about nanotech, writes well, and was in the right place at the right time -- and nothing more than that.
(It's also consistent with his being more expert than that. But it doesn't seem like it's much evidence for that's being so.)
Replies from: Gunnar_Zarncke↑ comment by Gunnar_Zarncke · 2021-11-07T14:18:58.304Z · LW(p) · GW(p)
I guess very few people live up to your requirements for domain expertise.
Replies from: gjm↑ comment by gjm · 2021-11-07T16:39:06.953Z · LW(p) · GW(p)
In nanotech? True enough, because I am not convinced that there is any domain expertise in the sort of nanotech Storrs Hall writes about. It seems like a field that consists mostly of advertising. (There is genuine science and genuine engineering in nano-stuff; for instance, MEMS really is a thing. But the sort of "let's build teeny-tiny mechanical devices, designed and built at the molecular level, which will be able to do amazing things previously-existing tech can't" that Storrs Hall has advocated seems not to have panned out.)
But more generally, that isn't so at all. What I'm looking for by way of domain expertise in a technological field is a history of demonstrated technological achievements. Storrs Hall has one such achievement that I can see, and even that is doubtful. (He founded and was "chief scientist" of a company that made software for simulating molecular dynamics. I am not in a position to tell either how well the software actually worked or how much of it was JSH's doing.) More generally, I want to see a history of demonstrated difficult accomplishments in the field, as opposed to merely writing about the field.
Selecting some random books from my shelves (literally random; I have a list and am selecting random lines from the file, discarding fiction and anthologies), I find:
- Nigel Slater, "Real fast food". Slater is more food-journalist than chef, but there the proof is in the eating. I have made many things from his books and found that they consistently taste good and don't require excessive effort, which for me is sufficient reason to consider him a domain expert. Non-technical domain, though.
- Alec Vidler, "Essays in liberality". A book of theological essays. Highly non-technical domain, much worse even than nanotech in terms of whether such a thing as domain expertise exists.
- Richard Dawkins, "Unweaving the rainbow". I like Dawkins, but it's debatable whether he passes my domain-expert test; the main thing he's known for in evolutionary biology is the "selfish gene" concept, which is more a new perspective on ideas others had already had than a new discovery in its own right.
- John Potter, "The Cambridge companion to singing". Potter is a tenor who has made >100 commercial recordings and performed in groups such as the Hilliard Ensemble. Singing well enough that anyone wants to sell your performances, or well enough to be a member of a group like the Hilliard Ensemble, is a difficult accomplishment in the field, and for this I consider him a domain expert. (Until his retirement he was also on the music faculty at a good university, but that's only indirect evidence.)
- Peter Sagal, "The book of vice". Highly non-technical domain; author is a humourist and journalist. Not a domain expert.
- Ruppert, Wand & Carroll, "Semiparametric regression". I'll consider only the first-named author. Author of what looks like about 100 statistical papers. Many of them are applied stats papers in journals with other subjects, suggesting that what he does is useful outside statistics itself. I had a look at one of his papers; it looks as if it is doing actual mathematics, discovering useful things about the merits of a couple of different approaches to data-smoothing. Sure looks like a domain expert.
- H G Atkins, biography of Heinrich Heine. Non-technical domain. All I can easily find out about the author is that he was a professor of German at a good English university. Writing and publishing a biography of Heine is arguably itself evidence of domain expertise in, er, the life of Heine, but this one isn't easy to assess with confidence.
- Laura Miller, "The magician's book: a skeptic's adventures in Narnia". About those books and C S Lewis's life. Author does not appear to be a domain expert by my criterion.
- Matthew Chapman, "40 days and 40 nights", about the Dover intelligent design trial. I guess the domain is something like "history of church-and-state fights in the US"; I don't think the author is a domain expert by my criterion.
- A K Dewdney, "The Planiverse" (a sort of updated "Flatland"). Not clear what would constitute domain expertise, and maybe this should be excluded from the list for being fiction, though clearly its main point is not storytelling. At any rate, Dewdney is a professor of computer science but I don't think he qualifies as a domain expert on two-dimensional universes occupied by intelligent beings.
That's 10 books, which seems like a reasonable place to stop. Somewhere between 3 and 5 of the authors are domain experts by my criterion (generalized in what I think is an honest way to domains other than technology). Maybe 3 of the books are concerned with technical domains (Dawkins, Ruppert et al, Dewdney) and 1 or 2 of the 3 authors qualify.
I picked some more random books to bring the number of technical-domain ones up to 10. I'll summarize more briefly. "(P)" means pop-science/pop-maths; "(T)" means technical works aimed at experts. Ruelle, "Chaotic evolution and strange attractors" (T); domain expert. O'Neill, "Doing data science: straight talk from the frontline" (P): marginal, leaning toward domain expert. Mlodinow, "The drunkard's walk: how randomness rules our lives" (P): marginal, leaning toward not. (Author is a domain expert in theoretical physics, not so much here.) Steele, "Probability theory and combinatorial optimization" (T): domain expert. Nick Lane, "The vital question: why is life the way it is?" (P): not a domain expert. Gribbin, "In search of Schrödinger's cat" (P): not a domain expert. Hatton, "Safer C: developing software for high-integrity and safety-critical systems" (T): domain expert. So, counting Dawkins, O'Neill, Mlodinow as half-domain-experts, I make that five out of 10.
So it seems like if you pull a book on a technical topic off my bookshelves at random, about half the time the author is a domain expert in this (admittedly fairly strong) sense; it seems to be pretty much all the time for hardcore technical works for expert audiences, whereas popular books are more likely to be written by journalists or by experts in other fields.
I wouldn't call that "very few people", in this context. (As a fraction of the whole population it's "very few", and I'm perfectly OK with that and assume you are too.)
Replies from: Gunnar_Zarncke↑ comment by Gunnar_Zarncke · 2021-11-07T17:51:34.287Z · LW(p) · GW(p)
Thank you for this comprehensive answer. I like the requirement of "actual practical accomplishments in the field".
Googling a bit I found this article on miniaturization:
https://www.designnews.com/miniaturization-not-just-electronics-anymore
Would you consider the cited Thomas L. Hicks from American Laubscher a domain expert?
Replies from: gjmcomment by Thomas Kwa (thomas-kwa) · 2020-12-07T03:01:00.644Z · LW(p) · GW(p)
Is it possible to make an hourglass that measures different amounts of time in one direction than the other? Say, 25 minutes right-side up, and 5 minutes upside down, for pomodoros. Moving parts are okay (flaps that close by gravity or something) but it should not take additional effort to flip.
Replies from: mingyuan, effective-egret, mr-hire↑ comment by mingyuan · 2020-12-13T00:54:01.057Z · LW(p) · GW(p)
I don't see why this wouldn't be possible? It seems pretty straightforward to me; the only hard part would be the thing that seems hard about making any hourglass, which is getting it to take the right amount of time, but that's a problem hourglass manufacturers have already solved. It's just a valve that doesn't close all the way:
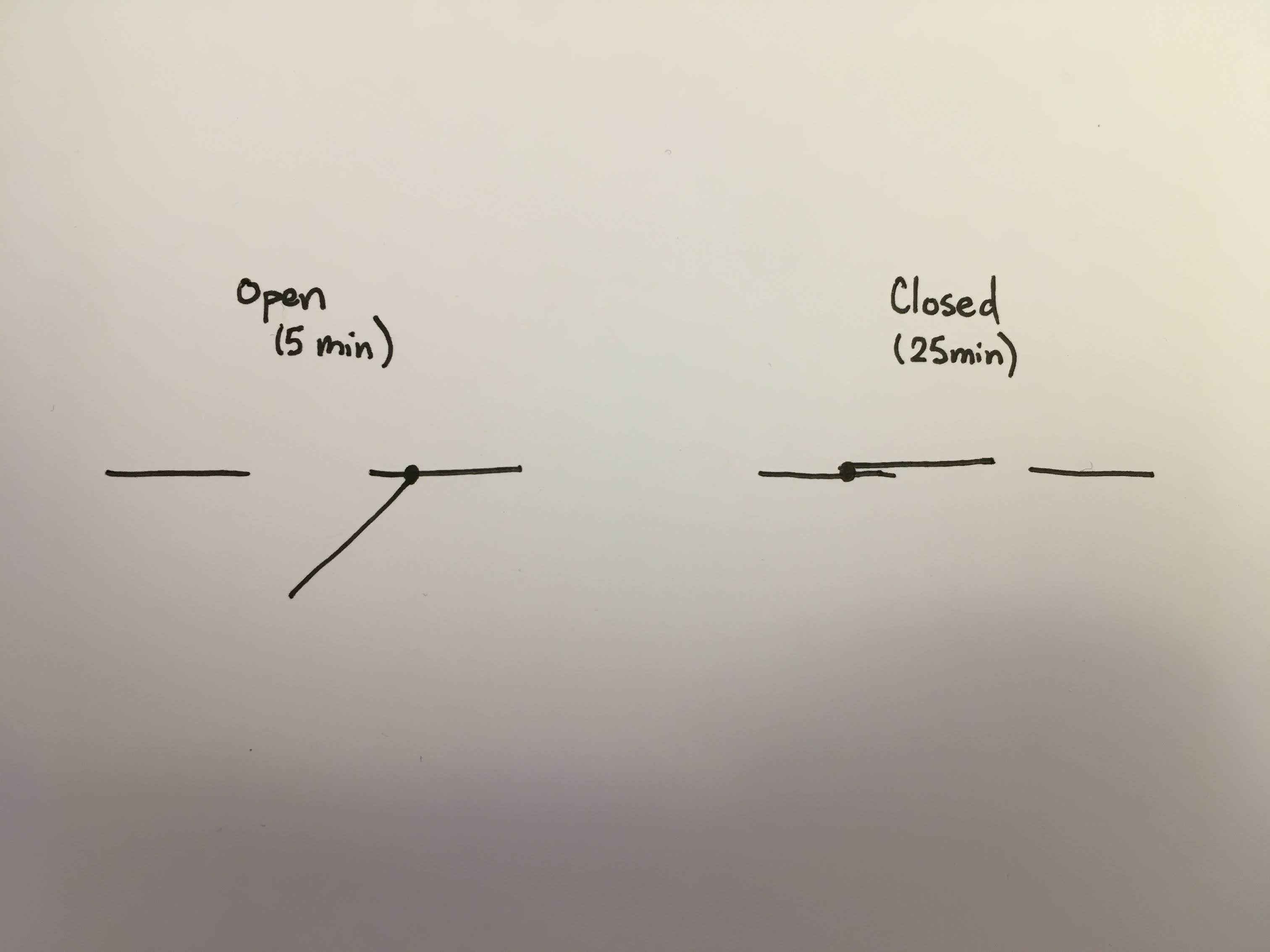
Unless you meant, "how can I make such an hourglass myself, out of things I have at home?" in which case, idk bro.
Replies from: mr-hire↑ comment by Matt Goldenberg (mr-hire) · 2020-12-13T01:07:18.915Z · LW(p) · GW(p)
One question I have about both your solution and mine is how easy it is to vary the time drastically by changing the size of the hole. My intuition says that too large holes behave much differently than smaller holes and if you want a drastic 5x difference in speed you might get into this "too large and the sand sort of just rushes through" behavior.
↑ comment by effective-egret · 2020-12-10T06:20:56.570Z · LW(p) · GW(p)
While I'm sure there's a mechanical solution, my preferred solution (in terms of implementation time) would be to simply buy two hourglasses - one that measures 25 minutes and one that measures 5 minutes - and alternate between them.
Replies from: Gunnar_Zarncke↑ comment by Gunnar_Zarncke · 2021-11-07T14:23:58.438Z · LW(p) · GW(p)
Or just bundle them together like this: https://www.amazon.de/Bredemeijer-B0011-Classic-Teatimer-Edelstahl/dp/B00SN5U5E0/
↑ comment by Matt Goldenberg (mr-hire) · 2020-12-07T04:10:07.468Z · LW(p) · GW(p)
First thought is to have two separate holes of slightly different sizes, each one blocked by a little angled platform from one direction. I am not at all confident you could get this to work in practice
comment by Thomas Kwa (thomas-kwa) · 2020-09-19T00:44:57.788Z · LW(p) · GW(p)
Given that social science research often doesn't replicate [LW · GW], is there a good way to search a social science finding or paper and see if it's valid?
Ideally, one would be able to type in e.g. "growth mindset" or a link to Dweck's original research, and see:
- a statement of the idea e.g. 'When "students believe their basic abilities, their intelligence, their talents, are just fixed traits", they underperform students who "understand that their talents and abilities can be developed through effort, good teaching and persistence." Carol Dweck initially studied this in 2012, measuring 5th graders on IQ tests.'
- an opinion from someone reputable
- any attempted replications, or meta-analyses that mention it
- the Replication Markets predicted replication probability, if no replications have been attempted.
↑ comment by habryka (habryka4) · 2020-09-19T17:17:59.598Z · LW(p) · GW(p)
Alas, the best I have usually been able to do is "<Name of the paper> replication" or "<Name of the author> replication".
comment by Thomas Kwa (thomas-kwa) · 2023-11-25T02:28:16.434Z · LW(p) · GW(p)
An idea for removing knowledge from models
Suppose we have a model with parameters , and we want to destroy a capability-- doing well on loss function -- so completely that fine-tuning can't recover it. Fine-tuning would use gradients , so what if we fine-tune the model and do gradient descent on the norm of the gradients during fine-tuning, or its directional derivative where ? Then maybe if we add the accumulated parameter vector, the new copy of the model won't have useful gradients to fine-tune on.
This is a simple enough idea that it's probably somewhere in the literature, but I don't know where to search; maybe it's been done in an adversarial training context?
Replies from: Depose1121↑ comment by Depose1121 · 2023-11-25T05:37:22.962Z · LW(p) · GW(p)
You are looking for "Fast Gradient Sign Method"
comment by Thomas Kwa (thomas-kwa) · 2023-11-13T23:00:12.133Z · LW(p) · GW(p)
We might want to keep our AI from learning a certain fact about the world, like particular cognitive biases humans have that could be used for manipulation. But a sufficiently intelligent agent might discover this fact despite our best efforts. Is it possible to find out when it does this through monitoring, and trigger some circuit breaker?
Evals can measure the agent's propensity for catastrophic behavior, and mechanistic anomaly detection hopes to do better by looking at the agent's internals without assuming interpretability, but if we can measure the agent's beliefs, we can catch the problem earlier. Maybe there can be more specific evals we give to the agent, which are puzzles that can only be solved if the agent knows some particular fact. Or maybe the agent is factorable into a world-model and planner, and we can extract whether it knows the fact from the world-model.
Have the situational awareness people already thought about this? Does anything change when we're actively trying to erase a belief?
Replies from: arthur-conmy↑ comment by Arthur Conmy (arthur-conmy) · 2023-11-14T16:58:13.730Z · LW(p) · GW(p)
mechanistic anomaly detection hopes to do better by looking at the agent's internals as a black box
Do you mean "black box" in the sense that MAD does not assume interpretability of the agent? If so this is kinda confusing as "black box" is often used in contrast to "white box", ie "black box" means you have no access to model internals, just inputs+outputs (which wouldn't make sense in your context)
Replies from: thomas-kwa↑ comment by Thomas Kwa (thomas-kwa) · 2023-11-14T17:17:29.166Z · LW(p) · GW(p)
Yes, changed the wording
comment by Thomas Kwa (thomas-kwa) · 2022-08-28T03:12:03.860Z · LW(p) · GW(p)
Somewhat related to this post [LW · GW] and this post [? · GW]:
Coherence implies mutual information between actions. That is, to be coherent, your actions can't be independent. This is true under several different definitions of coherence, and can be seen in the following circumstances:
- When trading between resources (uncertainty over utility function). If you trade 3 apples for 2 bananas, this is information that you won't trade 3 bananas for 2 apples, if there's some prior distribution over your utility function.
- When taking multiple actions from the same utility function (uncertainty over utility function). Your actions will all have to act like a phased array [? · GW] pushing the variables you care about in some direction.
- When taking multiple actions based on the same observation (uncertainty over observation / world-state). Suppose that you're trying to juggle, and your vision is either reversed or not reversed. The actions of your left arm and right arm will have mutual information, because they both depend on whether your vision has been reversed in related ways.
This would be a full post, but I don't think it's important enough to write up.
Replies from: Vladimir_Nesov↑ comment by Vladimir_Nesov · 2022-08-28T11:17:47.873Z · LW(p) · GW(p)
Your actions will all have to act like a phased array pushing the variables you care about in some direction.
The nice thing is that this should work even if you are a policy selected by a decision making algorithm, but you are not yourself a decision making algorithm anymore. There is no preference in any of the possible runs of the policy at that point, you don't care about anything now, you only know what you must do here, and not elsewhere. But if all possible runs of the policy are considered altogether (in the updateless sense of maps from epistemic situations to action and future policy), the preference is there, in the shape of the whole thing across all epistemic counterfactuals. (Basically you reassemble a function from pairs (from, to) of things it maps, found in individual situations.)
I guess the at-a-distance part could make use of composition of an agent with some of its outer shells into a behavior that forgets internal interactions (within the agent, and between the agent and its proximate environment). The resulting "large agent" will still have basically the same preference, with respect to distant targets in environment, without a need to look inside the small agent's head, if the large agent's external actions in a sufficient range of epistemic situations can be modeled. (These large agents exist in each inidividual possible situation, they are larger than the small agent within the situation, and they can be compared with other variants of the large agent from different possible situations.)
Not clear what to do with dependence on the epistemic situation of the small agent. It wants to reduce to dependence on a situation in terms of the large agent, but that doesn't seem to work. Possibly this needs something like the telephone theorem, with any relevant-in-some-sense dependence of behavior (of the large agent) on something becoming dependence of behavior on natural external observations (of the large agent) and not on internal noise (or epistemic state of the small agent).
comment by Thomas Kwa (thomas-kwa) · 2022-03-21T20:43:46.786Z · LW(p) · GW(p)
Many people think that AI alignment is intractable (<50% chance of success) and also believe that a universe optimized towards elephant CEV, or the CEV of aliens that had a similar evolutionary environment to humans, would be at least 50% as good as a universe optimized towards human CEV. Doesn't this mean we should be spending significant effort (say, at least 1% of the effort spent on alignment) finding tractable plans to create a successor species in case alignment fails?
Replies from: AprilSR↑ comment by AprilSR · 2022-03-22T16:23:46.338Z · LW(p) · GW(p)
If alignment fails I don’t think it’s possible to safely prepare a successor species. We could maybe try to destroy the earth slightly before the AI turns on rather than slightly after, in the hopes that the aliens don’t screw up the chance we give them?
comment by Thomas Kwa (thomas-kwa) · 2020-07-08T22:30:27.358Z · LW(p) · GW(p)
Are there ring species where the first and last populations actually can interbreed? What evolutionary process could feasibly create one?
Replies from: thomas-kwa, Richard_Kennaway, Pongo↑ comment by Thomas Kwa (thomas-kwa) · 2020-10-01T22:41:52.382Z · LW(p) · GW(p)
One of my professors says this often happens with circular island chains; populations from any two adjacent islands can interbreed, but not those from islands farther apart. I don't have a source. Presumably this doesn't require an expanding geographic barrier.
↑ comment by Richard_Kennaway · 2020-07-09T08:34:14.341Z · LW(p) · GW(p)
Wouldn't that just be a species?
Replies from: Pattern, thomas-kwa↑ comment by Thomas Kwa (thomas-kwa) · 2020-07-09T20:54:25.739Z · LW(p) · GW(p)
I'm thinking of a situation where there are subspecies A through (say) H; A can interbreed with B, B with C, etc., and H with A, but no non-adjacent subspecies can produce fertile offspring.
comment by Thomas Kwa (thomas-kwa) · 2020-06-10T00:56:00.308Z · LW(p) · GW(p)
2.5 million jobs were created in May 2020, according to the jobs report. Metaculus was something like [99.5% or 99.7% confident](https://www.metaculus.com/questions/4184/what-will-the-may-2020-us-nonfarm-payrolls-figure-be/) that the number would be smaller, with the median at -11.0 and 99th percentile at -2.8. This seems like an obvious sign Metaculus is miscalibrated, but we have to consider both tails, making this merely a 1 in 100 or 1 in 150 event, which doesn't seem too bad.
comment by Thomas Kwa (thomas-kwa) · 2024-06-05T08:49:52.888Z · LW(p) · GW(p)
I don't know how to say this without sounding elitist, but my guess is that people who prolifically write LW comments and whose karma:(comments+posts) ratio is less than around 1.5:1 or maybe even 2:1 should be more selective in what they say. Around this range, it would be unwarranted for mods to rate-limit you, but perhaps the bottom 30% of content you produce is net negative considering the opportunity cost to readers.
Of course, one should not Goodhart for karma and Eliezer is not especially virtuous by having a 16:1 ratio, but 1.5:1 is a quite low ratio. You get 1.5 karma if 0.75 high-karma users or 1.5 low-karma users weakly upvote your comment. Considering that it takes barely a second to upvote and comments get tens of views, this is not a high bar for the average comment.
Caveats:
- If your absolute number of comments + posts is low, the risk of making new users participate less outweighs the risk of comments clogging up the frontpage.
- In some contexts votes might underestimate quality, like if your comment is on some esoteric topic that few people engage with.
↑ comment by Garrett Baker (D0TheMath) · 2024-06-05T15:14:35.516Z · LW(p) · GW(p)
Another circumstance where votes underestimate quality is where you often get caught up in long reply-chains (on posts that aren’t “the post of the week”), which seems like a very beneficial use of LessWrong, but typically has much lower readership & upvote rates, but uses a lot of comments.
↑ comment by ilm · 2024-06-05T15:12:45.842Z · LW(p) · GW(p)
Aiming to improve the quality of threads by telling users to post/comment less in a public forum seems to me like it's not going to be very helpful in the long term. If users self-select by simulating how much karma their post/comment gets (perhaps via extrapolating the average karma ratio of their previous posts) it might work for a subset, but will fail for other cases:
Namely:
- you'll miss people who would have benefited from the advice but predict the quality of their comment wrong ("yes I have a low average but this next post will be my big break")
- you'll affect people who the advice wasn't directed to / who wouldn't have needed it but implemented it anyway ("it must be my low quality thoughts that are the problem, better leave all writing to Eliezer" or "looks like I have an average score of {TOO_LOW}, better not post this {ACTUALLY_INSIGHTFUL_INSIGHT}.")
As you said yourself, it only takes a few seconds to upvote - so it does to downvote. I would expect the system to converge to lower quality comments being voted down more so the observed quality of the comment will roughly align with the votes on the comment.
If the system does not allow you to filter out comments that are below some downvote/upvote ratio, then perhaps the system needs to be tweaked to allow this kind of filtering - but the solution is not telling users to post less. (And if someone is serially creating low quality content, this person can usually be approached individually, rather than trying to optimize for the public forum.)
This seems worthwhile to care about for two reasons:
- There's a real risk of driving away people who would have something valuable to contribute but who end up self censoring before they post anything (and, AIUI, the bar for LessWrong is already really high).
- There's a risk of people optimizing for karma ratio rather than quality of the comment they're posting. The mental shortcut to take is "Now I have enough karma to make a low effort comment" which is probably not the effect you want.
(edit: improved the first paragraph to better articulate what I mean to say.)
↑ comment by FlorianH (florian-habermacher) · 2024-06-05T18:23:35.525Z · LW(p) · GW(p)
Don't fully disagree, but still inclined to not view non-upvoted-but-neither-downvoted things too harshly:
If I'm no exception, not upvote may often mean: 'Still enjoyed the quick thought-stimulation even if it seems ultimately not a particularly pertinent message'. One can always down vote, if one really feels its warranted.
Also: If one erratically reads LW, and hence comments on old posts: recipe for fewer upvotes afaik. So one'd have to adjust for this quite strongly.
↑ comment by Orpheus16 (akash-wasil) · 2024-06-05T15:54:01.038Z · LW(p) · GW(p)
*Quickly checks my ratio*
"Phew, I've survived the Kwa Purge"
↑ comment by Dagon · 2024-06-05T13:59:51.188Z · LW(p) · GW(p)
Are all users' post/comment counts and karma available in bulk? I'd be curious what the distribution looks like. I'm also curious what my ratio is for recent comments - the fact that it's 2.3 over more than a decade doesn't tell me much.
I often have to argue at work against the tyranny of metrics (I more often have to argue against the ignorance of not enough metrics, but that's not important to this point). This is a classic example of something people should probably look at and consider often, but it's highly context- and individual-dependent whether a change is warranted.
Karma isn't consistent nor rigorous enough to set targets for.
edit: I’m actually more curious about variance in the supply side. I vary pretty widely in how much I vote based on topic and mood, not on any unidimensional quality estimate. Showing use users their recent vote/read ratio could encourage voting.
↑ comment by Alex_Altair · 2024-06-05T17:34:40.798Z · LW(p) · GW(p)
[Continuing to sound elitist,] I have a related gripe/hot take that comments give people too much karma. I feel like I often see people who are "noisy" in that they comment a lot and have a lot of karma from that,[1] but have few or no valuable posts, and who I also don't have a memory of reading valuable comments from. It makes me feel incentivized to acquire more of a habit of using LW as a social media feed, rather than just commenting when a thought I have passes my personal bar of feeling useful.
- ^
Note that self-karma contributes to a comments position within the sorting, but doesn't contribute to the karma count on your account, so you can't get a bunch of karma just by leaving a bunch of comments that no one upvotes. So these people are getting at least a consolation prize upvote from others.
↑ comment by the gears to ascension (lahwran) · 2024-06-05T22:24:21.985Z · LW(p) · GW(p)
Right at the top of that range, I agree, I probably comment too much for the amount I have to contribute. talking to people is fun though. a flipside to this: if you agree with Thomas, consider downvoting stuff that you didn't find to be worth the time, to offset participation-trophy level upvotes.
comment by Thomas Kwa (thomas-kwa) · 2024-04-30T07:16:23.496Z · LW(p) · GW(p)
Hangnails are Largely Optional
Hangnails are annoying and painful, and most people deal with them poorly. [1] Instead, use a drop of superglue to glue it to your nail plate. It's $10 for 12 small tubes on Amazon. Superglue is also useful for cuts and minor repairs, so I already carry it around everywhere.
Hangnails manifest as either separated nail fragments or dry peeling skin on the paronychium (area around the nail). In my experience superglue works for nail separation, and a paper (available free on Scihub) claims it also works for peeling skin on the paronychium.
Is this safe?
Cyanoacrylate glue is regularly used in medicine to close wounds, and now frequently replaces stitches. Medical superglue has slightly different types of cyanoacrylate, but doctors I know say it's basically the same thing.
I think medical superglue exists to prevent rare reactions and for large wounds where the exothermic reaction from a large quantity might burn you, and the safety difference for hangnails is minimal [2]. But to be extra safe you could just use 3M medical grade superglue or Dermabond.
[1]: Typical responses to hangnails include:
- Pulling them out, which can lead to further bleeding or infection.
- Trimming them with nail clippers, which often leaves a jagged edge.
- Wrapping the affected finger in a bandage, requiring daily changes.
[2]: There have been studies showing cytotoxicity in rabbits when injecting it in their eyes, or performing internal (bone or cartilage) grafts. A 2013 review says that although some studies have found internal toxicity, "[f]or wound closure and various other procedures, there have been a considerable number of studies finding histologic equivalence between ECA [commercial superglue] and more widely accepted modalities of repair."
Replies from: nim↑ comment by nim · 2024-04-30T14:36:01.624Z · LW(p) · GW(p)
If you don't need 12 tubes of superglue, dollar stores often carry 4 tiny tubes for a buck or so.
I'm glad that superglue is working for you! I personally find that a combination of sharp nail clippers used at the first sign of a hangnail, and keeping my hands moisturized, works for me. Flush cutters of the sort you'd use to trim the sprues off of plastic models are also amazing for removing proto-hangnails without any jagged edge.
Another trick to avoiding hangnails is to prevent the cuticles from growing too long, by pushing them back regularly. I personally like to use my teeth to push back my cuticles when showering, since the cuticle is soft from the water, my hands are super clean, and it requires no extra tools. I recognize that this is a weird habit, though, and I think the more normal ways to push cuticles are to use your fingernails or a wooden stick (manicurists use a special type of dowel but a popsicle stick works fine).
You can also buy cuticle remover online, which is a chemical that softens the dried skin of the cuticle and makes it easier to remove from your nails. It's probably unnecessary, but if you're really trying to get your hands into a condition where they stop developing hangnails, it's worth considering.
comment by Thomas Kwa (thomas-kwa) · 2024-01-01T16:16:01.142Z · LW(p) · GW(p)
Current posts in the pipeline:
- Dialogue on whether agent foundations is valuable, with Alex Altair. I might not finish this.
- Why junior AI safety researchers should go to ML conferences
- Summary of ~50 interesting or safety-relevant papers from ICML and NeurIPS this year
- More research log entries
- A list of my mistakes from the last two years. For example, spending too much money
- Several flowcharts / tech trees for various alignment agendas.
comment by Thomas Kwa (thomas-kwa) · 2023-11-16T20:05:05.120Z · LW(p) · GW(p)
comment by Thomas Kwa (thomas-kwa) · 2023-10-15T04:17:51.191Z · LW(p) · GW(p)
I'm reading a series of blogposts called Extropia's Children about the Extropians mailing list and its effects on the rationalist and EA communities. It seems quite good although a bit negative at times.
Replies from: Viliam↑ comment by Viliam · 2023-10-15T16:05:42.585Z · LW(p) · GW(p)
In my opinion, it is clickbait, but I didn't notice any falsehoods (I didn't check carefully, just skimmed).
For people familiar with the rationalist community, it is a good reminder of bad things that happened. For people unfamiliar with the community... it will probably make them believe that the rationalist community consists mostly of Leverage, neoreaction, and Zizians.
Replies from: thomas-kwa↑ comment by Thomas Kwa (thomas-kwa) · 2023-10-15T20:06:03.506Z · LW(p) · GW(p)
Seems reasonable. Though I will note that bad things that happened are a significant fraction of the community early on, so people who read sections 1-3 with the reminder that it's a focus on the negative will probably not get the wrong idea.
I did notice a misleading section which might indicate there are several more:
MIRI tends to portray [TDT/UDT/FDT] as technical, practical, immediately applicable work. Others, even EA/rationalists, describe [EA · GW] it as “philosophical decision theory” which “has gained significantly fewer advocates among professional philosophers than I’d expect it to if it were very promising.”
Both sentences are wrong. I don't think MIRI portrays any agent foundations as "practical, immediately applicable work", and in fact the linked post by Demski is listing some basic theoretical problems.
The quote by Daniel Dewey is taken out of context: he's investigated their decision theory work in depth by talking to other professional philosophers, and found it to be promising, so the claim that it has "significantly fewer advocates among professional philosophers than I’d expect it to if it were very promising" is a claim that professional philosophers won't automatically advocate for a promising idea, not that the work is unpromising.
Replies from: Viliam↑ comment by Viliam · 2023-10-15T20:47:48.088Z · LW(p) · GW(p)
bad things that happened are a significant fraction of the community early on
I'd like to read an impartial account, which would specify how large each fraction actually was.
For instance, if I remember correctly, in some survey 2% of Less Wrong readers identified as neoreactionaries. From some perspective, 2% is too much, because the only acceptable number is 0%. From a different perspective, 2% is less than the Lizardman's Constant. Also, if I remember correctly, a much larger fraction of LessWrong readership identified on the survey as communist, and yet for some reason there are no people writing blogs or Wikipedia articles about how Less Wrong is a communist website. Or a socialist website. Or a Democrat website. Or... whatever else was in the poll.
The section on Zizians is weird, because it correctly starts with saying that Zizians opposed MIRI and CFAR... and yet concludes that the this is evidence that people attracted to rationalism are disproportionately prone to death spirals off the deep end. Notice the sleight of hand: "people attracted to you" technically includes your enemies who can't stop thinking about you. -- Using the same rhetorical trick: Westboro Baptist Church is evidence that people attracted to (the topic of) homosexuality are often crazy. Also, by the same logic, every celebrity is responsible for her stalkers.
There are cases when the rationalist community actually promoted harmful people and groups, such as Vassar or Leverage. I'd like to read a serious analysis of how and why that happened, and how to prevent something like that in future. But if another Ziz appears in future, and starts recruiting people in another crazy cult opposed to rationalists, I am not sure how exactly to prevent that.
comment by Thomas Kwa (thomas-kwa) · 2020-03-22T23:51:18.114Z · LW(p) · GW(p)
Eliezer Yudkowsky wrote in 2016:
At an early singularity summit, Jürgen Schmidhuber, who did some of the pioneering work on self-modifying agents that preserve their own utility functions with his Gödel machine, also solved the friendly AI problem. Yes, he came up with the one true utility function that is all you need to program into AGIs!
(For God’s sake, don’t try doing this yourselves. Everyone does it. They all come up with different utility functions. It’s always horrible.)
His one true utility function was “increasing the compression of environmental data.” Because science increases the compression of environmental data: if you understand science better, you can better compress what you see in the environment. Art, according to him, also involves compressing the environment better. I went up in Q&A and said, “Yes, science does let you compress the environment better, but you know what really maxes out your utility function? Building something that encrypts streams of 1s and 0s using a cryptographic key, and then reveals the cryptographic key to you.”
At first it seemed to me that EY refutes the entire idea that "increasing the compression of environmental data" is intrinsically valuable. This surprised me because my intuition says it is intrinsically valuable, though less so than other things I value.
But EY's larger point was just that it's highly nontrivial for people to imagine the global maximum of a function. In this specific case, building a machine that encrypts random data seems like a failure of embedded agency [LW · GW] rather than a flaw in the idea behind the utility function. What's going on here?
Replies from: Viliam↑ comment by Viliam · 2020-03-24T00:46:12.470Z · LW(p) · GW(p)
Something like Goodhart's Law, I suppose. There are natural situations where X is associated with something good, but literally maximizing X is actually quite bad. (Having more gold would be nice. Converting the entire universe into atoms of gold, not necessarily so.)
EY has practiced the skill of trying to see things like a machine. When people talk about "maximizing X", they usually mean "trying to increase X in a way that proves my point"; i.e. they use motivated thinking.
Whatever X you take, the priors are almost 100% that literally maximizing X would be horrible. That includes the usual applause lights, whether they appeal to normies or nerds.
comment by Thomas Kwa (thomas-kwa) · 2023-01-24T19:58:47.896Z · LW(p) · GW(p)
What was the equation for research progress referenced in Ars Longa, Vita Brevis?
Replies from: gwern“Then we will talk this over, though rightfully it should be an equation. The first term is the speed at which a student can absorb already-discovered architectural knowledge. The second term is the speed at which a master can discover new knowledge. The third term represents the degree to which one must already be on the frontier of knowledge to make new discoveries; at zero, everyone discovers equally regardless of what they already know; at one, one must have mastered every previously-discovered fact before one can discover anything new. The fourth term represents potential for specialization; at one, it is impossible to understand any part without understanding the whole; at zero, it can be subdivided freely. The fifth…”
↑ comment by gwern · 2023-01-24T21:38:40.838Z · LW(p) · GW(p)
I don't think Scott had a specific concrete equation in mind. (I don't know of any myself, and Scott would likely have referenced or written it up on SSC/ACX by now if he had one in mind.) However, conceptually, it's just a variation on the rocket equation or jeep problem, I think.
comment by Thomas Kwa (thomas-kwa) · 2022-06-27T13:56:21.385Z · LW(p) · GW(p)
Showerthought: what's the simplest way to tell that the human body is less than 50% efficient at converting chemical energy to mechanical work via running? I think it's that running uphill makes you warmer than running downhill at the same speed.
When running up a hill at mechanical power p and efficiency f, you have to exert p/f total power and so p(1/f - 1) is dissipated as heat. When running down the hill you convert p to heat. p(1/f - 1) > p implies that f > 0.5.
Maybe this story is wrong somehow. I'm pretty sure your body has no way of recovering your potential energy on the way down; I'd expect most of the waste heat to go in your joints and muscles but maybe some of it goes into your shoes.
Replies from: ejacobcomment by Thomas Kwa (thomas-kwa) · 2022-06-25T20:17:48.363Z · LW(p) · GW(p)
Are there approximate versions of the selection theorems [LW · GW]? I haven't seen anyone talk about them, but they might be easy to prove.
Approximate version of Kelly criteron: any agent that follows a strategy different by at least epsilon from Kelly betting will almost surely lose money compared to a Kelly-betting agent at a rate f(epsilon)
Approximate version of VNM: Any agent that satisfies some weakened version of the VNM axioms will have high likelihood under Boltzmann rationality (or some other metric of approximate utility maximization). The closest thing I've seen is logical inductors.
Approximate version of good regulator theorem: any approximately optimal regulator is equivalent to something that approximately models variables in its environment
Probably there are others.
Ideally, the assumptions and/or conclusions would describe how agents like humans, companies, animals, and ML systems actually work.
comment by Thomas Kwa (thomas-kwa) · 2022-04-23T15:36:53.151Z · LW(p) · GW(p)
Is there somewhere I can find a graph of the number of AI alignment researchers vs AI capabilities researchers over time, from say 2005 to the present day?
comment by Thomas Kwa (thomas-kwa) · 2020-10-29T21:38:10.766Z · LW(p) · GW(p)
Is there software that would let me automatically switch between microphones on my computer when I put on my headset?
I imagine this might work as a piece of software that integrates all microphones connected to my computer into a single input device, then transmits the audio stream from the best-quality source.
A partial solution would be something that automatically switches to the headset microphone when I switch to the headset speakers.
Replies from: Dagon↑ comment by Dagon · 2020-10-30T00:03:22.723Z · LW(p) · GW(p)
Depending on connection method for your headset, you might be able to just use a simple switch. Mine is USB, and https://smile.amazon.com/dp/B00JX1ZS5O lets me just leave it disconnected when not in use. My Windows box uses the speakers when it's disconnected (I don't have a separate mic, but I expect it would work the same), and switches output and input to the headset when connected. I've seen similar switchers for 3.5mm audio connectors - I have no doubt they'd work for microphone instead of speaker, but I don't know any that combine them.
Replies from: thomas-kwa↑ comment by Thomas Kwa (thomas-kwa) · 2020-12-07T04:41:31.964Z · LW(p) · GW(p)
Thanks. I tried a couple different switches on my setup (3.5mm through USB-C hub), and the computer didn't disconnect upon opening the switch, so I'm giving up on this until I change hardware.